Join the conversation
Join the community of Machine Learners and AI enthusiasts.
Sign UpAll HF Hub posts
Post
2963
The Chinese community is shipping ๐ข
DeepSeek V3 (685 B MoE) has quietly released on the hub!
Base: deepseek-ai/DeepSeek-V3-Base
Instruct: deepseek-ai/DeepSeek-V3
Canโt wait to see whatโs next!
DeepSeek V3 (685 B MoE) has quietly released on the hub!
Base: deepseek-ai/DeepSeek-V3-Base
Instruct: deepseek-ai/DeepSeek-V3
Canโt wait to see whatโs next!
Post
3864
๐ฌ Revolutionize Your Video Creation
Dokdo Multimodal AI Transform a single image into a stunning video with perfect audio harmony! ๐
Superior Technology ๐ซ
Advanced Flow Matching: Smoother video transitions surpassing Kling and Sora
Intelligent Sound System: Automatically generates perfect audio by analyzing video mood
Multimodal Framework: Advanced AI integrating image, text, and audio analysis
Outstanding Performance ๐ฏ
Ultra-High Resolution: 4K video quality with bfloat16 acceleration
Real-Time Optimization: 3x faster processing with PyTorch GPU acceleration
Smart Sound Matching: Real-time audio effects based on scene transitions and motion
Exceptional Features โจ
Custom Audio Creation: Natural soundtrack matching video tempo and rhythm
Intelligent Watermarking: Adaptive watermark adjusting to video characteristics
Multilingual Support: Precise translation engine powered by Helsinki-NLP
Versatile Applications ๐
Social Media Marketing: Create engaging shorts for Instagram and YouTube
Product Promotion: Dynamic promotional videos highlighting product features
Educational Content: Interactive learning materials with enhanced engagement
Portfolio Enhancement: Professional-grade videos showcasing your work
Experience the video revolution with Dokdo Multimodal, where anyone can create professional-quality content from a single image. Elevate your content with perfectly synchronized video and audio that captivates your audience! ๐จ
Start creating stunning videos that stand out from the crowd - whether you're a marketer, educator, content creator, or business owner. Join the future of AI-powered video creation today!
ginipick/Dokdo-multimodal
#VideoInnovation #AITechnology #PremiumContent #MarketingSolution
๐ Please turn on your sound for the best viewing experience!
Dokdo Multimodal AI Transform a single image into a stunning video with perfect audio harmony! ๐
Superior Technology ๐ซ
Advanced Flow Matching: Smoother video transitions surpassing Kling and Sora
Intelligent Sound System: Automatically generates perfect audio by analyzing video mood
Multimodal Framework: Advanced AI integrating image, text, and audio analysis
Outstanding Performance ๐ฏ
Ultra-High Resolution: 4K video quality with bfloat16 acceleration
Real-Time Optimization: 3x faster processing with PyTorch GPU acceleration
Smart Sound Matching: Real-time audio effects based on scene transitions and motion
Exceptional Features โจ
Custom Audio Creation: Natural soundtrack matching video tempo and rhythm
Intelligent Watermarking: Adaptive watermark adjusting to video characteristics
Multilingual Support: Precise translation engine powered by Helsinki-NLP
Versatile Applications ๐
Social Media Marketing: Create engaging shorts for Instagram and YouTube
Product Promotion: Dynamic promotional videos highlighting product features
Educational Content: Interactive learning materials with enhanced engagement
Portfolio Enhancement: Professional-grade videos showcasing your work
Experience the video revolution with Dokdo Multimodal, where anyone can create professional-quality content from a single image. Elevate your content with perfectly synchronized video and audio that captivates your audience! ๐จ
Start creating stunning videos that stand out from the crowd - whether you're a marketer, educator, content creator, or business owner. Join the future of AI-powered video creation today!
ginipick/Dokdo-multimodal
#VideoInnovation #AITechnology #PremiumContent #MarketingSolution
๐ Please turn on your sound for the best viewing experience!
Post
844
๐ DeepSeek ๐v3 achieves a solid 7 point jump than v2.5, surpassing GPT-4o, but is still behind ๐ o1 ๐and Claude 3.5.
onekq-ai/WebApp1K-models-leaderboard
onekq-ai/WebApp1K-models-leaderboard
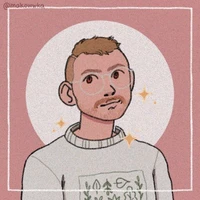
davanstrienย
posted an update
1 day ago
Post
1786
๐ธ๐ฐ Hovorte po slovensky? Help build better AI for Slovak!
