add data resource to readme
Browse files- README.md +34 -24
- configs/evaluate.json +11 -0
- configs/inference.json +1 -1
- configs/metadata.json +6 -3
- configs/train.json +2 -2
- docs/README.md +34 -24
README.md
CHANGED
@@ -5,19 +5,16 @@ tags:
|
|
5 |
library_name: monai
|
6 |
license: apache-2.0
|
7 |
---
|
8 |
-
# Description
|
9 |
-
A pre-trained model for volumetric (3D) detection of the lung lesion from CT image.
|
10 |
-
|
11 |
# Model Overview
|
12 |
-
|
13 |
|
14 |
-
|
15 |
|
16 |
-
|
17 |
|
18 |
## 1. Data
|
19 |
### 1.1 Data description
|
20 |
-
The dataset we are experimenting in this example is LUNA16 (https://luna16.grand-challenge.org/Home/), which is based on [LIDC
|
21 |
|
22 |
LUNA16 is a public dataset of CT lung nodule detection. Using raw CT scans, the goal is to identify locations of possible nodules, and to assign a probability for being a nodule to each location.
|
23 |
|
@@ -36,31 +33,44 @@ In this model, we resampled them into 0.703125 x 0.703125 x 1.25 mm.
|
|
36 |
|
37 |
Please following the instruction in Section 3.1 of https://github.com/Project-MONAI/tutorials/tree/main/detection to do the resampling.
|
38 |
|
|
|
|
|
|
|
|
|
|
|
39 |
## 2. Training configuration
|
40 |
-
The training was
|
|
|
|
|
41 |
|
42 |
Actual Model Input: 192 x 192 x 80
|
43 |
|
44 |
-
|
45 |
-
|
|
|
|
|
|
|
|
|
|
|
|
|
|
|
|
|
|
|
|
|
|
|
46 |
|
47 |
-
|
48 |
-
list of dictionary of predicted box, classification label, and classification score in evaluation mode.
|
49 |
|
50 |
-
##
|
51 |
-
The
|
52 |
|
53 |
-
|
54 |
|
55 |
-
|
56 |
-
| :---: | :---: | :---: | :---: | :---: | :---: | :---: | :---: |
|
57 |
-
| [Liu et al. (2019)](https://arxiv.org/pdf/1906.03467.pdf) | **0.848** | 0.876 | 0.905 | 0.933 | 0.943 | 0.957 | 0.970 |
|
58 |
-
| [nnDetection (2021)](https://arxiv.org/pdf/2106.00817.pdf) | 0.812 | **0.885** | 0.927 | 0.950 | 0.969 | 0.979 | 0.985 |
|
59 |
-
| MONAI detection | 0.835 | **0.885** | **0.931** | **0.957** | **0.974** | **0.983** | **0.988** |
|
60 |
|
61 |
-
|
62 |
|
63 |
-
##
|
64 |
Execute training:
|
65 |
```
|
66 |
python -m monai.bundle run training --meta_file configs/metadata.json --config_file configs/train.json --logging_file configs/logging.conf
|
@@ -71,11 +81,11 @@ Override the `train` config to execute evaluation with the trained model:
|
|
71 |
python -m monai.bundle run evaluating --meta_file configs/metadata.json --config_file "['configs/train.json','configs/evaluate.json']" --logging_file configs/logging.conf
|
72 |
```
|
73 |
|
74 |
-
Execute inference on resampled LUNA16 images
|
75 |
```
|
76 |
python -m monai.bundle run evaluating --meta_file configs/metadata.json --config_file configs/inference.json --logging_file configs/logging.conf
|
77 |
```
|
78 |
-
With the same command, we can execute inference on
|
79 |
|
80 |
Note that in inference.json, the transform "LoadImaged" in "preprocessing" and "AffineBoxToWorldCoordinated" in "postprocessing" has `"affine_lps_to_ras": true`.
|
81 |
This depends on the input images. LUNA16 needs `"affine_lps_to_ras": true`.
|
|
|
5 |
library_name: monai
|
6 |
license: apache-2.0
|
7 |
---
|
|
|
|
|
|
|
8 |
# Model Overview
|
9 |
+
A pre-trained model for volumetric (3D) detection of the lung nodule from CT image.
|
10 |
|
11 |
+
This model is trained on LUNA16 dataset (https://luna16.grand-challenge.org/Home/), using the RetinaNet (Lin, Tsung-Yi, et al. "Focal loss for dense object detection." ICCV 2017. https://arxiv.org/abs/1708.02002).
