Dan Jacobellis
commited on
Commit
·
fc74cbd
1
Parent(s):
97437b1
add visual comparison
Browse files- README.ipynb +51 -18
- README.md +44 -17
- README_files/README_13_0.jpg +2 -2
- README_files/README_13_0.png +2 -2
- README_files/README_15_0.jpg +0 -0
- README_files/README_15_0.png +0 -0
- README_files/README_18_0.png +2 -2
- README_files/README_22_0.jpg +2 -2
- README_files/README_22_0.png +2 -2
- README_files/README_24_0.jpg +2 -2
- README_files/README_24_0.png +0 -0
- README_files/README_26_0.jpg +0 -0
- README_files/README_41_0.png +2 -2
- README_files/README_44_1.jpg +0 -0
- README_files/README_44_1.png +0 -0
- README_files/README_47_0.png +0 -0
README.ipynb
CHANGED
@@ -21,7 +21,29 @@
|
|
21 |
"\n",
|
22 |
"- [Project page and documentation](https://danjacobellis.net/walloc)\n",
|
23 |
"- [Paper: \"Learned Compression for Compressed Learning\"](https://danjacobellis.net/_static/walloc.pdf)\n",
|
24 |
-
"- [Additional code accompanying the paper](https://github.com/danjacobellis/lccl)"
|
|
|
|
|
|
|
|
|
|
|
|
|
|
|
|
|
|
|
|
|
|
|
|
|
|
|
|
|
|
|
|
|
|
|
|
|
|
|
|
|
|
|
|
|
25 |
]
|
26 |
},
|
27 |
{
|
@@ -35,24 +57,39 @@
|
|
35 |
]
|
36 |
},
|
37 |
{
|
38 |
-
"attachments": {},
|
39 |
"cell_type": "markdown",
|
40 |
-
"id": "
|
|
|
|
|
|
|
|
|
|
|
|
|
|
|
|
|
|
|
|
|
|
|
|
|
41 |
"metadata": {},
|
42 |
"source": [
|
43 |
-
"$. An inverse level consists of upsampling $(\\uparrow 2)$ followed by $\\text{L}_{\\text{S}}$\n",
|
45 |
-
"and $\\text{H}_{\\text{S}}$, then summing the two channels. The full WPT $\\tilde{\\textbf{X}}$ of consists of $J$ levels."
|
46 |
]
|
47 |
},
|
48 |
{
|
49 |
"cell_type": "markdown",
|
50 |
-
"id": "
|
51 |
"metadata": {},
|
52 |
"source": [
|
53 |
-
"
|
54 |
-
"
|
55 |
-
"
|
|
|
|
|
|
|
|
|
|
|
|
|
56 |
]
|
57 |
},
|
58 |
{
|
@@ -60,10 +97,6 @@
|
|
60 |
"id": "612736c3-c47f-4447-9d1a-07a07cfe4c47",
|
61 |
"metadata": {},
|
62 |
"source": [
|
63 |
-
"# Wavelet Learned Lossy Compression (WaLLoC)\n",
|
64 |
-
"\n",
|
65 |
-
"WaLLoC sandwiches a convolutional autoencoder between time-frequency analysis and synthesis transforms using CDF 9/7 wavelet filters. The time-frequency transform increases the number of signal channels, but reduces the temporal or spatial resolution, resulting in lower GPU memory consumption and higher throughput. WaLLoC's training procedure is highly simplified compared to other $\\beta$-VAEs, VQ-VAEs, and neural codecs, but still offers significant dimensionality reduction and compression. This makes it suitable for dataset storage and compressed-domain learning. It currently supports 1D and 2D signals, including mono, stereo, or multi-channel audio, and grayscale, RGB, or hyperspectral images.\n",
|
66 |
-
"\n",
|
67 |
"## Installation\n",
|
68 |
"\n",
|
69 |
"1. Follow the installation instructions for [torch](https://pytorch.org/get-started/locally/)\n",
|
@@ -775,7 +808,7 @@
|
|
775 |
},
|
776 |
{
|
777 |
"cell_type": "code",
|
778 |
-
"execution_count":
|
779 |
"id": "0ae9e5bb-d616-4d22-a9c9-6743e4216802",
|
780 |
"metadata": {},
|
781 |
"outputs": [
|
@@ -785,7 +818,7 @@
|
|
785 |
"text": [
|
786 |
"[NbConvertApp] Converting notebook README.ipynb to markdown\n",