We only need 90 more annotations to include Slovak in the next Hugging Face FineWeb2-C dataset ( data-is-better-together/fineweb-c) release!
Your contribution will help create better language models for 5+ million Slovak speakers.
Annotate here: data-is-better-together/fineweb-c.
Read more about why we're doing it: https://huggingface.co/blog/davanstrien/fineweb2-community
We only need 90 more annotations to include Slovak in the next Hugging Face FineWeb2-C dataset ( data-is-better-together/fineweb-c) release!
Your contribution will help create better language models for 5+ million Slovak speakers.
Annotate here: data-is-better-together/fineweb-c.
Read more about why we're doing it: https://huggingface.co/blog/davanstrien/fineweb2-community
Post
1272
CS2 Highlights Video Dataset -
nyuuzyou/cs2-highlights
A collection of 4,857 high-quality Counter-Strike 2 gameplay highlights featuring:
- Professional and competitive gameplay recordings at 1080p resolution
- Complete metadata including Steam IDs and clip titles
- Preview thumbnails for all videos
- Both 60 FPS (842 clips) and 120 FPS (4,015 clips) content
- Gameplay from Faceit and official competitive modes
This extensive highlights collection provides a valuable resource for developing and evaluating video-based AI applications, especially in esports and competitive gaming contexts. Released under Creative Commons Zero (CC0) license.
A collection of 4,857 high-quality Counter-Strike 2 gameplay highlights featuring:
- Professional and competitive gameplay recordings at 1080p resolution
- Complete metadata including Steam IDs and clip titles
- Preview thumbnails for all videos
- Both 60 FPS (842 clips) and 120 FPS (4,015 clips) content
- Gameplay from Faceit and official competitive modes
This extensive highlights collection provides a valuable resource for developing and evaluating video-based AI applications, especially in esports and competitive gaming contexts. Released under Creative Commons Zero (CC0) license.
Post
1346
๐ข Deligted to share the most recent milestone on quick deployment of Named Entity Recognition (NER) in Gen-AI powered systems.
Releasing the bulk-ner 0.25.0 which represent a tiny framework that would save you time for deploing NER with any model.
๐ Why is this important? In the era of GenAI the handling out textual output might be challenging. Instead, recognizing named-entities via domain-oriented systems for your donwstream LLM would be preferable option.
๐ฆ: https://pypi.org/project/bulk-ner/0.25.0/
๐: https://github.com/nicolay-r/bulk-ner
I noticed that the direct adaptaion of the LM for NER would result in spending signifcant amount of time on formatting your texts according to the NER-model needs.
In particular:
1. Processing CONLL format with B-I-O tags from model outputs
2. Input trimming: long input content might not be completely fitted
To cope with these problems, in version 0.25.0 I made a huge steps forward by providing:
โ ๐ Python API support: see screenshot below for a quick deployment (see screenshot below ๐ธ)
โ ๐ชถ No-string: dependencies are now clear, so it is purely Python implementation for API calls.
โ ๐ Simplified output formatting: we use lists to represent texts with inner lists that refer to annotated objects (see screenshot below ๐ธ)
๐ We have a colab for a quick start here (or screenshot for bash / Python API ๐ธ)
https://colab.research.google.com/github/nicolay-r/ner-service/blob/main/NER_annotation_service.ipynb
๐ The code for pipeline deployment is taken from the AREkit project:
https://github.com/nicolay-r/AREkit
Releasing the bulk-ner 0.25.0 which represent a tiny framework that would save you time for deploing NER with any model.
๐ Why is this important? In the era of GenAI the handling out textual output might be challenging. Instead, recognizing named-entities via domain-oriented systems for your donwstream LLM would be preferable option.
๐ฆ: https://pypi.org/project/bulk-ner/0.25.0/
๐: https://github.com/nicolay-r/bulk-ner
I noticed that the direct adaptaion of the LM for NER would result in spending signifcant amount of time on formatting your texts according to the NER-model needs.
In particular:
1. Processing CONLL format with B-I-O tags from model outputs
2. Input trimming: long input content might not be completely fitted
To cope with these problems, in version 0.25.0 I made a huge steps forward by providing:
โ ๐ Python API support: see screenshot below for a quick deployment (see screenshot below ๐ธ)
โ ๐ชถ No-string: dependencies are now clear, so it is purely Python implementation for API calls.
