File size: 15,945 Bytes
1dc1bad |
1 2 3 4 5 6 7 8 9 10 11 12 13 14 15 16 17 18 19 20 21 22 23 24 25 26 27 28 29 30 31 32 33 34 35 36 37 38 39 40 41 42 43 44 45 46 47 48 49 50 51 52 53 54 55 56 57 58 59 60 61 62 63 64 65 66 67 68 69 70 71 72 73 74 75 76 77 78 79 80 81 82 83 84 85 86 87 88 89 90 91 92 93 94 95 96 97 98 99 100 101 102 103 104 105 106 107 108 109 110 111 112 113 114 115 116 117 118 119 120 121 122 123 124 125 126 127 128 129 130 131 132 133 134 135 136 137 138 139 140 141 142 143 144 145 146 147 148 149 150 151 152 153 154 155 156 157 158 159 160 161 162 163 164 165 166 167 168 169 170 171 172 173 174 175 176 177 178 179 180 181 182 183 184 185 186 187 188 189 190 191 192 193 194 195 196 197 198 199 200 201 202 203 204 205 206 207 208 209 210 211 212 213 214 215 216 217 218 219 220 221 222 223 224 225 226 227 228 229 230 231 232 233 234 235 236 237 238 239 240 241 242 243 244 245 246 247 248 249 250 251 252 253 254 255 256 257 258 259 260 261 262 263 264 265 266 267 268 269 270 271 272 273 274 275 276 277 278 279 280 281 282 283 284 285 286 287 288 289 290 291 292 293 294 295 296 297 298 299 300 301 302 303 304 305 306 307 308 309 310 311 312 313 314 315 316 317 318 319 320 321 322 323 324 325 326 327 328 329 330 331 332 333 334 335 336 337 338 339 340 341 342 343 344 345 346 347 348 349 |
---
base_model: meta-llama/Llama-3.2-3B
library_name: transformers
datasets:
- lianghsun/tw-novel-1.1B
- lianghsun/tw-finance-159M
- lianghsun/tw-legal-news-24M
- lianghsun/tw-gov-news-90M
- lianghsun/tw-gov-556k
- lianghsun/tw-news-551M
- lianghsun/tw-health-43M
- lianghsun/tw-science-24M
- lianghsun/tw-book-43M
- lianghsun/tw-society-88M
- lianghsun/tw-law-article-evolution
- lianghsun/tw-processed-judgments
- lianghsun/tw-legal-methodology
- lianghsun/tw-legal-qa
- lianghsun/tw-judgment-gist
- lianghsun/reasoning-base-20k
- lianghsun/wikipedia-zh-filtered
- AWeirdDev/zh-tw-pts-articles-sm
- bhxiang/c4_calibrate_mini
- benchang1110/pretrainedtw
- benchang1110/sciencetw
- intfloat/multilingual_cc_news
language:
- zh
- en
license: llama3.2
tags:
- ROC
- Taiwan
- zh-tw
- llama-factory
new_version: lianghsun/Llama-3.2-Taiwan-3B-Instruct
pipeline_tag: text-generation
widget:
- text: 中華民國憲法第一條
---
[](https://hf.co/QuantFactory)