|
12 |
|
13 |
+
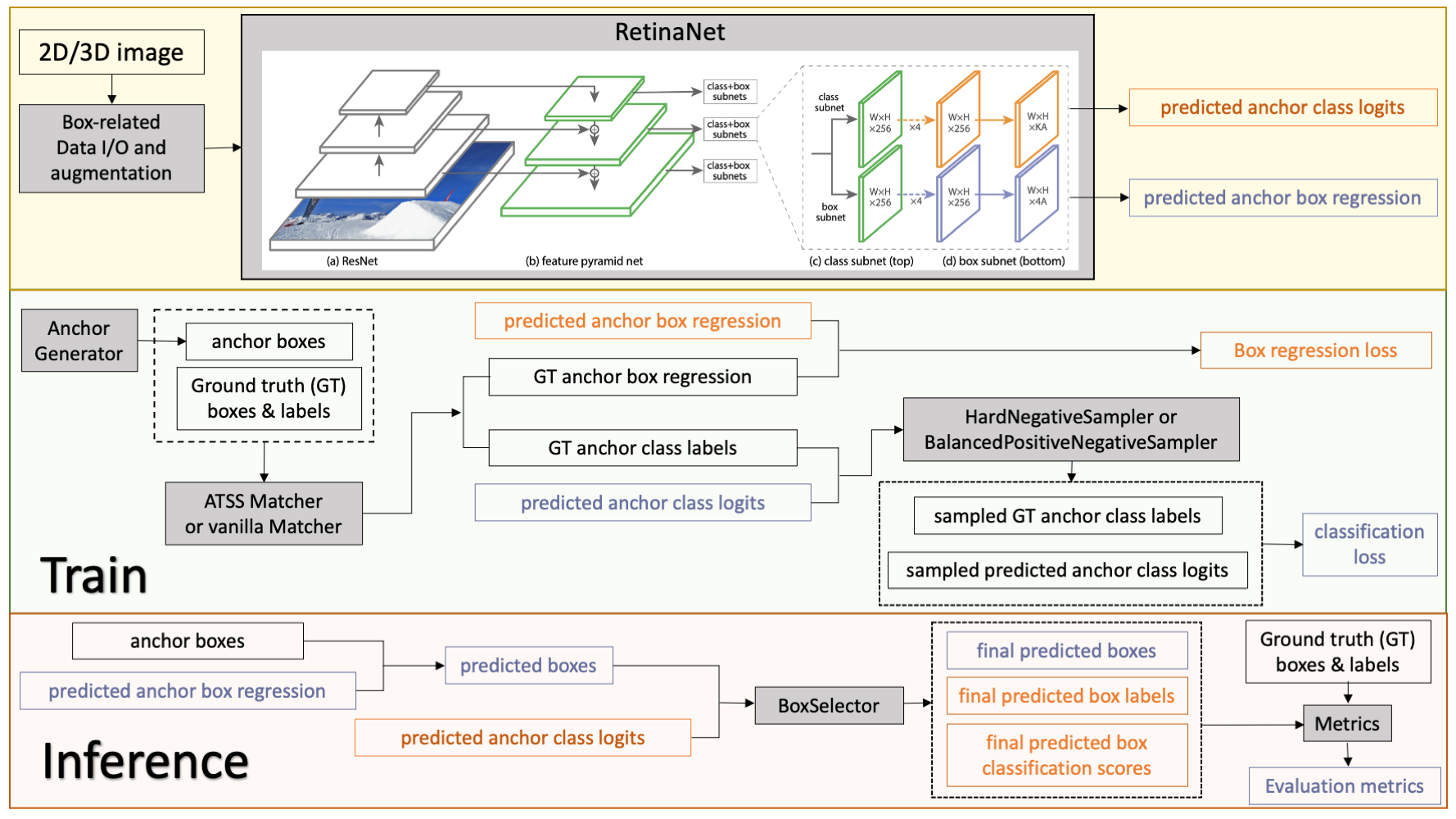
|
14 |
|
15 |
## 1. Data
|
16 |
### 1.1 Data description
|
17 |
+
The dataset we are experimenting in this example is LUNA16 (https://luna16.grand-challenge.org/Home/), which is based on [LIDC-IDRI database](https://wiki.cancerimagingarchive.net/display/Public/LIDC-IDRI) [3,4,5].
|
18 |
|
19 |
LUNA16 is a public dataset of CT lung nodule detection. Using raw CT scans, the goal is to identify locations of possible nodules, and to assign a probability for being a nodule to each location.
|
20 |
|
|
|
33 |
|
34 |
Please following the instruction in Section 3.1 of https://github.com/Project-MONAI/tutorials/tree/main/detection to do the resampling.
|
35 |
|
36 |
+
### 1.4 Data download
|
37 |
+
The mhd/raw original data can be downloaded from [LUNA16](https://luna16.grand-challenge.org/Home/). The DICOM original data can be downloaded from [LIDC-IDRI database](https://wiki.cancerimagingarchive.net/display/Public/LIDC-IDRI) [3,4,5]. You will need to resample the original data to start training.
|
38 |
+
|
39 |
+
Alternatively, we provide [resampled nifti images](https://drive.google.com/drive/folders/1JozrufA1VIZWJIc5A1EMV3J4CNCYovKK?usp=share_link) and a copy of [original mhd/raw images](https://drive.google.com/drive/folders/1-enN4eNEnKmjltevKg3W2V-Aj0nriQWE?usp=share_link) from [LUNA16](https://luna16.grand-challenge.org/Home/) for users to download.
