Upload new k-quant GGML quantised models.
Browse files
README.md
CHANGED
@@ -1,18 +1,8 @@
|
|
1 |
---
|
2 |
-
license: other
|
3 |
-
language:
|
4 |
-
- en
|
5 |
-
library_name: transformers
|
6 |
inference: false
|
7 |
-
|
8 |
-
tags:
|
9 |
-
- gpt
|
10 |
-
- llm
|
11 |
-
- large language model
|
12 |
-
- LLaMa
|
13 |
-
datasets:
|
14 |
-
- h2oai/h2ogpt-oig-oasst1-instruct-cleaned-v2
|
15 |
---
|
|
|
16 |
<!-- header start -->
|
17 |
<div style="width: 100%;">
|
18 |
<img src="https://i.imgur.com/EBdldam.jpg" alt="TheBlokeAI" style="width: 100%; min-width: 400px; display: block; margin: auto;">
|
@@ -27,56 +17,89 @@ datasets:
|
|
27 |
</div>
|
28 |
<!-- header end -->
|
29 |
|
30 |
-
#
|
31 |
|
32 |
-
These files are GGML format model files
|
33 |
|
34 |
-
GGML files are for CPU inference using [llama.cpp](https://github.com/ggerganov/llama.cpp)
|
|
|
|
|
|
|
|
|
|
|
35 |
|
36 |
## Repositories available
|
37 |
|
38 |
-
* [
|
39 |
-
* [
|
40 |
-
* [
|
|
|
|
|
|
|
|
|
|
|
|
|
|
|
|
|
|
|
|
|
|
|
|
|
|
|
41 |
|
42 |
-
|
43 |
|
44 |
-
|
45 |
|
46 |
-
|
|
|
|
|
|
|
|
|
|
|
|
|
|
|
|
|
|
|
47 |
|
48 |
-
For files compatible with the previous version of llama.cpp, please see branch `previous_llama_ggmlv2`.
|
49 |
## Provided files
|
50 |
-
| Name | Quant method | Bits | Size | RAM required | Use case |
|
51 |
| ---- | ---- | ---- | ---- | ---- | ----- |
|
52 |
-
|
53 |
-
|
54 |
-
|
55 |
-
|
56 |
-
|
|
|
|
|
|
|
|
|
|
|
|
|
|
|
|
|
|
|
|
|
|
|
|
|
57 |
|
58 |
## How to run in `llama.cpp`
|
59 |
|
60 |
I use the following command line; adjust for your tastes and needs:
|
61 |
|
62 |
```
|
63 |
-
./main -t
|
64 |
-
### Instruction:
|
65 |
-
Write a story about llamas
|
66 |
-
### Response:"
|
67 |
```
|
68 |
-
Change `-t
|
|
|
|
|
69 |
|
70 |
If you want to have a chat-style conversation, replace the `-p <PROMPT>` argument with `-i -ins`
|
71 |
|
72 |
## How to run in `text-generation-webui`
|
73 |
|
74 |
-
GGML models can be loaded into text-generation-webui by installing the llama.cpp module, then placing the ggml model file in a model folder as usual.
|
75 |
-
|
76 |
Further instructions here: [text-generation-webui/docs/llama.cpp-models.md](https://github.com/oobabooga/text-generation-webui/blob/main/docs/llama.cpp-models.md).
|
77 |
|
78 |
-
Note: at this time text-generation-webui may not support the new May 19th llama.cpp quantisation methods for q4_0, q4_1 and q8_0 files.
|
79 |
-
|
80 |
<!-- footer start -->
|
81 |
## Discord
|
82 |
|
@@ -97,230 +120,23 @@ Donaters will get priority support on any and all AI/LLM/model questions and req
|
|
97 |
* Patreon: https://patreon.com/TheBlokeAI
|
98 |
* Ko-Fi: https://ko-fi.com/TheBlokeAI
|
99 |
|
100 |
-
**
|
|
|
|
|
101 |
|
102 |
Thank you to all my generous patrons and donaters!
|
|
|
103 |
<!-- footer end -->
|
104 |
|
105 |
-
# Original
|
|
|
|
|
106 |
## Summary
|
107 |
|
108 |
H2O.ai's `h2oai/h2ogpt-research-oig-oasst1-512-30b` is a 30 billion parameter instruction-following large language model for research use only.
