Spaces:
Configuration error
Configuration error
kzielins
commited on
Commit
·
dbf90d0
1
Parent(s):
a8dd03e
motion bert project structure added
Browse filesThis view is limited to 50 files because it contains too many changes.
See raw diff
- README.md +110 -12
- checkpoint/pose2d/256x192_res50_lr1e-3_1x.yaml +65 -0
- configs/action/MB_ft_NTU120_oneshot.yaml +35 -0
- configs/action/MB_ft_NTU60_xsub.yaml +35 -0
- configs/action/MB_ft_NTU60_xview.yaml +35 -0
- configs/action/MB_train_NTU120_oneshot.yaml +35 -0
- configs/action/MB_train_NTU60_xsub.yaml +35 -0
- configs/action/MB_train_NTU60_xview.yaml +35 -0
- configs/mesh/MB_ft_h36m.yaml +51 -0
- configs/mesh/MB_ft_pw3d.yaml +53 -0
- configs/mesh/MB_train_h36m.yaml +51 -0
- configs/mesh/MB_train_pw3d.yaml +53 -0
- configs/pose3d/MB_ft_h36m.yaml +50 -0
- configs/pose3d/MB_ft_h36m_global.yaml +50 -0
- configs/pose3d/MB_ft_h36m_global_lite.yaml +50 -0
- configs/pose3d/MB_train_h36m.yaml +51 -0
- configs/pretrain/MB_lite.yaml +53 -0
- configs/pretrain/MB_pretrain.yaml +53 -0
- docs/action.md +86 -0
- docs/inference.md +48 -0
- docs/mesh.md +61 -0
- docs/pose3d.md +51 -0
- docs/pretrain.md +81 -0
- infer_wild.py +97 -0
- infer_wild_mesh.py +157 -0
- lib/data/augmentation.py +99 -0
- lib/data/datareader_h36m.py +136 -0
- lib/data/datareader_mesh.py +59 -0
- lib/data/dataset_action.py +206 -0
- lib/data/dataset_mesh.py +97 -0
- lib/data/dataset_motion_2d.py +148 -0
- lib/data/dataset_motion_3d.py +68 -0
- lib/data/dataset_wild.py +102 -0
- lib/model/DSTformer.py +362 -0
- lib/model/drop.py +43 -0
- lib/model/loss.py +204 -0
- lib/model/loss_mesh.py +68 -0
- lib/model/loss_supcon.py +98 -0
- lib/model/model_action.py +71 -0
- lib/model/model_mesh.py +101 -0
- lib/utils/learning.py +102 -0
- lib/utils/tools.py +69 -0
- lib/utils/utils_data.py +112 -0
- lib/utils/utils_mesh.py +521 -0
- lib/utils/utils_smpl.py +88 -0
- lib/utils/vismo.py +347 -0
- params/d2c_params.pkl +3 -0
- run.sh +2 -1
- tools/compress_amass.py +62 -0
- tools/convert_amass.py +67 -0
README.md
CHANGED
@@ -1,12 +1,110 @@
|
|
1 |
-
|
2 |
-
|
3 |
-
|
4 |
-
|
5 |
-
|
6 |
-
|
7 |
-
|
8 |
-
|
9 |
-
|
10 |
-
|
11 |
-
|
12 |
-
|
|
|
|
|
|
|
|
|
|
|
|
|
|
|
|
|
|
|
|
|
|
|
|
|
|
|
|
|
|
|
|
|
|
|
|
|
|
|
|
|
|
|
|
|
|
|
|
|
|
|
|
|
|
|
|
|
|
|
|
|
|
|
|
|
|
|
|
|
|
|
|
|
|
|
|
|
|
|
|
|
|
|
|
|
|
|
|
|
|
|
|
|
|
|
|
|
|
|
|
|
|
|
|
|
|
|
|
|
|
|
|
|
|
|
|
|
|
|
|
|
|
|
|
|
|
|
|
|
|
|
|
|
|
|
|
|
|
|
|
|
|
|
|
|
|
|
|
|
|
|
|
|
|
|
|
|
|
|
|
|
|
|
|
|
|
|
|
|
|
|
|
|
|
|
|
|
|
|
|
|
|
|
|
|
|
|
|
|
|
|
|
|
|
|
|
|
|
1 |
+
# MotionBERT: A Unified Perspective on Learning Human Motion Representations
|
2 |
+
|
3 |
+
<a href="https://pytorch.org/get-started/locally/"><img alt="PyTorch" src="https://img.shields.io/badge/PyTorch-ee4c2c?logo=pytorch&logoColor=white"></a> [](https://arxiv.org/abs/2210.06551) <a href="https://motionbert.github.io/"><img alt="Project" src="https://img.shields.io/badge/-Project%20Page-lightgrey?logo=Google%20Chrome&color=informational&logoColor=white"></a> <a href="https://youtu.be/slSPQ9hNLjM"><img alt="Demo" src="https://img.shields.io/badge/-Demo-ea3323?logo=youtube"></a> [](https://huggingface.co/walterzhu/MotionBERT)
|
4 |
+
|
5 |
+
[](https://paperswithcode.com/sota/monocular-3d-human-pose-estimation-on-human3?p=motionbert-unified-pretraining-for-human)
|
6 |
+
[](https://paperswithcode.com/sota/one-shot-3d-action-recognition-on-ntu-rgbd?p=motionbert-unified-pretraining-for-human)
|
7 |
+
[](https://paperswithcode.com/sota/3d-human-pose-estimation-on-3dpw?p=motionbert-unified-pretraining-for-human)
|
8 |
+
|
9 |
+
This is the official PyTorch implementation of the paper *"[MotionBERT: A Unified Perspective on Learning Human Motion Representations](https://arxiv.org/pdf/2210.06551.pdf)"* (ICCV 2023).
|
10 |
+
|
11 |
+
<img src="https://motionbert.github.io/assets/teaser.gif" alt="" style="zoom: 60%;" />
|
12 |
+
|
13 |
+
## Installation
|
14 |
+
|
15 |
+
```bash
|
16 |
+
conda create -n motionbert python=3.7 anaconda
|
17 |
+
conda activate motionbert
|
18 |
+
# Please install PyTorch according to your CUDA version.
|
19 |
+
conda install pytorch torchvision torchaudio pytorch-cuda=11.6 -c pytorch -c nvidia
|
20 |
+
pip install -r requirements.txt
|
21 |
+
```
|
22 |
+
|
23 |
+
|
24 |
+
|
25 |
+
## Getting Started
|
26 |
+
|
27 |
+
| Task | Document |
|
28 |
+
| --------------------------------- | ------------------------------------------------------------ |
|
29 |
+
| Pretrain | [docs/pretrain.md](docs/pretrain.md) |
|
30 |
+
| 3D human pose estimation | [docs/pose3d.md](docs/pose3d.md) |
|
31 |
+
| Skeleton-based action recognition | [docs/action.md](docs/action.md) |
|
32 |
+
| Mesh recovery | [docs/mesh.md](docs/mesh.md) |
|
33 |
+
|
34 |
+
|
35 |
+
|
36 |
+
## Applications
|
37 |
+
|
38 |
+
### In-the-wild inference (for custom videos)
|
39 |
+
|
40 |
+
Please refer to [docs/inference.md](docs/inference.md).
|
41 |
+
|
42 |
+
### Using MotionBERT for *human-centric* video representations
|
43 |
+
|
44 |
+
```python
|
45 |
+
'''
|
46 |
+
x: 2D skeletons
|
47 |
+
type = <class 'torch.Tensor'>
|
48 |
+
shape = [batch size * frames * joints(17) * channels(3)]
|
49 |
+
|
50 |
+
MotionBERT: pretrained human motion encoder
|
51 |
+
type = <class 'lib.model.DSTformer.DSTformer'>
|
52 |
+
|
53 |
+
E: encoded motion representation
|
54 |
+
type = <class 'torch.Tensor'>
|
55 |
+
shape = [batch size * frames * joints(17) * channels(512)]
|
56 |
+
'''
|
57 |
+
E = MotionBERT.get_representation(x)
|
58 |
+
```
|
59 |
+
|
60 |
+
|
61 |
+
|
62 |
+
> **Hints**
|
63 |
+
>
|
64 |
+
> 1. The model could handle different input lengths (no more than 243 frames). No need to explicitly specify the input length elsewhere.
|
65 |
+
> 2. The model uses 17 body keypoints ([H36M format](https://github.com/JimmySuen/integral-human-pose/blob/master/pytorch_projects/common_pytorch/dataset/hm36.py#L32)). If you are using other formats, please convert them before feeding to MotionBERT.
|
66 |
+
> 3. Please refer to [model_action.py](lib/model/model_action.py) and [model_mesh.py](lib/model/model_mesh.py) for examples of (easily) adapting MotionBERT to different downstream tasks.
|
67 |
+
> 4. For RGB videos, you need to extract 2D poses ([inference.md](docs/inference.md)), convert the keypoint format ([dataset_wild.py](lib/data/dataset_wild.py)), and then feed to MotionBERT ([infer_wild.py](infer_wild.py)).
|
68 |
+
>
|
69 |
+
|
70 |
+
|
71 |
+
|
72 |
+
## Model Zoo
|
73 |
+
|
74 |
+
<img src="https://motionbert.github.io/assets/demo.gif" alt="" style="zoom: 50%;" />
|
75 |
+
|
76 |
+
| Model | Download Link | Config | Performance |
|
77 |
+
| ------------------------------- | ------------------------------------------------------------ | ------------------------------------------------------------ | ---------------- |
|
78 |
+
| MotionBERT (162MB) | [OneDrive](https://1drv.ms/f/s!AvAdh0LSjEOlgS425shtVi9e5reN?e=6UeBa2) | [pretrain/MB_pretrain.yaml](configs/pretrain/MB_pretrain.yaml) | - |
|
79 |
+
| MotionBERT-Lite (61MB) | [OneDrive](https://1drv.ms/f/s!AvAdh0LSjEOlgS27Ydcbpxlkl0ng?e=rq2Btn) | [pretrain/MB_lite.yaml](configs/pretrain/MB_lite.yaml) | - |
|
80 |
+
| 3D Pose (H36M-SH, scratch) | [OneDrive](https://1drv.ms/f/s!AvAdh0LSjEOlgSvNejMQ0OHxMGZC?e=KcwBk1) | [pose3d/MB_train_h36m.yaml](configs/pose3d/MB_train_h36m.yaml) | 39.2mm (MPJPE) |
|
81 |
+
| 3D Pose (H36M-SH, ft) | [OneDrive](https://1drv.ms/f/s!AvAdh0LSjEOlgSoTqtyR5Zsgi8_Z?e=rn4VJf) | [pose3d/MB_ft_h36m.yaml](configs/pose3d/MB_ft_h36m.yaml) | 37.2mm (MPJPE) |
|
82 |
+
| Action Recognition (x-sub, ft) | [OneDrive](https://1drv.ms/f/s!AvAdh0LSjEOlgTX23yT_NO7RiZz-?e=nX6w2j) | [action/MB_ft_NTU60_xsub.yaml](configs/action/MB_ft_NTU60_xsub.yaml) | 97.2% (Top1 Acc) |
|
83 |
+
| Action Recognition (x-view, ft) | [OneDrive](https://1drv.ms/f/s!AvAdh0LSjEOlgTaNiXw2Nal-g37M?e=lSkE4T) | [action/MB_ft_NTU60_xview.yaml](configs/action/MB_ft_NTU60_xview.yaml) | 93.0% (Top1 Acc) |
|
84 |
+
| Mesh (with 3DPW, ft) | [OneDrive](https://1drv.ms/f/s!AvAdh0LSjEOlgTmgYNslCDWMNQi9?e=WjcB1F) | [mesh/MB_ft_pw3d.yaml](configs/mesh/MB_ft_pw3d.yaml) | 88.1mm (MPVE) |
|
85 |
+
|
86 |
+
In most use cases (especially with finetuning), `MotionBERT-Lite` gives a similar performance with lower computation overhead.
|
87 |
+
|
88 |
+
|
89 |
+
|
90 |
+
## TODO
|
91 |
+
|
92 |
+
- [x] Scripts and docs for pretraining
|
93 |
+
|
94 |
+
- [x] Demo for custom videos
|
95 |
+
|
96 |
+
|
97 |
+
|
98 |
+
## Citation
|
99 |
+
|
100 |
+
If you find our work useful for your project, please consider citing the paper:
|
101 |
+
|
102 |
+
```bibtex
|
103 |
+
@inproceedings{motionbert2022,
|
104 |
+
title = {MotionBERT: A Unified Perspective on Learning Human Motion Representations},
|
105 |
+
author = {Zhu, Wentao and Ma, Xiaoxuan and Liu, Zhaoyang and Liu, Libin and Wu, Wayne and Wang, Yizhou},
|
106 |
+
booktitle = {Proceedings of the IEEE/CVF International Conference on Computer Vision},
|
107 |
+
year = {2023},
|
108 |
+
}
|
109 |
+
```
|
110 |
+
|
checkpoint/pose2d/256x192_res50_lr1e-3_1x.yaml
ADDED
@@ -0,0 +1,65 @@
|
|
|
|
|
|
|
|
|
|
|
|
|
|
|
|
|
|
|
|
|
|
|
|
|
|
|
|
|
|
|
|
|
|
|
|
|
|
|
|
|
|
|
|
|
|
|
|
|
|
|
|
|
|
|
|
|
|
|
|
|
|
|
|
|
|
|
|
|
|
|
|
|
|
|
|
|
|
|
|
|
|
|
|
|
|
|
|
|
|
|
|
|
|
|
|
|
|
|
|
|
|
|
|
|
|
|
|
|
|
|
|
|
|
|
|
|
|
|
|
|
|
|
|
|
|
|
|
|
|
|
|
|
1 |
+
DATASET:
|
2 |
+
TRAIN:
|
3 |
+
TYPE: 'Halpe_26'
|
4 |
+
ROOT: './data/halpe/'
|
5 |
+
IMG_PREFIX: 'images/train2015'
|
6 |
+
ANN: 'annotations/halpe_train_v1.json'
|
7 |
+
AUG:
|
8 |
+
FLIP: true
|
9 |
+
ROT_FACTOR: 40
|
10 |
+
SCALE_FACTOR: 0.3
|
11 |
+
NUM_JOINTS_HALF_BODY: 11
|
12 |
+
PROB_HALF_BODY: -1
|
13 |
+
VAL:
|
14 |
+
TYPE: 'Halpe_26'
|
15 |
+
ROOT: './data/halpe/'
|
16 |
+
IMG_PREFIX: 'images/val2017'
|
17 |
+
ANN: 'annotations/halpe_val_v1.json'
|
18 |
+
TEST:
|
19 |
+
TYPE: 'Halpe_26_det'
|
20 |
+
ROOT: './data/halpe/'
|
21 |
+
IMG_PREFIX: 'images/val2017'
|
22 |
+
DET_FILE: './exp/json/test_det_yolo.json'
|
23 |
+
ANN: 'annotations/halpe_val_v1.json'
|
24 |
+
DATA_PRESET:
|
25 |
+
TYPE: 'simple'
|
26 |
+
SIGMA: 2
|
27 |
+
NUM_JOINTS: 26
|
28 |
+
IMAGE_SIZE:
|
29 |
+
- 256
|
30 |
+
- 192
|
31 |
+
HEATMAP_SIZE:
|
32 |
+
- 64
|
33 |
+
- 48
|
34 |
+
MODEL:
|
35 |
+
TYPE: 'FastPose'
|
36 |
+
PRETRAINED: ''
|
37 |
+
TRY_LOAD: ''
|
38 |
+
NUM_DECONV_FILTERS:
|
39 |
+
- 256
|
40 |
+
- 256
|
41 |
+
- 256
|
42 |
+
NUM_LAYERS: 50
|
43 |
+
LOSS:
|
44 |
+
TYPE: 'MSELoss'
|
45 |
+
DETECTOR:
|
46 |
+
NAME: 'yolo'
|
47 |
+
CONFIG: 'detector/yolo/cfg/yolov3-spp.cfg'
|
48 |
+
WEIGHTS: 'detector/yolo/data/yolov3-spp.weights'
|
49 |
+
NMS_THRES: 0.6
|
50 |
+
CONFIDENCE: 0.05
|
51 |
+
TRAIN:
|
52 |
+
WORLD_SIZE: 4
|
53 |
+
BATCH_SIZE: 48
|
54 |
+
BEGIN_EPOCH: 0
|
55 |
+
END_EPOCH: 200
|
56 |
+
OPTIMIZER: 'adam'
|
57 |
+
LR: 0.001
|
58 |
+
LR_FACTOR: 0.1
|
59 |
+
LR_STEP:
|
60 |
+
- 50
|
61 |
+
- 70
|
62 |
+
DPG_MILESTONE: 90
|
63 |
+
DPG_STEP:
|
64 |
+
- 110
|
65 |
+
- 130
|
configs/action/MB_ft_NTU120_oneshot.yaml
ADDED
@@ -0,0 +1,35 @@
|
|
|
|
|
|
|
|
|
|
|
|
|
|
|
|
|
|
|
|
|
|
|
|
|
|
|
|
|
|
|
|
|
|
|
|
|
|
|
|
|
|
|
|
|
|
|
|
|
|
|
|
|
|
|
|
|
|
|
|
|
|
|
|
|
|
|
|
|
|
|
|
|
1 |
+
# General
|
2 |
+
finetune: True
|
3 |
+
partial_train: null
|
4 |
+
|
5 |
+
# Traning
|
6 |
+
n_views: 2
|
7 |
+
temp: 0.1
|
8 |
+
|
9 |
+
epochs: 50
|
10 |
+
batch_size: 32
|
11 |
+
lr_backbone: 0.0001
|
12 |
+
lr_head: 0.001
|
13 |
+
weight_decay: 0.01
|
14 |
+
lr_decay: 0.99
|
15 |
+
|
16 |
+
# Model
|
17 |
+
model_version: embed
|
18 |
+
maxlen: 243
|
19 |
+
dim_feat: 512
|
20 |
+
mlp_ratio: 2
|
21 |
+
depth: 5
|
22 |
+
dim_rep: 512
|
23 |
+
num_heads: 8
|
24 |
+
att_fuse: True
|
25 |
+
num_joints: 17
|
26 |
+
hidden_dim: 2048
|
27 |
+
dropout_ratio: 0.1
|
28 |
+
|
29 |
+
# Data
|
30 |
+
clip_len: 100
|
31 |
+
|
32 |
+
# Augmentation
|
33 |
+
random_move: True
|
34 |
+
scale_range_train: [1, 3]
|
35 |
+
scale_range_test: [2, 2]
|
configs/action/MB_ft_NTU60_xsub.yaml
ADDED
@@ -0,0 +1,35 @@
|
|
|
|
|
|
|
|
|
|
|
|
|
|
|
|
|
|
|
|
|
|
|
|
|
|
|
|
|
|
|
|
|
|
|
|
|
|
|
|
|
|
|
|
|
|
|
|
|
|
|
|
|
|
|
|
|
|
|
|
|
|
|
|
|
|
|
|
|
|
|
|
|
1 |
+
# General
|
2 |
+
finetune: True
|
3 |
+
partial_train: null
|
4 |
+
|
5 |
+
# Traning
|
6 |
+
epochs: 300
|
7 |
+
batch_size: 32
|
8 |
+
lr_backbone: 0.0001
|
9 |
+
lr_head: 0.001
|
10 |
+
weight_decay: 0.01
|
11 |
+
lr_decay: 0.99
|
12 |
+
|
13 |
+
# Model
|
14 |
+
model_version: class
|
15 |
+
maxlen: 243
|
16 |
+
dim_feat: 512
|
17 |
+
mlp_ratio: 2
|
18 |
+
depth: 5
|
19 |
+
dim_rep: 512
|
20 |
+
num_heads: 8
|
21 |
+
att_fuse: True
|
22 |
+
num_joints: 17
|
23 |
+
hidden_dim: 2048
|
24 |
+
dropout_ratio: 0.5
|
25 |
+
|
26 |
+
# Data
|
27 |
+
dataset: ntu60_hrnet
|
28 |
+
data_split: xsub
|
29 |