|
40 |
+
|
41 |
## 2. Training configuration
|
42 |
+
The training was the following:
|
43 |
+
|
44 |
+
GPU: at least 16GB GPU memory
|
45 |
|
46 |
Actual Model Input: 192 x 192 x 80
|
47 |
|
48 |
+
AMP: True
|
49 |
+
|
50 |
+
Optimizer: Adam
|
51 |
+
|
52 |
+
Learning Rate: 1e-2
|
53 |
+
|
54 |
+
Loss: BCE loss and L1 loss
|
55 |
+
|
56 |
+
### Input
|
57 |
+
list of 1 channel 3D CT patches
|
58 |
+
|
59 |
+
### Output
|
60 |
+
In training mode: dictionary of classification and box regression loss in training mode;
|
61 |
|
62 |
+
In evaluation mode: list of dictionary of predicted box, classification label, and classification score in evaluation mode.
|
|
|
63 |
|
64 |
+
## 3. Performance
|
65 |
+
Coco metric is used for evaluating the performance of the model. The pre-trained model was trained and validated on data fold 0. This model achieves a mAP=0.853, mAR=0.994, AP(IoU=0.1)=0.862, AR(IoU=0.1)=1.0.
|
66 |
|
67 |
+
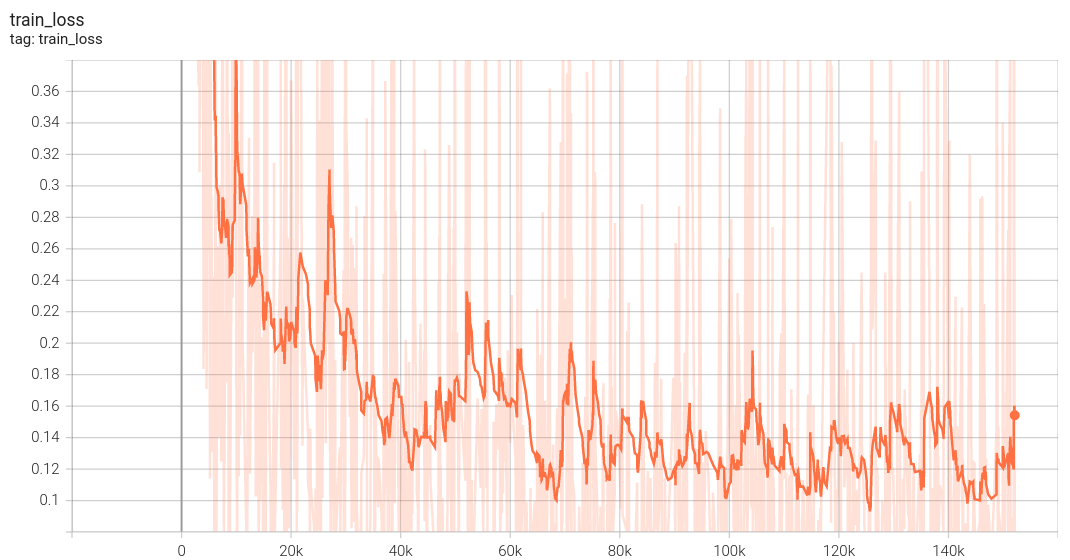
|
68 |
|
69 |
+
The validation accuracy in this curve is the mean of mAP, mAR, AP(IoU=0.1), and AR(IoU=0.1) in Coco metric.
|
|
|
|
|
|
|
|
|
70 |
|
71 |
+
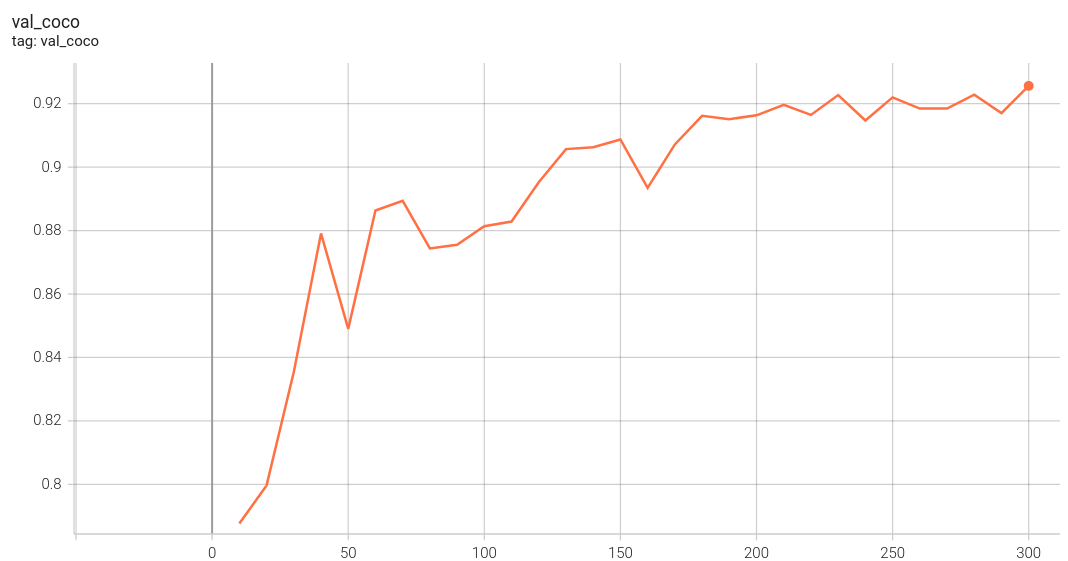
|
72 |
|
73 |
+
## 4. Commands example
|
74 |
Execute training:
|
75 |
```
|
76 |
python -m monai.bundle run training --meta_file configs/metadata.json --config_file configs/train.json --logging_file configs/logging.conf
|
|
|
81 |
python -m monai.bundle run evaluating --meta_file configs/metadata.json --config_file "['configs/train.json','configs/evaluate.json']" --logging_file configs/logging.conf
|
82 |
```
|
83 |
|
84 |
+
Execute inference on resampled LUNA16 images by setting `"whether_raw_luna16": false` in `inference.json`:
|
85 |
```
|
86 |
python -m monai.bundle run evaluating --meta_file configs/metadata.json --config_file configs/inference.json --logging_file configs/logging.conf
|
87 |
```
|
88 |
+
With the same command, we can execute inference on original LUNA16 images by setting `"whether_raw_luna16": true` in `inference.json`. Remember to also set `"data_list_file_path": "$@bundle_root + '/LUNA16_datasplit/mhd_original/dataset_fold0.json'"` and change `"data_file_base_dir"`.