|
109 |
|
110 |
-
|
111 |
-
|
112 |
-
-
|
113 |
-
- Fine-tuning dataset: [h2oai/h2ogpt-oig-oasst1-instruct-cleaned-v2](https://huggingface.co/datasets/h2oai/h2ogpt-oig-oasst1-instruct-cleaned-v2)
|
114 |
-
- Data-prep and fine-tuning code: [H2O.ai GitHub](https://github.com/h2oai/h2ogpt)
|
115 |
-
- Training logs: [zip](https://huggingface.co/h2oai/h2ogpt-research-oig-oasst1-512-30b/blob/main/llama-30b-hf.h2oaih2ogpt-oig-oasst1-instruct-cleaned-v2.2.0_epochs.131f6d098b43236b5f91e76fc074ad089d6df368.llama30b_17.zip)
|
116 |
-
|
117 |
-
The model was trained using h2oGPT code as:
|
118 |
-
|
119 |
-
```python
|
120 |
-
torchrun --nproc_per_node=8 finetune.py --base_model=decapoda-research/llama-30b-hf --micro_batch_size=1 --batch_size=8 --cutoff_len=512 --num_epochs=2.0 --val_set_size=0 --eval_steps=100000 --save_steps=17000 --save_total_limit=20 --prompt_type=plain --save_code=True --train_8bit=False --run_id=llama30b_17 --llama_flash_attn=True --lora_r=64 --lora_target_modules=['q_proj', 'k_proj', 'v_proj', 'o_proj'] --learning_rate=2e-4 --lora_alpha=32 --drop_truncations=True --data_path=h2oai/h2ogpt-oig-oasst1-instruct-cleaned-v2 --data_mix_in_path=h2oai/openassistant_oasst1_h2ogpt --data_mix_in_factor=1.0 --data_mix_in_prompt_type=plain --data_mix_in_col_dict={'input': 'input'}
|
121 |
-
```
|
122 |
-
On h2oGPT Hash: 131f6d098b43236b5f91e76fc074ad089d6df368
|
123 |
-
|
124 |
-
Only the last checkpoint at epoch 2.0 and step 137,846 is provided in this model repository because the LORA state is large enough and there are enough checkpoints to make total run 19GB. Feel free to request additional checkpoints and we can consider adding more.
|
125 |
-
|
126 |
-
## Chatbot
|
127 |
-
|
128 |
-
- Run your own chatbot: [H2O.ai GitHub](https://github.com/h2oai/h2ogpt)
|
129 |
-
[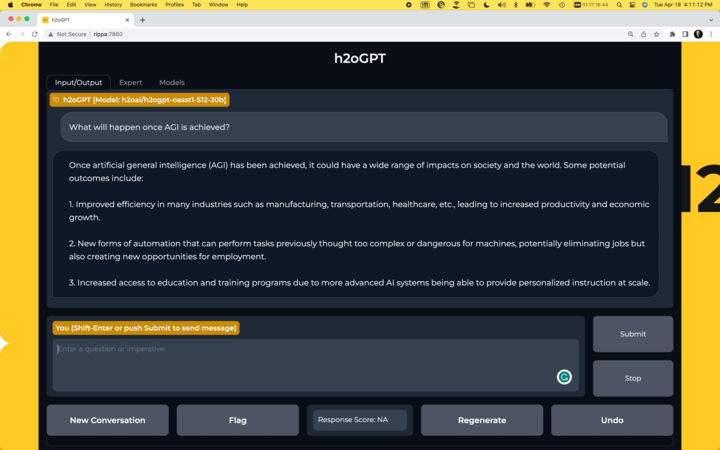](https://github.com/h2oai/h2ogpt)
|
130 |
-
|
131 |
-
## Usage:
|
132 |
-
|
133 |
-
### Usage as LORA:
|
134 |
-
|
135 |
-
### Build HF model:
|
136 |
-
|
137 |
-
Use: https://github.com/h2oai/h2ogpt/blob/main/export_hf_checkpoint.py and change:
|
138 |
-
|
139 |
-
```python
|
140 |
-
BASE_MODEL = 'decapoda-research/llama-30b-hf'
|
141 |
-
LORA_WEIGHTS = '<lora_weights_path>'
|
142 |
-
OUTPUT_NAME = "local_h2ogpt-research-oasst1-512-30b"
|
143 |
-
```
|
144 |
-
where `<lora_weights_path>` is a directory of some name that contains the files in this HF model repository:
|
145 |
-
|
146 |
-
* adapter_config.json
|
147 |
-
* adapter_model.bin
|
148 |
-
* special_tokens_map.json
|
149 |
-
* tokenizer.model
|
150 |
-
* tokenizer_config.json
|
151 |
-
|
152 |
-
Once the HF model is built, to use the model with the `transformers` library on a machine with GPUs, first make sure you have the `transformers` and `accelerate` libraries installed.