+
clip_len: 243
|
30 |
+
action_classes: 60
|
31 |
+
|
32 |
+
# Augmentation
|
33 |
+
random_move: True
|
34 |
+
scale_range_train: [1, 3]
|
35 |
+
scale_range_test: [2, 2]
|
configs/action/MB_ft_NTU60_xview.yaml
ADDED
@@ -0,0 +1,35 @@
|
|
|
|
|
|
|
|
|
|
|
|
|
|
|
|
|
|
|
|
|
|
|
|
|
|
|
|
|
|
|
|
|
|
|
|
|
|
|
|
|
|
|
|
|
|
|
|
|
|
|
|
|
|
|
|
|
|
|
|
|
|
|
|
|
|
|
|
|
|
|
|
|
1 |
+
# General
|
2 |
+
finetune: True
|
3 |
+
partial_train: null
|
4 |
+
|
5 |
+
# Traning
|
6 |
+
epochs: 300
|
7 |
+
batch_size: 32
|
8 |
+
lr_backbone: 0.0001
|
9 |
+
lr_head: 0.001
|
10 |
+
weight_decay: 0.01
|
11 |
+
lr_decay: 0.99
|
12 |
+
|
13 |
+
# Model
|
14 |
+
model_version: class
|
15 |
+
maxlen: 243
|
16 |
+
dim_feat: 512
|
17 |
+
mlp_ratio: 2
|
18 |
+
depth: 5
|
19 |
+
dim_rep: 512
|
20 |
+
num_heads: 8
|
21 |
+
att_fuse: True
|
22 |
+
num_joints: 17
|
23 |
+
hidden_dim: 2048
|
24 |
+
dropout_ratio: 0.5
|
25 |
+
|
26 |
+
# Data
|
27 |
+
dataset: ntu60_hrnet
|
28 |
+
data_split: xview
|
29 |
+
clip_len: 243
|
30 |
+
action_classes: 60
|
31 |
+
|
32 |
+
# Augmentation
|
33 |
+
random_move: True
|
34 |
+
scale_range_train: [1, 3]
|
35 |
+
scale_range_test: [2, 2]
|
configs/action/MB_train_NTU120_oneshot.yaml
ADDED
@@ -0,0 +1,35 @@
|
|
|
|
|
|
|
|
|
|
|
|
|
|
|
|
|
|
|
|
|
|
|
|
|
|
|
|
|
|
|
|
|
|
|
|
|
|
|
|
|
|
|
|
|
|
|
|
|
|
|
|
|
|
|
|
|
|
|
|
|
|
|
|
|
|
|
|
|
|
|
|
|
1 |
+
# General
|
2 |
+
finetune: False
|
3 |
+
partial_train: null
|
4 |
+
|
5 |
+
# Traning
|
6 |
+
n_views: 2
|
7 |
+
temp: 0.1
|
8 |
+
|
9 |
+
epochs: 50
|
10 |
+
batch_size: 32
|
11 |
+
lr_backbone: 0.0001
|
12 |
+
lr_head: 0.001
|
13 |
+
weight_decay: 0.01
|
14 |
+
lr_decay: 0.99
|
15 |
+
|
16 |
+
# Model
|
17 |
+
model_version: embed
|
18 |
+
maxlen: 243
|
19 |
+
dim_feat: 512
|
20 |
+
mlp_ratio: 2
|
21 |
+
depth: 5
|
22 |
+
dim_rep: 512
|
23 |
+
num_heads: 8
|
24 |
+
att_fuse: True
|
25 |
+
num_joints: 17
|
26 |
+
hidden_dim: 2048
|
27 |
+
dropout_ratio: 0.1
|
28 |
+
|
29 |
+
# Data
|
30 |
+
clip_len: 100
|
31 |
+
|
32 |
+
# Augmentation
|
33 |
+
random_move: True
|
34 |
+
scale_range_train: [1, 3]
|
35 |
+
scale_range_test: [2, 2]
|
configs/action/MB_train_NTU60_xsub.yaml
ADDED
@@ -0,0 +1,35 @@
|
|
|
|
|
|
|
|
|
|
|
|
|
|
|
|
|
|
|
|
|
|
|
|
|
|
|
|
|
|
|
|
|
|
|
|
|
|
|
|
|
|
|
|
|
|
|
|
|
|
|
|
|
|
|
|
|
|
|
|
|
|
|
|
|
|
|
|
|
|
|
|
|
1 |
+
# General
|
2 |
+
finetune: False
|
3 |
+
partial_train: null
|
4 |
+
|
5 |
+
# Traning
|
6 |
+
epochs: 300
|
7 |
+
batch_size: 32
|
8 |
+
lr_backbone: 0.0001
|
9 |
+
lr_head: 0.0001
|
10 |
+
weight_decay: 0.01
|
11 |
+
lr_decay: 0.99
|
12 |
+
|
13 |
+
# Model
|
14 |
+
model_version: class
|
15 |
+
maxlen: 243
|
16 |
+
dim_feat: 512
|
17 |
+
mlp_ratio: 2
|
18 |
+
depth: 5
|
19 |
+
dim_rep: 512
|
20 |
+
num_heads: 8
|
21 |
+
att_fuse: True
|
22 |
+
num_joints: 17
|
23 |
+
hidden_dim: 2048
|
24 |
+
dropout_ratio: 0.5
|
25 |
+
|
26 |
+
# Data
|
27 |
+
dataset: ntu60_hrnet
|
28 |
+
data_split: xsub
|
29 |
+
clip_len: 243
|
30 |
+
action_classes: 60
|
31 |
+
|
32 |
+
# Augmentation
|
33 |
+
random_move: True
|
34 |
+
scale_range_train: [1, 3]
|
35 |
+
scale_range_test: [2, 2]
|
configs/action/MB_train_NTU60_xview.yaml
ADDED
@@ -0,0 +1,35 @@
|
|
|
|
|
|
|
|
|
|
|
|
|
|
|
|
|
|
|
|
|
|
|
|
|
|
|
|
|
|
|
|
|
|
|
|
|
|
|
|
|
|
|
|
|
|
|
|
|
|
|
|
|
|
|
|
|
|
|
|
|
|
|
|
|
|
|
|
|
|
|
|
|
1 |
+
# General
|
2 |
+
finetune: False
|
3 |
+
partial_train: null
|
4 |
+
|
5 |
+
# Traning
|
6 |
+
epochs: 300
|
7 |
+
batch_size: 32
|
8 |
+
lr_backbone: 0.0001
|
9 |
+
lr_head: 0.0001
|
10 |
+
weight_decay: 0.01
|
11 |
+
lr_decay: 0.99
|
12 |
+
|
13 |
+
# Model
|
14 |
+
model_version: class
|
15 |
+
maxlen: 243
|
16 |
+
dim_feat: 512
|
17 |
+
mlp_ratio: 2
|
18 |
+
depth: 5
|
19 |
+
dim_rep: 512
|
20 |
+
num_heads: 8
|
21 |
+
att_fuse: True
|
22 |
+
num_joints: 17
|
23 |
+
hidden_dim: 2048
|
24 |
+
dropout_ratio: 0.5
|
25 |
+
|
26 |
+
# Data
|
27 |
+
dataset: ntu60_hrnet
|
28 |
+
data_split: xview
|
29 |
+
clip_len: 243
|
30 |
+
action_classes: 60
|
31 |
+
|
32 |
+
# Augmentation
|
33 |
+
random_move: True
|
34 |
+
scale_range_train: [1, 3]
|
35 |
+
scale_range_test: [2, 2]
|
configs/mesh/MB_ft_h36m.yaml
ADDED
@@ -0,0 +1,51 @@
|
|
|
|
|
|
|
|
|
|
|
|
|
|
|
|
|
|
|
|
|
|
|
|
|
|
|
|
|
|
|
|
|
|
|
|
|
|
|
|
|
|
|
|
|
|
|
|
|
|
|
|
|
|
|
|
|
|
|
|
|
|
|
|
|
|
|
|
|
|
|
|
|
|
|
|
|
|
|
|
|
|
|
|
|
|
|
|
|
|
|
|
|
|
|
|
|
|
|
|
|
|
|
|
|
1 |
+
# General
|
2 |
+
finetune: True
|
3 |
+
partial_train: null
|
4 |
+
train_pw3d: False
|
5 |
+
warmup_h36m: 100
|
6 |
+
|
7 |
+
# Traning
|
8 |
+
epochs: 60
|
9 |
+
checkpoint_frequency: 20
|
10 |
+
batch_size: 128
|
11 |
+
batch_size_img: 512
|
12 |
+
dropout: 0.1
|
13 |
+
dropout_loc: 1
|
14 |
+
lr_backbone: 0.00005
|
15 |
+
lr_head: 0.0005
|
16 |
+
weight_decay: 0.01
|
17 |
+
lr_decay: 0.98
|
18 |
+
|
19 |
+
# Model
|
20 |
+
maxlen: 243
|
21 |
+
dim_feat: 512
|
22 |
+
mlp_ratio: 2
|
23 |
+
depth: 5
|
24 |
+
dim_rep: 512
|
25 |
+
num_heads: 8
|
26 |
+
att_fuse: True
|
27 |
+
hidden_dim: 1024
|
28 |
+
|
29 |
+
# Data
|
30 |
+
data_root: data/mesh
|
31 |
+
dt_file_h36m: mesh_det_h36m.pkl
|
32 |
+
clip_len: 16
|
33 |
+
data_stride: 8
|
34 |
+
sample_stride: 1
|
35 |
+
num_joints: 17
|
36 |
+
|
37 |
+
# Loss
|
38 |
+
lambda_3d: 0.5
|
39 |
+
lambda_scale: 0
|
40 |
+
lambda_3dv: 10
|
41 |
+
lambda_lv: 0
|
42 |
+
lambda_lg: 0
|
43 |
+
lambda_a: 0
|
44 |
+
lambda_av: 0
|
45 |
+
lambda_pose: 1000
|
46 |
+
lambda_shape: 1
|
47 |
+
lambda_norm: 20
|
48 |
+
loss_type: 'L1'
|
49 |
+
|
50 |
+
# Augmentation
|
51 |
+
flip: True
|
configs/mesh/MB_ft_pw3d.yaml
ADDED
@@ -0,0 +1,53 @@
|
|
|
|
|
|
|
|
|
|
|
|
|
|
|
|
|
|
|
|
|
|
|
|
|
|
|
|
|
|
|
|
|
|
|
|
|
|
|
|
|
|
|
|
|
|
|
|
|
|
|
|
|
|
|
|
|
|
|
|
|
|
|
|
|
|
|
|
|
|
|
|
|
|
|
|
|
|
|
|
|
|
|
|
|
|
|
|
|
|
|
|
|
|
|
|
|
|
|
|
|
|
|
|
|
|
|
|
|
1 |
+
# General
|
2 |
+
finetune: True
|
3 |
+
partial_train: null
|
4 |
+
train_pw3d: True
|
5 |
+
warmup_h36m: 20
|
6 |
+
warmup_coco: 100
|
7 |
+
|
8 |
+
# Traning
|
9 |
+
epochs: 60
|
10 |
+
checkpoint_frequency: 20
|
11 |
+
batch_size: 128
|
12 |
+
batch_size_img: 512
|
13 |
+
dropout: 0.1
|
14 |
+
lr_backbone: 0.00005
|
15 |
+
lr_head: 0.0005
|
16 |
+
weight_decay: 0.01
|
17 |
+
lr_decay: 0.98
|
18 |
+
|
19 |
+
# Model
|
20 |
+
maxlen: 243
|
21 |
+
dim_feat: 512
|
22 |
+
mlp_ratio: 2
|
23 |
+
depth: 5
|
24 |
+
dim_rep: 512
|
25 |
+
num_heads: 8
|
26 |
+
att_fuse: True
|
27 |
+
hidden_dim: 1024
|
28 |
+
|
29 |
+
# Data
|
30 |
+
data_root: data/mesh
|
31 |
+
dt_file_h36m: mesh_det_h36m.pkl
|
32 |
+
dt_file_coco: mesh_det_coco.pkl
|
33 |
+
dt_file_pw3d: mesh_det_pw3d.pkl
|
34 |
+
clip_len: 16
|
35 |
+
data_stride: 8
|
36 |
+
sample_stride: 1
|
37 |
+
num_joints: 17
|
38 |
+
|
39 |
+
# Loss
|
40 |
+
lambda_3d: 0.5
|
41 |
+
lambda_scale: 0
|
42 |
+
lambda_3dv: 10
|
43 |
+
lambda_lv: 0
|
44 |
+
lambda_lg: 0
|
45 |
+
lambda_a: 0
|
46 |
+
lambda_av: 0
|
47 |
+
lambda_pose: 1000
|
48 |
+
lambda_shape: 1
|
49 |
+
lambda_norm: 20
|
50 |
+
loss_type: 'L1'
|
51 |
+
|
52 |
+
# Augmentation
|
53 |
+
flip: True
|
configs/mesh/MB_train_h36m.yaml
ADDED
@@ -0,0 +1,51 @@
|
|
|
|
|
|
|
|
|
|
|
|
|
|
|
|
|
|
|
|
|
|
|
|
|
|
|
|
|
|
|
|
|
|
|
|
|
|
|
|
|
|
|
|
|
|
|
|
|
|
|
|
|
|
|
|
|
|
|
|
|
|
|
|
|
|
|
|
|
|
|
|
|
|
|
|
|
|
|
|
|
|
|
|
|
|
|
|
|
|
|
|
|
|
|
|
|
|
|
|
|
|
|
|
|
1 |
+
# General
|
2 |
+
finetune: False
|
3 |
+
partial_train: null
|
4 |
+
train_pw3d: False
|
5 |
+
warmup_h36m: 100
|
6 |
+
|
7 |
+
# Traning
|
8 |
+
epochs: 100
|
9 |
+
checkpoint_frequency: 20
|
10 |
+
batch_size: 128
|
11 |
+
batch_size_img: 512
|
12 |
+
dropout: 0.1
|
13 |
+
dropout_loc: 1
|
14 |
+
lr_backbone: 0.0001
|
15 |
+
lr_head: 0.0001
|
16 |
+
weight_decay: 0.01
|
17 |
+
lr_decay: 0.98
|
18 |
+
|
19 |
+
# Model
|
20 |
+
maxlen: 243
|
21 |
+
dim_feat: 512
|
22 |
+
mlp_ratio: 2
|
23 |
+
depth: 5
|
24 |
+
dim_rep: 512
|
25 |
+
num_heads: 8
|
26 |
+
att_fuse: True
|
27 |
+
hidden_dim: 1024
|
28 |
+
|
29 |
+
# Data
|
30 |
+
data_root: data/mesh
|
31 |
+
dt_file_h36m: mesh_det_h36m.pkl
|
32 |
+
clip_len: 16
|
33 |
+
data_stride: 8
|
34 |
+
sample_stride: 1
|
35 |
+
num_joints: 17
|
36 |
+
|
37 |
+
# Loss
|
38 |
+
lambda_3d: 0.5
|
39 |
+
lambda_scale: 0
|
40 |
+
lambda_3dv: 10
|
41 |
+
lambda_lv: 0
|
42 |
+
lambda_lg: 0
|
43 |
+
lambda_a: 0
|
44 |
+
lambda_av: 0
|
45 |
+
lambda_pose: 1000
|
46 |
+
lambda_shape: 1
|
47 |
+
lambda_norm: 20
|
48 |
+
loss_type: 'L1'
|
49 |
+
|
50 |
+
# Augmentation
|
51 |
+
flip: True
|
configs/mesh/MB_train_pw3d.yaml
ADDED
@@ -0,0 +1,53 @@
|
|
|
|
|
|
|
|
|
|
|
|
|
|
|
|
|
|
|
|
|
|
|
|
|
|
|
|
|
|
|
|
|
|
|
|
|
|
|
|
|
|
|
|
|
|
|
|
|
|
|
|
|
|
|
|
|
|
|
|
|
|
|
|
|
|
|
|
|
|
|
|
|
|
|
|
|
|
|
|
|
|
|
|
|
|
|
|
|
|
|
|
|
|
|
|
|
|
|
|
|
|
|
|
|
|
|
|
|
1 |
+
# General
|
2 |
+
finetune: False
|
3 |
+
partial_train: null
|
4 |
+
train_pw3d: True
|
5 |
+
warmup_h36m: 20
|
6 |
+
warmup_coco: 100
|
7 |
+
|
8 |
+
# Traning
|
9 |
+
epochs: 60
|
10 |
+
checkpoint_frequency: 20
|
11 |
+
batch_size: 128
|
12 |
+
batch_size_img: 512
|
13 |
+
dropout: 0.1
|
14 |
+
lr_backbone: 0.0001
|
15 |
+
lr_head: 0.0001
|
16 |
+
weight_decay: 0.01
|
17 |
+
lr_decay: 0.98
|
18 |
+
|
19 |
+
# Model
|
20 |
+
maxlen: 243
|
21 |
+
dim_feat: 512
|
22 |
+
mlp_ratio: 2
|
23 |
+
depth: 5
|
24 |
+
dim_rep: 512
|
25 |
+
num_heads: 8
|
26 |
+
att_fuse: True
|
27 |
+
hidden_dim: 1024
|
28 |
+
|
29 |
+
# Data
|
30 |
+
data_root: data/mesh
|
31 |
+
dt_file_h36m: mesh_det_h36m.pkl
|
32 |
+
dt_file_coco: mesh_det_coco.pkl
|
33 |
+
dt_file_pw3d: mesh_det_pw3d.pkl
|
34 |
+
clip_len: 16
|
35 |
+
data_stride: 8
|
36 |
+
sample_stride: 1
|
37 |
+
num_joints: 17
|
38 |
+
|
39 |
+
# Loss
|
40 |
+
lambda_3d: 0.5
|
41 |
+
lambda_scale: 0
|
42 |
+
lambda_3dv: 10
|
43 |
+
lambda_lv: 0
|
44 |
+
lambda_lg: 0
|
45 |
+
lambda_a: 0
|
46 |
+
lambda_av: 0
|
47 |
+
lambda_pose: 1000
|
48 |
+
lambda_shape: 1
|
49 |
+
lambda_norm: 20
|
50 |
+
loss_type: 'L1'
|
51 |
+
|
52 |
+
# Augmentation
|
53 |
+
flip: True
|
configs/pose3d/MB_ft_h36m.yaml
ADDED
@@ -0,0 +1,50 @@
|
|
|
|
|
|
|
|
|
|
|
|
|
|
|
|
|
|
|
|
|
|
|
|
|
|
|
|
|
|
|
|
|
|
|
|
|
|
|
|
|
|
|
|
|
|
|
|
|
|
|
|
|
|
|
|
|
|
|
|
|
|
|
|
|
|
|
|
|
|
|
|
|
|
|
|
|
|
|
|
|
|
|
|
|
|
|
|
|
|
|
|
|
|
|
|
|
|
|
|
|
|
|
1 |
+
# General
|
2 |
+
train_2d: False
|
3 |
+
no_eval: False
|
4 |
+
finetune: True
|
5 |
+
partial_train: null
|
6 |
+
|
7 |
+
# Traning
|
8 |
+
epochs: 60
|
9 |
+
checkpoint_frequency: 30
|
10 |
+
batch_size: 32
|
11 |
+
dropout: 0.0
|
12 |
+
learning_rate: 0.0002
|
13 |
+
weight_decay: 0.01
|
14 |
+
lr_decay: 0.99
|
15 |
+
|
16 |
+
# Model
|
17 |
+
maxlen: 243
|
18 |
+
dim_feat: 512
|
19 |
+
mlp_ratio: 2
|
20 |
+
depth: 5
|
21 |
+
dim_rep: 512
|
22 |
+
num_heads: 8
|
23 |
+
att_fuse: True
|
24 |
+
|
25 |
+
# Data
|
26 |
+
data_root: data/motion3d/MB3D_f243s81/
|
27 |
+
subset_list: [H36M-SH]
|
28 |
+
dt_file: h36m_sh_conf_cam_source_final.pkl
|
29 |
+
clip_len: 243
|
30 |
+
data_stride: 81
|
31 |
+
rootrel: True
|
32 |
+
sample_stride: 1
|
33 |
+
num_joints: 17
|
34 |
+
no_conf: False
|
35 |
+
gt_2d: False
|
36 |
+
|
37 |
+
# Loss
|
38 |
+
lambda_3d_velocity: 20.0
|
39 |
+
lambda_scale: 0.5
|
40 |
+
lambda_lv: 0.0
|
41 |
+
lambda_lg: 0.0
|
42 |
+
lambda_a: 0.0
|
43 |
+
lambda_av: 0.0
|
44 |
+
|
45 |
+
# Augmentation
|
46 |
+
synthetic: False
|
47 |
+
flip: True
|
48 |
+
mask_ratio: 0.
|
49 |
+
mask_T_ratio: 0.
|
50 |
+
noise: False
|
configs/pose3d/MB_ft_h36m_global.yaml
ADDED
@@ -0,0 +1,50 @@
|
|
|
|
|
|
|
|
|
|
|
|
|
|
|
|
|
|
|
|
|
|
|
|
|
|
|
|
|
|
|
|
|
|
|
|
|
|
|
|
|
|
|
|
|
|
|
|
|
|
|
|
|
|
|
|
|
|
|
|
|
|
|
|
|
|
|
|
|
|
|
|
|
|
|
|
|
|
|
|
|
|
|
|
|
|
|
|
|
|
|
|
|
|
|
|
|
|
|
|
|
|
|
1 |
+
# General
|
2 |
+
train_2d: False
|
3 |
+
no_eval: False
|
4 |
+
finetune: True
|
5 |
+
partial_train: null
|
6 |
+
|
7 |
+
# Traning
|
8 |
+
epochs: 60
|
9 |
+
checkpoint_frequency: 30
|
10 |
+
batch_size: 32
|
11 |
+
dropout: 0.0
|
12 |
+
learning_rate: 0.0002
|
13 |
+
weight_decay: 0.01
|
14 |
+
lr_decay: 0.99
|
15 |
+
|
16 |
+
# Model
|
17 |
+
maxlen: 243
|
18 |
+
dim_feat: 512
|
19 |
+
mlp_ratio: 2
|
20 |
+
depth: 5
|
21 |
+
dim_rep: 512
|
22 |
+
num_heads: 8
|
23 |
+
att_fuse: True
|
24 |
+
|
25 |
+
# Data
|
26 |
+
data_root: data/motion3d/MB3D_f243s81/
|
27 |
+
subset_list: [H36M-SH]
|
28 |
+
dt_file: h36m_sh_conf_cam_source_final.pkl
|
29 |
+
clip_len: 243
|
30 |
+
data_stride: 81
|
31 |
+
rootrel: False
|
32 |
+
sample_stride: 1
|
33 |
+
num_joints: 17
|
34 |
+
no_conf: False
|
35 |
+
gt_2d: False
|
36 |
+
|
37 |
+
# Loss
|
38 |
+
lambda_3d_velocity: 20.0
|
39 |
+
lambda_scale: 0.5
|
40 |
+
lambda_lv: 0.0
|
41 |
+
lambda_lg: 0.0
|
42 |
+
lambda_a: 0.0
|
43 |
+
lambda_av: 0.0
|
44 |
+
|
45 |
+
# Augmentation
|
46 |
+
synthetic: False
|
47 |
+
flip: True
|
48 |
+
mask_ratio: 0.
|
49 |
+
mask_T_ratio: 0.