|
787 |
"[NbConvertApp] Support files will be in README_files/\n",
|
788 |
-
"[NbConvertApp] Writing
|
789 |
]
|
790 |
}
|
791 |
],
|
@@ -795,7 +828,7 @@
|
|
795 |
},
|
796 |
{
|
797 |
"cell_type": "code",
|
798 |
-
"execution_count":
|
799 |
"id": "3d4794d5-a26a-4789-804e-7aa543db89d6",
|
800 |
"metadata": {},
|
801 |
"outputs": [],
|
@@ -805,7 +838,7 @@
|
|
805 |
},
|
806 |
{
|
807 |
"cell_type": "code",
|
808 |
-
"execution_count":
|
809 |
"id": "8a386d35-9cdc-4e0b-8d5a-b58c4e055c28",
|
810 |
"metadata": {},
|
811 |
"outputs": [],
|
|
|
21 |
"\n",
|
22 |
"- [Project page and documentation](https://danjacobellis.net/walloc)\n",
|
23 |
"- [Paper: \"Learned Compression for Compressed Learning\"](https://danjacobellis.net/_static/walloc.pdf)\n",
|
24 |
+
"- [Additional code accompanying the paper](https://github.com/danjacobellis/lccl)\n",
|
25 |
+
"\n",
|
26 |
+
"WaLLoC (Wavelet-Domain Learned Lossy Compression) is an architecture for learned compression that simultaneously satisfies three key\n",
|
27 |
+
"requirements of compressed-domain learning:\n",
|
28 |
+
"\n",
|
29 |
+
"1. **Computationally efficient encoding** to reduce overhead in compressed-domain learning and support resource constrained mobile and remote sensors. WaLLoC uses a wavelet packet transform to expose signal redundancies prior to autoencoding. This allows us to replace the encoding DNN with a single linear layer (<100k parameters) without significant loss in quality. WaLLoC incurs <5% of the encoding cost compared to other neural codecs.\n",
|
30 |
+
"\n",
|
31 |
+
"2. **High compression ratio** for storage and transmission efficiency. Lossy codecs typically achieve high compression with a combination of quantization and entropy coding. However, naive quantization of autoencoder latents leads to unpredictable and unbounded distortion. Instead, we apply additive noise during training as an\n",
|
32 |
+
"entropy bottleneck, leading to quantization-resiliant latents. When combined with entropy coding, this provides nearly 12× higher compression ratio compared to the VAE used in Stable Diffusion 3, despite offering a higher degree of dimensionality reduction and similar quality.\n",
|
33 |
+
"\n",
|
34 |
+
"3. **Dimensionality reduction** to accelerate compressed-domain modeling. WaLLoC’s encoder projects high-dimensional signal patches to low-dimensional latent representations, providing a reduction of up to 20×. This allows WaLLoC to be used as a drop-in replacement for resolution reduction while providing superior detail preservation and downstream accuracy.\n",
|
35 |
+
"\n",
|
36 |
+
"WaLLoC does not require perceptual or adversarial losses to represent high-frequency detail, making it compatible with a wide variety of signal types. It currently supports 1D and 2D signals, including mono, stereo, and multi-channel audio and grayscale, RGB, and hyperspectral images."
|
37 |
+
]
|
38 |
+
},
|
39 |
+
{
|
40 |
+
"cell_type": "markdown",
|
41 |
+
"id": "84b21fc4-875c-468e-b0ee-201e71bd5737",
|
42 |
+
"metadata": {},
|
43 |
+
"source": [
|
44 |
+
"\n",
|
45 |
+