|
153 |
-
|
154 |
-
```bash
|
155 |
-
pip install transformers==4.28.1
|
156 |
-
pip install accelerate==0.18.0
|
157 |
-
```
|
158 |
-
|
159 |
-
```python
|
160 |
-
import torch
|
161 |
-
from transformers import pipeline
|
162 |
-
|
163 |
-
generate_text = pipeline(model="local_h2ogpt-research-oasst1-512-30b", torch_dtype=torch.bfloat16, trust_remote_code=True, device_map="auto")
|
164 |
-
|
165 |
-
res = generate_text("Why is drinking water so healthy?", max_new_tokens=100)
|
166 |
-
print(res[0]["generated_text"])
|
167 |
-
```
|
168 |
-
|
169 |
-
Alternatively, if you prefer to not use `trust_remote_code=True` you can download [instruct_pipeline.py](https://huggingface.co/h2oai/h2ogpt-oasst1-512-20b/blob/main/h2oai_pipeline.py),
|
170 |
-
store it alongside your notebook, and construct the pipeline yourself from the loaded model and tokenizer:
|
171 |
-
|
172 |
-
```python
|
173 |
-
import torch
|
174 |
-
from h2oai_pipeline import H2OTextGenerationPipeline
|
175 |
-
from transformers import AutoModelForCausalLM, AutoTokenizer
|
176 |
-
|
177 |
-
tokenizer = AutoTokenizer.from_pretrained("local_h2ogpt-research-oasst1-512-30b", padding_side="left")
|
178 |
-
model = AutoModelForCausalLM.from_pretrained("local_h2ogpt-research-oasst1-512-30b", torch_dtype=torch.bfloat16, device_map="auto")
|
179 |
-
generate_text = H2OTextGenerationPipeline(model=model, tokenizer=tokenizer)
|
180 |
-
|
181 |
-
res = generate_text("Why is drinking water so healthy?", max_new_tokens=100)
|
182 |
-
print(res[0]["generated_text"])
|
183 |
-
```
|
184 |
-
|
185 |
-
## Model Architecture with LORA and flash attention
|
186 |
-
|
187 |
-
```
|
188 |
-
PeftModelForCausalLM(
|
189 |
-
(base_model): LoraModel(
|
190 |
-
(model): LlamaForCausalLM(
|
191 |
-
(model): LlamaModel(
|
192 |
-
(embed_tokens): Embedding(32000, 6656, padding_idx=31999)
|
193 |
-
(layers): ModuleList(
|
194 |
-
(0-59): 60 x LlamaDecoderLayer(
|
195 |
-
(self_attn): LlamaAttention(
|
196 |
-
(q_proj): Linear(
|
197 |
-
in_features=6656, out_features=6656, bias=False
|
198 |
-
(lora_dropout): ModuleDict(
|
199 |
-
(default): Dropout(p=0.05, inplace=False)
|
200 |
-
)
|
201 |
-
(lora_A): ModuleDict(
|
202 |
-
(default): Linear(in_features=6656, out_features=64, bias=False)
|
203 |
-
)
|
204 |
-
(lora_B): ModuleDict(
|
205 |
-
(default): Linear(in_features=64, out_features=6656, bias=False)
|
206 |
-
)
|
207 |
-
)
|
208 |
-
(k_proj): Linear(
|
209 |
-
in_features=6656, out_features=6656, bias=False
|
210 |
-
(lora_dropout): ModuleDict(
|
211 |
-
(default): Dropout(p=0.05, inplace=False)
|
212 |
-
)
|
213 |
-
(lora_A): ModuleDict(
|
214 |
-
(default): Linear(in_features=6656, out_features=64, bias=False)
|
215 |
-
)
|
216 |
-
(lora_B): ModuleDict(
|
217 |
-
(default): Linear(in_features=64, out_features=6656, bias=False)
|
218 |
-
)
|
219 |
-
)
|
220 |
-
(v_proj): Linear(
|
221 |
-
in_features=6656, out_features=6656, bias=False
|
222 |
-
(lora_dropout): ModuleDict(
|
223 |
-
(default): Dropout(p=0.05, inplace=False)
|
224 |
-
)
|
225 |
-