|
89 |
|
90 |
Note that in inference.json, the transform "LoadImaged" in "preprocessing" and "AffineBoxToWorldCoordinated" in "postprocessing" has `"affine_lps_to_ras": true`.
|
91 |
This depends on the input images. LUNA16 needs `"affine_lps_to_ras": true`.
|
configs/evaluate.json
CHANGED
@@ -5,6 +5,17 @@
|
|
5 |
"data": "$@test_datalist",
|
6 |
"transform": "@validate#preprocessing"
|
7 |
},
|
|
|
|
|
|
|
|
|
|
|
|
|
|
|
|
|
|
|
|
|
|
|
8 |
"validate#handlers": [
|
9 |
{
|
10 |
"_target_": "CheckpointLoader",
|
|
|
5 |
"data": "$@test_datalist",
|
6 |
"transform": "@validate#preprocessing"
|
7 |
},
|
8 |
+
"validate#key_metric": {
|
9 |
+
"val_coco": {
|
10 |
+
"_target_": "scripts.cocometric_ignite.IgniteCocoMetric",
|
11 |
+
"coco_metric_monai": "$monai.apps.detection.metrics.coco.COCOMetric(classes=['nodule'], iou_list=[0.1], max_detection=[100])",
|
12 |
+
"output_transform": "$monai.handlers.from_engine(['pred', 'label'])",
|
13 |
+
"box_key": "box",
|
14 |
+
"label_key": "label",
|
15 |
+
"pred_score_key": "label_scores",
|
16 |
+
"reduce_scalar": false
|
17 |
+
}
|
18 |
+
},
|
19 |
"validate#handlers": [
|
20 |
{
|
21 |
"_target_": "CheckpointLoader",
|
configs/inference.json
CHANGED
@@ -9,7 +9,7 @@
|
|
9 |
"ckpt_dir": "$@bundle_root + '/models'",
|
10 |
"output_dir": "$@bundle_root + '/eval'",
|
11 |
"data_list_file_path": "$@bundle_root + '/LUNA16_datasplit/dataset_fold0.json'",
|
12 |
-
"data_file_base_dir": "/
|
13 |
"test_datalist": "$monai.data.load_decathlon_datalist(@data_list_file_path, is_segmentation=True, data_list_key='validation', base_dir=@data_file_base_dir)",
|
14 |
"device": "$torch.device('cuda:0' if torch.cuda.is_available() else 'cpu')",
|
15 |
"amp": true,
|
|
|
9 |
"ckpt_dir": "$@bundle_root + '/models'",
|
10 |
"output_dir": "$@bundle_root + '/eval'",
|
11 |
"data_list_file_path": "$@bundle_root + '/LUNA16_datasplit/dataset_fold0.json'",
|
12 |
+
"data_file_base_dir": "/datasets/LUNA16_Images_resample",
|
13 |
"test_datalist": "$monai.data.load_decathlon_datalist(@data_list_file_path, is_segmentation=True, data_list_key='validation', base_dir=@data_file_base_dir)",
|
14 |
"device": "$torch.device('cuda:0' if torch.cuda.is_available() else 'cpu')",
|
15 |
"amp": true,
|
configs/metadata.json
CHANGED
@@ -1,7 +1,8 @@
|
|
1 |
{
|
2 |
"schema": "https://github.com/Project-MONAI/MONAI-extra-test-data/releases/download/0.8.1/meta_schema_20220324.json",
|
3 |
-
"version": "0.4.