|
50 |
+
noise: False
|
configs/pose3d/MB_ft_h36m_global_lite.yaml
ADDED
@@ -0,0 +1,50 @@
|
|
|
|
|
|
|
|
|
|
|
|
|
|
|
|
|
|
|
|
|
|
|
|
|
|
|
|
|
|
|
|
|
|
|
|
|
|
|
|
|
|
|
|
|
|
|
|
|
|
|
|
|
|
|
|
|
|
|
|
|
|
|
|
|
|
|
|
|
|
|
|
|
|
|
|
|
|
|
|
|
|
|
|
|
|
|
|
|
|
|
|
|
|
|
|
|
|
|
|
|
|
|
1 |
+
# General
|
2 |
+
train_2d: False
|
3 |
+
no_eval: False
|
4 |
+
finetune: True
|
5 |
+
partial_train: null
|
6 |
+
|
7 |
+
# Traning
|
8 |
+
epochs: 60
|
9 |
+
checkpoint_frequency: 30
|
10 |
+
batch_size: 32
|
11 |
+
dropout: 0.0
|
12 |
+
learning_rate: 0.0005
|
13 |
+
weight_decay: 0.01
|
14 |
+
lr_decay: 0.99
|
15 |
+
|
16 |
+
# Model
|
17 |
+
maxlen: 243
|
18 |
+
dim_feat: 256
|
19 |
+
mlp_ratio: 4
|
20 |
+
depth: 5
|
21 |
+
dim_rep: 512
|
22 |
+
num_heads: 8
|
23 |
+
att_fuse: True
|
24 |
+
|
25 |
+
# Data
|
26 |
+
data_root: data/motion3d/MB3D_f243s81/
|
27 |
+
subset_list: [H36M-SH]
|
28 |
+
dt_file: h36m_sh_conf_cam_source_final.pkl
|
29 |
+
clip_len: 243
|
30 |
+
data_stride: 81
|
31 |
+
rootrel: False
|
32 |
+
sample_stride: 1
|
33 |
+
num_joints: 17
|
34 |
+
no_conf: False
|
35 |
+
gt_2d: False
|
36 |
+
|
37 |
+
# Loss
|
38 |
+
lambda_3d_velocity: 20.0
|
39 |
+
lambda_scale: 0.5
|
40 |
+
lambda_lv: 0.0
|
41 |
+
lambda_lg: 0.0
|
42 |
+
lambda_a: 0.0
|
43 |
+
lambda_av: 0.0
|
44 |
+
|
45 |
+
# Augmentation
|
46 |
+
synthetic: False
|
47 |
+
flip: True
|
48 |
+
mask_ratio: 0.
|
49 |
+
mask_T_ratio: 0.
|
50 |
+
noise: False
|
configs/pose3d/MB_train_h36m.yaml
ADDED
@@ -0,0 +1,51 @@
|
|
|
|
|
|
|
|
|
|
|
|
|
|
|
|
|
|
|
|
|
|
|
|
|
|
|
|
|
|
|
|
|
|
|
|
|
|
|
|
|
|
|
|
|
|
|
|
|
|
|
|
|
|
|
|
|
|
|
|
|
|
|
|
|
|
|
|
|
|
|
|
|
|
|
|
|
|
|
|
|
|
|
|
|
|
|
|
|
|
|
|
|
|
|
|
|
|
|
|
|
|
|
|
|
1 |
+
# General
|
2 |
+
train_2d: False
|
3 |
+
no_eval: False
|
4 |
+
finetune: False
|
5 |
+
partial_train: null
|
6 |
+
|
7 |
+
# Traning
|
8 |
+
epochs: 120
|
9 |
+
checkpoint_frequency: 30
|
10 |
+
batch_size: 32
|
11 |
+
dropout: 0.0
|
12 |
+
learning_rate: 0.0002
|
13 |
+
weight_decay: 0.01
|
14 |
+
lr_decay: 0.99
|
15 |
+
|
16 |
+
# Model
|
17 |
+
maxlen: 243
|
18 |
+
dim_feat: 512
|
19 |
+
mlp_ratio: 2
|
20 |
+
depth: 5
|
21 |
+
dim_rep: 512
|
22 |
+
num_heads: 8
|
23 |
+
att_fuse: True
|
24 |
+
|
25 |
+
# Data
|
26 |
+
data_root: data/motion3d/MB3D_f243s81/
|
27 |
+
subset_list: [H36M-SH]
|
28 |
+
dt_file: h36m_sh_conf_cam_source_final.pkl
|
29 |
+
clip_len: 243
|
30 |
+
data_stride: 81
|
31 |
+
rootrel: True
|
32 |
+
sample_stride: 1
|
33 |
+
num_joints: 17
|
34 |
+
no_conf: False
|
35 |
+
gt_2d: False
|
36 |
+
|
37 |
+
# Loss
|
38 |
+
lambda_3d_velocity: 20.0
|
39 |
+
lambda_scale: 0.5
|
40 |
+
lambda_lv: 0.0
|
41 |
+
lambda_lg: 0.0
|
42 |
+
lambda_a: 0.0
|
43 |
+
lambda_av: 0.0
|
44 |
+
|
45 |
+
# Augmentation
|
46 |
+
synthetic: False
|
47 |
+
flip: True
|
48 |
+
mask_ratio: 0.
|
49 |
+
mask_T_ratio: 0.
|
50 |
+
noise: False
|
51 |
+
|
configs/pretrain/MB_lite.yaml
ADDED
@@ -0,0 +1,53 @@
|
|
|
|
|
|
|
|
|
|
|
|
|
|
|
|
|
|
|
|
|
|
|
|
|
|
|
|
|
|
|
|
|
|
|
|
|
|
|
|
|
|
|
|
|
|
|
|
|
|
|
|
|
|
|
|
|
|
|
|
|
|
|
|
|
|
|
|
|
|
|
|
|
|
|
|
|
|
|
|
|
|
|
|
|
|
|
|
|
|
|
|
|
|
|
|
|
|
|
|
|
|
|
|
|
|
|
|
|
1 |
+
# General
|
2 |
+
train_2d: True
|
3 |
+
no_eval: False
|
4 |
+
finetune: False
|
5 |
+
partial_train: null
|
6 |
+
|
7 |
+
# Traning
|
8 |
+
epochs: 90
|
9 |
+
checkpoint_frequency: 30
|
10 |
+
batch_size: 64
|
11 |
+
dropout: 0.0
|
12 |
+
learning_rate: 0.0005
|
13 |
+
weight_decay: 0.01
|
14 |
+
lr_decay: 0.99
|
15 |
+
pretrain_3d_curriculum: 30
|
16 |
+
|
17 |
+
# Model
|
18 |
+
maxlen: 243
|
19 |
+
dim_feat: 256
|
20 |
+
mlp_ratio: 4
|
21 |
+
depth: 5
|
22 |
+
dim_rep: 512
|
23 |
+
num_heads: 8
|
24 |
+
att_fuse: True
|
25 |
+
|
26 |
+
# Data
|
27 |
+
data_root: data/motion3d/MB3D_f243s81/
|
28 |
+
subset_list: [AMASS, H36M-SH]
|
29 |
+
dt_file: h36m_sh_conf_cam_source_final.pkl
|
30 |
+
clip_len: 243
|
31 |
+
data_stride: 81
|
32 |
+
rootrel: True
|
33 |
+
sample_stride: 1
|
34 |
+
num_joints: 17
|
35 |
+
no_conf: False
|
36 |
+
gt_2d: False
|
37 |
+
|
38 |
+
# Loss
|
39 |
+
lambda_3d_velocity: 20.0
|
40 |
+
lambda_scale: 0.5
|
41 |
+
lambda_lv: 0.0
|
42 |
+
lambda_lg: 0.0
|
43 |
+
lambda_a: 0.0
|
44 |
+
lambda_av: 0.0
|
45 |
+
|
46 |
+
# Augmentation
|
47 |
+
synthetic: True # synthetic: don't use 2D detection results, fake it (from 3D)
|
48 |
+
flip: True
|
49 |
+
mask_ratio: 0.05
|
50 |
+
mask_T_ratio: 0.1
|
51 |
+
noise: True
|
52 |
+
noise_path: params/synthetic_noise.pth
|
53 |
+
d2c_params_path: params/d2c_params.pkl
|
configs/pretrain/MB_pretrain.yaml
ADDED
@@ -0,0 +1,53 @@
|
|
|
|
|
|
|
|
|
|
|
|
|
|
|
|
|
|
|
|
|
|
|
|
|
|
|
|
|
|
|
|
|
|
|
|
|
|
|
|
|
|
|
|
|
|
|
|
|
|
|
|
|
|
|
|
|
|
|
|
|
|
|
|
|
|
|
|
|
|
|
|
|
|
|
|
|
|
|
|
|
|
|
|
|
|
|
|
|
|
|
|
|
|
|
|
|
|
|
|
|
|
|
|
|
|
|
|
|
1 |
+
# General
|
2 |
+
train_2d: True
|
3 |
+
no_eval: False
|
4 |
+
finetune: False
|
5 |
+
partial_train: null
|
6 |
+
|
7 |
+
# Traning
|
8 |
+
epochs: 90
|
9 |
+
checkpoint_frequency: 30
|
10 |
+
batch_size: 64
|
11 |
+
dropout: 0.0
|
12 |
+
learning_rate: 0.0005
|
13 |
+
weight_decay: 0.01
|
14 |
+
lr_decay: 0.99
|
15 |
+
pretrain_3d_curriculum: 30
|
16 |
+
|
17 |
+
# Model
|
18 |
+
maxlen: 243
|
19 |
+
dim_feat: 512
|
20 |
+
mlp_ratio: 2
|
21 |
+
depth: 5
|
22 |
+
dim_rep: 512
|
23 |
+
num_heads: 8
|
24 |
+
att_fuse: True
|
25 |
+
|
26 |
+
# Data
|
27 |
+
data_root: data/motion3d/MB3D_f243s81/
|
28 |
+
subset_list: [AMASS, H36M-SH]
|
29 |
+
dt_file: h36m_sh_conf_cam_source_final.pkl
|
30 |
+
clip_len: 243
|
31 |
+
data_stride: 81
|
32 |
+
rootrel: True
|
33 |
+
sample_stride: 1
|
34 |
+
num_joints: 17
|
35 |
+
no_conf: False
|
36 |
+
gt_2d: False
|
37 |
+
|
38 |
+
# Loss
|
39 |
+
lambda_3d_velocity: 20.0
|
40 |
+
lambda_scale: 0.5
|
41 |
+
lambda_lv: 0.0
|
42 |
+
lambda_lg: 0.0
|
43 |
+
lambda_a: 0.0
|
44 |
+
lambda_av: 0.0
|
45 |
+
|
46 |
+
# Augmentation
|
47 |
+
synthetic: True # synthetic: don't use 2D detection results, fake it (from 3D)
|
48 |
+
flip: True
|
49 |
+
mask_ratio: 0.05
|
50 |
+
mask_T_ratio: 0.1
|
51 |
+
noise: True
|
52 |
+
noise_path: params/synthetic_noise.pth
|
53 |
+
d2c_params_path: params/d2c_params.pkl
|
docs/action.md
ADDED
@@ -0,0 +1,86 @@
|
|
|
|
|
|
|
|
|
|
|
|
|
|
|
|
|
|
|
|
|
|
|
|
|
|
|
|
|
|
|
|
|
|
|
|
|
|
|
|
|
|
|
|
|
|
|
|
|
|
|
|
|
|
|
|
|
|
|
|
|
|
|
|
|
|
|
|
|
|
|
|
|
|
|
|
|
|
|
|
|
|
|
|
|
|
|
|
|
|
|
|
|
|
|
|
|
|
|
|
|
|
|
|
|
|
|
|
|
|
|
|
|
|
|
|
|
|
|
|
|
|
|
|
|
|
|
|
|
|
|
|
|
|
|
|
|
|
|
|
|
|
|
|
|
|
|
|
|
|
|
|
|
|
|
|
|
|
|
|
|
|
|
|
|
|
|
|
|
|
|
|
|
|
|
1 |
+
# Skeleton-based Action Recognition
|
2 |
+
|
3 |
+
## Data
|
4 |
+
|
5 |
+
The NTURGB+D 2D detection results are provided by [pyskl](https://github.com/kennymckormick/pyskl/blob/main/tools/data/README.md) using HRNet.
|
6 |
+
|
7 |
+
1. Download [`ntu60_hrnet.pkl`](https://download.openmmlab.com/mmaction/pyskl/data/nturgbd/ntu60_hrnet.pkl) and [`ntu120_hrnet.pkl`](https://download.openmmlab.com/mmaction/pyskl/data/nturgbd/ntu120_hrnet.pkl) to `data/action/`.
|
8 |
+
2. Download the 1-shot split [here](https://1drv.ms/f/s!AvAdh0LSjEOlfi-hqlHxdVMZxWM) and put it to `data/action/`.
|
9 |
+
|
10 |
+
## Running
|
11 |
+
|
12 |
+
### NTURGB+D
|
13 |
+
|
14 |
+
**Train from scratch:**
|
15 |
+
|
16 |
+
```shell
|
17 |
+
# Cross-subject
|
18 |
+
python train_action.py \
|
19 |
+
--config configs/action/MB_train_NTU60_xsub.yaml \
|
20 |
+
--checkpoint checkpoint/action/MB_train_NTU60_xsub
|
21 |
+
|
22 |
+
# Cross-view
|
23 |
+
python train_action.py \
|
24 |
+
--config configs/action/MB_train_NTU60_xview.yaml \
|
25 |
+
--checkpoint checkpoint/action/MB_train_NTU60_xview
|
26 |
+
```
|
27 |
+
|
28 |
+
**Finetune from pretrained MotionBERT:**
|
29 |
+
|
30 |
+
```shell
|
31 |
+
# Cross-subject
|
32 |
+
python train_action.py \
|
33 |
+
--config configs/action/MB_ft_NTU60_xsub.yaml \
|
34 |
+
--pretrained checkpoint/pretrain/MB_release \
|
35 |
+
--checkpoint checkpoint/action/FT_MB_release_MB_ft_NTU60_xsub
|
36 |
+
|
37 |
+
# Cross-view
|
38 |
+
python train_action.py \
|
39 |
+
--config configs/action/MB_ft_NTU60_xview.yaml \
|
40 |
+
--pretrained checkpoint/pretrain/MB_release \
|
41 |
+
--checkpoint checkpoint/action/FT_MB_release_MB_ft_NTU60_xview
|
42 |
+
```
|
43 |
+
|
44 |
+
**Evaluate:**
|
45 |
+
|
46 |
+
```bash
|
47 |
+
# Cross-subject
|
48 |
+
python train_action.py \
|
49 |
+
--config configs/action/MB_train_NTU60_xsub.yaml \
|
50 |
+
--evaluate checkpoint/action/MB_train_NTU60_xsub/best_epoch.bin
|
51 |
+
|
52 |
+
# Cross-view
|
53 |
+
python train_action.py \
|
54 |
+
--config configs/action/MB_train_NTU60_xview.yaml \
|
55 |
+
--evaluate checkpoint/action/MB_train_NTU60_xview/best_epoch.bin
|
56 |
+
```
|
57 |
+
|
58 |
+
### NTURGB+D-120 (1-shot)
|
59 |
+
|
60 |
+
**Train from scratch:**
|
61 |
+
|
62 |
+
```bash
|
63 |
+
python train_action_1shot.py \
|
64 |
+
--config configs/action/MB_train_NTU120_oneshot.yaml \
|
65 |
+
--checkpoint checkpoint/action/MB_train_NTU120_oneshot
|
66 |
+
```
|
67 |
+
|
68 |
+
**Finetune from a pretrained model:**
|
69 |
+
|
70 |
+
```bash
|
71 |
+
python train_action_1shot.py \
|
72 |
+
--config configs/action/MB_ft_NTU120_oneshot.yaml \
|
73 |
+
--pretrained checkpoint/pretrain/MB_release \
|
74 |
+
--checkpoint checkpoint/action/FT_MB_release_MB_ft_NTU120_oneshot
|
75 |
+
```
|
76 |
+
|
77 |
+
**Evaluate:**
|
78 |
+
|
79 |
+
```bash
|
80 |
+
python train_action_1shot.py \
|
81 |
+
--config configs/action/MB_train_NTU120_oneshot.yaml \
|
82 |
+
--evaluate checkpoint/action/MB_train_NTU120_oneshot/best_epoch.bin
|
83 |
+
```
|
84 |
+
|
85 |
+
|
86 |
+
|
docs/inference.md
ADDED
@@ -0,0 +1,48 @@
|
|
|
|
|
|
|
|
|
|
|
|
|
|
|
|
|
|
|
|
|
|
|
|
|
|
|
|
|
|
|
|
|
|
|
|
|
|
|
|
|
|
|
|
|
|
|
|
|
|
|
|
|
|
|
|
|
|
|
|
|
|
|
|
|
|
|
|
|
|
|
|
|
|
|
|
|
|
|
|
|
|
|
|
|
|
|
|
|
|
|
|
|
|
|
|
|
|
|
1 |
+
# In-the-wild Inference
|
2 |
+
|
3 |
+
## 2D Pose
|
4 |
+
|
5 |
+
Please use [AlphaPose](https://github.com/MVIG-SJTU/AlphaPose#quick-start) to extract the 2D keypoints for your video first. We use the *Fast Pose* model trained on *Halpe* dataset ([Link](https://github.com/MVIG-SJTU/AlphaPose/blob/master/docs/MODEL_ZOO.md#halpe-dataset-26-keypoints)).
|
6 |
+
|
7 |
+
Note: Currently we only support single person. If your video contains multiple person, you may need to use the [Pose Tracking Module for AlphaPose](https://github.com/MVIG-SJTU/AlphaPose/tree/master/trackers) and set `--focus` to specify the target person id.
|
8 |
+
|
9 |
+
|
10 |
+
|
11 |
+
## 3D Pose
|
12 |
+
|
13 |
+
|  | 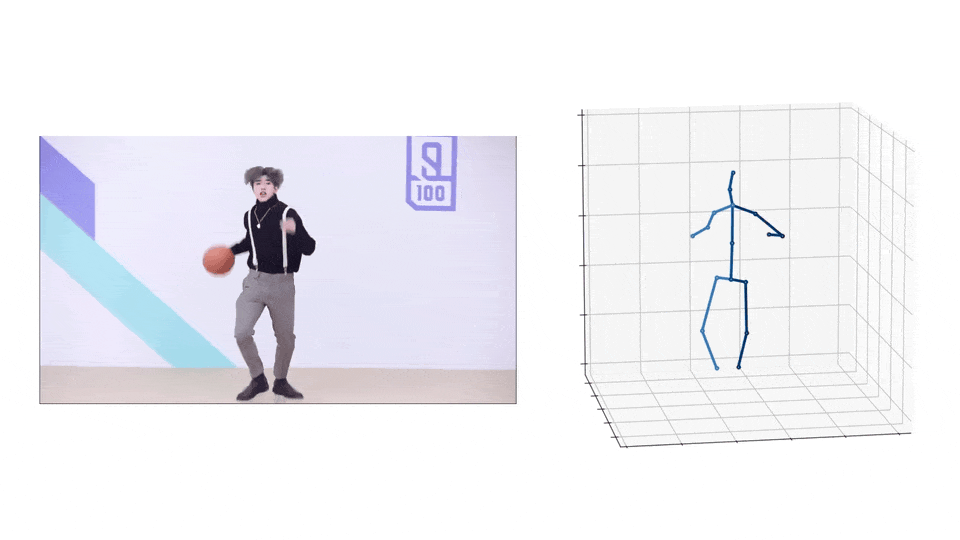 |
|
14 |
+
| ------------------------------------------------------------ | ------------------------------------------------------------ |
|
15 |
+
|
16 |
+
|
17 |
+
1. Please download the checkpoint [here](https://1drv.ms/f/s!AvAdh0LSjEOlgT67igq_cIoYvO2y?e=bfEc73) and put it to `checkpoint/pose3d/FT_MB_lite_MB_ft_h36m_global_lite/`.
|
18 |
+
1. Run the following command to infer from the extracted 2D poses:
|
19 |
+
```bash
|
20 |
+
python infer_wild.py \
|
21 |
+
--vid_path <your_video.mp4> \
|
22 |
+
--json_path <alphapose-results.json> \
|
23 |
+
--out_path <output_path>
|
24 |
+
```
|
25 |
+
|
26 |
+
|
27 |
+
|
28 |
+
## Mesh
|
29 |
+
|
30 |
+
| 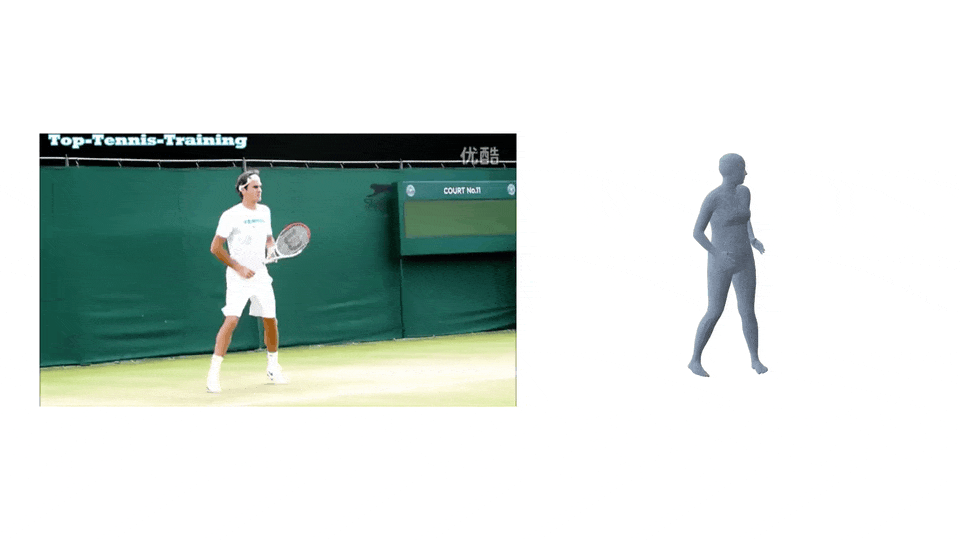 |  |
|
31 |
+
| ------------------------------------------------------------ | ----------- |
|
32 |
+
|
33 |
+
1. Please download the checkpoint [here](https://1drv.ms/f/s!AvAdh0LSjEOlgTmgYNslCDWMNQi9?e=WjcB1F) and put it to `checkpoint/mesh/FT_MB_release_MB_ft_pw3d/`
|
34 |
+
2. Run the following command to infer from the extracted 2D poses:
|
35 |
+
```bash
|
36 |
+
python infer_wild_mesh.py \
|
37 |
+
--vid_path <your_video.mp4> \
|
38 |
+
--json_path <alphapose-results.json> \
|
39 |
+
--out_path <output_path> \
|
40 |
+
--ref_3d_motion_path <3d-pose-results.npy> # Optional, use the estimated 3D motion for root trajectory.