# QuantFactory/Llama-3.2-Taiwan-3B-GGUF
This is quantized version of [lianghsun/Llama-3.2-Taiwan-3B](https://huggingface.co/lianghsun/Llama-3.2-Taiwan-3B) created using llama.cpp
# Original Model Card
# Model Card for lianghsun/Llama-3.2-Taiwan-3B
<!-- Provide a quick summary of what the model is/does. -->
<a href="https://discord.gg/fj6WbHMvfs" target="_blank">[Discord]</a>
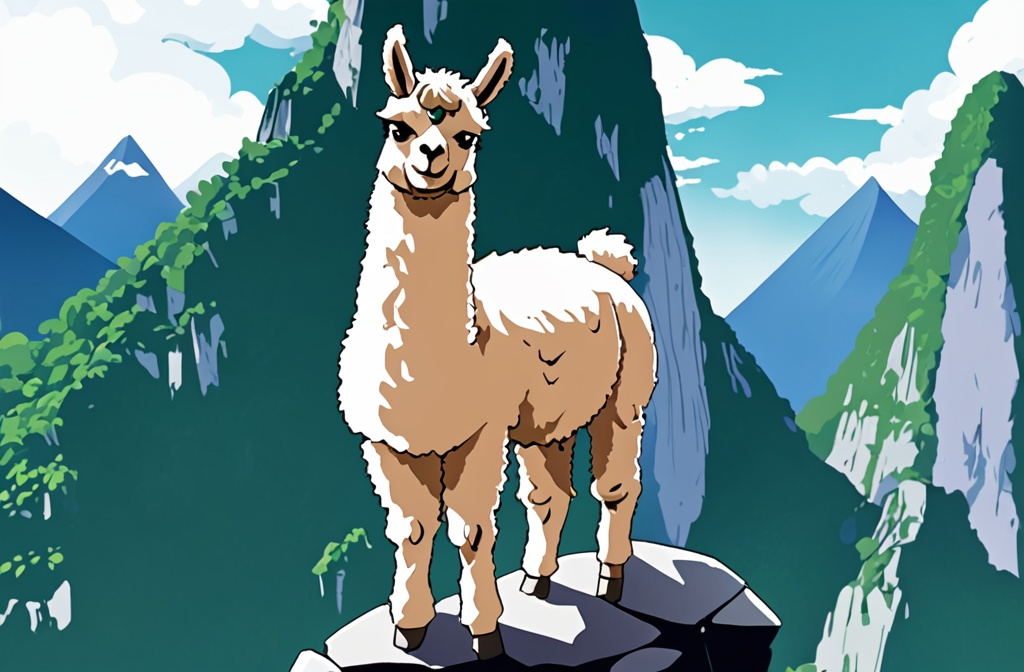
*圖像生成來自 [OpenArt](https://openart.ai/home):An anime-style 🦙 standing proudly atop the summit of Taiwan’s [Yushan (Jade Mountain)](https://zh.wikipedia.org/wiki/%E7%8E%89%E5%B1%B1), gazing forward.*
採用 [meta-llama/Llama-3.2-3B](https://huggingface.co/meta-llama/Llama-3.2-3B) 為[基礎模型(foundation model)](https://en.wikipedia.org/wiki/Foundation_model),使用大量[中華民國台灣](https://zh.wikipedia.org/zh-tw/%E8%87%BA%E7%81%A3)的繁體中文語料和多國語料進行模型[持績預訓練(continual pretraining, CPT)](https://docs.unsloth.ai/basics/continued-pretraining),旨在訓練出具有中華民國台灣知識及風格的[小語言模型(small langugae model, SLM)](https://www.ibm.com/think/topics/small-language-models)。
<details>
<summary><b>Model Change Log</b></summary>
| Update Date | Model Version | Key Changes |
|--------------|-----------------------|-------------------------------------|
| 2025-01-01 | v2024.12.28 | This version is trained on a larger corpus of Traditional Chinese texts and multilingual data. |
| 2024-11-15 | v2024.11.15 | The model continues training on multilingual and Traditional Chinese corpora. |
</details>
## Model Details
本模型基於 `meta-llama/Llama-3.2-3B` 持續預訓練,整合了大量繁體中文語料和多國語系語料。繁體中文語料主要來自中華民國政府官方與其他可信賴的繁體中文來源,確保語料純正,避免模型生成時出現繁簡中文用語混雜的情況。同時,此舉也使模型能更準確地貼近中華民國台灣社會的語言使用習慣。
採用小語言模型(相較於 7B+)的設計目標之一是降低硬體資源需求,即便在較少 GPU 硬體資源的環境中,也能讓使用者能更輕鬆地進行[指令微調(instruction fine-tuning)](https://www.ibm.com/topics/instruction-tuning)或部署推論端點(inference endpoint)。
> 需要大量繁體中文語料和高算力的部分,這個基礎模型已經處理好了,各位訓練家只要專注在微調的部分 💪。
*👋 如果你有更多公開資料想讓模型進行預訓練或者你對指令微調有什麼想法,也請歡迎來信討論。請不吝嗇給予 ❤️ 以支持此開源專案。以下提供本基礎模型的持續預訓練細節,協助各位減少探索所需的時間。*
### Model Description
<!-- Provide a longer summary of what this model is. -->
- **Developed by:** [Huang Liang Hsun](https://www.linkedin.com/in/lianghsunhuang)
- **Model type:** LlamaForCausalLM
- **Language(s) (NLP):** zh-tw
- **License:** [llama3.2](https://huggingface.co/meta-llama/Llama-3.2-1B/blob/main/LICENSE.txt)