|
4 |
"changelog": {
|
|
|
5 |
"0.4.3": "update val patch size to avoid warning in monai 1.0.1",
|
6 |
"0.4.2": "update to use monai 1.0.1",
|
7 |
"0.4.1": "fix license Copyright error",
|
@@ -29,8 +30,10 @@
|
|
29 |
"label_classes": "dict data, containing Nx6 box and Nx1 classification labels.",
|
30 |
"pred_classes": "dict data, containing Nx6 box, Nx1 classification labels, Nx1 classification scores.",
|
31 |
"eval_metrics": {
|
32 |
-
"
|
33 |
-
"
|
|
|
|
|
34 |
},
|
35 |
"intended_use": "This is an example, not to be used for diagnostic purposes",
|
36 |
"references": [
|
|
|
1 |
{
|
2 |
"schema": "https://github.com/Project-MONAI/MONAI-extra-test-data/releases/download/0.8.1/meta_schema_20220324.json",
|
3 |
+
"version": "0.4.4",
|
4 |
"changelog": {
|
5 |
+
"0.4.4": "add data resource to readme",
|
6 |
"0.4.3": "update val patch size to avoid warning in monai 1.0.1",
|
7 |
"0.4.2": "update to use monai 1.0.1",
|
8 |
"0.4.1": "fix license Copyright error",
|
|
|
30 |
"label_classes": "dict data, containing Nx6 box and Nx1 classification labels.",
|
31 |
"pred_classes": "dict data, containing Nx6 box, Nx1 classification labels, Nx1 classification scores.",
|
32 |
"eval_metrics": {
|
33 |
+
"mAP_IoU_0.10_0.50_0.05_MaxDet_100": 0.853,
|
34 |
+
"AP_IoU_0.10_MaxDet_100": 0.862,
|
35 |
+
"mAR_IoU_0.10_0.50_0.05_MaxDet_100": 0.994,
|
36 |
+
"AR_IoU_0.10_MaxDet_100": 1.0
|
37 |
},
|
38 |
"intended_use": "This is an example, not to be used for diagnostic purposes",
|
39 |
"references": [
|
configs/train.json
CHANGED
@@ -7,14 +7,14 @@
|
|
7 |
"ckpt_dir": "$@bundle_root + '/models'",
|
8 |
"output_dir": "$@bundle_root + '/eval'",
|
9 |
"data_list_file_path": "$@bundle_root + '/LUNA16_datasplit/dataset_fold0.json'",
|
10 |
-
"data_file_base_dir": "/
|
11 |
"train_datalist": "$monai.data.load_decathlon_datalist(@data_list_file_path, is_segmentation=True, data_list_key='training', base_dir=@data_file_base_dir)",
|
12 |
"device": "$torch.device('cuda:0' if torch.cuda.is_available() else 'cpu')",
|
13 |
"epochs": 300,
|
14 |
"val_interval": 10,
|
15 |
"learning_rate": 0.01,
|
16 |
"amp": true,
|
17 |
-
"batch_size":
|
18 |
"patch_size": [
|
19 |
192,
|
20 |
192,
|
|
|
7 |
"ckpt_dir": "$@bundle_root + '/models'",
|
8 |
"output_dir": "$@bundle_root + '/eval'",
|
9 |
"data_list_file_path": "$@bundle_root + '/LUNA16_datasplit/dataset_fold0.json'",
|
10 |
+
"data_file_base_dir": "/datasets/LUNA16_Images_resample",
|
11 |
"train_datalist": "$monai.data.load_decathlon_datalist(@data_list_file_path, is_segmentation=True, data_list_key='training', base_dir=@data_file_base_dir)",
|
12 |
"device": "$torch.device('cuda:0' if torch.cuda.is_available() else 'cpu')",
|
13 |
"epochs": 300,
|
14 |
"val_interval": 10,
|
15 |
"learning_rate": 0.01,
|
16 |
"amp": true,
|
17 |
+
"batch_size": 4,
|
18 |
"patch_size": [
|
19 |
192,
|
20 |
192,
|
docs/README.md
CHANGED
@@ -1,16 +1,13 @@
|
|
1 |
-
# Description
|
2 |
-
A pre-trained model for volumetric (3D) detection of the lung lesion from CT image.
|
3 |
-
|
4 |
# Model Overview
|
5 |
-
|
6 |
|
7 |
-
|
8 |
|
9 |
-
|
10 |
|
11 |
## 1. Data
|
12 |
### 1.1 Data description
|
13 |
-
The dataset we are experimenting in this example is LUNA16 (https://luna16.grand-challenge.org/Home/), which is based on [LIDC
|
14 |
|
15 |
LUNA16 is a public dataset of CT lung nodule detection. Using raw CT scans, the goal is to identify locations of possible nodules, and to assign a probability for being a nodule to each location.
|
16 |
|
@@ -29,31 +26,44 @@ In this model, we resampled them into 0.703125 x 0.703125 x 1.25 mm.
|
|
29 |
|
30 |
Please following the instruction in Section 3.1 of https://github.com/Project-MONAI/tutorials/tree/main/detection to do the resampling.