|
41 |
+
```
|
42 |
+
|
43 |
+
|
44 |
+
|
45 |
+
|
46 |
+
|
47 |
+
|
48 |
+
|
docs/mesh.md
ADDED
@@ -0,0 +1,61 @@
|
|
|
|
|
|
|
|
|
|
|
|
|
|
|
|
|
|
|
|
|
|
|
|
|
|
|
|
|
|
|
|
|
|
|
|
|
|
|
|
|
|
|
|
|
|
|
|
|
|
|
|
|
|
|
|
|
|
|
|
|
|
|
|
|
|
|
|
|
|
|
|
|
|
|
|
|
|
|
|
|
|
|
|
|
|
|
|
|
|
|
|
|
|
|
|
|
|
|
|
|
|
|
|
|
|
|
|
|
|
|
|
|
|
|
|
|
|
|
|
|
|
|
|
|
1 |
+
# Human Mesh Recovery
|
2 |
+
|
3 |
+
## Data
|
4 |
+
|
5 |
+
1. Download the datasets [here](https://1drv.ms/f/s!AvAdh0LSjEOlfy-hqlHxdVMZxWM) and put them to `data/mesh/`. We use Human3.6M, COCO, and PW3D for training and testing. Descriptions of the joint regressors could be found in [SPIN](https://github.com/nkolot/SPIN/tree/master/data).
|
6 |
+
2. Download the SMPL model(`basicModel_neutral_lbs_10_207_0_v1.0.0.pkl`) from [SMPLify](https://smplify.is.tue.mpg.de/), put it to `data/mesh/`, and rename it as `SMPL_NEUTRAL.pkl`
|
7 |
+
|
8 |
+
|
9 |
+
## Running
|
10 |
+
|
11 |
+
**Train from scratch:**
|
12 |
+
|
13 |
+
```bash
|
14 |
+
# with 3DPW
|
15 |
+
python train_mesh.py \
|
16 |
+
--config configs/mesh/MB_train_pw3d.yaml \
|
17 |
+
--checkpoint checkpoint/mesh/MB_train_pw3d
|
18 |
+
|
19 |
+
# H36M
|
20 |
+
python train_mesh.py \
|
21 |
+
--config configs/mesh/MB_train_h36m.yaml \
|
22 |
+
--checkpoint checkpoint/mesh/MB_train_h36m
|
23 |
+
```
|
24 |
+
|
25 |
+
**Finetune from a pretrained model:**
|
26 |
+
|
27 |
+
```bash
|
28 |
+
# with 3DPW
|
29 |
+
python train_mesh.py \
|
30 |
+
--config configs/mesh/MB_ft_pw3d.yaml \
|
31 |
+
--pretrained checkpoint/pretrain/MB_release \
|
32 |
+
--checkpoint checkpoint/mesh/FT_MB_release_MB_ft_pw3d
|
33 |
+
|
34 |
+
# H36M
|
35 |
+
python train_mesh.py \
|
36 |
+
--config configs/mesh/MB_ft_h36m.yaml \
|
37 |
+
--pretrained checkpoint/pretrain/MB_release \
|
38 |
+
--checkpoint checkpoint/mesh/FT_MB_release_MB_ft_h36m
|
39 |
+
|
40 |
+
```
|
41 |
+
|
42 |
+
**Evaluate:**
|
43 |
+
|
44 |
+
```bash
|
45 |
+
# with 3DPW
|
46 |
+
python train_mesh.py \
|
47 |
+
--config configs/mesh/MB_train_pw3d.yaml \
|
48 |
+
--evaluate checkpoint/mesh/MB_train_pw3d/best_epoch.bin
|
49 |
+
|
50 |
+
# H36M
|
51 |
+
python train_mesh.py \
|
52 |
+
--config configs/mesh/MB_train_h36m.yaml \
|
53 |
+
--evaluate checkpoint/mesh/MB_train_h36m/best_epoch.bin
|
54 |
+
```
|
55 |
+
|
56 |
+
|
57 |
+
|
58 |
+
|
59 |
+
|
60 |
+
|
61 |
+
|
docs/pose3d.md
ADDED
@@ -0,0 +1,51 @@
|
|
|
|
|
|
|
|
|
|
|
|
|
|
|
|
|
|
|
|
|
|
|
|
|
|
|
|
|
|
|
|
|
|
|
|
|
|
|
|
|
|
|
|
|
|
|
|
|
|
|
|
|
|
|
|
|
|
|
|
|
|
|
|
|
|
|
|
|
|
|
|
|
|
|
|
|
|
|
|
|
|
|
|
|
|
|
|
|
|
|
|
|
|
|
|
|
|
|
|
|
|
|
|
|
1 |
+
# 3D Human Pose Estimation
|
2 |
+
|
3 |
+
## Data
|
4 |
+
|
5 |
+
1. Download the finetuned Stacked Hourglass detections and our preprocessed H3.6M data [here](https://1drv.ms/u/s!AvAdh0LSjEOlgU7BuUZcyafu8kzc?e=vobkjZ) and unzip it to `data/motion3d`.
|
6 |
+
|
7 |
+
> Note that the preprocessed data is only intended for reproducing our results more easily. If you want to use the dataset, please register to the [Human3.6m website](http://vision.imar.ro/human3.6m/) and download the dataset in its original format. Please refer to [LCN](https://github.com/CHUNYUWANG/lcn-pose#data) for how we prepare the H3.6M data.
|
8 |
+
|
9 |
+
2. Slice the motion clips (len=243, stride=81)
|
10 |
+
|
11 |
+
```bash
|
12 |
+
python tools/convert_h36m.py
|
13 |
+
```
|
14 |
+
|
15 |
+
## Running
|
16 |
+
|
17 |
+
**Train from scratch:**
|
18 |
+
|
19 |
+
```bash
|
20 |
+
python train.py \
|
21 |
+
--config configs/pose3d/MB_train_h36m.yaml \
|
22 |
+
--checkpoint checkpoint/pose3d/MB_train_h36m
|
23 |
+
```
|
24 |
+
|
25 |
+
**Finetune from pretrained MotionBERT:**
|
26 |
+
|
27 |
+
```bash
|
28 |
+
python train.py \
|
29 |
+
--config configs/pose3d/MB_ft_h36m.yaml \
|
30 |
+
--pretrained checkpoint/pretrain/MB_release \
|
31 |
+
--checkpoint checkpoint/pose3d/FT_MB_release_MB_ft_h36m
|
32 |
+
```
|
33 |
+
|
34 |
+
**Evaluate:**
|
35 |
+
|
36 |
+
```bash
|
37 |
+
python train.py \
|
38 |
+
--config configs/pose3d/MB_train_h36m.yaml \
|
39 |
+
--evaluate checkpoint/pose3d/MB_train_h36m/best_epoch.bin
|
40 |
+
```
|
41 |
+
|
42 |
+
|
43 |
+
|
44 |
+
|
45 |
+
|
46 |
+
|
47 |
+
|
48 |
+
|
49 |
+
|
50 |
+
|
51 |
+
|
docs/pretrain.md
ADDED
@@ -0,0 +1,81 @@
|
|
|
|
|
|
|
|
|
|
|
|
|
|
|
|
|
|
|
|
|
|
|
|
|
|
|
|
|
|
|
|
|
|
|
|
|
|
|
|
|
|
|
|
|
|
|
|
|
|
|
|
|
|
|
|
|
|
|
|
|
|
|
|
|
|
|
|
|
|
|
|
|
|
|
|
|
|
|
|
|
|
|
|
|
|
|
|
|
|
|
|
|
|
|
|
|
|
|
|
|
|
|
|
|
|
|
|
|
|
|
|
|
|
|
|
|
|
|
|
|
|
|
|
|
|
|
|
|
|
|
|
|
|
|
|
|
|
|
|
|
|
|
|
|
|
|
|
|
|
|
|
|
|
|
|
|
|
|
|
|
|
|
|
|
1 |
+
# Pretrain
|
2 |
+
|
3 |
+
## Data
|
4 |
+
|
5 |
+
### AMASS
|
6 |
+
|
7 |
+
1. Please download data from the [official website](https://amass.is.tue.mpg.de/download.php) (SMPL+H).
|
8 |
+
2. We provide the preprocessing scripts as follows. Minor modifications might be necessary.
|
9 |
+
- [tools/compress_amass.py](../tools/compress_amass.py): downsample the frame rate
|
10 |
+
- [tools/preprocess_amass.py](../tools/preprocess_amass.py): render the mocap data and extract the 3D keypoints
|
11 |
+
- [tools/convert_amass.py](../tools/convert_amass.py): slice them to motion clips
|
12 |
+
|
13 |
+
|
14 |
+
|
15 |
+
### Human 3.6M
|
16 |
+
|
17 |
+
Please refer to [pose3d.md](pose3d.md#data).
|
18 |
+
|
19 |
+
|
20 |
+
|
21 |
+
### PoseTrack
|
22 |
+
|
23 |
+
Please download PoseTrack18 from [MMPose](https://mmpose.readthedocs.io/en/latest/dataset_zoo/2d_body_keypoint.html#posetrack18) (annotation files) and unzip to `data/motion2d`.
|
24 |
+
|
25 |
+
|
26 |
+
|
27 |
+
### InstaVariety
|
28 |
+
|
29 |
+
1. Please download data from [human_dynamics](https://github.com/akanazawa/human_dynamics/blob/master/doc/insta_variety.md#generating-tfrecords) to `data/motion2d`.
|
30 |
+
1. Use [tools/convert_insta.py](../tools/convert_insta.py) to preprocess the 2D keypoints (need to specify `name_action` ).
|
31 |
+
1. Load all the processed `.pkl` files from step 2, concatenate them to `motion_list`, then run
|
32 |
+
|
33 |
+
```python
|
34 |
+
import numpy as np
|
35 |
+
ids = []
|
36 |
+
for i, x in enumerate(motion_list):
|
37 |
+
ids.append(np.ones(len(x))*i)
|
38 |
+
motion_all = np.concatenate(motion_list)
|
39 |
+
id_all = np.concatenate(ids)
|
40 |
+
np.save('data/motion2d/InstaVariety/motion_all.npy', motion_all)
|
41 |
+
np.save('data/motion2d/InstaVariety/id_all.npy', id_all)
|
42 |
+
|
43 |
+
```
|
44 |
+
|
45 |
+
You can also download the preprocessed 2D keypoints from [here](https://1drv.ms/u/s!AvAdh0LSjEOlgVElzkVkWoFcJ1MR?e=TU2CeI) and unzip it to `data/motion2d/`.
|
46 |
+
|
47 |
+
|
48 |
+
|
49 |
+
|
50 |
+
|
51 |
+
The processed directory tree should look like this:
|
52 |
+
|
53 |
+
```
|
54 |
+
.
|
55 |
+
└── data/
|
56 |
+
├── motion3d/
|
57 |
+
│ └── MB3D_f243s81/
|
58 |
+
│ ├── AMASS
|
59 |
+
│ └── H36M-SH
|
60 |
+
├── motion2d/
|
61 |
+
│ ├── InstaVariety/
|
62 |
+
│ │ ├── motion_all.npy
|
63 |
+
│ │ └── id_all.npy
|
64 |
+
│ └── posetrack18_annotations/
|
65 |
+
│ ├── train
|
66 |
+
│ └── ...
|
67 |
+
└── ...
|
68 |
+
```
|
69 |
+
|
70 |
+
|
71 |
+
|
72 |
+
## Train
|
73 |
+
|
74 |
+
```bash
|
75 |
+
python train.py \
|
76 |
+
--config configs/pretrain/MB_pretrain.yaml \
|
77 |
+
-c checkpoint/pretrain/MB_pretrain
|
78 |
+
```
|
79 |
+
|
80 |
+
|
81 |
+
|
infer_wild.py
ADDED
@@ -0,0 +1,97 @@
|
|
|
|
|
|
|
|
|
|
|
|
|
|
|
|
|
|
|
|
|
|
|
|
|
|
|
|
|
|
|
|
|
|
|
|
|
|
|
|
|
|
|
|
|
|
|
|
|
|
|
|
|
|
|
|
|
|
|
|
|
|
|
|
|
|
|
|
|
|
|
|
|
|
|
|
|
|
|
|
|
|
|
|
|
|
|
|
|
|
|
|
|
|
|
|
|
|
|
|
|
|
|
|
|
|
|
|
|
|
|
|
|
|
|
|
|
|
|
|
|
|
|
|
|
|
|
|
|
|
|
|
|
|
|
|
|
|
|
|
|
|
|
|
|
|
|
|
|
|
|
|
|
|
|
|
|
|
|
|
|
|
|
|
|
|
|
|
|
|
|
|
|
|
|
|
|
|
|
|
|
|
|
|
|
|
|
|
|
|
|
|
|
|
|
|
|
1 |
+
import os
|
2 |
+
import numpy as np
|
3 |
+
import argparse
|
4 |
+
from tqdm import tqdm
|
5 |
+
import imageio
|
6 |
+
import torch
|
7 |
+
import torch.nn as nn
|
8 |
+
from torch.utils.data import DataLoader
|
9 |
+
from lib.utils.tools import *
|
10 |
+
from lib.utils.learning import *
|
11 |
+
from lib.utils.utils_data import flip_data
|
12 |
+
from lib.data.dataset_wild import WildDetDataset
|
13 |
+
from lib.utils.vismo import render_and_save
|
14 |
+
|
15 |
+
def parse_args():
|
16 |
+
parser = argparse.ArgumentParser()
|
17 |
+
parser.add_argument("--config", type=str, default="configs/pose3d/MB_ft_h36m_global_lite.yaml", help="Path to the config file.")
|
18 |
+
parser.add_argument('-e', '--evaluate', default='checkpoint/pose3d/FT_MB_lite_MB_ft_h36m_global_lite/best_epoch.bin', type=str, metavar='FILENAME', help='checkpoint to evaluate (file name)')
|
19 |
+
parser.add_argument('-j', '--json_path', type=str, help='alphapose detection result json path')
|
20 |
+
parser.add_argument('-v', '--vid_path', type=str, help='video path')
|
21 |
+
parser.add_argument('-o', '--out_path', type=str, help='output path')
|
22 |
+
parser.add_argument('--pixel', action='store_true', help='align with pixle coordinates')
|
23 |
+
parser.add_argument('--focus', type=int, default=None, help='target person id')
|
24 |
+
parser.add_argument('--clip_len', type=int, default=243, help='clip length for network input')
|
25 |
+
opts = parser.parse_args()
|
26 |
+
return opts
|
27 |
+
|
28 |
+
opts = parse_args()
|
29 |
+
args = get_config(opts.config)
|
30 |
+
|
31 |
+
model_backbone = load_backbone(args)
|
32 |
+
if torch.cuda.is_available():
|
33 |
+
model_backbone = nn.DataParallel(model_backbone)
|
34 |
+
model_backbone = model_backbone.cuda()
|
35 |
+
|
36 |
+
print('Loading checkpoint', opts.evaluate)
|
37 |
+
checkpoint = torch.load(opts.evaluate, map_location=lambda storage, loc: storage)
|
38 |
+
model_backbone.load_state_dict(checkpoint['model_pos'], strict=True)
|
39 |
+
model_pos = model_backbone
|
40 |
+
model_pos.eval()
|
41 |
+
testloader_params = {
|
42 |
+
'batch_size': 1,
|
43 |
+
'shuffle': False,
|
44 |
+
'num_workers': 8,
|
45 |
+
'pin_memory': True,
|
46 |
+
'prefetch_factor': 4,
|
47 |
+
'persistent_workers': True,
|
48 |
+
'drop_last': False
|
49 |
+
}
|
50 |
+
|
51 |
+
vid = imageio.get_reader(opts.vid_path, 'ffmpeg')
|
52 |
+
fps_in = vid.get_meta_data()['fps']
|
53 |
+
vid_size = vid.get_meta_data()['size']
|
54 |
+
os.makedirs(opts.out_path, exist_ok=True)
|
55 |
+
|
56 |
+
if opts.pixel:
|
57 |
+
# Keep relative scale with pixel coornidates
|
58 |
+
wild_dataset = WildDetDataset(opts.json_path, clip_len=opts.clip_len, vid_size=vid_size, scale_range=None, focus=opts.focus)
|
59 |
+
else:
|
60 |
+
# Scale to [-1,1]
|
61 |
+
wild_dataset = WildDetDataset(opts.json_path, clip_len=opts.clip_len, scale_range=[1,1], focus=opts.focus)
|
62 |
+
|
63 |
+
test_loader = DataLoader(wild_dataset, **testloader_params)
|
64 |
+
|
65 |
+
results_all = []
|
66 |
+
with torch.no_grad():
|
67 |
+
for batch_input in tqdm(test_loader):
|
68 |
+
N, T = batch_input.shape[:2]
|
69 |
+
if torch.cuda.is_available():
|
70 |
+
batch_input = batch_input.cuda()
|
71 |
+
if args.no_conf:
|
72 |
+
batch_input = batch_input[:, :, :, :2]
|
73 |
+
if args.flip:
|
74 |
+
batch_input_flip = flip_data(batch_input)
|
75 |
+
predicted_3d_pos_1 = model_pos(batch_input)
|
76 |
+
predicted_3d_pos_flip = model_pos(batch_input_flip)
|
77 |
+
predicted_3d_pos_2 = flip_data(predicted_3d_pos_flip) # Flip back
|
78 |
+
predicted_3d_pos = (predicted_3d_pos_1 + predicted_3d_pos_2) / 2.0
|
79 |
+
else:
|
80 |
+
predicted_3d_pos = model_pos(batch_input)
|
81 |
+
if args.rootrel:
|
82 |
+
predicted_3d_pos[:,:,0,:]=0 # [N,T,17,3]
|
83 |
+
else:
|
84 |
+
predicted_3d_pos[:,0,0,2]=0
|
85 |
+
pass
|
86 |
+
if args.gt_2d:
|
87 |
+
predicted_3d_pos[...,:2] = batch_input[...,:2]
|
88 |
+
results_all.append(predicted_3d_pos.cpu().numpy())
|
89 |
+
|
90 |
+
results_all = np.hstack(results_all)
|
91 |
+
results_all = np.concatenate(results_all)
|
92 |
+
render_and_save(results_all, '%s/X3D.mp4' % (opts.out_path), keep_imgs=False, fps=fps_in)
|
93 |
+
if opts.pixel:
|
94 |
+
# Convert to pixel coordinates
|
95 |
+
results_all = results_all * (min(vid_size) / 2.0)
|
96 |
+
results_all[:,:,:2] = results_all[:,:,:2] + np.array(vid_size) / 2.0
|
97 |
+
np.save('%s/X3D.npy' % (opts.out_path), results_all)
|
infer_wild_mesh.py
ADDED
@@ -0,0 +1,157 @@
|
|
|
|
|
|
|
|
|
|
|
|
|
|
|
|
|
|
|
|
|
|
|
|
|
|
|
|
|
|
|
|
|
|
|
|
|
|
|
|
|
|
|
|
|
|
|
|
|
|
|
|
|
|
|
|
|
|
|
|
|
|
|
|
|
|
|
|
|
|
|
|
|
|
|
|
|
|
|
|
|
|
|
|
|
|
|
|
|
|
|
|
|
|
|
|
|
|
|
|
|
|
|
|
|
|
|
|
|
|
|
|
|
|
|
|
|
|
|
|
|
|
|
|
|
|
|
|
|
|
|
|
|
|
|
|
|
|
|
|
|
|
|
|
|
|
|
|
|
|
|
|
|
|
|
|
|
|
|
|
|
|
|
|
|
|
|
|
|
|
|
|
|
|
|
|
|
|
|
|
|
|
|
|
|
|
|
|
|
|
|
|
|
|
|
|
|
|
|
|
|
|
|
|
|
|
|
|
|
|
|
|
|
|
|
|
|
|
|
|
|
|
|
|
|
|
|
|
|
|
|
|
|
|
|
|
|
|
|
|
|
|
|
|
|
|
|
|
|
|
|
|
|
|
|
|
|
|
|
|
|
|
|
|
|
|
|
|
|
|
|
|
|
|
|
|
|
|
|
|
|
|
|
|
|
|
|
|
|
|
|
|
|
|
|
|
|
|
|
|
|
|
|
|
|
|
|
|
|
|
|
|
|
|
|
|
|
1 |
+
import os
|
2 |
+
import os.path as osp
|
3 |
+
import numpy as np
|
4 |
+
import argparse
|
5 |
+
import pickle
|
6 |
+
from tqdm import tqdm
|
7 |
+
import time
|
8 |
+
import random
|
9 |
+
import imageio
|
10 |
+
|
11 |
+
import torch
|
12 |
+
import torch.nn as nn
|
13 |
+
import torch.nn.functional as F
|
14 |
+
import torch.optim as optim
|
15 |
+
from torch.utils.data import DataLoader
|
16 |
+
|
17 |
+
from lib.utils.tools import *
|
18 |
+
from lib.utils.learning import *
|
19 |
+
from lib.utils.utils_data import flip_data
|
20 |
+
from lib.utils.utils_mesh import flip_thetas_batch
|
21 |
+
from lib.data.dataset_wild import WildDetDataset
|
22 |
+
# from lib.model.loss import *
|
23 |
+
from lib.model.model_mesh import MeshRegressor
|
24 |
+
from lib.utils.vismo import render_and_save, motion2video_mesh
|
25 |
+
from lib.utils.utils_smpl import *
|
26 |
+
from scipy.optimize import least_squares
|
27 |
+
|
28 |
+
def parse_args():
|
29 |
+
parser = argparse.ArgumentParser()
|
30 |
+
parser.add_argument("--config", type=str, default="configs/mesh/MB_ft_pw3d.yaml", help="Path to the config file.")