- **Continual pre-trained from model:** [meta-llama/Llama-3.2-3B](https://huggingface.co/meta-llama/Llama-3.2-3B)
### Model Sources
<!-- Provide the basic links for the model. -->
- **Repository:** [lianghsun/Llama-3.2-Taiwan-3B](https://huggingface.co/lianghsun/Llama-3.2-Taiwan-3B)
- **Demo**:
```markdown
# v2024.11.15
prompt: 台灣是
text: 個有地震風險的地方,地震的地層不斷變化
prompt: 中華民國總統
text: 當選人賴清德今日抵台揭露住所。
prompt: 學校營養午餐
text: 之食物營養成分及食材數量評鑑手冊
```
## Uses
<!-- Address questions around how the model is intended to be used, including the foreseeable users of the model and those affected by the model. -->
### Direct Use
<!-- This section is for the model use without fine-tuning or plugging into a larger ecosystem/app. -->
本基礎模型透過大量繁體中文和多國語料進行持續預訓練,在不進行指令微調的前題下,你可以佈署此基礎模型觀察其對繁中語料的接龍程度,從中得知是否具備一定的繁中能力。
```markdown
# v2024.11.15
prompt: 刑法第一條
text: 規定之立法目的,以維護社會生活的和諧之
```
### Downstream Use
<!-- This section is for the model use when fine-tuned for a task, or when plugged into a larger ecosystem/app -->
本基礎模型透過大量繁體中文和多國語料進行持續預訓練,經檢查模型的輸出已經具有繁中用語能力,使用者可以以此基礎模型進行指令微調成具有指令回調的模型,以下是我們使用大量繁體中文指令集進行微調後的模型結果:
```markdown
# lianghsun/Llama-3.2-Taiwan-3B-Instruct (not released yet)
user: 請介紹台灣
assistant: 台灣,位於亞洲東部,地處太平洋與菲律賓海之間,面積約36,000平方公里,人口約2,300萬,是民主自由的國家,經濟實力強勁,擁有世界第10大經濟體。台灣以美食、文化、自然美景著稱,還有豐富的歷史與傳統,吸引全球遊客。台灣語為官方語言,但中文也廣為使用,英語也常用於國際交流。台灣政治多元,執政黨為民進黨,台灣是全球科技產業的重鎮,擁有先進的製造業與服務業。台灣氣候溫暖潮濕,四季分明,夏季炎熱,冬季涼爽,雨季則在5月至10月。台灣的美食以小吃為主,如滷肉飯、珍珠
```
### Out-of-Scope Use
<!-- This section addresses misuse, malicious use, and uses that the model will not work well for. -->
我們用來訓練此基礎模型的繁體中文資料集涵蓋許多不同年代,基礎模型如果在未微調的前題下,進一步詢問有關具有時效性的問題可能會得到非預期的結果,比如
```markdown
# v2024.11.15
prompt: 民法第184條
text: 第1項立法目的及易淹水事件開放安全窗 # 錯誤
```
## Bias, Risks, and Limitations
<!-- This section is meant to convey both technical and sociotechnical limitations. -->
雖然此模型已經透過大量繁體中文的薰陶,並且資料集中含有大量中華民國台灣政府的公開資訊,但仍可能出現時空背景錯誤、使用非台灣本地用語或其他誤植回覆的可能性(我們持績在補充更多的繁中語料)。使用者在參考這些資料時,應謹慎檢查生成的內容,並避免將模型輸出視為正確回覆。建議可以再透過 *持績預訓練* 或 *指令微調* 進一步降低前述的問題。
### Recommendations
<!-- This section is meant to convey recommendations with respect to the bias, risk, and technical limitations. -->
Users (both direct and downstream) should be made aware of the risks, biases and limitations of the model. More information needed for further recommendations.