(lora_A): ModuleDict(
|
226 |
-
(default): Linear(in_features=6656, out_features=64, bias=False)
|
227 |
-
)
|
228 |
-
(lora_B): ModuleDict(
|
229 |
-
(default): Linear(in_features=64, out_features=6656, bias=False)
|
230 |
-
)
|
231 |
-
)
|
232 |
-
(o_proj): Linear(
|
233 |
-
in_features=6656, out_features=6656, bias=False
|
234 |
-
(lora_dropout): ModuleDict(
|
235 |
-
(default): Dropout(p=0.05, inplace=False)
|
236 |
-
)
|
237 |
-
(lora_A): ModuleDict(
|
238 |
-
(default): Linear(in_features=6656, out_features=64, bias=False)
|
239 |
-
)
|
240 |
-
(lora_B): ModuleDict(
|
241 |
-
(default): Linear(in_features=64, out_features=6656, bias=False)
|
242 |
-
)
|
243 |
-
)
|
244 |
-
(rotary_emb): LlamaRotaryEmbedding()
|
245 |
-
)
|
246 |
-
(mlp): LlamaMLP(
|
247 |
-
(gate_proj): Linear(in_features=6656, out_features=17920, bias=False)
|
248 |
-
(down_proj): Linear(in_features=17920, out_features=6656, bias=False)
|
249 |
-
(up_proj): Linear(in_features=6656, out_features=17920, bias=False)
|
250 |
-
(act_fn): SiLUActivation()
|
251 |
-
)
|
252 |
-
(input_layernorm): LlamaRMSNorm()
|
253 |
-
(post_attention_layernorm): LlamaRMSNorm()
|
254 |
-
)
|
255 |
-
)
|
256 |
-
(norm): LlamaRMSNorm()
|
257 |
-
)
|
258 |
-
(lm_head): Linear(in_features=6656, out_features=32000, bias=False)
|
259 |
-
)
|
260 |
-
)
|
261 |
-
)
|
262 |
-
trainable params: 204472320 || all params: 32733415936 || trainable%: 0.6246592790675496
|
263 |
-
```
|
264 |
-
|
265 |
-
## Model Configuration
|
266 |
-
|
267 |
-
```json
|
268 |
-
{
|
269 |
-
"base_model_name_or_path": "decapoda-research/llama-30b-hf",
|
270 |
-
"bias": "none",
|
271 |
-
"fan_in_fan_out": false,
|
272 |
-
"inference_mode": true,
|
273 |
-
"init_lora_weights": true,
|
274 |
-
"lora_alpha": 32,
|
275 |
-
"lora_dropout": 0.05,
|
276 |
-
"modules_to_save": null,
|
277 |
-
"peft_type": "LORA",
|
278 |
-
"r": 64,
|
279 |
-
"target_modules": [
|
280 |
-
"q_proj",
|
281 |
-
"k_proj",
|
282 |
-
"v_proj",
|
283 |
-
"o_proj"
|
284 |
-
],
|
285 |
-
"task_type": "CAUSAL_LM"
|
286 |
-
```
|
287 |
-
|
288 |
-
## Model Validation
|
289 |
-
|
290 |
-
Classical benchmarks align with base LLaMa 30B model, but are not useful for conversational purposes. One could use GPT3.5 or GPT4 to evaluate responses, while here we use a [RLHF based reward model](OpenAssistant/reward-model-deberta-v3-large-v2). This is run using h2oGPT:
|
291 |
-
|
292 |
-
```python
|
293 |
-
python generate.py --base_model=decapoda-research/llama-30b-hf --gradio=False --infer_devices=False --eval_sharegpt_prompts_only=100 --eval_sharegpt_as_output=False --lora_weights=llama-30b-hf.h2oaih2ogpt-oig-oasst1-instruct-cleaned-v2.2.0_epochs.131f6d098b43236b5f91e76fc074ad089d6df368.llama30b_17
|
294 |
-
```
|
295 |
-
|
296 |
-
So the model gets a reward model score mean of 0.55 and median of 0.58. This compares to our [20B model](https://huggingface.co/h2oai/h2ogpt-oasst1-512-20b) that gets 0.49 mean and 0.48 median or [Dollyv2](https://huggingface.co/databricks/dolly-v2-12b) that gets 0.37 mean and 0.27 median.