|
31 |
+
parser.add_argument('-e', '--evaluate', default='checkpoint/mesh/FT_MB_release_MB_ft_pw3d/best_epoch.bin', type=str, metavar='FILENAME', help='checkpoint to evaluate (file name)')
|
32 |
+
parser.add_argument('-j', '--json_path', type=str, help='alphapose detection result json path')
|
33 |
+
parser.add_argument('-v', '--vid_path', type=str, help='video path')
|
34 |
+
parser.add_argument('-o', '--out_path', type=str, help='output path')
|
35 |
+
parser.add_argument('--ref_3d_motion_path', type=str, default=None, help='3D motion path')
|
36 |
+
parser.add_argument('--pixel', action='store_true', help='align with pixle coordinates')
|
37 |
+
parser.add_argument('--focus', type=int, default=None, help='target person id')
|
38 |
+
parser.add_argument('--clip_len', type=int, default=243, help='clip length for network input')
|
39 |
+
opts = parser.parse_args()
|
40 |
+
return opts
|
41 |
+
|
42 |
+
def err(p, x, y):
|
43 |
+
return np.linalg.norm(p[0] * x + np.array([p[1], p[2], p[3]]) - y, axis=-1).mean()
|
44 |
+
|
45 |
+
def solve_scale(x, y):
|
46 |
+
print('Estimating camera transformation.')
|
47 |
+
best_res = 100000
|
48 |
+
best_scale = None
|
49 |
+
for init_scale in tqdm(range(0,2000,5)):
|
50 |
+
p0 = [init_scale, 0.0, 0.0, 0.0]
|
51 |
+
est = least_squares(err, p0, args = (x.reshape(-1,3), y.reshape(-1,3)))
|
52 |
+
if est['fun'] < best_res:
|
53 |
+
best_res = est['fun']
|
54 |
+
best_scale = est['x'][0]
|
55 |
+
print('Pose matching error = %.2f mm.' % best_res)
|
56 |
+
return best_scale
|
57 |
+
|
58 |
+
opts = parse_args()
|
59 |
+
args = get_config(opts.config)
|
60 |
+
|
61 |
+
# root_rel
|
62 |
+
# args.rootrel = True
|
63 |
+
|
64 |
+
smpl = SMPL(args.data_root, batch_size=1).cuda()
|
65 |
+
J_regressor = smpl.J_regressor_h36m
|
66 |
+
|
67 |
+
end = time.time()
|
68 |
+
model_backbone = load_backbone(args)
|
69 |
+
print(f'init backbone time: {(time.time()-end):02f}s')
|
70 |
+
end = time.time()
|
71 |
+
model = MeshRegressor(args, backbone=model_backbone, dim_rep=args.dim_rep, hidden_dim=args.hidden_dim, dropout_ratio=args.dropout)
|
72 |
+
print(f'init whole model time: {(time.time()-end):02f}s')
|
73 |
+
|
74 |
+
if torch.cuda.is_available():
|
75 |
+
model = nn.DataParallel(model)
|
76 |
+
model = model.cuda()
|
77 |
+
|
78 |
+
chk_filename = opts.evaluate if opts.evaluate else opts.resume
|
79 |
+
print('Loading checkpoint', chk_filename)
|
80 |
+
checkpoint = torch.load(chk_filename, map_location=lambda storage, loc: storage)
|
81 |
+
model.load_state_dict(checkpoint['model'], strict=True)
|
82 |
+
model.eval()
|
83 |
+
|
84 |
+
testloader_params = {
|
85 |
+
'batch_size': 1,
|
86 |
+
'shuffle': False,
|
87 |
+
'num_workers': 8,
|
88 |
+
'pin_memory': True,
|
89 |
+
'prefetch_factor': 4,
|
90 |
+
'persistent_workers': True,
|
91 |
+
'drop_last': False
|
92 |
+
}
|
93 |
+
|
94 |
+
vid = imageio.get_reader(opts.vid_path, 'ffmpeg')
|
95 |
+
fps_in = vid.get_meta_data()['fps']
|
96 |
+
vid_size = vid.get_meta_data()['size']
|
97 |
+
os.makedirs(opts.out_path, exist_ok=True)
|
98 |
+
|
99 |
+
if opts.pixel:
|
100 |
+
# Keep relative scale with pixel coornidates
|
101 |
+
wild_dataset = WildDetDataset(opts.json_path, clip_len=opts.clip_len, vid_size=vid_size, scale_range=None, focus=opts.focus)
|
102 |
+
else:
|
103 |
+
# Scale to [-1,1]
|
104 |
+
wild_dataset = WildDetDataset(opts.json_path, clip_len=opts.clip_len, scale_range=[1,1], focus=opts.focus)
|
105 |
+
|
106 |
+
test_loader = DataLoader(wild_dataset, **testloader_params)
|
107 |
+
|
108 |
+
verts_all = []
|
109 |
+
reg3d_all = []
|
110 |
+
with torch.no_grad():
|
111 |
+
for batch_input in tqdm(test_loader):
|
112 |
+
batch_size, clip_frames = batch_input.shape[:2]
|
113 |
+
if torch.cuda.is_available():
|
114 |
+
batch_input = batch_input.cuda().float()
|
115 |
+
output = model(batch_input)
|
116 |
+
batch_input_flip = flip_data(batch_input)
|
117 |
+
output_flip = model(batch_input_flip)
|
118 |
+
output_flip_pose = output_flip[0]['theta'][:, :, :72]
|
119 |
+
output_flip_shape = output_flip[0]['theta'][:, :, 72:]
|
120 |
+
output_flip_pose = flip_thetas_batch(output_flip_pose)
|
121 |
+
output_flip_pose = output_flip_pose.reshape(-1, 72)
|
122 |
+
output_flip_shape = output_flip_shape.reshape(-1, 10)
|
123 |
+
output_flip_smpl = smpl(
|
124 |
+
betas=output_flip_shape,
|
125 |
+
body_pose=output_flip_pose[:, 3:],
|
126 |
+
global_orient=output_flip_pose[:, :3],
|
127 |
+
pose2rot=True
|
128 |
+
)
|
129 |
+
output_flip_verts = output_flip_smpl.vertices.detach()
|
130 |
+
J_regressor_batch = J_regressor[None, :].expand(output_flip_verts.shape[0], -1, -1).to(output_flip_verts.device)
|
131 |
+
output_flip_kp3d = torch.matmul(J_regressor_batch, output_flip_verts) # (NT,17,3)
|
132 |
+
output_flip_back = [{
|
133 |
+
'verts': output_flip_verts.reshape(batch_size, clip_frames, -1, 3) * 1000.0,
|
134 |
+
'kp_3d': output_flip_kp3d.reshape(batch_size, clip_frames, -1, 3),
|
135 |
+
}]
|
136 |
+
output_final = [{}]
|
137 |
+
for k, v in output_flip_back[0].items():
|
138 |
+
output_final[0][k] = (output[0][k] + output_flip_back[0][k]) / 2.0
|
139 |
+
output = output_final
|
140 |
+
verts_all.append(output[0]['verts'].cpu().numpy())
|
141 |
+
reg3d_all.append(output[0]['kp_3d'].cpu().numpy())
|
142 |
+
|
143 |
+
verts_all = np.hstack(verts_all)
|
144 |
+
verts_all = np.concatenate(verts_all)
|
145 |
+
reg3d_all = np.hstack(reg3d_all)
|
146 |
+
reg3d_all = np.concatenate(reg3d_all)
|
147 |
+
|
148 |
+
if opts.ref_3d_motion_path:
|
149 |
+
ref_pose = np.load(opts.ref_3d_motion_path)
|
150 |
+
x = ref_pose - ref_pose[:, :1]
|
151 |
+
y = reg3d_all - reg3d_all[:, :1]
|
152 |
+
scale = solve_scale(x, y)
|
153 |
+
root_cam = ref_pose[:, :1] * scale
|
154 |
+
verts_all = verts_all - reg3d_all[:,:1] + root_cam
|
155 |
+
|
156 |
+
render_and_save(verts_all, osp.join(opts.out_path, 'mesh.mp4'), keep_imgs=False, fps=fps_in, draw_face=True)
|
157 |
+
|
lib/data/augmentation.py
ADDED
@@ -0,0 +1,99 @@
|
|
|
|
|
|
|
|
|
|
|
|
|
|
|
|
|
|
|
|
|
|
|
|
|
|
|
|
|
|
|
|
|
|
|
|
|
|
|
|
|
|
|
|
|
|
|
|
|
|
|
|
|
|
|
|
|
|
|
|
|
|
|
|
|
|
|
|
|
|
|
|
|
|
|
|
|
|
|
|
|
|
|
|
|
|
|
|
|
|
|
|
|
|
|
|
|
|
|
|
|
|
|
|
|
|
|
|
|
|
|
|
|
|
|
|
|
|
|
|
|
|
|
|
|
|
|
|
|
|
|
|
|
|
|
|
|
|
|
|
|
|
|
|
|
|
|
|
|
|
|
|
|
|
|
|
|
|
|
|
|
|
|
|
|
|
|
|
|
|
|
|
|
|
|
|
|
|
|
|
|
|
|
|
|
|
|
|
|
|
|
|
|
|
|
|
|
|
|
|
|
1 |
+
import numpy as np
|
2 |
+
import os
|
3 |
+
import random
|
4 |
+
import torch
|
5 |
+
import copy
|
6 |
+
import torch.nn as nn
|
7 |
+
from lib.utils.tools import read_pkl
|
8 |
+
from lib.utils.utils_data import flip_data, crop_scale_3d
|
9 |
+
|
10 |
+
class Augmenter2D(object):
|
11 |
+
"""
|
12 |
+
Make 2D augmentations on the fly. PyTorch batch-processing GPU version.
|
13 |
+
"""
|
14 |
+
def __init__(self, args):
|
15 |
+
self.d2c_params = read_pkl(args.d2c_params_path)
|
16 |
+
self.noise = torch.load(args.noise_path)
|
17 |
+
self.mask_ratio = args.mask_ratio
|
18 |
+
self.mask_T_ratio = args.mask_T_ratio
|
19 |
+
self.num_Kframes = 27
|
20 |
+
self.noise_std = 0.002
|
21 |
+
|
22 |
+
def dis2conf(self, dis, a, b, m, s):
|
23 |
+
f = a/(dis+a)+b*dis
|
24 |
+
shift = torch.randn(*dis.shape)*s + m
|
25 |
+
# if torch.cuda.is_available():
|
26 |
+
shift = shift.to(dis.device)
|
27 |
+
return f + shift
|
28 |
+
|
29 |
+
def add_noise(self, motion_2d):
|
30 |
+
a, b, m, s = self.d2c_params["a"], self.d2c_params["b"], self.d2c_params["m"], self.d2c_params["s"]
|
31 |
+
if "uniform_range" in self.noise.keys():
|
32 |
+
uniform_range = self.noise["uniform_range"]
|
33 |
+
else:
|
34 |
+
uniform_range = 0.06
|
35 |
+
motion_2d = motion_2d[:,:,:,:2]
|
36 |
+
batch_size = motion_2d.shape[0]
|
37 |
+
num_frames = motion_2d.shape[1]
|
38 |
+
num_joints = motion_2d.shape[2]
|
39 |
+
mean = self.noise['mean'].float()
|
40 |
+
std = self.noise['std'].float()
|
41 |
+
weight = self.noise['weight'][:,None].float()
|
42 |
+
sel = torch.rand((batch_size, self.num_Kframes, num_joints, 1))
|
43 |
+
gaussian_sample = (torch.randn(batch_size, self.num_Kframes, num_joints, 2) * std + mean)
|
44 |
+
uniform_sample = (torch.rand((batch_size, self.num_Kframes, num_joints, 2))-0.5) * uniform_range
|
45 |
+
noise_mean = 0
|
46 |
+
delta_noise = torch.randn(num_frames, num_joints, 2) * self.noise_std + noise_mean
|
47 |
+
# if torch.cuda.is_available():
|
48 |
+
mean = mean.to(motion_2d.device)
|
49 |
+
std = std.to(motion_2d.device)
|
50 |
+
weight = weight.to(motion_2d.device)
|
51 |
+
gaussian_sample = gaussian_sample.to(motion_2d.device)
|
52 |
+
uniform_sample = uniform_sample.to(motion_2d.device)
|
53 |
+
sel = sel.to(motion_2d.device)
|
54 |
+
delta_noise = delta_noise.to(motion_2d.device)
|
55 |
+
|
56 |
+
delta = gaussian_sample*(sel<weight) + uniform_sample*(sel>=weight)
|
57 |
+
delta_expand = torch.nn.functional.interpolate(delta.unsqueeze(1), [num_frames, num_joints, 2], mode='trilinear', align_corners=True)[:,0]
|
58 |
+
delta_final = delta_expand + delta_noise
|
59 |
+
motion_2d = motion_2d + delta_final
|
60 |
+
dx = delta_final[:,:,:,0]
|
61 |
+
dy = delta_final[:,:,:,1]
|
62 |
+
dis2 = dx*dx+dy*dy
|
63 |
+
dis = torch.sqrt(dis2)
|
64 |
+
conf = self.dis2conf(dis, a, b, m, s).clip(0,1).reshape([batch_size, num_frames, num_joints, -1])
|
65 |
+
return torch.cat((motion_2d, conf), dim=3)
|
66 |
+
|
67 |
+
def add_mask(self, x):
|
68 |
+
''' motion_2d: (N,T,17,3)
|
69 |
+
'''
|
70 |
+
N,T,J,C = x.shape
|
71 |
+
mask = torch.rand(N,T,J,1, dtype=x.dtype, device=x.device) > self.mask_ratio
|
72 |
+
mask_T = torch.rand(1,T,1,1, dtype=x.dtype, device=x.device) > self.mask_T_ratio
|
73 |
+
x = x * mask * mask_T
|
74 |
+
return x
|
75 |
+
|
76 |
+
def augment2D(self, motion_2d, mask=False, noise=False):
|
77 |
+
if noise:
|
78 |
+
motion_2d = self.add_noise(motion_2d)
|
79 |
+
if mask:
|
80 |
+
motion_2d = self.add_mask(motion_2d)
|
81 |
+
return motion_2d
|
82 |
+
|
83 |
+
class Augmenter3D(object):
|
84 |
+
"""
|
85 |
+
Make 3D augmentations when dataloaders get items. NumPy single motion version.
|
86 |
+
"""
|
87 |
+
def __init__(self, args):
|
88 |
+
self.flip = args.flip
|
89 |
+
if hasattr(args, "scale_range_pretrain"):
|
90 |
+
self.scale_range_pretrain = args.scale_range_pretrain
|
91 |
+
else:
|
92 |
+
self.scale_range_pretrain = None
|
93 |
+
|
94 |
+
def augment3D(self, motion_3d):
|
95 |
+
if self.scale_range_pretrain:
|
96 |
+
motion_3d = crop_scale_3d(motion_3d, self.scale_range_pretrain)
|
97 |
+
if self.flip and random.random()>0.5:
|
98 |
+
motion_3d = flip_data(motion_3d)
|
99 |
+
return motion_3d
|
lib/data/datareader_h36m.py
ADDED
@@ -0,0 +1,136 @@
|
|
|
|
|
|
|
|
|
|
|
|
|
|
|
|
|
|
|
|
|
|
|
|
|
|
|
|
|
|
|
|
|
|
|
|
|
|
|
|
|
|
|
|
|
|
|
|
|
|
|
|
|
|
|
|
|
|
|
|
|
|
|
|
|
|
|
|
|
|
|
|
|
|
|
|
|
|
|
|
|
|
|
|
|
|
|
|
|
|
|
|
|
|
|
|
|
|
|
|
|
|
|
|
|
|
|
|
|
|
|
|
|
|
|
|
|
|
|
|
|
|
|
|
|
|
|
|
|
|
|
|
|
|
|
|
|
|
|
|
|
|
|
|
|
|
|
|
|
|
|
|
|
|
|
|
|
|
|
|
|
|
|
|
|
|
|
|
|
|
|
|
|
|
|
|
|
|
|
|
|
|
|
|
|
|
|
|
|
|
|
|
|
|
|
|
|
|
|
|
|
|
|
|
|
|
|
|
|
|
|
|
|
|
|
|
|
|
|
|
|
|
|
|
|
|
|
|
|
|
|
|
|
|
|
|
|
|
|
|
|
|
|
|
|
|
|
|
|
|
|
|
|
|
|
|
|
|
|
|
|
|
|
|
|
|
|
|
|
|
|
|
|
|
|
1 |
+
# Adapted from Optimizing Network Structure for 3D Human Pose Estimation (ICCV 2019) (https://github.com/CHUNYUWANG/lcn-pose/blob/master/tools/data.py)
|
2 |
+
|
3 |
+
import numpy as np
|
4 |
+
import os, sys
|
5 |
+
import random
|
6 |
+
import copy
|
7 |
+
from lib.utils.tools import read_pkl
|
8 |
+
from lib.utils.utils_data import split_clips
|
9 |
+
random.seed(0)
|
10 |
+
|
11 |
+
class DataReaderH36M(object):
|
12 |
+
def __init__(self, n_frames, sample_stride, data_stride_train, data_stride_test, read_confidence=True, dt_root = 'data/motion3d', dt_file = 'h36m_cpn_cam_source.pkl'):
|
13 |
+
self.gt_trainset = None
|
14 |
+
self.gt_testset = None
|
15 |
+
self.split_id_train = None
|
16 |
+
self.split_id_test = None
|
17 |
+
self.test_hw = None
|
18 |
+
self.dt_dataset = read_pkl('%s/%s' % (dt_root, dt_file))
|
19 |
+
self.n_frames = n_frames
|
20 |
+
self.sample_stride = sample_stride
|
21 |
+
self.data_stride_train = data_stride_train
|
22 |
+
self.data_stride_test = data_stride_test
|
23 |
+
self.read_confidence = read_confidence
|
24 |
+
|
25 |
+
def read_2d(self):
|
26 |
+
trainset = self.dt_dataset['train']['joint_2d'][::self.sample_stride, :, :2].astype(np.float32) # [N, 17, 2]
|
27 |
+
testset = self.dt_dataset['test']['joint_2d'][::self.sample_stride, :, :2].astype(np.float32) # [N, 17, 2]
|
28 |
+
# map to [-1, 1]
|
29 |
+
for idx, camera_name in enumerate(self.dt_dataset['train']['camera_name']):
|
30 |
+
if camera_name == '54138969' or camera_name == '60457274':
|
31 |
+
res_w, res_h = 1000, 1002
|
32 |
+
elif camera_name == '55011271' or camera_name == '58860488':
|
33 |
+
res_w, res_h = 1000, 1000
|
34 |
+
else:
|
35 |
+
assert 0, '%d data item has an invalid camera name' % idx
|
36 |
+
trainset[idx, :, :] = trainset[idx, :, :] / res_w * 2 - [1, res_h / res_w]
|
37 |
+
for idx, camera_name in enumerate(self.dt_dataset['test']['camera_name']):
|
38 |
+
if camera_name == '54138969' or camera_name == '60457274':
|
39 |
+
res_w, res_h = 1000, 1002
|
40 |
+
elif camera_name == '55011271' or camera_name == '58860488':
|
41 |
+
res_w, res_h = 1000, 1000
|
42 |
+
else:
|
43 |
+
assert 0, '%d data item has an invalid camera name' % idx
|
44 |
+
testset[idx, :, :] = testset[idx, :, :] / res_w * 2 - [1, res_h / res_w]
|
45 |
+
if self.read_confidence:
|
46 |
+
if 'confidence' in self.dt_dataset['train'].keys():
|
47 |
+
train_confidence = self.dt_dataset['train']['confidence'][::self.sample_stride].astype(np.float32)
|
48 |
+
test_confidence = self.dt_dataset['test']['confidence'][::self.sample_stride].astype(np.float32)
|
49 |
+
if len(train_confidence.shape)==2: # (1559752, 17)
|
50 |
+
train_confidence = train_confidence[:,:,None]
|
51 |
+
test_confidence = test_confidence[:,:,None]
|
52 |
+
else:
