---
title: Cinemo
app_file: demo.py
sdk: gradio
sdk_version: 4.37.2
tags:
- Image-2-Video
- LLM
- Large Language Model
short_description: Multimodal Image-to-Video
emoji: 🎥
colorFrom: green
colorTo: indigo
---
## Cinemo: Consistent and Controllable Image Animation with Motion Diffusion Models
Official PyTorch Implementation
[](https://arxiv.org/abs/2407.15642)
[](https://maxin-cn.github.io/cinemo_project/)
This repo contains pre-trained weights, and sampling code for our paper exploring image animation with motion diffusion models (Cinemo). You can find more visualizations on our [project page](https://maxin-cn.github.io/cinemo_project/).
In this project, we propose a novel method called Cinemo, which can perform motion-controllable image animation with strong consistency and smoothness. To improve motion smoothness, Cinemo learns the distribution of motion residuals, rather than directly generating subsequent frames. Additionally, a structural similarity index-based method is proposed to control the motion intensity. Furthermore, we propose a noise refinement technique based on discrete cosine transformation to ensure temporal consistency. These three methods help Cinemo generate highly consistent, smooth, and motion-controlled image animation results. Compared to previous methods, Cinemo offers simpler and more precise user control and better generative performance.
## News
- (🔥 New) Jul. 23, 2024. 💥 Our paper is released on [arxiv](https://arxiv.org/abs/2407.15642).
- (🔥 New) Jun. 2, 2024. 💥 The inference code is released. The checkpoint can be found [here](https://huggingface.co/maxin-cn/Cinemo/tree/main).
## Setup
First, download and set up the repo:
```bash
git clone https://github.com/maxin-cn/Cinemo
cd Cinemo
```
We provide an [`environment.yml`](environment.yml) file that can be used to create a Conda environment. If you only want
to run pre-trained models locally on CPU, you can remove the `cudatoolkit` and `pytorch-cuda` requirements from the file.
```bash
conda env create -f environment.yml
conda activate cinemo
```
## Animation
You can sample from our **pre-trained Cinemo models** with [`animation.py`](pipelines/animation.py). Weights for our pre-trained Cinemo model can be found [here](https://huggingface.co/maxin-cn/Cinemo/tree/main). The script has various arguments for adjusting sampling steps, changing the classifier-free guidance scale, etc:
```bash
bash pipelines/animation.sh
```
All related checkpoints will download automatically and then you will get the following results,
Input image |
Output video |
Input image |
Output video |
 |
 |
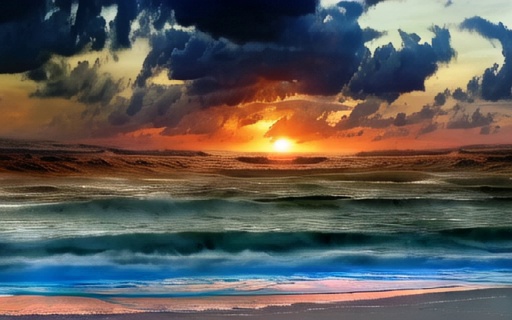 |
 |
"People Walking" |
"Sea Swell" |
 |
 |
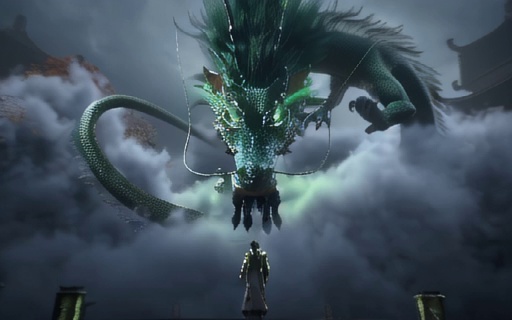 |
 |
"Girl Dancing under the Stars" |
"Dragon Glowing Eyes" |
## Other Applications
You can also utilize Cinemo for other applications, such as motion transfer and video editing:
```bash
bash pipelines/video_editing.sh
```
All related checkpoints will download automatically and you will get the following results,
Input video |
First frame |
Edited first frame |
Output video |
 |
 |
 |
 |
## Citation
If you find this work useful for your research, please consider citing it.
```bibtex
@article{ma2024cinemo,
title={Cinemo: Latent Diffusion Transformer for Video Generation},
author={Ma, Xin and Wang, Yaohui and Jia, Gengyun and Chen, Xinyuan and Li, Yuan-Fang and Chen, Cunjian and Qiao, Yu},
journal={arXiv preprint arXiv:2407.15642},
year={2024}
}
```
## Acknowledgments
Cinemo has been greatly inspired by the following amazing works and teams: [LaVie](https://github.com/Vchitect/LaVie) and [SEINE](https://github.com/Vchitect/SEINE), we thank all the contributors for open-sourcing.
## License
The code and model weights are licensed under [LICENSE](LICENSE).