|
297 |
-
|
298 |
-
[Logs](https://huggingface.co/h2oai/h2ogpt-research-oig-oasst1-512-30b/blob/main/score_llama30b_jon17d.log) and [prompt-response pairs](https://huggingface.co/h2oai/h2ogpt-research-oig-oasst1-512-30b/blob/main/df_scores_100_100_1234_False_llama-30b-hf_llama-30b-hf.h2oaih2ogpt-oig-oasst1-instruct-cleaned-v2.2.0_epochs.131f6d098b43236b5f91e76fc074ad089d6df368.llama30b_17.parquet)
|
299 |
-
|
300 |
-
The full distribution of scores is shown here:
|
301 |
-
|
302 |
-

|
303 |
-
|
304 |
-
Same plot for our h2oGPT 20B:
|
305 |
-
|
306 |
-

|
307 |
-
|
308 |
-
Same plot for DB Dollyv2:
|
309 |
-
|
310 |
-

|
311 |
-
|
312 |
-
|
313 |
-
|
314 |
-
## Disclaimer
|
315 |
-
|
316 |
-
Please read this disclaimer carefully before using the large language model provided in this repository. Your use of the model signifies your agreement to the following terms and conditions.
|
317 |
-
|
318 |
-
- The LORA contained in this repository is only for research (non-commercial) purposes.
|
319 |
-
- Biases and Offensiveness: The large language model is trained on a diverse range of internet text data, which may contain biased, racist, offensive, or otherwise inappropriate content. By using this model, you acknowledge and accept that the generated content may sometimes exhibit biases or produce content that is offensive or inappropriate. The developers of this repository do not endorse, support, or promote any such content or viewpoints.
|
320 |
-
- Limitations: The large language model is an AI-based tool and not a human. It may produce incorrect, nonsensical, or irrelevant responses. It is the user's responsibility to critically evaluate the generated content and use it at their discretion.
|
321 |
-
- Use at Your Own Risk: Users of this large language model must assume full responsibility for any consequences that may arise from their use of the tool. The developers and contributors of this repository shall not be held liable for any damages, losses, or harm resulting from the use or misuse of the provided model.
|
322 |
-
- Ethical Considerations: Users are encouraged to use the large language model responsibly and ethically. By using this model, you agree not to use it for purposes that promote hate speech, discrimination, harassment, or any form of illegal or harmful activities.
|
323 |
-
- Reporting Issues: If you encounter any biased, offensive, or otherwise inappropriate content generated by the large language model, please report it to the repository maintainers through the provided channels. Your feedback will help improve the model and mitigate potential issues.
|
324 |
-
- Changes to this Disclaimer: The developers of this repository reserve the right to modify or update this disclaimer at any time without prior notice. It is the user's responsibility to periodically review the disclaimer to stay informed about any changes.
|
325 |
|
326 |
-
|
|
|
1 |
---
|
|
|
|
|
|
|
|
|
2 |
inference: false
|
3 |
+
license: other
|
|
|
|
|
|
|
|
|
|
|
|
|
|
|
4 |
---
|
5 |
+
|
6 |
<!-- header start -->
|
7 |
<div style="width: 100%;">
|
8 |
<img src="https://i.imgur.com/EBdldam.jpg" alt="TheBlokeAI" style="width: 100%; min-width: 400px; display: block; margin: auto;">
|
|
|
17 |
</div>
|
18 |
<!-- header end -->
|
19 |
|
20 |
+
# H2OGPT's OASST1-512 30B GGML
|
21 |
|
22 |
+
These files are GGML format model files for [H2OGPT's OASST1-512 30B](https://huggingface.co/h2oai/h2ogpt-research-oig-oasst1-512-30b).
|
23 |
|
24 |
+
GGML files are for CPU + GPU inference using [llama.cpp](https://github.com/ggerganov/llama.cpp) and libraries and UIs which support this format, such as:
|
25 |
+
* [text-generation-webui](https://github.com/oobabooga/text-generation-webui)
|
26 |
+
* [KoboldCpp](https://github.com/LostRuins/koboldcpp)
|
27 |
+
* [ParisNeo/GPT4All-UI](https://github.com/ParisNeo/gpt4all-ui)
|
28 |
+
* [llama-cpp-python](https://github.com/abetlen/llama-cpp-python)
|
29 |
+
* [ctransformers](https://github.com/marella/ctransformers)
|
30 |
|
31 |
## Repositories available
|
32 |
|
33 |
+
* [4-bit GPTQ models for GPU inference](https://huggingface.co/TheBloke/h2ogpt-oasst1-512-30B-GPTQ)
|
34 |
+
* [2, 3, 4, 5, 6 and 8-bit GGML models for CPU+GPU inference](https://huggingface.co/TheBloke/h2ogpt-oasst1-512-30B-GGML)
|
35 |
+
* [Unquantised fp16 model in pytorch format, for GPU inference and for further conversions](https://huggingface.co/TheBloke/h2ogpt-oasst1-512-30B-HF)
|
36 |
+
|
37 |
+
<!-- compatibility_ggml start -->
|
38 |
+
## Compatibility
|
39 |
+
|
40 |
+
### Original llama.cpp quant methods: `q4_0, q4_1, q5_0, q5_1, q8_0`
|
41 |
+
|
42 |
+
I have quantized these 'original' quantisation methods using an older version of llama.cpp so that they remain compatible with llama.cpp as of May 19th, commit `2d5db48`.