|
53 |
+
# No conf provided, fill with 1.
|
54 |
+
train_confidence = np.ones(trainset.shape)[:,:,0:1]
|
55 |
+
test_confidence = np.ones(testset.shape)[:,:,0:1]
|
56 |
+
trainset = np.concatenate((trainset, train_confidence), axis=2) # [N, 17, 3]
|
57 |
+
testset = np.concatenate((testset, test_confidence), axis=2) # [N, 17, 3]
|
58 |
+
return trainset, testset
|
59 |
+
|
60 |
+
def read_3d(self):
|
61 |
+
train_labels = self.dt_dataset['train']['joint3d_image'][::self.sample_stride, :, :3].astype(np.float32) # [N, 17, 3]
|
62 |
+
test_labels = self.dt_dataset['test']['joint3d_image'][::self.sample_stride, :, :3].astype(np.float32) # [N, 17, 3]
|
63 |
+
# map to [-1, 1]
|
64 |
+
for idx, camera_name in enumerate(self.dt_dataset['train']['camera_name']):
|
65 |
+
if camera_name == '54138969' or camera_name == '60457274':
|
66 |
+
res_w, res_h = 1000, 1002
|
67 |
+
elif camera_name == '55011271' or camera_name == '58860488':
|
68 |
+
res_w, res_h = 1000, 1000
|
69 |
+
else:
|
70 |
+
assert 0, '%d data item has an invalid camera name' % idx
|
71 |
+
train_labels[idx, :, :2] = train_labels[idx, :, :2] / res_w * 2 - [1, res_h / res_w]
|
72 |
+
train_labels[idx, :, 2:] = train_labels[idx, :, 2:] / res_w * 2
|
73 |
+
|
74 |
+
for idx, camera_name in enumerate(self.dt_dataset['test']['camera_name']):
|
75 |
+
if camera_name == '54138969' or camera_name == '60457274':
|
76 |
+
res_w, res_h = 1000, 1002
|
77 |
+
elif camera_name == '55011271' or camera_name == '58860488':
|
78 |
+
res_w, res_h = 1000, 1000
|
79 |
+
else:
|
80 |
+
assert 0, '%d data item has an invalid camera name' % idx
|
81 |
+
test_labels[idx, :, :2] = test_labels[idx, :, :2] / res_w * 2 - [1, res_h / res_w]
|
82 |
+
test_labels[idx, :, 2:] = test_labels[idx, :, 2:] / res_w * 2
|
83 |
+
|
84 |
+
return train_labels, test_labels
|
85 |
+
def read_hw(self):
|
86 |
+
if self.test_hw is not None:
|
87 |
+
return self.test_hw
|
88 |
+
test_hw = np.zeros((len(self.dt_dataset['test']['camera_name']), 2))
|
89 |
+
for idx, camera_name in enumerate(self.dt_dataset['test']['camera_name']):
|
90 |
+
if camera_name == '54138969' or camera_name == '60457274':
|
91 |
+
res_w, res_h = 1000, 1002
|
92 |
+
elif camera_name == '55011271' or camera_name == '58860488':
|
93 |
+
res_w, res_h = 1000, 1000
|
94 |
+
else:
|
95 |
+
assert 0, '%d data item has an invalid camera name' % idx
|
96 |
+
test_hw[idx] = res_w, res_h
|
97 |
+
self.test_hw = test_hw
|
98 |
+
return test_hw
|
99 |
+
|
100 |
+
def get_split_id(self):
|
101 |
+
if self.split_id_train is not None and self.split_id_test is not None:
|
102 |
+
return self.split_id_train, self.split_id_test
|
103 |
+
vid_list_train = self.dt_dataset['train']['source'][::self.sample_stride] # (1559752,)
|
104 |
+
vid_list_test = self.dt_dataset['test']['source'][::self.sample_stride] # (566920,)
|
105 |
+
self.split_id_train = split_clips(vid_list_train, self.n_frames, data_stride=self.data_stride_train)
|
106 |
+
self.split_id_test = split_clips(vid_list_test, self.n_frames, data_stride=self.data_stride_test)
|
107 |
+
return self.split_id_train, self.split_id_test
|
108 |
+
|
109 |
+
def get_hw(self):
|
110 |
+
# Only Testset HW is needed for denormalization
|
111 |
+
test_hw = self.read_hw() # train_data (1559752, 2) test_data (566920, 2)
|
112 |
+
split_id_train, split_id_test = self.get_split_id()
|
113 |
+
test_hw = test_hw[split_id_test][:,0,:] # (N, 2)
|
114 |
+
return test_hw
|
115 |
+
|
116 |
+
def get_sliced_data(self):
|
117 |
+
train_data, test_data = self.read_2d() # train_data (1559752, 17, 3) test_data (566920, 17, 3)
|
118 |
+
train_labels, test_labels = self.read_3d() # train_labels (1559752, 17, 3) test_labels (566920, 17, 3)
|
119 |
+
split_id_train, split_id_test = self.get_split_id()
|
120 |
+
train_data, test_data = train_data[split_id_train], test_data[split_id_test] # (N, 27, 17, 3)
|
121 |
+
train_labels, test_labels = train_labels[split_id_train], test_labels[split_id_test] # (N, 27, 17, 3)
|
122 |
+
# ipdb.set_trace()
|
123 |
+
return train_data, test_data, train_labels, test_labels
|
124 |
+
|
125 |
+
def denormalize(self, test_data):
|
126 |
+
# data: (N, n_frames, 51) or data: (N, n_frames, 17, 3)
|
127 |
+
n_clips = test_data.shape[0]
|
128 |
+
test_hw = self.get_hw()
|
129 |
+
data = test_data.reshape([n_clips, -1, 17, 3])
|
130 |
+
assert len(data) == len(test_hw)
|
131 |
+
# denormalize (x,y,z) coordiantes for results
|
132 |
+
for idx, item in enumerate(data):
|
133 |
+
res_w, res_h = test_hw[idx]
|
134 |
+
data[idx, :, :, :2] = (data[idx, :, :, :2] + np.array([1, res_h / res_w])) * res_w / 2
|
135 |
+
data[idx, :, :, 2:] = data[idx, :, :, 2:] * res_w / 2
|
136 |
+
return data # [n_clips, -1, 17, 3]
|
lib/data/datareader_mesh.py
ADDED
@@ -0,0 +1,59 @@
|
|
|
|
|
|
|
|
|
|
|
|
|
|
|
|
|
|
|
|
|
|
|
|
|
|
|
|
|
|
|
|
|
|
|
|
|
|
|
|
|
|
|
|
|
|
|
|
|
|
|
|
|
|
|
|
|
|
|
|
|
|
|
|
|
|
|
|
|
|
|
|
|
|
|
|
|
|
|
|
|
|
|
|
|
|
|
|
|
|
|
|
|
|
|
|
|
|
|
|
|
|
|
|
|
|
|
|
|
|
|
|
|
|
|
|
|
|
|
|
|
1 |
+
import numpy as np
|
2 |
+
import os, sys
|
3 |
+
import copy
|
4 |
+
from lib.utils.tools import read_pkl
|
5 |
+
from lib.utils.utils_data import split_clips
|
6 |
+
|
7 |
+
class DataReaderMesh(object):
|
8 |
+
def __init__(self, n_frames, sample_stride, data_stride_train, data_stride_test, read_confidence=True, dt_root = 'data/mesh', dt_file = 'pw3d_det.pkl', res=[1920, 1920]):
|
9 |
+
self.split_id_train = None
|
10 |
+
self.split_id_test = None
|
11 |
+
self.dt_dataset = read_pkl('%s/%s' % (dt_root, dt_file))
|
12 |
+
self.n_frames = n_frames
|
13 |
+
self.sample_stride = sample_stride
|
14 |
+
self.data_stride_train = data_stride_train
|
15 |
+
self.data_stride_test = data_stride_test
|
16 |
+
self.read_confidence = read_confidence
|
17 |
+
self.res = res
|
18 |
+
|
19 |
+
def read_2d(self):
|
20 |
+
if self.res is not None:
|
21 |
+
res_w, res_h = self.res
|
22 |
+
offset = [1, res_h / res_w]
|
23 |
+
else:
|
24 |
+
res = np.array(self.dt_dataset['train']['img_hw'])[::self.sample_stride].astype(np.float32)
|
25 |
+
res_w, res_h = res.max(1)[:, None, None], res.max(1)[:, None, None]
|
26 |
+
offset = 1
|
27 |
+
trainset = self.dt_dataset['train']['joint_2d'][::self.sample_stride, :, :2].astype(np.float32) # [N, 17, 2]
|
28 |
+
testset = self.dt_dataset['test']['joint_2d'][::self.sample_stride, :, :2].astype(np.float32) # [N, 17, 2]
|
29 |
+
# res_w, res_h = self.res
|
30 |
+
trainset = trainset / res_w * 2 - offset
|
31 |
+
testset = testset / res_w * 2 - offset
|
32 |
+
if self.read_confidence:
|
33 |
+
train_confidence = self.dt_dataset['train']['confidence'][::self.sample_stride].astype(np.float32)
|
34 |
+
test_confidence = self.dt_dataset['test']['confidence'][::self.sample_stride].astype(np.float32)
|
35 |
+
if len(train_confidence.shape)==2:
|
36 |
+
train_confidence = train_confidence[:,:,None]
|
37 |
+
test_confidence = test_confidence[:,:,None]
|
38 |
+
trainset = np.concatenate((trainset, train_confidence), axis=2) # [N, 17, 3]
|
39 |
+
testset = np.concatenate((testset, test_confidence), axis=2) # [N, 17, 3]
|
40 |
+
return trainset, testset
|
41 |
+
|
42 |
+
def get_split_id(self):
|
43 |
+
if self.split_id_train is not None and self.split_id_test is not None:
|
44 |
+
return self.split_id_train, self.split_id_test
|
45 |
+
vid_list_train = self.dt_dataset['train']['source'][::self.sample_stride]
|
46 |
+
vid_list_test = self.dt_dataset['test']['source'][::self.sample_stride]
|
47 |
+
self.split_id_train = split_clips(vid_list_train, self.n_frames, self.data_stride_train)
|
48 |
+
self.split_id_test = split_clips(vid_list_test, self.n_frames, self.data_stride_test)
|
49 |
+
return self.split_id_train, self.split_id_test
|
50 |
+
|
51 |
+
def get_sliced_data(self):
|
52 |
+
train_data, test_data = self.read_2d()
|
53 |
+
train_labels, test_labels = self.read_3d()
|
54 |
+
split_id_train, split_id_test = self.get_split_id()
|
55 |
+
train_data, test_data = train_data[split_id_train], test_data[split_id_test] # (N, 27, 17, 3)
|
56 |
+
train_labels, test_labels = train_labels[split_id_train], test_labels[split_id_test] # (N, 27, 17, 3)
|
57 |
+
return train_data, test_data, train_labels, test_labels
|
58 |
+
|
59 |
+
|
lib/data/dataset_action.py
ADDED
@@ -0,0 +1,206 @@
|
|
|
|
|
|
|
|
|
|
|
|
|
|
|
|
|
|
|
|
|
|
|
|
|
|
|
|
|
|
|
|
|
|
|
|
|
|
|
|
|
|
|
|
|
|
|
|
|
|
|
|
|
|
|
|
|
|
|
|
|
|
|
|
|
|
|
|
|
|
|
|
|
|
|
|
|
|
|
|
|
|
|
|
|
|
|
|
|
|
|
|
|
|
|
|
|
|
|
|
|
|
|
|
|
|
|
|
|
|
|
|
|
|
|
|
|
|
|
|
|
|
|
|
|
|
|
|
|
|
|
|
|
|
|
|
|
|
|
|
|
|
|
|
|
|
|
|
|
|
|
|
|
|
|
|
|
|
|
|
|
|
|
|
|
|
|
|
|
|
|
|
|
|
|
|
|
|
|
|
|
|
|
|
|
|
|
|
|
|
|
|
|
|
|
|
|
|
|
|
|
|
|
|
|
|
|
|
|
|
|
|
|
|
|
|
|
|
|
|
|
|
|
|
|
|
|
|
|
|
|
|
|
|
|
|
|
|
|
|
|
|
|
|
|
|
|
|
|
|
|
|
|
|
|
|
|
|
|
|
|
|
|
|
|
|
|
|
|
|
|
|
|
|
|
|
|
|
|
|
|
|
|
|
|
|
|
|
|
|
|
|
|
|
|
|
|
|
|
|
|
|
|
|
|
|
|
|
|
|
|
|
|
|
|
|
|
|
|
|
|
|
|
|
|
|
|
|
|
|
|
|
|
|
|
|
|
|
|
|
|
|
|
|
|
|
|
|
|
|
|
|
|
|
|
|
|
|
|
|
|
|
|
|
|
|
|
|
|
|
|
|
|
|
|
|
|
|
|
|
|
|
|
|
|
|
|
|
|
|
|
|
|
|
|
|
|
|
|
|
|
|
|
|
|
|
|
|
|
|
|
|
|
|
|
1 |
+
import torch
|
2 |
+
import numpy as np
|
3 |
+
import os
|
4 |
+
import random
|
5 |
+
import copy
|
6 |
+
from torch.utils.data import Dataset, DataLoader
|
7 |
+
from lib.utils.utils_data import crop_scale, resample
|
8 |
+
from lib.utils.tools import read_pkl
|
9 |
+
|
10 |
+
def get_action_names(file_path = "data/action/ntu_actions.txt"):
|
11 |
+
f = open(file_path, "r")
|
12 |
+
s = f.read()
|
13 |
+
actions = s.split('\n')
|
14 |
+
action_names = []
|
15 |
+
for a in actions:
|
16 |
+
action_names.append(a.split('.')[1][1:])
|
17 |
+
return action_names
|
18 |
+
|
19 |
+
def make_cam(x, img_shape):
|
20 |
+
'''
|
21 |
+
Input: x (M x T x V x C)
|
22 |
+
img_shape (height, width)
|
23 |
+
'''
|
24 |
+
h, w = img_shape
|
25 |
+
if w >= h:
|
26 |
+
x_cam = x / w * 2 - 1
|
27 |
+
else:
|
28 |
+
x_cam = x / h * 2 - 1
|
29 |
+
return x_cam
|
30 |
+
|
31 |
+
def coco2h36m(x):
|
32 |
+
'''
|
33 |
+
Input: x (M x T x V x C)
|
34 |
+
|
35 |
+
COCO: {0-nose 1-Leye 2-Reye 3-Lear 4Rear 5-Lsho 6-Rsho 7-Lelb 8-Relb 9-Lwri 10-Rwri 11-Lhip 12-Rhip 13-Lkne 14-Rkne 15-Lank 16-Rank}
|
36 |
+
|
37 |
+
H36M:
|
38 |
+
0: 'root',
|
39 |
+
1: 'rhip',
|
40 |
+
2: 'rkne',
|
41 |
+
3: 'rank',
|
42 |
+
4: 'lhip',
|
43 |
+
5: 'lkne',
|
44 |
+
6: 'lank',
|
45 |
+
7: 'belly',
|
46 |
+
8: 'neck',
|
47 |
+
9: 'nose',
|
48 |
+
10: 'head',
|
49 |
+
11: 'lsho',
|
50 |
+
12: 'lelb',
|
51 |
+
13: 'lwri',
|
52 |
+
14: 'rsho',
|
53 |
+
15: 'relb',
|
54 |
+
16: 'rwri'
|
55 |
+
'''
|
56 |
+
y = np.zeros(x.shape)
|
57 |
+
y[:,:,0,:] = (x[:,:,11,:] + x[:,:,12,:]) * 0.5
|
58 |
+
y[:,:,1,:] = x[:,:,12,:]
|
59 |
+
y[:,:,2,:] = x[:,:,14,:]
|
60 |
+
y[:,:,3,:] = x[:,:,16,:]
|
61 |
+
y[:,:,4,:] = x[:,:,11,:]
|
62 |
+
y[:,:,5,:] = x[:,:,13,:]
|
63 |
+
y[:,:,6,:] = x[:,:,15,:]
|
64 |
+
y[:,:,8,:] = (x[:,:,5,:] + x[:,:,6,:]) * 0.5
|
65 |
+
y[:,:,7,:] = (y[:,:,0,:] + y[:,:,8,:]) * 0.5
|
66 |
+
y[:,:,9,:] = x[:,:,0,:]
|
67 |
+
y[:,:,10,:] = (x[:,:,1,:] + x[:,:,2,:]) * 0.5
|
68 |
+
y[:,:,11,:] = x[:,:,5,:]
|
69 |
+
y[:,:,12,:] = x[:,:,7,:]
|
70 |
+
y[:,:,13,:] = x[:,:,9,:]
|
71 |
+
y[:,:,14,:] = x[:,:,6,:]
|
72 |
+
y[:,:,15,:] = x[:,:,8,:]
|
73 |
+
y[:,:,16,:] = x[:,:,10,:]
|
74 |
+
return y
|
75 |
+
|
76 |
+
def random_move(data_numpy,
|
77 |
+
angle_range=[-10., 10.],
|
78 |
+
scale_range=[0.9, 1.1],
|
79 |
+
transform_range=[-0.1, 0.1],
|
80 |
+
move_time_candidate=[1]):
|
81 |
+
data_numpy = np.transpose(data_numpy, (3,1,2,0)) # M,T,V,C-> C,T,V,M
|
82 |
+
C, T, V, M = data_numpy.shape
|
83 |
+
move_time = random.choice(move_time_candidate)
|
84 |
+
node = np.arange(0, T, T * 1.0 / move_time).round().astype(int)
|
85 |
+
node = np.append(node, T)
|
86 |
+
num_node = len(node)
|
87 |
+
A = np.random.uniform(angle_range[0], angle_range[1], num_node)
|
88 |
+
S = np.random.uniform(scale_range[0], scale_range[1], num_node)
|
89 |
+
T_x = np.random.uniform(transform_range[0], transform_range[1], num_node)
|
90 |
+
T_y = np.random.uniform(transform_range[0], transform_range[1], num_node)
|
91 |
+
a = np.zeros(T)
|
92 |
+
s = np.zeros(T)
|
93 |
+
t_x = np.zeros(T)
|
94 |
+
t_y = np.zeros(T)
|
95 |
+
# linspace
|
96 |
+
for i in range(num_node - 1):
|
97 |
+
a[node[i]:node[i + 1]] = np.linspace(
|
98 |
+
A[i], A[i + 1], node[i + 1] - node[i]) * np.pi / 180
|
99 |
+
s[node[i]:node[i + 1]] = np.linspace(S[i], S[i + 1], node[i + 1] - node[i])
|
100 |
+
t_x[node[i]:node[i + 1]] = np.linspace(T_x[i], T_x[i + 1], node[i + 1] - node[i])
|
101 |
+
t_y[node[i]:node[i + 1]] = np.linspace(T_y[i], T_y[i + 1], node[i + 1] - node[i])
|
102 |
+
theta = np.array([[np.cos(a) * s, -np.sin(a) * s],
|
103 |
+
[np.sin(a) * s, np.cos(a) * s]])
|
104 |
+
# perform transformation
|
105 |
+
for i_frame in range(T):
|
106 |
+
xy = data_numpy[0:2, i_frame, :, :]
|
107 |
+
new_xy = np.dot(theta[:, :, i_frame], xy.reshape(2, -1))
|
108 |
+
new_xy[0] += t_x[i_frame]
|
109 |
+
new_xy[1] += t_y[i_frame]
|
110 |
+
data_numpy[0:2, i_frame, :, :] = new_xy.reshape(2, V, M)
|
111 |
+
data_numpy = np.transpose(data_numpy, (3,1,2,0)) # C,T,V,M -> M,T,V,C
|
112 |
+
return data_numpy
|
113 |
+
|
114 |
+
def human_tracking(x):
|
115 |
+
M, T = x.shape[:2]
|
116 |
+
if M==1:
|
117 |
+
return x
|
118 |
+
else:
|
119 |
+
diff0 = np.sum(np.linalg.norm(x[0,1:] - x[0,:-1], axis=-1), axis=-1) # (T-1, V, C) -> (T-1)
|
120 |
+
diff1 = np.sum(np.linalg.norm(x[0,1:] - x[1,:-1], axis=-1), axis=-1)
|
121 |
+
x_new = np.zeros(x.shape)
|
122 |
+
sel = np.cumsum(diff0 > diff1) % 2
|
123 |
+
sel = sel[:,None,None]
|
124 |
+
x_new[0][0] = x[0][0]
|
125 |
+
x_new[1][0] = x[1][0]
|
126 |
+
x_new[0,1:] = x[1,1:] * sel + x[0,1:] * (1-sel)
|
127 |
+
x_new[1,1:] = x[0,1:] * sel + x[1,1:] * (1-sel)
|
128 |
+
return x_new
|
129 |
+
|
130 |
+
class ActionDataset(Dataset):
|
131 |
+
def __init__(self, data_path, data_split, n_frames=243, random_move=True, scale_range=[1,1], check_split=True): # data_split: train/test etc.