"WaLLoC’s encode-decode pipeline. The entropy bottleneck and entropy coding steps are only required to achieve high compression ratios for storage and transmission. For compressed-domain learning where dimensionality reduction is the primary goal, these steps can be skipped to reduce overhead and completely eliminate CPU-GPU transfers.\n",
|
46 |
+
"\n"
|
47 |
]
|
48 |
},
|
49 |
{
|
|
|
57 |
]
|
58 |
},
|
59 |
{
|
|
|
60 |
"cell_type": "markdown",
|
61 |
+
"id": "6dd81040-1e6e-45e3-b37d-1a1aabce8962",
|
62 |
+
"metadata": {},
|
63 |
+
"source": [
|
64 |
+
"\n",
|
65 |
+
"\n",
|
66 |
+
"\n",
|
67 |
+
"\n",
|
68 |
+
""
|
69 |
+
]
|
70 |
+
},
|
71 |
+
{
|
72 |
+
"cell_type": "markdown",
|
73 |
+
"id": "3651a78e-c5c9-44e3-9f6d-ef44928ef0a7",
|
74 |
"metadata": {},
|
75 |
"source": [
|
76 |
+
""
|
|
|
|
|
77 |
]
|
78 |
},
|
79 |
{
|
80 |
"cell_type": "markdown",
|
81 |
+
"id": "e756f6fb-6caa-4587-9767-9735043291f4",
|
82 |
"metadata": {},
|
83 |
"source": [
|
84 |
+
"```\n",
|
85 |
+
"@article{jacobellis2024learned,\n",
|
86 |
+
" title={Learned Compression for Compressed Learning},\n",
|
87 |
+
" author={Jacobellis, Dan and Yadwadkar, Neeraja J.},\n",
|
88 |
+
" year={2024},\n",
|
89 |
+
" note={Under review},\n",
|
90 |
+
" url={http://danjacobellis.net/walloc}\n",
|
91 |
+
"}\n",
|
92 |
+
"```"
|
93 |
]
|
94 |
},
|
95 |
{
|
|
|
97 |
"id": "612736c3-c47f-4447-9d1a-07a07cfe4c47",
|
98 |
"metadata": {},
|
99 |
"source": [
|
|
|
|
|
|
|
|
|
100 |
"## Installation\n",
|
101 |
"\n",
|
102 |
"1. Follow the installation instructions for [torch](https://pytorch.org/get-started/locally/)\n",
|
|
|
808 |
},
|
809 |
{
|
810 |
"cell_type": "code",
|
811 |
+
"execution_count": 1,
|
812 |
"id": "0ae9e5bb-d616-4d22-a9c9-6743e4216802",
|
813 |
"metadata": {},
|
814 |
"outputs": [
|
|
|
818 |
"text": [
|
819 |
"[NbConvertApp] Converting notebook README.ipynb to markdown\n",
|
820 |
"[NbConvertApp] Support files will be in README_files/\n",
|
821 |
+
"[NbConvertApp] Writing 12900 bytes to README.md\n"
|
822 |
]
|
823 |
}
|
824 |
],
|
|
|
828 |
},
|
829 |
{
|
830 |
"cell_type": "code",
|
831 |
+
"execution_count": 2,
|
832 |
"id": "3d4794d5-a26a-4789-804e-7aa543db89d6",
|
833 |
"metadata": {},
|
834 |
"outputs": [],
|
|
|
838 |
},
|
839 |
{
|
840 |
"cell_type": "code",
|
841 |
+
"execution_count": 3,
|
842 |
"id": "8a386d35-9cdc-4e0b-8d5a-b58c4e055c28",
|
843 |
"metadata": {},
|
844 |
"outputs": [],
|
README.md
CHANGED
@@ -9,23 +9,45 @@ datasets:
|
|
9 |
- [Paper: "Learned Compression for Compressed Learning"](https://danjacobellis.net/_static/walloc.pdf)
|
10 |
- [Additional code accompanying the paper](https://github.com/danjacobellis/lccl)
|
11 |
|
12 |
-
|
13 |
-
|
|
|
|
|
14 |
|
|
|
|
|
15 |
|
|
|
16 |
|
17 |
-
|
18 |
-
Example of forward and inverse WPT with $J=2$ levels. Each level applies filters $\text{L}_{\text{A}}$ and $\text{H}_{\text{A}}$ independently to each of the signal channels, followed by downsampling by two $(\downarrow 2)$. An inverse level consists of upsampling $(\uparrow 2)$ followed by $\text{L}_{\text{S}}$
|
19 |
-
and $\text{H}_{\text{S}}$, then summing the two channels. The full WPT $\tilde{\textbf{X}}$ of consists of $J$ levels.
|
20 |
|
21 |