|
31 |
|
|
|
|
|
|
|
|
|
|
|
32 |
## 2. Training configuration
|
33 |
-
The training was
|
|
|
|
|
34 |
|
35 |
Actual Model Input: 192 x 192 x 80
|
36 |
|
37 |
-
|
38 |
-
|
|
|
|
|
|
|
|
|
|
|
|
|
|
|
|
|
|
|
|
|
|
|
39 |
|
40 |
-
|
41 |
-
list of dictionary of predicted box, classification label, and classification score in evaluation mode.
|
42 |
|
43 |
-
##
|
44 |
-
The
|
45 |
|
46 |
-
|
47 |
|
48 |
-
|
49 |
-
| :---: | :---: | :---: | :---: | :---: | :---: | :---: | :---: |
|
50 |
-
| [Liu et al. (2019)](https://arxiv.org/pdf/1906.03467.pdf) | **0.848** | 0.876 | 0.905 | 0.933 | 0.943 | 0.957 | 0.970 |
|
51 |
-
| [nnDetection (2021)](https://arxiv.org/pdf/2106.00817.pdf) | 0.812 | **0.885** | 0.927 | 0.950 | 0.969 | 0.979 | 0.985 |
|
52 |
-
| MONAI detection | 0.835 | **0.885** | **0.931** | **0.957** | **0.974** | **0.983** | **0.988** |
|
53 |
|
54 |
-
|
55 |
|
56 |
-
##
|
57 |
Execute training:
|
58 |
```
|
59 |
python -m monai.bundle run training --meta_file configs/metadata.json --config_file configs/train.json --logging_file configs/logging.conf
|
@@ -64,11 +74,11 @@ Override the `train` config to execute evaluation with the trained model:
|
|
64 |
python -m monai.bundle run evaluating --meta_file configs/metadata.json --config_file "['configs/train.json','configs/evaluate.json']" --logging_file configs/logging.conf
|
65 |
```
|
66 |
|
67 |
-
Execute inference on resampled LUNA16 images
|
68 |
```
|
69 |
python -m monai.bundle run evaluating --meta_file configs/metadata.json --config_file configs/inference.json --logging_file configs/logging.conf
|
70 |
```
|
71 |
-
With the same command, we can execute inference on
|
72 |
|
73 |
Note that in inference.json, the transform "LoadImaged" in "preprocessing" and "AffineBoxToWorldCoordinated" in "postprocessing" has `"affine_lps_to_ras": true`.
|
74 |
This depends on the input images. LUNA16 needs `"affine_lps_to_ras": true`.
|
|
|
|
|
|
|
|
|
1 |
# Model Overview
|
2 |
+
A pre-trained model for volumetric (3D) detection of the lung nodule from CT image.
|
3 |
|
4 |
+
This model is trained on LUNA16 dataset (https://luna16.grand-challenge.org/Home/), using the RetinaNet (Lin, Tsung-Yi, et al. "Focal loss for dense object detection." ICCV 2017. https://arxiv.org/abs/1708.02002).
|
5 |
|
6 |
+