|
43 |
+
|
44 |
+
They should be compatible with all current UIs and libraries that use llama.cpp, such as those listed at the top of this README.
|
45 |
+
|
46 |
+
### New k-quant methods: `q2_K, q3_K_S, q3_K_M, q3_K_L, q4_K_S, q4_K_M, q5_K_S, q6_K`
|
47 |
+
|
48 |
+
These new quantisation methods are only compatible with llama.cpp as of June 6th, commit `2d43387`.
|
49 |
|
50 |
+
They will NOT be compatible with koboldcpp, text-generation-ui, and other UIs and libraries yet. Support is expected to come over the next few days.
|
51 |
|
52 |
+
## Explanation of the new k-quant methods
|
53 |
|
54 |
+
The new methods available are:
|
55 |
+
* GGML_TYPE_Q2_K - "type-1" 2-bit quantization in super-blocks containing 16 blocks, each block having 16 weight. Block scales and mins are quantized with 4 bits. This ends up effectively using 2.5625 bits per weight (bpw)
|
56 |
+
* GGML_TYPE_Q3_K - "type-0" 3-bit quantization in super-blocks containing 16 blocks, each block having 16 weights. Scales are quantized with 6 bits. This end up using 3.4375 bpw.
|
57 |
+
* GGML_TYPE_Q4_K - "type-1" 4-bit quantization in super-blocks containing 8 blocks, each block having 32 weights. Scales and mins are quantized with 6 bits. This ends up using 4.5 bpw.
|
58 |
+
* GGML_TYPE_Q5_K - "type-1" 5-bit quantization. Same super-block structure as GGML_TYPE_Q4_K resulting in 5.5 bpw
|
59 |
+
* GGML_TYPE_Q6_K - "type-0" 6-bit quantization. Super-blocks with 16 blocks, each block having 16 weights. Scales are quantized with 8 bits. This ends up using 6.5625 bpw
|
60 |
+
* GGML_TYPE_Q8_K - "type-0" 8-bit quantization. Only used for quantizing intermediate results. The difference to the existing Q8_0 is that the block size is 256. All 2-6 bit dot products are implemented for this quantization type.
|
61 |
+
|
62 |
+
Refer to the Provided Files table below to see what files use which methods, and how.
|
63 |
+
<!-- compatibility_ggml end -->
|
64 |
|
|
|
65 |
## Provided files
|
66 |
+
| Name | Quant method | Bits | Size | Max RAM required | Use case |
|
67 |
| ---- | ---- | ---- | ---- | ---- | ----- |
|
68 |
+
| h2ogptq-oasst1-512-30B.ggmlv3.q2_K.bin | q2_K | 2 | 13.60 GB | 16.10 GB | New k-quant method. Uses GGML_TYPE_Q4_K for the attention.vw and feed_forward.w2 tensors, GGML_TYPE_Q2_K for the other tensors. |
|
69 |
+
| h2ogptq-oasst1-512-30B.ggmlv3.q3_K_L.bin | q3_K_L | 3 | 17.20 GB | 19.70 GB | New k-quant method. Uses GGML_TYPE_Q5_K for the attention.wv, attention.wo, and feed_forward.w2 tensors, else GGML_TYPE_Q3_K |
|
70 |
+
| h2ogptq-oasst1-512-30B.ggmlv3.q3_K_M.bin | q3_K_M | 3 | 15.64 GB | 18.14 GB | New k-quant method. Uses GGML_TYPE_Q4_K for the attention.wv, attention.wo, and feed_forward.w2 tensors, else GGML_TYPE_Q3_K |
|
71 |
+
| h2ogptq-oasst1-512-30B.ggmlv3.q3_K_S.bin | q3_K_S | 3 | 13.98 GB | 16.48 GB | New k-quant method. Uses GGML_TYPE_Q3_K for all tensors |
|
72 |
+