|
132 |
+
np.random.seed(0)
|
133 |
+
dataset = read_pkl(data_path)
|
134 |
+
if check_split:
|
135 |
+
assert data_split in dataset['split'].keys()
|
136 |
+
self.split = dataset['split'][data_split]
|
137 |
+
annotations = dataset['annotations']
|
138 |
+
self.random_move = random_move
|
139 |
+
self.is_train = "train" in data_split or (check_split==False)
|
140 |
+
if "oneshot" in data_split:
|
141 |
+
self.is_train = False
|
142 |
+
self.scale_range = scale_range
|
143 |
+
motions = []
|
144 |
+
labels = []
|
145 |
+
for sample in annotations:
|
146 |
+
if check_split and (not sample['frame_dir'] in self.split):
|
147 |
+
continue
|
148 |
+
resample_id = resample(ori_len=sample['total_frames'], target_len=n_frames, randomness=self.is_train)
|
149 |
+
motion_cam = make_cam(x=sample['keypoint'], img_shape=sample['img_shape'])
|
150 |
+
motion_cam = human_tracking(motion_cam)
|
151 |
+
motion_cam = coco2h36m(motion_cam)
|
152 |
+
motion_conf = sample['keypoint_score'][..., None]
|
153 |
+
motion = np.concatenate((motion_cam[:,resample_id], motion_conf[:,resample_id]), axis=-1)
|
154 |
+
if motion.shape[0]==1: # Single person, make a fake zero person
|
155 |
+
fake = np.zeros(motion.shape)
|
156 |
+
motion = np.concatenate((motion, fake), axis=0)
|
157 |
+
motions.append(motion.astype(np.float32))
|
158 |
+
labels.append(sample['label'])
|
159 |
+
self.motions = np.array(motions)
|
160 |
+
self.labels = np.array(labels)
|
161 |
+
|
162 |
+
def __len__(self):
|
163 |
+
'Denotes the total number of samples'
|
164 |
+
return len(self.motions)
|
165 |
+
|
166 |
+
def __getitem__(self, index):
|
167 |
+
raise NotImplementedError
|
168 |
+
|
169 |
+
class NTURGBD(ActionDataset):
|
170 |
+
def __init__(self, data_path, data_split, n_frames=243, random_move=True, scale_range=[1,1]):
|
171 |
+
super(NTURGBD, self).__init__(data_path, data_split, n_frames, random_move, scale_range)
|
172 |
+
|
173 |
+
def __getitem__(self, idx):
|
174 |
+
'Generates one sample of data'
|
175 |
+
motion, label = self.motions[idx], self.labels[idx] # (M,T,J,C)
|
176 |
+
if self.random_move:
|
177 |
+
motion = random_move(motion)
|
178 |
+
if self.scale_range:
|
179 |
+
result = crop_scale(motion, scale_range=self.scale_range)
|
180 |
+
else:
|
181 |
+
result = motion
|
182 |
+
return result.astype(np.float32), label
|
183 |
+
|
184 |
+
class NTURGBD1Shot(ActionDataset):
|
185 |
+
def __init__(self, data_path, data_split, n_frames=243, random_move=True, scale_range=[1,1], check_split=False):
|
186 |
+
super(NTURGBD1Shot, self).__init__(data_path, data_split, n_frames, random_move, scale_range, check_split)
|
187 |
+
oneshot_classes = [0, 6, 12, 18, 24, 30, 36, 42, 48, 54, 60, 66, 72, 78, 84, 90, 96, 102, 108, 114]
|
188 |
+
new_classes = set(range(120)) - set(oneshot_classes)
|
189 |
+
old2new = {}
|
190 |
+
for i, cid in enumerate(new_classes):
|
191 |
+
old2new[cid] = i
|
192 |
+
filtered = [not (x in oneshot_classes) for x in self.labels]
|
193 |
+
self.motions = self.motions[filtered]
|
194 |
+
filtered_labels = self.labels[filtered]
|
195 |
+
self.labels = [old2new[x] for x in filtered_labels]
|
196 |
+
|
197 |
+
def __getitem__(self, idx):
|
198 |
+
'Generates one sample of data'
|
199 |
+
motion, label = self.motions[idx], self.labels[idx] # (M,T,J,C)
|
200 |
+
if self.random_move:
|
201 |
+
motion = random_move(motion)
|
202 |
+
if self.scale_range:
|
203 |
+
result = crop_scale(motion, scale_range=self.scale_range)
|
204 |
+
else:
|
205 |
+
result = motion
|
206 |
+
return result.astype(np.float32), label
|
lib/data/dataset_mesh.py
ADDED
@@ -0,0 +1,97 @@
|
|
|
|
|
|
|
|
|
|
|
|
|
|
|
|
|
|
|
|
|
|
|
|
|
|
|
|
|
|
|
|
|
|
|
|
|
|
|
|
|
|
|
|
|
|
|
|
|
|
|
|
|
|
|
|
|
|
|
|
|
|
|
|
|
|
|
|
|
|
|
|
|
|
|
|
|
|
|
|
|
|
|
|
|
|
|
|
|
|
|
|
|
|
|
|
|
|
|
|
|
|
|
|
|
|
|
|
|
|
|
|
|
|
|
|
|
|
|
|
|
|
|
|
|
|
|
|
|
|
|
|
|
|
|
|
|
|
|
|
|
|
|
|
|
|
|
|
|
|
|
|
|
|
|
|
|
|
|
|
|
|
|
|
|
|
|
|
|
|
|
|
|
|
|
|
|
|
|
|
|
|
|
|
|
|
|
|
|
|
|
|
|
|
|
|
|
1 |
+
import torch
|
2 |
+
import numpy as np
|
3 |
+
import glob
|
4 |
+
import os
|
5 |
+
import io
|
6 |
+
import random
|
7 |
+
import pickle
|
8 |
+
from torch.utils.data import Dataset, DataLoader
|
9 |
+
from lib.data.augmentation import Augmenter3D
|
10 |
+
from lib.utils.tools import read_pkl
|
11 |
+
from lib.utils.utils_data import flip_data, crop_scale
|
12 |
+
from lib.utils.utils_mesh import flip_thetas
|
13 |
+
from lib.utils.utils_smpl import SMPL
|
14 |
+
from torch.utils.data import Dataset, DataLoader
|
15 |
+
from lib.data.datareader_h36m import DataReaderH36M
|
16 |
+
from lib.data.datareader_mesh import DataReaderMesh
|
17 |
+
from lib.data.dataset_action import random_move
|
18 |
+
|
19 |
+
class SMPLDataset(Dataset):
|
20 |
+
def __init__(self, args, data_split, dataset): # data_split: train/test; dataset: h36m, coco, pw3d
|
21 |
+
random.seed(0)
|
22 |
+
np.random.seed(0)
|
23 |
+
self.clip_len = args.clip_len
|
24 |
+
self.data_split = data_split
|
25 |
+
if dataset=="h36m":
|
26 |
+
datareader = DataReaderH36M(n_frames=self.clip_len, sample_stride=args.sample_stride, data_stride_train=args.data_stride, data_stride_test=self.clip_len, dt_root=args.data_root, dt_file=args.dt_file_h36m)
|
27 |
+
elif dataset=="coco":
|
28 |
+
datareader = DataReaderMesh(n_frames=1, sample_stride=args.sample_stride, data_stride_train=1, data_stride_test=1, dt_root=args.data_root, dt_file=args.dt_file_coco, res=[640, 640])
|
29 |
+
elif dataset=="pw3d":
|
30 |
+
datareader = DataReaderMesh(n_frames=self.clip_len, sample_stride=args.sample_stride, data_stride_train=args.data_stride, data_stride_test=self.clip_len, dt_root=args.data_root, dt_file=args.dt_file_pw3d, res=[1920, 1920])
|
31 |
+
else:
|
32 |
+
raise Exception("Mesh dataset undefined.")
|
33 |
+
|
34 |
+
split_id_train, split_id_test = datareader.get_split_id() # Index of clips
|
35 |
+
train_data, test_data = datareader.read_2d()
|
36 |
+
train_data, test_data = train_data[split_id_train], test_data[split_id_test] # Input: (N, T, 17, 3)
|
37 |
+
self.motion_2d = {'train': train_data, 'test': test_data}[data_split]
|
38 |
+
|
39 |
+
dt = datareader.dt_dataset
|
40 |
+
smpl_pose_train = dt['train']['smpl_pose'][split_id_train] # (N, T, 72)
|
41 |
+
smpl_shape_train = dt['train']['smpl_shape'][split_id_train] # (N, T, 10)
|
42 |
+
smpl_pose_test = dt['test']['smpl_pose'][split_id_test] # (N, T, 72)
|
43 |
+
smpl_shape_test = dt['test']['smpl_shape'][split_id_test] # (N, T, 10)
|
44 |
+
|
45 |
+
self.motion_smpl_3d = {'train': {'pose': smpl_pose_train, 'shape': smpl_shape_train}, 'test': {'pose': smpl_pose_test, 'shape': smpl_shape_test}}[data_split]
|
46 |
+
self.smpl = SMPL(
|
47 |
+
args.data_root,
|
48 |
+
batch_size=1,
|
49 |
+
)
|
50 |
+
|
51 |
+
def __len__(self):
|
52 |
+
'Denotes the total number of samples'
|
53 |
+
return len(self.motion_2d)
|
54 |
+
|
55 |
+
def __getitem__(self, index):
|
56 |
+
raise NotImplementedError
|
57 |
+
|
58 |
+
class MotionSMPL(SMPLDataset):
|
59 |
+
def __init__(self, args, data_split, dataset):
|
60 |
+
super(MotionSMPL, self).__init__(args, data_split, dataset)
|
61 |
+
self.flip = args.flip
|
62 |
+
|
63 |
+
def __getitem__(self, index):
|
64 |
+
'Generates one sample of data'
|
65 |
+
# Select sample
|
66 |
+
motion_2d = self.motion_2d[index] # motion_2d: (T,17,3)
|
67 |
+
motion_2d[:,:,2] = np.clip(motion_2d[:,:,2], 0, 1)
|
68 |
+
motion_smpl_pose = self.motion_smpl_3d['pose'][index].reshape(-1, 24, 3) # motion_smpl_3d: (T, 24, 3)
|
69 |
+
motion_smpl_shape = self.motion_smpl_3d['shape'][index] # motion_smpl_3d: (T,10)
|
70 |
+
|
71 |
+
if self.data_split=="train":
|
72 |
+
if self.flip and random.random() > 0.5: # Training augmentation - random flipping
|
73 |
+
motion_2d = flip_data(motion_2d)
|
74 |
+
motion_smpl_pose = flip_thetas(motion_smpl_pose)
|
75 |
+
|
76 |
+
|
77 |
+
motion_smpl_pose = torch.from_numpy(motion_smpl_pose).reshape(-1, 72).float()
|
78 |
+
motion_smpl_shape = torch.from_numpy(motion_smpl_shape).reshape(-1, 10).float()
|
79 |
+
motion_smpl = self.smpl(
|
80 |
+
betas=motion_smpl_shape,
|
81 |
+
body_pose=motion_smpl_pose[:, 3:],
|
82 |
+
global_orient=motion_smpl_pose[:, :3],
|
83 |
+
pose2rot=True
|
84 |
+
)
|
85 |
+
motion_verts = motion_smpl.vertices.detach()*1000.0
|
86 |
+
J_regressor = self.smpl.J_regressor_h36m
|
87 |
+
J_regressor_batch = J_regressor[None, :].expand(motion_verts.shape[0], -1, -1).to(motion_verts.device)
|
88 |
+
motion_3d_reg = torch.matmul(J_regressor_batch, motion_verts) # motion_3d: (T,17,3)
|
89 |
+
motion_verts = motion_verts - motion_3d_reg[:, :1, :]
|
90 |
+
motion_3d_reg = motion_3d_reg - motion_3d_reg[:, :1, :] # motion_3d: (T,17,3)
|
91 |
+
motion_theta = torch.cat((motion_smpl_pose, motion_smpl_shape), -1)
|
92 |
+
motion_smpl_3d = {
|
93 |
+
'theta': motion_theta, # smpl pose and shape
|
94 |
+
'kp_3d': motion_3d_reg, # 3D keypoints
|
95 |
+
'verts': motion_verts, # 3D mesh vertices
|
96 |
+
}
|
97 |
+
return motion_2d, motion_smpl_3d
|
lib/data/dataset_motion_2d.py
ADDED
@@ -0,0 +1,148 @@
|
|
|
|
|
|
|
|
|
|
|
|
|
|
|
|
|
|
|
|
|
|
|
|
|
|
|
|
|
|
|
|
|
|
|
|
|
|
|
|
|
|
|
|
|
|
|
|
|
|
|
|
|
|
|
|
|
|
|
|
|
|
|
|
|
|
|
|
|
|
|
|
|
|
|
|
|
|
|
|
|
|
|
|
|
|
|
|
|
|
|
|
|
|
|
|
|
|
|
|
|
|
|
|
|
|
|
|
|
|
|
|
|
|
|
|
|
|
|
|
|
|
|
|
|
|
|
|
|
|
|
|
|
|
|
|
|
|
|
|
|
|
|
|
|
|
|
|
|
|
|
|
|
|
|
|
|
|
|
|
|
|
|
|
|
|
|
|
|
|
|
|
|
|
|
|
|
|
|
|
|
|
|
|
|
|
|
|
|
|
|
|
|
|
|
|
|
|
|
|
|
|
|
|
|
|
|
|
|
|
|
|
|
|
|
|
|
|
|
|
|
|
|
|
|
|
|
|
|
|
|
|
|
|
|
|
|
|
|
|
|
|
|
|
|
|
|
|
|
|
|
|
|
|
|
|
|
|
|
|
|
|
|
|
|
|
|
|
|
|
|
|
|
|
|
|
|
|
|
|
|
|
|
|
|
|
|
|
|
|
|
|
|
|
|
|
|
|
|
1 |
+
import sys
|
2 |
+
import torch
|
3 |
+
import torch.nn as nn
|
4 |
+
import torch.nn.functional as F
|
5 |
+
from torch.utils.data import Dataset, DataLoader
|
6 |
+
import numpy as np
|
7 |
+
import os
|
8 |
+
import random
|
9 |
+
import copy
|
10 |
+
import json
|
11 |
+
from collections import defaultdict
|
12 |
+
from lib.utils.utils_data import crop_scale, flip_data, resample, split_clips
|
13 |
+
|
14 |
+
def posetrack2h36m(x):
|
15 |
+
'''
|
16 |
+
Input: x (T x V x C)
|
17 |
+
|
18 |
+
PoseTrack keypoints = [ 'nose',
|
19 |
+
'head_bottom',
|
20 |
+
'head_top',
|
21 |
+
'left_ear',
|
22 |
+
'right_ear',
|
23 |
+
'left_shoulder',
|
24 |
+
'right_shoulder',
|
25 |
+
'left_elbow',
|
26 |
+
'right_elbow',
|
27 |
+
'left_wrist',
|
28 |
+
'right_wrist',
|
29 |
+
'left_hip',
|
30 |
+
'right_hip',
|
31 |
+
'left_knee',
|
32 |
+
'right_knee',
|
33 |
+
'left_ankle',
|
34 |
+
'right_ankle']
|
35 |
+
H36M:
|
36 |
+
0: 'root',
|
37 |
+
1: 'rhip',
|
38 |
+
2: 'rkne',
|
39 |
+
3: 'rank',
|
40 |
+
4: 'lhip',
|
41 |
+
5: 'lkne',
|
42 |
+
6: 'lank',
|
43 |
+
7: 'belly',
|
44 |
+
8: 'neck',
|
45 |
+
9: 'nose',
|
46 |
+
10: 'head',
|
47 |
+
11: 'lsho',
|
48 |
+
12: 'lelb',
|
49 |
+
13: 'lwri',
|
50 |
+
14: 'rsho',
|
51 |
+
15: 'relb',
|
52 |
+
16: 'rwri'
|
53 |
+
'''
|
54 |
+
y = np.zeros(x.shape)
|
55 |
+
y[:,0,:] = (x[:,11,:] + x[:,12,:]) * 0.5
|
56 |
+
y[:,1,:] = x[:,12,:]
|
57 |
+
y[:,2,:] = x[:,14,:]
|
58 |
+
y[:,3,:] = x[:,16,:]
|
59 |
+
y[:,4,:] = x[:,11,:]
|
60 |
+
y[:,5,:] = x[:,13,:]
|
61 |
+
y[:,6,:] = x[:,15,:]
|
62 |
+
y[:,8,:] = x[:,1,:]
|
63 |
+
y[:,7,:] = (y[:,0,:] + y[:,8,:]) * 0.5
|
64 |
+
y[:,9,:] = x[:,0,:]
|
65 |
+
y[:,10,:] = x[:,2,:]
|
66 |
+
y[:,11,:] = x[:,5,:]
|
67 |
+
y[:,12,:] = x[:,7,:]
|
68 |
+
y[:,13,:] = x[:,9,:]
|
69 |
+
y[:,14,:] = x[:,6,:]
|
70 |
+
y[:,15,:] = x[:,8,:]
|
71 |
+
y[:,16,:] = x[:,10,:]
|
72 |
+
y[:,0,2] = np.minimum(x[:,11,2], x[:,12,2])
|
73 |
+
y[:,7,2] = np.minimum(y[:,0,2], y[:,8,2])
|
74 |
+
return y
|
75 |
+
|
76 |
+
|
77 |
+
class PoseTrackDataset2D(Dataset):
|
78 |
+
def __init__(self, flip=True, scale_range=[0.25, 1]):
|
79 |
+
super(PoseTrackDataset2D, self).__init__()
|
80 |
+
self.flip = flip
|
81 |
+
data_root = "data/motion2d/posetrack18_annotations/train/"
|
82 |
+
file_list = sorted(os.listdir(data_root))
|
83 |
+
all_motions = []
|
84 |
+
all_motions_filtered = []
|
85 |
+
self.scale_range = scale_range
|
86 |
+
for filename in file_list:
|
87 |
+
with open(os.path.join(data_root, filename), 'r') as file:
|
88 |
+
json_dict = json.load(file)
|
89 |
+
annots = json_dict['annotations']
|
90 |
+
imgs = json_dict['images']
|
91 |
+
motions = defaultdict(list)
|
92 |
+
for annot in annots:
|
93 |
+
tid = annot['track_id']
|
94 |
+
pose2d = np.array(annot['keypoints']).reshape(-1,3)
|
95 |
+
motions[tid].append(pose2d)
|
96 |
+
all_motions += list(motions.values())
|
97 |
+
for motion in all_motions:
|
98 |
+
if len(motion)<30:
|
99 |
+
continue
|
100 |
+
motion = np.array(motion[:30])
|
101 |
+
if np.sum(motion[:,:,2]) <= 306: # Valid joint num threshold
|
102 |
+
continue
|
103 |
+
motion = crop_scale(motion, self.scale_range)
|
104 |
+
motion = posetrack2h36m(motion)
|
105 |
+
motion[motion[:,:,2]==0] = 0
|
106 |
+
if np.sum(motion[:,0,2]) < 30:
|
107 |
+
continue # Root all visible (needed for framewise rootrel)
|
108 |
+
all_motions_filtered.append(motion)
|
109 |
+
all_motions_filtered = np.array(all_motions_filtered)
|
110 |
+
self.motions_2d = all_motions_filtered
|
111 |
+
|
112 |
+
def __len__(self):
|
113 |
+
'Denotes the total number of samples'
|
114 |
+
return len(self.motions_2d)
|
115 |
+
|
116 |
+
def __getitem__(self, index):
|
117 |
+
'Generates one sample of data'
|
118 |
+
motion_2d = torch.FloatTensor(self.motions_2d[index])
|
119 |
+
if self.flip and random.random()>0.5:
|
120 |
+
motion_2d = flip_data(motion_2d)
|
121 |
+
return motion_2d, motion_2d
|
122 |
+
|
123 |
+
class InstaVDataset2D(Dataset):
|
124 |
+
def __init__(self, n_frames=81, data_stride=27, flip=True, valid_threshold=0.0, scale_range=[0.25, 1]):
|
125 |
+
super(InstaVDataset2D, self).__init__()
|
126 |
+
self.flip = flip
|
127 |
+
self.scale_range = scale_range
|
128 |
+
motion_all = np.load('data/motion2d/InstaVariety/motion_all.npy')
|
129 |
+
id_all = np.load('data/motion2d/InstaVariety/id_all.npy')
|
130 |
+
split_id = split_clips(id_all, n_frames, data_stride)
|
131 |
+
motions_2d = motion_all[split_id] # [N, T, 17, 3]
|
132 |
+
valid_idx = (motions_2d[:,0,0,2] > valid_threshold)
|
133 |
+
self.motions_2d = motions_2d[valid_idx]
|
134 |
+
|
135 |
+
def __len__(self):
|
136 |
+
'Denotes the total number of samples'
|
137 |
+
return len(self.motions_2d)
|
138 |
+
|
139 |
+
def __getitem__(self, index):
|
140 |
+
'Generates one sample of data'
|
141 |
+
motion_2d = self.motions_2d[index]
|
142 |
+
motion_2d = crop_scale(motion_2d, self.scale_range)
|
143 |
+
motion_2d[motion_2d[:,:,2]==0] = 0
|
144 |
+
if self.flip and random.random()>0.5:
|
145 |
+
motion_2d = flip_data(motion_2d)
|
146 |
+
motion_2d = torch.FloatTensor(motion_2d)
|
147 |
+
return motion_2d, motion_2d
|
148 |
+
|
lib/data/dataset_motion_3d.py
ADDED
@@ -0,0 +1,68 @@
|
|
|
|
|
|
|
|
|
|
|
|
|
|
|
|
|
|
|
|
|
|
|
|
|
|
|
|
|
|
|
|
|
|
|
|
|
|
|
|
|
|
|
|
|
|
|
|
|
|
|
|
|
|
|
|
|
|
|
|
|
|
|
|
|
|
|
|
|
|
|
|
|
|
|
|
|
|
|
|
|
|
|
|
|
|
|
|
|
|
|
|
|
|
|
|
|
|
|
|
|
|
|
|
|
|
|
|
|
|
|
|
|
|
|
|
|
|
|
|
|
|
|
|
|
|
|
|
|
|
|
|
|
|
|
|
|
|
|
1 |
+
import torch
|
2 |
+
import numpy as np
|
3 |
+
import glob
|
4 |
+
import os
|
5 |
+
import io
|
6 |
+
import random
|
7 |
+
import pickle
|
8 |
+
from torch.utils.data import Dataset, DataLoader
|
9 |
+
from lib.data.augmentation import Augmenter3D
|
10 |
+
from lib.utils.tools import read_pkl
|
11 |
+
from lib.utils.utils_data import flip_data
|
12 |
+
|
13 |
+
class MotionDataset(Dataset):
|
14 |
+
def __init__(self, args, subset_list, data_split): # data_split: train/test
|
15 |
+
np.random.seed(0)
|
16 |
+
self.data_root = args.data_root
|
17 |
+
self.subset_list = subset_list
|
18 |
+
self.data_split = data_split
|
19 |
+
file_list_all = []
|
20 |
+
for subset in self.subset_list:
|
21 |
+
data_path = os.path.join(self.data_root, subset, self.data_split)
|
22 |
+
motion_list = sorted(os.listdir(data_path))
|
23 |
+
for i in motion_list:
|
24 |
+
file_list_all.append(os.path.join(data_path, i))
|
25 |
+
self.file_list = file_list_all
|
26 |
+
|
27 |
+
def __len__(self):
|
28 |
+
'Denotes the total number of samples'
|
29 |
+
return len(self.file_list)
|
30 |
+
|
31 |
+
def __getitem__(self, index):
|
32 |
+
raise NotImplementedError
|
33 |
+
|
34 |
+
class MotionDataset3D(MotionDataset):
|
35 |
+
def __init__(self, args, subset_list, data_split):
|
36 |
+
super(MotionDataset3D, self).__init__(args, subset_list, data_split)
|
37 |
+
self.flip = args.flip
|
38 |
+
self.synthetic = args.synthetic
|
39 |
+
self.aug = Augmenter3D(args)
|
40 |
+
self.gt_2d = args.gt_2d
|
41 |
+
|
42 |
+
def __getitem__(self, index):
|
43 |
+
'Generates one sample of data'
|
44 |
+
# Select sample
|
45 |
+
file_path = self.file_list[index]
|
46 |
+
motion_file = read_pkl(file_path)
|
47 |
+
motion_3d = motion_file["data_label"]
|
48 |
+
if self.data_split=="train":
|
49 |
+
if self.synthetic or self.gt_2d:
|
50 |
+
motion_3d = self.aug.augment3D(motion_3d)
|
51 |
+
motion_2d = np.zeros(motion_3d.shape, dtype=np.float32)
|
52 |
+
motion_2d[:,:,:2] = motion_3d[:,:,:2]
|
53 |
+
motion_2d[:,:,2] = 1 # No 2D detection, use GT xy and c=1.