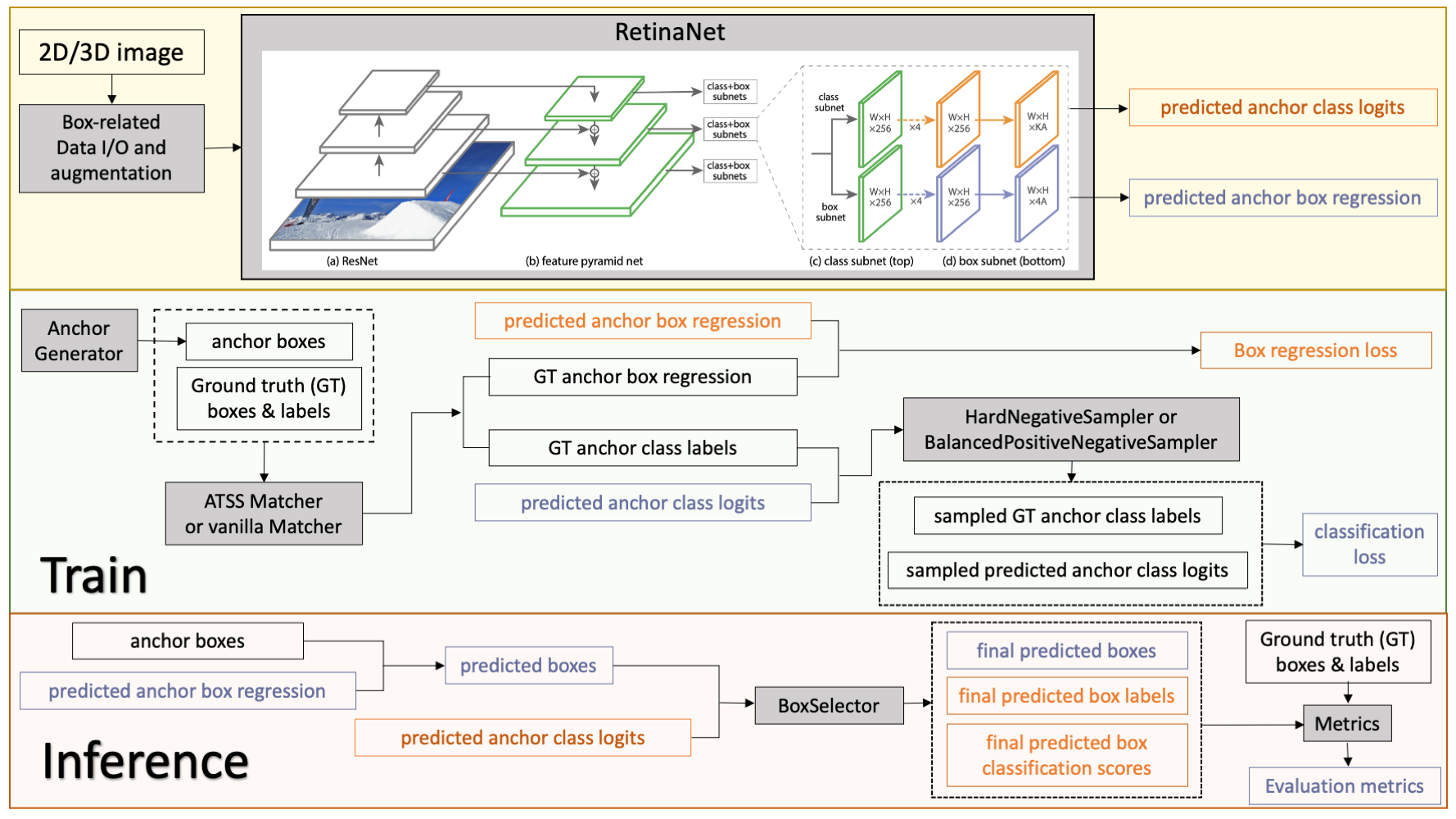
|
7 |
|
8 |
## 1. Data
|
9 |
### 1.1 Data description
|
10 |
+
The dataset we are experimenting in this example is LUNA16 (https://luna16.grand-challenge.org/Home/), which is based on [LIDC-IDRI database](https://wiki.cancerimagingarchive.net/display/Public/LIDC-IDRI) [3,4,5].
|
11 |
|
12 |
LUNA16 is a public dataset of CT lung nodule detection. Using raw CT scans, the goal is to identify locations of possible nodules, and to assign a probability for being a nodule to each location.
|
13 |
|
|
|
26 |
|
27 |
Please following the instruction in Section 3.1 of https://github.com/Project-MONAI/tutorials/tree/main/detection to do the resampling.
|
28 |
|
29 |
+
### 1.4 Data download
|
30 |
+
The mhd/raw original data can be downloaded from [LUNA16](https://luna16.grand-challenge.org/Home/). The DICOM original data can be downloaded from [LIDC-IDRI database](https://wiki.cancerimagingarchive.net/display/Public/LIDC-IDRI) [3,4,5]. You will need to resample the original data to start training.
|
31 |
+
|
32 |
+
Alternatively, we provide [resampled nifti images](https://drive.google.com/drive/folders/1JozrufA1VIZWJIc5A1EMV3J4CNCYovKK?usp=share_link) and a copy of [original mhd/raw images](https://drive.google.com/drive/folders/1-enN4eNEnKmjltevKg3W2V-Aj0nriQWE?usp=share_link) from [LUNA16](https://luna16.grand-challenge.org/Home/) for users to download.
|
33 |
+
|
34 |
## 2. Training configuration
|
35 |
+
The training was the following:
|
36 |
+
|
37 |
+
GPU: at least 16GB GPU memory
|
38 |
|
39 |
Actual Model Input: 192 x 192 x 80
|
40 |
|
41 |
+
AMP: True
|
42 |
+
|
43 |
+
Optimizer: Adam
|
44 |
+
|
45 |
+
Learning Rate: 1e-2
|
46 |
+
|
47 |
+
Loss: BCE loss and L1 loss
|
48 |
+
|
49 |
+
### Input
|
50 |
+
list of 1 channel 3D CT patches
|
51 |
+
|
52 |
+
### Output
|
53 |
+
In training mode: dictionary of classification and box regression loss in training mode;
|
54 |
|
55 |
+
In evaluation mode: list of dictionary of predicted box, classification label, and classification score in evaluation mode.
|
|
|
56 |
|
57 |
+
## 3. Performance
|
58 |
+
Coco metric is used for evaluating the performance of the model. The pre-trained model was trained and validated on data fold 0. This model achieves a mAP=0.853, mAR=0.994, AP(IoU=0.1)=0.862, AR(IoU=0.1)=1.0.