| h2ogptq-oasst1-512-30B.ggmlv3.q4_0.bin | q4_0 | 4 | 18.30 GB | 20.80 GB | Original llama.cpp quant method, 4-bit. |
|
73 |
+
| h2ogptq-oasst1-512-30B.ggmlv3.q4_1.bin | q4_1 | 4 | 20.33 GB | 22.83 GB | Original llama.cpp quant method, 4-bit. Higher accuracy than q4_0 but not as high as q5_0. However has quicker inference than q5 models. |
|
74 |
+
| h2ogptq-oasst1-512-30B.ggmlv3.q4_K_M.bin | q4_K_M | 4 | 19.57 GB | 22.07 GB | New k-quant method. Uses GGML_TYPE_Q6_K for half of the attention.wv and feed_forward.w2 tensors, else GGML_TYPE_Q4_K |
|
75 |
+
| h2ogptq-oasst1-512-30B.ggmlv3.q4_K_S.bin | q4_K_S | 4 | 18.30 GB | 20.80 GB | New k-quant method. Uses GGML_TYPE_Q4_K for all tensors |
|
76 |
+
| h2ogptq-oasst1-512-30B.ggmlv3.q5_0.bin | q5_0 | 5 | 22.37 GB | 24.87 GB | Original llama.cpp quant method, 5-bit. Higher accuracy, higher resource usage and slower inference. |
|
77 |
+
| h2ogptq-oasst1-512-30B.ggmlv3.q5_1.bin | q5_1 | 5 | 24.40 GB | 26.90 GB | Original llama.cpp quant method, 5-bit. Even higher accuracy, resource usage and slower inference. |
|
78 |
+
| h2ogptq-oasst1-512-30B.ggmlv3.q5_K_M.bin | q5_K_M | 5 | 23.02 GB | 25.52 GB | New k-quant method. Uses GGML_TYPE_Q6_K for half of the attention.wv and feed_forward.w2 tensors, else GGML_TYPE_Q5_K |
|
79 |
+
| h2ogptq-oasst1-512-30B.ggmlv3.q5_K_S.bin | q5_K_S | 5 | 22.37 GB | 24.87 GB | New k-quant method. Uses GGML_TYPE_Q5_K for all tensors |
|
80 |
+
| h2ogptq-oasst1-512-30B.ggmlv3.q6_K.bin | q6_K | 6 | 26.69 GB | 29.19 GB | New k-quant method. Uses GGML_TYPE_Q8_K - 6-bit quantization - for all tensors |
|
81 |
+
| h2ogptq-oasst1-512-30B.ggmlv3.q8_0.bin | q8_0 | 8 | 34.56 GB | 37.06 GB | Original llama.cpp quant method, 8-bit. Almost indistinguishable from float16. High resource use and slow. Not recommended for most users. |
|
82 |
+
|
83 |
+
|
84 |
+
**Note**: the above RAM figures assume no GPU offloading. If layers are offloaded to the GPU, this will reduce RAM usage and use VRAM instead.
|
85 |
|
86 |
## How to run in `llama.cpp`
|
87 |
|
88 |
I use the following command line; adjust for your tastes and needs:
|
89 |
|
90 |
```
|
91 |
+
./main -t 10 -ngl 32 -m h2ogptq-oasst1-512-30B.ggmlv3.q5_0.bin --color -c 2048 --temp 0.7 --repeat_penalty 1.1 -n -1 -p "### Instruction: Write a story about llamas\n### Response:"
|
|
|
|
|
|
|
92 |
```
|
93 |
+
Change `-t 10` to the number of physical CPU cores you have. For example if your system has 8 cores/16 threads, use `-t 8`.
|
94 |
+
|
95 |
+
Change `-ngl 32` to the number of layers to offload to GPU. Remove it if you don't have GPU acceleration.
|
96 |
|
97 |
If you want to have a chat-style conversation, replace the `-p <PROMPT>` argument with `-i -ins`
|
98 |
|
99 |
## How to run in `text-generation-webui`
|
100 |
|
|
|
|
|
101 |
Further instructions here: [text-generation-webui/docs/llama.cpp-models.md](https://github.com/oobabooga/text-generation-webui/blob/main/docs/llama.cpp-models.md).