|
22 |
WaLLoC’s encode-decode pipeline. The entropy bottleneck and entropy coding steps are only required to achieve high compression ratios for storage and transmission. For compressed-domain learning where dimensionality reduction is the primary goal, these steps can be skipped to reduce overhead and completely eliminate CPU-GPU transfers.
|
23 |
|
24 |
|
25 |
|
26 |
-
|
|
|
|
|
27 |
|
28 |
-
|
|
|
|
|
|
|
|
|
|
|
|
|
|
|
|
|
|
|
|
|
|
|
|
|
|
|
|
|
|
|
|
|
|
|
29 |
|
30 |
## Installation
|
31 |
|
@@ -90,7 +112,7 @@ img
|
|
90 |
|
91 |
|
92 |
|
93 |
-
(x_hat[0]+0.5)
|
|
115 |
|
116 |
|
117 |
|
118 |
-
;
|
|
151 |
|
152 |
|
153 |
|
154 |
-
/os.path.getsize("latent.png"))
|
|
183 |
|
184 |
|
185 |
|
186 |
-
/os.path.getsize("latent.webp"))
|
|
205 |
|
206 |
|
207 |
|
208 |
-
/os.path.getsize("latent.tif"))
|
|
228 |
|
229 |
|
230 |
|
231 |
-
;
|
|
357 |
|
358 |
|
359 |
|
360 |
-
;
|
|
438 |
|
439 |
|
440 |
|
441 |
-
;
|
|
449 |
|
450 |
[NbConvertApp] Converting notebook README.ipynb to markdown
|
451 |
[NbConvertApp] Support files will be in README_files/
|
452 |
-
[NbConvertApp] Writing
|
453 |
|
454 |
|
455 |
|
456 |
```python
|
457 |
!sed -i 's|!\[png](README_files/\(README_[0-9]*_[0-9]*\.png\))||g' README.md
|
458 |
```
|
|
|
|
|
|
|
|
|
|
|
|
9 |
- [Paper: "Learned Compression for Compressed Learning"](https://danjacobellis.net/_static/walloc.pdf)
|
10 |
- [Additional code accompanying the paper](https://github.com/danjacobellis/lccl)
|
11 |
|
12 |
+
WaLLoC (Wavelet-Domain Learned Lossy Compression) is an architecture for learned compression that simultaneously satisfies three key
|
13 |
+
requirements of compressed-domain learning:
|
14 |
+
|
15 |
+
1. **Computationally efficient encoding** to reduce overhead in compressed-domain learning and support resource constrained mobile and remote sensors. WaLLoC uses a wavelet packet transform to expose signal redundancies prior to autoencoding. This allows us to replace the encoding DNN with a single linear layer (<100k parameters) without significant loss in quality. WaLLoC incurs <5% of the encoding cost compared to other neural codecs.
|
16 |
|
17 |
+
2. **High compression ratio** for storage and transmission efficiency. Lossy codecs typically achieve high compression with a combination of quantization and entropy coding. However, naive quantization of autoencoder latents leads to unpredictable and unbounded distortion. Instead, we apply additive noise during training as an
|
18 |
+
entropy bottleneck, leading to quantization-resiliant latents. When combined with entropy coding, this provides nearly 12× higher compression ratio compared to the VAE used in Stable Diffusion 3, despite offering a higher degree of dimensionality reduction and similar quality.
|
19 |
|
20 |
+
3. **Dimensionality reduction** to accelerate compressed-domain modeling. WaLLoC’s encoder projects high-dimensional signal patches to low-dimensional latent representations, providing a reduction of up to 20×. This allows WaLLoC to be used as a drop-in replacement for resolution reduction while providing superior detail preservation and downstream accuracy.
|
21 |
|
22 |
+
WaLLoC does not require perceptual or adversarial losses to represent high-frequency detail, making it compatible with a wide variety of signal types. It currently supports 1D and 2D signals, including mono, stereo, and multi-channel audio and grayscale, RGB, and hyperspectral images.
|
|
|
|
|
23 |
|
24 |