|
59 |
|
60 |
+
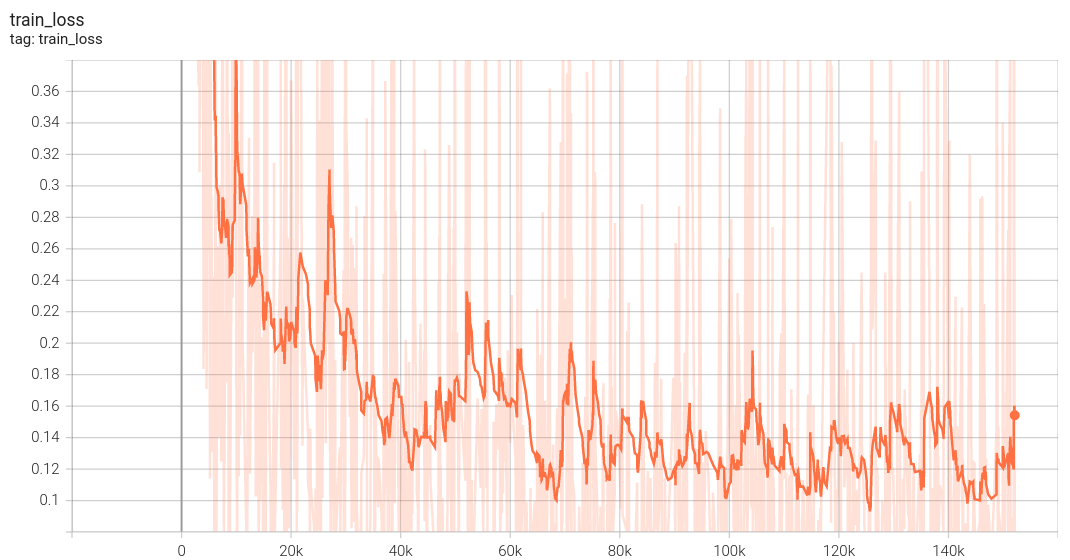
|
61 |
|
62 |
+
The validation accuracy in this curve is the mean of mAP, mAR, AP(IoU=0.1), and AR(IoU=0.1) in Coco metric.
|
|
|
|
|
|
|
|
|
63 |
|
64 |
+
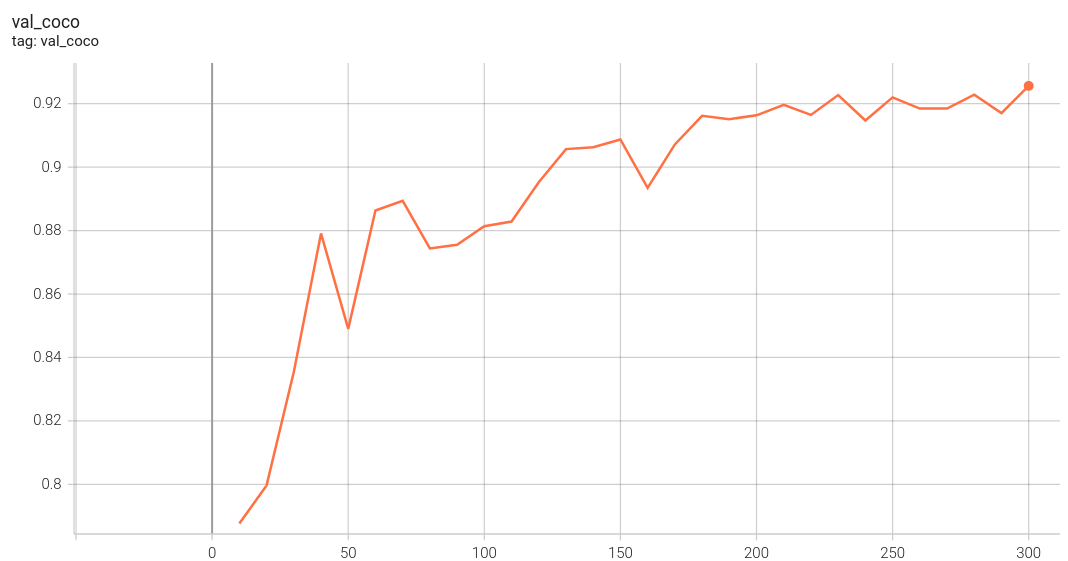
|
65 |
|
66 |
+
## 4. Commands example
|
67 |
Execute training:
|
68 |
```
|
69 |
python -m monai.bundle run training --meta_file configs/metadata.json --config_file configs/train.json --logging_file configs/logging.conf
|
|
|
74 |
python -m monai.bundle run evaluating --meta_file configs/metadata.json --config_file "['configs/train.json','configs/evaluate.json']" --logging_file configs/logging.conf
|
75 |
```
|
76 |
|
77 |
+
Execute inference on resampled LUNA16 images by setting `"whether_raw_luna16": false` in `inference.json`:
|
78 |
```
|
79 |
python -m monai.bundle run evaluating --meta_file configs/metadata.json --config_file configs/inference.json --logging_file configs/logging.conf
|
80 |
```
|
81 |
+
With the same command, we can execute inference on original LUNA16 images by setting `"whether_raw_luna16": true` in `inference.json`. Remember to also set `"data_list_file_path": "$@bundle_root + '/LUNA16_datasplit/mhd_original/dataset_fold0.json'"` and change `"data_file_base_dir"`.
|
82 |
|
83 |
Note that in inference.json, the transform "LoadImaged" in "preprocessing" and "AffineBoxToWorldCoordinated" in "postprocessing" has `"affine_lps_to_ras": true`.
|
84 |
This depends on the input images. LUNA16 needs `"affine_lps_to_ras": true`.
|