## How to Get Started with the Model
<!-- Use the code below to get started with the model. -->
要使用 [vLLM Docker image](https://docs.vllm.ai/en/latest/serving/deploying_with_docker.html) 來啟動此模型,您可以按照以下操作:
```bash
docker run --runtime nvidia --gpus all \
-v ~/.cache/huggingface:/root/.cache/huggingface \
--env "HUGGING_FACE_HUB_TOKEN=<secret>" \
-p 8000:8000 \
--ipc=host \
vllm/vllm-openai:latest \
--model lianghsun/Llama-3.2-Taiwan-3B
```
請注意,如果想要使用不同版本的 checkpoint,請加上 `--revision <tag_name>`
```bash
docker run --runtime nvidia --gpus all \
-v ~/.cache/huggingface:/root/.cache/huggingface \
--env "HUGGING_FACE_HUB_TOKEN=<secret>" \
-p 8000:8000 \
--ipc=host \
vllm/vllm-openai:latest \
--model lianghsun/Llama-3.2-Taiwan-3B --revision <tag_name>
```
## Training Details
### Training Data
<!-- This should link to a Dataset Card, perhaps with a short stub of information on what the training data is all about as well as documentation related to data pre-processing or additional filtering. -->
<details>
<summary><b>繁體中文語料資料集</b></summary>
- [lianghsun/tw-novel-1.1B](https://huggingface.co/datasets/lianghsun/tw-novel-1.1B)
- [lianghsun/tw-finance-159M](https://huggingface.co/datasets/lianghsun/tw-finance-159M)
- [lianghsun/tw-legal-news-24M](https://huggingface.co/datasets/lianghsun/tw-legal-news-24M)
- [lianghsun/tw-gov-news-90M](https://huggingface.co/datasets/lianghsun/tw-gov-news-90M)
- [lianghsun/tw-news-408M](https://huggingface.co/datasets/lianghsun/tw-news-408M)
- [lianghsun/tw-health-36M](https://huggingface.co/datasets/lianghsun/tw-health-36M)
- [lianghsun/tw-law-article-evolution](https://huggingface.co/datasets/lianghsun/tw-law-article-evolution)
- [lianghsun/tw-processed-judgments](https://huggingface.co/datasets/lianghsun/tw-processed-judgments)
- [lianghsun/tw-legal-methodology](https://huggingface.co/datasets/lianghsun/tw-legal-methodology)
- [lianghsun/tw-legal-qa](https://huggingface.co/datasets/lianghsun/tw-legal-qa)
- [lianghsun/tw-judgment-gist](https://huggingface.co/datasets/lianghsun/tw-judgment-gist)
- [lianghsun/reasoning-base-20k](https://huggingface.co/datasets/lianghsun/reasoning-base-20k)
- [AWeirdDev/zh-tw-pts-articles-sm](https://huggingface.co/datasets/AWeirdDev/zh-tw-pts-articles-sm)
- [bhxiang/c4_calibrate_mini](https://huggingface.co/datasets/bhxiang/c4_calibrate_mini)
- [benchang1110/pretrainedtw](https://huggingface.co/datasets/benchang1110/pretrainedtw)
- [benchang1110/sciencetw](https://huggingface.co/datasets/benchang1110/sciencetw)
</details>
<details>
<summary><b>多國語系語料資料集</b></summary>
- [intfloat/multilingual_cc_news](https://huggingface.co/datasets/intfloat/multilingual_cc_news)
</details>
### Training Procedure
<!-- This relates heavily to the Technical Specifications. Content here should link to that section when it is relevant to the training procedure. -->
#### Preprocessing
1. **語料格式化:** 繁體中文資料集面臨一些格式化的問題,句子中如果有中英文混雜,或者更通用的情況是全型和半型的文字及標點符號混合時,如果資料來源處未加注意,格式往往會是全型半型符號各種交錯使用、中文句子中間用半型逗號相接、中英文直接連在一起,這些源頭可能源自於從小使用 Microsoft Word 的習慣,因為 Word 會在「視覺上」幫中英文之間加上空格,導致繁體中文地區的撰寫者習慣會忽略在適當的位置加上空格及全型半型文字交錯時的正確格式,我們在此稱之為「不工整格式」。上述問題會導致採用如此的資料集的語言模型學到不工整的輸出,比如:
```markdown
# 不工整文本
- 你好嗎Jack這是我們的10c.c
- 他說:"Hello, how are you?"