|
25 |
WaLLoC’s encode-decode pipeline. The entropy bottleneck and entropy coding steps are only required to achieve high compression ratios for storage and transmission. For compressed-domain learning where dimensionality reduction is the primary goal, these steps can be skipped to reduce overhead and completely eliminate CPU-GPU transfers.
|
26 |
|
27 |
|
28 |
|
29 |
+

|
30 |
+
Comparison of WaLLoC with other autoencoder designs for RGB Images and stereo audio.
|
31 |
+
|
32 |
|
33 |
+
|
34 |
+

|
35 |
+
|
36 |
+

|
37 |
+
|
38 |
+

|
39 |
+
|
40 |
+

|
41 |
+
|
42 |
+
```
|
43 |
+
@article{jacobellis2024learned,
|
44 |
+
title={Learned Compression for Compressed Learning},
|
45 |
+
author={Jacobellis, Dan and Yadwadkar, Neeraja J.},
|
46 |
+
year={2024},
|
47 |
+
note={Under review},
|
48 |
+
url={http://danjacobellis.net/walloc}
|
49 |
+
}
|
50 |
+
```
|
51 |
|
52 |
## Installation
|
53 |
|
|
|
112 |
|
113 |
|
114 |
|
115 |
+

|
116 |
|
117 |
|
118 |
|
|
|
137 |
|
138 |
|
139 |
|
140 |
+
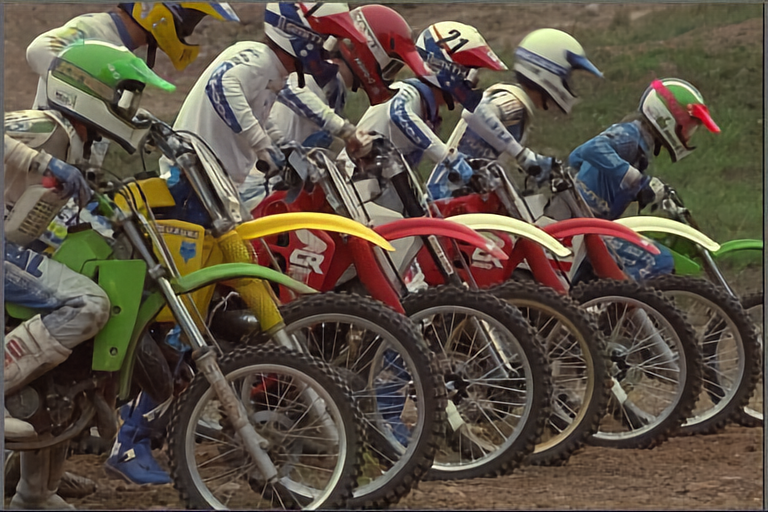
|
141 |
|
142 |
|
143 |
|
|
|
173 |
|
174 |
|
175 |
|
176 |
+
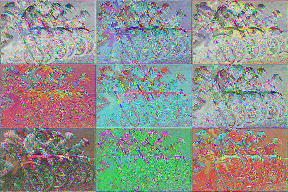
|
177 |
|
178 |
|
179 |
|
|
|
205 |
|
206 |
|
207 |
|
208 |
+

|
209 |
|
210 |
|
211 |
|
|
|
227 |
|
228 |
|
229 |
|
230 |
+
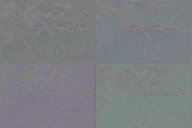
|
231 |
|
232 |
|
233 |
|
|
|
250 |
|
251 |
|
252 |
|
253 |
+