โ ๐ Simplified output formatting: we use lists to represent texts with inner lists that refer to annotated objects (see screenshot below ๐ธ)
๐ We have a colab for a quick start here (or screenshot for bash / Python API ๐ธ)
https://colab.research.google.com/github/nicolay-r/ner-service/blob/main/NER_annotation_service.ipynb
๐ The code for pipeline deployment is taken from the AREkit project:
https://github.com/nicolay-r/AREkit
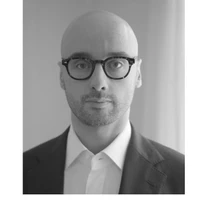
AkimfromParisย
posted an update
2 days ago
Post
1446
๐บ๐ธ ๐จ๐ฆ ๐ฌ๐ง Nobel Prize winners against USSR & Japanese AI pioneers โญ๐ฏ๐ต
๐ฉ๐ช Prof. Jรผrgen Schmidhuber:ย โThe #NobelPrize in Physics 2024 for Hopfield & Hinton turns out to be a Nobel Prize for plagiarism. They republished methodologies developed in #Ukraine and #Japan by Ivakhnenko and Amari in the 1960s & 1970s, as well as other techniques, without citing the original inventors.โ
1965 - First Deep Learning - USSR โญ (Ukraine ๐บ๐ฆ now)
Ivakhnenko and Lapa introduced the first deep learning in deep MLPs that learn internal representations of input data.
1967/68 - Deep Learning by Stochasticย Gradient Descent - Japan ๐ฏ๐ต
Shun-Ichi Amari trained MLPs with many layers in non-incremental end-to-end fashion from scratch by stochastic gradient descent (SGD).
1969 - Rectified linear unit - Japan ๐ฏ๐ต
In 1969, Kunihiko Fukushima introduced ReLU in the context of visual feature extraction in hierarchical neural networks.
1970 - Backpropagation - Finland ๐ซ๐ฎ ๐
In 1970, Seppo Linnainmaa was the first the reverse mode of automatic differentiation, now known as backpropagation.
1972 - Recurrent Neural Network - Japan ๐ฏ๐ต
In 1972, Shun-Ichi Amari published a learning recurrent neural network based on Lenz-Ising model (Amari's net was later called the "Hopfield network". Hopfield republished in 1982, without citing Amari papers.)
1979 - First Convolutional neural network - Japan ๐ฏ๐ต
CNN architecture was introduced in 1979 by Kunihiko Fukushima, also known as Neocognitron.
https://people.idsia.ch/~juergen/deep-learning-history.html#AMH2
๐ฉ๐ช Prof. Jรผrgen Schmidhuber:ย โThe #NobelPrize in Physics 2024 for Hopfield & Hinton turns out to be a Nobel Prize for plagiarism. They republished methodologies developed in #Ukraine and #Japan by Ivakhnenko and Amari in the 1960s & 1970s, as well as other techniques, without citing the original inventors.โ
1965 - First Deep Learning - USSR โญ (Ukraine ๐บ๐ฆ now)
Ivakhnenko and Lapa introduced the first deep learning in deep MLPs that learn internal representations of input data.
1967/68 - Deep Learning by Stochasticย Gradient Descent - Japan ๐ฏ๐ต
Shun-Ichi Amari trained MLPs with many layers in non-incremental end-to-end fashion from scratch by stochastic gradient descent (SGD).
1969 - Rectified linear unit - Japan ๐ฏ๐ต
In 1969, Kunihiko Fukushima introduced ReLU in the context of visual feature extraction in hierarchical neural networks.
1970 - Backpropagation - Finland ๐ซ๐ฎ ๐
In 1970, Seppo Linnainmaa was the first the reverse mode of automatic differentiation, now known as backpropagation.
1972 - Recurrent Neural Network - Japan ๐ฏ๐ต
In 1972, Shun-Ichi Amari published a learning recurrent neural network based on Lenz-Ising model (Amari's net was later called the "Hopfield network". Hopfield republished in 1982, without citing Amari papers.)
1979 - First Convolutional neural network - Japan ๐ฏ๐ต
CNN architecture was introduced in 1979 by Kunihiko Fukushima, also known as Neocognitron.
https://people.idsia.ch/~juergen/deep-learning-history.html#AMH2
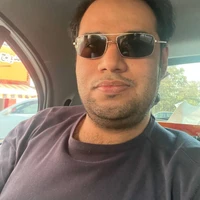
Muhammadrezaย
posted an update
2 days ago