```
為了解決這個問題,我們撰寫一個負責格式化文本的管道(pipeline)處理全型半型混合語料。
```markdown
# 格式化後的文本
- 你好嗎 Jack 這是我們的 10 c.c
- 他說:"Hello, how are you?"
```
2. **截斷長度:** 在選擇資料時,我們直接去除大於訓練模型的截斷長度(cutoff length)的文本,讓每一筆訓練的文本是完整的句子。基於上述觀察,我們將這個模型的截斷長度設置為 `4096`,並取總 token 數量小於 `4096` 文本。請注意這裡我們是以 `llama-3.2-*B` 的切詞器(tokenizer)去計算總 token 數量。
#### Training Hyperparameters
- **learning_rate:** 5e-6
- **min_learning_rate:** 5e-7
- **train_batch_size:** 8
- **eval_batch_size:** 4
- **seed:** 42
- **distributed_type:** single-node
- **num_devices:** 4
- **gradient_accumulation_steps:** 50
- **total_train_batch_size:** 1,600 (train_batch_size * gradient_accumulation_steps * num_devices)
- **optimizer:** adamw_torch_fused
- **lr_scheduler_type:** cosine
- **lr_scheduler_warmup_ratio:** 0.01
- **num_epochs:** 10
- **grad_norm:** 0.14920360027631302
- **global_step:** 2,770
#### Speeds, Sizes, Times
<!-- This section provides information about throughput, start/end time, checkpoint size if relevant, etc. -->
- **Duration**: 8d 1h 46m 24s
- **Train runtime**: 697,584.9221
- **Train samples per second**: 6.376
- **Train steps per second**: 0.004
- **Total training FLOPs**: 18,625,327,792,128,000
- **Train loss**: 1.7512
## Environmental Impact
<!-- Total emissions (in grams of CO2eq) and additional considerations, such as electricity usage, go here. Edit the suggested text below accordingly -->
- **Hardware Type:** 🚀
- **Hours used:** ⏳⏳⌛
- **Cloud Provider:** [鴻鵠國際股份有限公司](https://www.honghutech.com/)
- **Compute Region:** 🇹🇼
- **Carbon Emitted:** ♻️
## Technical Specifications [optional]
### Model Architecture and Objective
[More Information Needed]
### Compute Infrastructure
[More Information Needed]
#### Hardware
[More Information Needed]
#### Software
[More Information Needed]
## Citation
<!-- If there is a paper or blog post introducing the model, the APA and Bibtex information for that should go in this section. -->
```
@misc{
lianghsun2024llama32taiwan3b,
author = {Huang, Liang Hsun},
title = {Llama-3.2-Taiwan-3B},
year = {2024},
publisher = {Hugging Face},
howpublished = {\url{https://huggingface.co/lianghsun/Llama-3.2-Taiwan-3B}},
note = {Accessed: 2024-11-25}
}
```
## Glossary
<!-- If relevant, include terms and calculations in this section that can help readers understand the model or model card. -->
N/A
## More Information
### Acknowledge

在此致謝[鴻鵠國際股份有限公司](https://www.honghutech.com/)蔡長明先生無償地贊助算力,以及曾經幫忙過:廖振翔、chweng、Ben、kevin、Maxxchu、Lam 和陳林彥…等朋友們,才能讓這個模型得以訓練完成,提供算力者乃人生父母。
### Usage
如果你基於此基礎模型進行微調,希望能不吝嗇在 **模型卡片(model card)** 裡標註 **基礎模型** 為:
```
base_model: lianghsun/Llama-3.2-Taiwan-3B
```
標註和 ❤️ 是給予我們最大的鼓勵,謝謝。😀
## Model Card Authors
[Huang Liang Hsun](https://www.linkedin.com/in/lianghsunhuang)
## Model Card Contact
[Huang Liang Hsun](https://www.linkedin.com/in/lianghsunhuang)
### Framework versions
- Transformers 4.45.2
- Pytorch 2.4.1+cu121
- Datasets 2.21.0
- Tokenizers 0.20.0
|