|
254 |
|
255 |
|
256 |
|
|
|
379 |
|
380 |
|
381 |
|
382 |
+

|
383 |
|
384 |
|
385 |
|
|
|
420 |
|
421 |
|
422 |
|
423 |
+

|
424 |
|
425 |
|
426 |
|
|
|
460 |
|
461 |
|
462 |
|
463 |
+
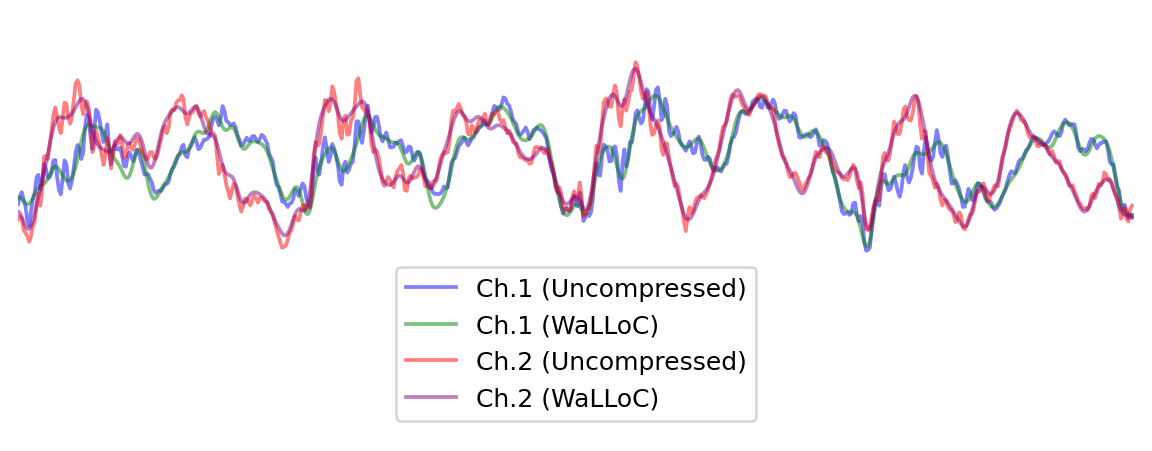
|
464 |
|
465 |
|
466 |
|
|
|
471 |
|
472 |
[NbConvertApp] Converting notebook README.ipynb to markdown
|
473 |
[NbConvertApp] Support files will be in README_files/
|
474 |
+
[NbConvertApp] Writing 11629 bytes to README.md
|
475 |
|
476 |
|
477 |
|
478 |
```python
|
479 |
!sed -i 's|!\[png](README_files/\(README_[0-9]*_[0-9]*\.png\))||g' README.md
|
480 |
```
|
481 |
+
|
482 |
+
|
483 |
+
```python
|
484 |
+
!sed -i 's|src="README_files/\(README_[0-9]*\.wav\)"|src="https://huggingface.co/danjacobellis/walloc/resolve/main/README_files/\1"|g' README.md
|
485 |
+
```
|
README_files/README_13_0.jpg
CHANGED
![]() |
Git LFS Details
|
![]() |
Git LFS Details
|
README_files/README_13_0.png
CHANGED
![]() |
Git LFS Details
|
![]() |
Git LFS Details
|
README_files/README_15_0.jpg
ADDED
![]() |
README_files/README_15_0.png
ADDED
![]() |
README_files/README_18_0.png
CHANGED
![]() |
Git LFS Details
|
![]() |
Git LFS Details
|
README_files/README_22_0.jpg
CHANGED
![]() |
Git LFS Details
|
![]() |
Git LFS Details
|
README_files/README_22_0.png
CHANGED
![]() |
Git LFS Details
|
![]() |
Git LFS Details
|
README_files/README_24_0.jpg
CHANGED
![]() |
Git LFS Details
|
![]() |
Git LFS Details
|
README_files/README_24_0.png
ADDED
![]() |
README_files/README_26_0.jpg
ADDED
![]() |
README_files/README_41_0.png
CHANGED
![]() |
Git LFS Details
|
![]() |
Git LFS Details
|
README_files/README_44_1.jpg
ADDED
![]() |
README_files/README_44_1.png
ADDED
![]() |
README_files/README_47_0.png
ADDED
![]() |