|
102 |
|
|
|
|
|
103 |
<!-- footer start -->
|
104 |
## Discord
|
105 |
|
|
|
120 |
* Patreon: https://patreon.com/TheBlokeAI
|
121 |
* Ko-Fi: https://ko-fi.com/TheBlokeAI
|
122 |
|
123 |
+
**Special thanks to**: Luke from CarbonQuill, Aemon Algiz, Dmitriy Samsonov.
|
124 |
+
|
125 |
+
**Patreon special mentions**: Oscar Rangel, Eugene Pentland, Talal Aujan, Cory Kujawski, Luke, Asp the Wyvern, Ai Maven, Pyrater, Alps Aficionado, senxiiz, Willem Michiel, Junyu Yang, trip7s trip, Sebastain Graf, Joseph William Delisle, Lone Striker, Jonathan Leane, Johann-Peter Hartmann, David Flickinger, Spiking Neurons AB, Kevin Schuppel, Mano Prime, Dmitriy Samsonov, Sean Connelly, Nathan LeClaire, Alain Rossmann, Fen Risland, Derek Yates, Luke Pendergrass, Nikolai Manek, Khalefa Al-Ahmad, Artur Olbinski, John Detwiler, Ajan Kanaga, Imad Khwaja, Trenton Dambrowitz, Kalila, vamX, webtim, Illia Dulskyi.
|
126 |
|
127 |
Thank you to all my generous patrons and donaters!
|
128 |
+
|
129 |
<!-- footer end -->
|
130 |
|
131 |
+
# Original model card: H2OGPT's OASST1-512 30B
|
132 |
+
|
133 |
+
# h2oGPT Model Card
|
134 |
## Summary
|
135 |
|
136 |
H2O.ai's `h2oai/h2ogpt-research-oig-oasst1-512-30b` is a 30 billion parameter instruction-following large language model for research use only.
|
137 |
|
138 |
+
- Base model [decapoda-research/llama-30b-hf](https://huggingface.co/decapoda-research/llama-30b-hf)
|
139 |
+
- LORA [h2oai/h2ogpt-research-oig-oasst1-512-30b-lora](https://huggingface.co/h2oai/h2ogpt-research-oig-oasst1-512-30b-lora)
|
140 |
+
- This HF version was built using the [export script and steps](https://huggingface.co/h2oai/h2ogpt-research-oig-oasst1-512-30b-lora#build-hf-model)
|
|
|
|
|
|
|
|
|
|
|
|
|
|
|
|
|
|
|
|
|
|
|
|
|
|
|
|
|
|
|
|
|
|
|
|
|
|
|
|
|
|
|
|
|
|
|
|
|
|
|
|
|
|
|
|
|
|
|
|
|
|
|
|
|
|
|
|
|
|
|
|
|
|
|
|
|
|
|
|
|
|
|
|
|
|
|
|
|
|
|
|
|
|
|
|
|
|
|
|
|
|
|
|
|
|
|
|
|
|
|
|
|
|
|
|
|
|
|
|
|
|
|
|
|
|
|
|
|
|
|
|
|
|
|
|
|
|
|
|
|
|
|
|
|
|
|
|
|
|
|
|
|
|
|
|
|
|
|
|
|
|
|
|
|
|
|
|
|
|
|
|
|
|
|
|
|
|
|
|
|
|
|
|
|
|
|
|
|
|
|
|
|
|
|
|
|
|
|
|
|
|
|
|
|
|
|
|
|
|
|
|
|
|
|
|
|
|
|
|
|
|
|
|
|
|
|
|
|
|
|
|
|
|
|
|
|
|
|
|
|
|
|
|
|
|
|
|
|
|
|
|
|
|
|
|
|
|
|
|
|
|
|
|
|
|
|
|
|
|
|
|
|
|
|
|
|
|
|
|
|
|
|
|
|
|
|
|
|
|
|
|
|
|
|
|
|
|
|
|
|
|
|
|
|
|
|
|
|
|
|
|
|
|
|
|
|
|
|
|
|
|
|
|
|
|
|
|
|
|
|
|
|
|
|
|
|
|
|
|
|
|
|
|
|
|
|
|
|
|
|
|
|
|
|
|
|
|
|
|
|
|
|
|
|
|
|
|
|
|
|
|
|
|
|
|
|
|
|
|
|
|
|
|
|
|
|
|
|
|
|
|
|
|
|
|
|
|
|
|
|
|
|
|
|
|
|
|
|
|
|
|
|
|
|
|
|
|
|
|
|
|
|
|
|
141 |
|
142 |
+
All details about performance etc. are provided in the [LORA Model Card](https://huggingface.co/h2oai/h2ogpt-research-oig-oasst1-512-30b-lora).
|