|
54 |
+
elif motion_file["data_input"] is not None: # Have 2D detection
|
55 |
+
motion_2d = motion_file["data_input"]
|
56 |
+
if self.flip and random.random() > 0.5: # Training augmentation - random flipping
|
57 |
+
motion_2d = flip_data(motion_2d)
|
58 |
+
motion_3d = flip_data(motion_3d)
|
59 |
+
else:
|
60 |
+
raise ValueError('Training illegal.')
|
61 |
+
elif self.data_split=="test":
|
62 |
+
motion_2d = motion_file["data_input"]
|
63 |
+
if self.gt_2d:
|
64 |
+
motion_2d[:,:,:2] = motion_3d[:,:,:2]
|
65 |
+
motion_2d[:,:,2] = 1
|
66 |
+
else:
|
67 |
+
raise ValueError('Data split unknown.')
|
68 |
+
return torch.FloatTensor(motion_2d), torch.FloatTensor(motion_3d)
|
lib/data/dataset_wild.py
ADDED
@@ -0,0 +1,102 @@
|
|
|
|
|
|
|
|
|
|
|
|
|
|
|
|
|
|
|
|
|
|
|
|
|
|
|
|
|
|
|
|
|
|
|
|
|
|
|
|
|
|
|
|
|
|
|
|
|
|
|
|
|
|
|
|
|
|
|
|
|
|
|
|
|
|
|
|
|
|
|
|
|
|
|
|
|
|
|
|
|
|
|
|
|
|
|
|
|
|
|
|
|
|
|
|
|
|
|
|
|
|
|
|
|
|
|
|
|
|
|
|
|
|
|
|
|
|
|
|
|
|
|
|
|
|
|
|
|
|
|
|
|
|
|
|
|
|
|
|
|
|
|
|
|
|
|
|
|
|
|
|
|
|
|
|
|
|
|
|
|
|
|
|
|
|
|
|
|
|
|
|
|
|
|
|
|
|
|
|
|
|
|
|
|
|
|
|
|
|
|
|
|
|
|
|
|
|
|
|
|
|
|
|
|
|
|
1 |
+
import torch
|
2 |
+
import numpy as np
|
3 |
+
import ipdb
|
4 |
+
import glob
|
5 |
+
import os
|
6 |
+
import io
|
7 |
+
import math
|
8 |
+
import random
|
9 |
+
import json
|
10 |
+
import pickle
|
11 |
+
import math
|
12 |
+
from torch.utils.data import Dataset, DataLoader
|
13 |
+
from lib.utils.utils_data import crop_scale
|
14 |
+
|
15 |
+
def halpe2h36m(x):
|
16 |
+
'''
|
17 |
+
Input: x (T x V x C)
|
18 |
+
//Halpe 26 body keypoints
|
19 |
+
{0, "Nose"},
|
20 |
+
{1, "LEye"},
|
21 |
+
{2, "REye"},
|
22 |
+
{3, "LEar"},
|
23 |
+
{4, "REar"},
|
24 |
+
{5, "LShoulder"},
|
25 |
+
{6, "RShoulder"},
|
26 |
+
{7, "LElbow"},
|
27 |
+
{8, "RElbow"},
|
28 |
+
{9, "LWrist"},
|
29 |
+
{10, "RWrist"},
|
30 |
+
{11, "LHip"},
|
31 |
+
{12, "RHip"},
|
32 |
+
{13, "LKnee"},
|
33 |
+
{14, "Rknee"},
|
34 |
+
{15, "LAnkle"},
|
35 |
+
{16, "RAnkle"},
|
36 |
+
{17, "Head"},
|
37 |
+
{18, "Neck"},
|
38 |
+
{19, "Hip"},
|
39 |
+
{20, "LBigToe"},
|
40 |
+
{21, "RBigToe"},
|
41 |
+
{22, "LSmallToe"},
|
42 |
+
{23, "RSmallToe"},
|
43 |
+
{24, "LHeel"},
|
44 |
+
{25, "RHeel"},
|
45 |
+
'''
|
46 |
+
T, V, C = x.shape
|
47 |
+
y = np.zeros([T,17,C])
|
48 |
+
y[:,0,:] = x[:,19,:]
|
49 |
+
y[:,1,:] = x[:,12,:]
|
50 |
+
y[:,2,:] = x[:,14,:]
|
51 |
+
y[:,3,:] = x[:,16,:]
|
52 |
+
y[:,4,:] = x[:,11,:]
|
53 |
+
y[:,5,:] = x[:,13,:]
|
54 |
+
y[:,6,:] = x[:,15,:]
|
55 |
+
y[:,7,:] = (x[:,18,:] + x[:,19,:]) * 0.5
|
56 |
+
y[:,8,:] = x[:,18,:]
|
57 |
+
y[:,9,:] = x[:,0,:]
|
58 |
+
y[:,10,:] = x[:,17,:]
|
59 |
+
y[:,11,:] = x[:,5,:]
|
60 |
+
y[:,12,:] = x[:,7,:]
|
61 |
+
y[:,13,:] = x[:,9,:]
|
62 |
+
y[:,14,:] = x[:,6,:]
|
63 |
+
y[:,15,:] = x[:,8,:]
|
64 |
+
y[:,16,:] = x[:,10,:]
|
65 |
+
return y
|
66 |
+
|
67 |
+
def read_input(json_path, vid_size, scale_range, focus):
|
68 |
+
with open(json_path, "r") as read_file:
|
69 |
+
results = json.load(read_file)
|
70 |
+
kpts_all = []
|
71 |
+
for item in results:
|
72 |
+
if focus!=None and item['idx']!=focus:
|
73 |
+
continue
|
74 |
+
kpts = np.array(item['keypoints']).reshape([-1,3])
|
75 |
+
kpts_all.append(kpts)
|
76 |
+
kpts_all = np.array(kpts_all)
|
77 |
+
kpts_all = halpe2h36m(kpts_all)
|
78 |
+
if vid_size:
|
79 |
+
w, h = vid_size
|
80 |
+
scale = min(w,h) / 2.0
|
81 |
+
kpts_all[:,:,:2] = kpts_all[:,:,:2] - np.array([w, h]) / 2.0
|
82 |
+
kpts_all[:,:,:2] = kpts_all[:,:,:2] / scale
|
83 |
+
motion = kpts_all
|
84 |
+
if scale_range:
|
85 |
+
motion = crop_scale(kpts_all, scale_range)
|
86 |
+
return motion.astype(np.float32)
|
87 |
+
|
88 |
+
class WildDetDataset(Dataset):
|
89 |
+
def __init__(self, json_path, clip_len=243, vid_size=None, scale_range=None, focus=None):
|
90 |
+
self.json_path = json_path
|
91 |
+
self.clip_len = clip_len
|
92 |
+
self.vid_all = read_input(json_path, vid_size, scale_range, focus)
|
93 |
+
|
94 |
+
def __len__(self):
|
95 |
+
'Denotes the total number of samples'
|
96 |
+
return math.ceil(len(self.vid_all) / self.clip_len)
|
97 |
+
|
98 |
+
def __getitem__(self, index):
|
99 |
+
'Generates one sample of data'
|
100 |
+
st = index*self.clip_len
|
101 |
+
end = min((index+1)*self.clip_len, len(self.vid_all))
|
102 |
+
return self.vid_all[st:end]
|
lib/model/DSTformer.py
ADDED
@@ -0,0 +1,362 @@
|
|
|
|
|
|
|
|
|
|
|
|
|
|
|
|
|
|
|
|
|
|
|
|
|
|
|
|
|
|
|
|
|
|
|
|
|
|
|
|
|
|
|
|
|
|
|
|
|
|
|
|
|
|
|
|
|
|
|
|
|
|
|
|
|
|
|
|
|
|
|
|
|
|
|
|
|
|
|
|
|
|
|
|
|
|
|
|
|
|
|
|
|
|
|
|
|
|
|
|
|
|
|
|
|
|
|
|
|
|
|
|
|
|
|
|
|
|
|
|
|
|
|
|
|
|
|
|
|
|
|
|
|
|
|
|
|
|
|
|
|
|
|
|
|
|
|
|
|
|
|
|
|
|
|
|
|
|
|
|
|
|
|
|
|
|
|
|
|
|
|
|
|
|
|
|
|
|
|
|
|
|
|
|
|
|
|
|
|
|
|
|
|
|
|
|
|
|
|
|
|
|
|
|
|
|
|
|
|
|
|
|
|
|
|
|
|
|
|
|
|
|
|
|
|
|
|
|
|
|
|
|
|
|
|
|
|
|
|
|
|
|
|
|
|
|
|
|
|
|
|
|
|
|
|
|
|
|
|
|
|
|
|
|
|
|
|
|
|
|
|
|
|
|
|
|
|
|
|
|
|
|
|
|
|
|
|
|
|
|
|
|
|
|
|
|
|
|
|
|
|
|
|
|
|
|
|
|
|
|
|
|
|
|
|
|
|
|
|
|
|
|
|
|
|
|
|
|
|
|
|
|
|
|
|
|
|
|
|
|
|
|
|
|
|
|
|
|
|
|
|
|
|
|
|
|
|
|
|
|
|
|
|
|
|
|
|
|
|
|
|
|
|
|
|
|
|
|
|
|
|
|
|
|
|
|
|
|
|
|
|
|
|
|
|
|
|
|
|
|
|
|
|
|
|
|
|
|
|
|
|
|
|
|
|
|
|
|
|
|
|
|
|
|
|
|
|
|
|
|
|
|
|
|
|
|
|
|
|
|
|
|
|
|
|
|
|
|
|
|
|
|
|
|
|
|
|
|
|
|
|
|
|
|
|
|
|
|
|
|
|
|
|
|
|
|
|
|
|
|
|
|
|
|
|
|
|
|
|
|
|
|
|
|
|
|
|
|
|
|
|
|
|
|
|
|
|
|
|
|
|
|
|
|
|
|
|
|
|
|
|
|
|
|
|
|
|
|
|
|
|
|
|
|
|
|
|
|
|
|
|
|
|
|
|
|
|
|
|
|
|
|
|
|
|
|
|
|
|
|
|
|
|
|
|
|
|
|
|
|
|
|
|
|
|
|
|
|
|
|
|
|
|
|
|
|
|
|
|
|
|
|
|
|
|
|
|
|
|
|
|
|
|
|
|
|
|
|
|
|
|
|
|
|
|
|
|
|
|
|
|
|
|
|
|
|
|
|
|
|
|
|
|
|
|
|
|
|
|
|
|
|
|
|
|
|
|
|
|
|
|
|
|
|
|
|
|
|
|
|
|
|
|
|
|
|
|
|
|
|
|
|
|
|
|
|
|
|
|
|
|
|
|
|
|
|
|
|
|
|
|
|
|
|
|
|
|
|
|
|
|
|
|
|
|
|
|
|
|
|
|
|
|
|
|
|
|
|
|
|
|
|
|
|
|
|
|
1 |
+
import torch
|
2 |
+
import torch.nn as nn
|
3 |
+
import math
|
4 |
+
import warnings
|
5 |
+
import random
|
6 |
+
import numpy as np
|
7 |
+
from collections import OrderedDict
|
8 |
+
from functools import partial
|
9 |
+
from itertools import repeat
|
10 |
+
from lib.model.drop import DropPath
|
11 |
+
|
12 |
+
def _no_grad_trunc_normal_(tensor, mean, std, a, b):
|
13 |
+
# Cut & paste from PyTorch official master until it's in a few official releases - RW
|
14 |
+
# Method based on https://people.sc.fsu.edu/~jburkardt/presentations/truncated_normal.pdf
|
15 |
+
def norm_cdf(x):
|
16 |
+
# Computes standard normal cumulative distribution function
|
17 |
+
return (1. + math.erf(x / math.sqrt(2.))) / 2.
|
18 |
+
|
19 |
+
if (mean < a - 2 * std) or (mean > b + 2 * std):
|
20 |
+
warnings.warn("mean is more than 2 std from [a, b] in nn.init.trunc_normal_. "
|
21 |
+
"The distribution of values may be incorrect.",
|
22 |
+
stacklevel=2)
|
23 |
+
|
24 |
+
with torch.no_grad():
|
25 |
+
# Values are generated by using a truncated uniform distribution and
|
26 |
+
# then using the inverse CDF for the normal distribution.
|
27 |
+
# Get upper and lower cdf values
|
28 |
+
l = norm_cdf((a - mean) / std)
|
29 |
+
u = norm_cdf((b - mean) / std)
|
30 |
+
|
31 |
+
# Uniformly fill tensor with values from [l, u], then translate to
|
32 |
+
# [2l-1, 2u-1].
|
33 |
+
tensor.uniform_(2 * l - 1, 2 * u - 1)
|
34 |
+
|
35 |
+
# Use inverse cdf transform for normal distribution to get truncated
|
36 |
+
# standard normal
|
37 |
+
tensor.erfinv_()
|
38 |
+
|
39 |
+
# Transform to proper mean, std
|
40 |
+
tensor.mul_(std * math.sqrt(2.))
|
41 |
+
tensor.add_(mean)
|
42 |
+
|
43 |
+
# Clamp to ensure it's in the proper range
|
44 |
+
tensor.clamp_(min=a, max=b)
|
45 |
+
return tensor
|
46 |
+
|
47 |
+
|
48 |
+
def trunc_normal_(tensor, mean=0., std=1., a=-2., b=2.):
|
49 |
+
# type: (Tensor, float, float, float, float) -> Tensor
|
50 |
+
r"""Fills the input Tensor with values drawn from a truncated
|
51 |
+
normal distribution. The values are effectively drawn from the
|
52 |
+
normal distribution :math:`\mathcal{N}(\text{mean}, \text{std}^2)`
|
53 |
+
with values outside :math:`[a, b]` redrawn until they are within
|
54 |
+
the bounds. The method used for generating the random values works
|
55 |
+
best when :math:`a \leq \text{mean} \leq b`.
|
56 |
+
Args:
|
57 |
+
tensor: an n-dimensional `torch.Tensor`
|
58 |
+
mean: the mean of the normal distribution
|
59 |
+
std: the standard deviation of the normal distribution
|
60 |
+
a: the minimum cutoff value
|
61 |
+
b: the maximum cutoff value
|
62 |
+
Examples:
|
63 |
+
>>> w = torch.empty(3, 5)
|
64 |
+
>>> nn.init.trunc_normal_(w)
|
65 |
+
"""
|
66 |
+
return _no_grad_trunc_normal_(tensor, mean, std, a, b)
|
67 |
+
|
68 |
+
|
69 |
+
class MLP(nn.Module):
|
70 |
+
def __init__(self, in_features, hidden_features=None, out_features=None, act_layer=nn.GELU, drop=0.):
|
71 |
+
super().__init__()
|
72 |
+
out_features = out_features or in_features
|
73 |
+
hidden_features = hidden_features or in_features
|
74 |
+
self.fc1 = nn.Linear(in_features, hidden_features)
|
75 |
+
self.act = act_layer()
|
76 |
+
self.fc2 = nn.Linear(hidden_features, out_features)
|
77 |
+
self.drop = nn.Dropout(drop)
|
78 |
+
|
79 |
+
def forward(self, x):
|
80 |
+
x = self.fc1(x)
|
81 |
+
x = self.act(x)
|
82 |
+
x = self.drop(x)
|
83 |
+
x = self.fc2(x)
|
84 |
+
x = self.drop(x)
|
85 |
+
return x
|
86 |
+
|
87 |
+
|
88 |
+
class Attention(nn.Module):
|
89 |
+
def __init__(self, dim, num_heads=8, qkv_bias=False, qk_scale=None, attn_drop=0., proj_drop=0., st_mode='vanilla'):
|
90 |
+
super().__init__()
|
91 |
+
self.num_heads = num_heads
|
92 |
+
head_dim = dim // num_heads
|
93 |
+
# NOTE scale factor was wrong in my original version, can set manually to be compat with prev weights
|
94 |
+
self.scale = qk_scale or head_dim ** -0.5
|
95 |
+
|
96 |
+
self.attn_drop = nn.Dropout(attn_drop)
|
97 |
+
self.proj = nn.Linear(dim, dim)
|
98 |
+
self.mode = st_mode
|
99 |
+
if self.mode == 'parallel':
|
100 |
+
self.ts_attn = nn.Linear(dim*2, dim*2)
|
101 |
+
self.qkv = nn.Linear(dim, dim * 3, bias=qkv_bias)
|
102 |
+
else:
|
103 |
+
self.qkv = nn.Linear(dim, dim * 3, bias=qkv_bias)
|
104 |
+
self.proj_drop = nn.Dropout(proj_drop)
|
105 |
+
|
106 |
+
self.attn_count_s = None
|
107 |
+
self.attn_count_t = None
|
108 |
+
|
109 |
+
def forward(self, x, seqlen=1):
|
110 |
+
B, N, C = x.shape
|
111 |
+
|
112 |
+
if self.mode == 'series':
|
113 |
+
qkv = self.qkv(x).reshape(B, N, 3, self.num_heads, C // self.num_heads).permute(2, 0, 3, 1, 4)
|
114 |
+
q, k, v = qkv[0], qkv[1], qkv[2] # make torchscript happy (cannot use tensor as tuple)
|
115 |
+
x = self.forward_spatial(q, k, v)
|
116 |
+
qkv = self.qkv(x).reshape(B, N, 3, self.num_heads, C // self.num_heads).permute(2, 0, 3, 1, 4)
|
117 |
+
q, k, v = qkv[0], qkv[1], qkv[2] # make torchscript happy (cannot use tensor as tuple)
|
118 |
+
x = self.forward_temporal(q, k, v, seqlen=seqlen)
|
119 |
+
elif self.mode == 'parallel':
|
120 |
+
qkv = self.qkv(x).reshape(B, N, 3, self.num_heads, C // self.num_heads).permute(2, 0, 3, 1, 4)
|
121 |
+
q, k, v = qkv[0], qkv[1], qkv[2] # make torchscript happy (cannot use tensor as tuple)
|
122 |
+
x_t = self.forward_temporal(q, k, v, seqlen=seqlen)
|
123 |
+
x_s = self.forward_spatial(q, k, v)
|
124 |
+
|
125 |
+
alpha = torch.cat([x_s, x_t], dim=-1)
|
126 |
+
alpha = alpha.mean(dim=1, keepdim=True)
|
127 |
+
alpha = self.ts_attn(alpha).reshape(B, 1, C, 2)
|
128 |
+
alpha = alpha.softmax(dim=-1)
|
129 |
+
x = x_t * alpha[:,:,:,1] + x_s * alpha[:,:,:,0]
|
130 |
+
elif self.mode == 'coupling':
|
131 |
+
qkv = self.qkv(x).reshape(B, N, 3, self.num_heads, C // self.num_heads).permute(2, 0, 3, 1, 4)
|
132 |
+
q, k, v = qkv[0], qkv[1], qkv[2] # make torchscript happy (cannot use tensor as tuple)
|
133 |
+
x = self.forward_coupling(q, k, v, seqlen=seqlen)
|
134 |
+
elif self.mode == 'vanilla':
|
135 |
+
qkv = self.qkv(x).reshape(B, N, 3, self.num_heads, C // self.num_heads).permute(2, 0, 3, 1, 4)
|
136 |
+
q, k, v = qkv[0], qkv[1], qkv[2] # make torchscript happy (cannot use tensor as tuple)
|
137 |
+
x = self.forward_spatial(q, k, v)
|
138 |
+
elif self.mode == 'temporal':
|
139 |
+
qkv = self.qkv(x).reshape(B, N, 3, self.num_heads, C // self.num_heads).permute(2, 0, 3, 1, 4)
|
140 |
+
q, k, v = qkv[0], qkv[1], qkv[2] # make torchscript happy (cannot use tensor as tuple)
|
141 |
+
x = self.forward_temporal(q, k, v, seqlen=seqlen)
|
142 |
+
elif self.mode == 'spatial':
|
143 |
+
qkv = self.qkv(x).reshape(B, N, 3, self.num_heads, C // self.num_heads).permute(2, 0, 3, 1, 4)
|
144 |
+
q, k, v = qkv[0], qkv[1], qkv[2] # make torchscript happy (cannot use tensor as tuple)
|
145 |
+
x = self.forward_spatial(q, k, v)
|
146 |
+
else:
|
147 |
+
raise NotImplementedError(self.mode)
|
148 |
+
x = self.proj(x)
|
149 |
+
x = self.proj_drop(x)
|
150 |
+
return x
|
151 |
+
|
152 |
+
def reshape_T(self, x, seqlen=1, inverse=False):
|
153 |
+
if not inverse:
|
154 |
+
N, C = x.shape[-2:]
|
155 |
+
x = x.reshape(-1, seqlen, self.num_heads, N, C).transpose(1,2)
|
156 |
+
x = x.reshape(-1, self.num_heads, seqlen*N, C) #(B, H, TN, c)
|
157 |
+
else:
|
158 |
+
TN, C = x.shape[-2:]
|
159 |
+
x = x.reshape(-1, self.num_heads, seqlen, TN // seqlen, C).transpose(1,2)
|
160 |
+
x = x.reshape(-1, self.num_heads, TN // seqlen, C) #(BT, H, N, C)
|
161 |
+
return x
|
162 |
+
|
163 |
+
def forward_coupling(self, q, k, v, seqlen=8):
|
164 |
+
BT, _, N, C = q.shape
|
165 |
+
q = self.reshape_T(q, seqlen)
|
166 |
+
k = self.reshape_T(k, seqlen)
|
167 |
+
v = self.reshape_T(v, seqlen)
|
168 |
+
|
169 |
+
attn = (q @ k.transpose(-2, -1)) * self.scale
|
170 |
+
attn = attn.softmax(dim=-1)
|
171 |
+
attn = self.attn_drop(attn)
|
172 |
+
|
173 |
+
x = attn @ v
|
174 |
+
x = self.reshape_T(x, seqlen, inverse=True)
|
175 |
+
x = x.transpose(1,2).reshape(BT, N, C*self.num_heads)
|
176 |
+
return x
|
177 |
+
|
178 |
+
def forward_spatial(self, q, k, v):
|
179 |
+
B, _, N, C = q.shape
|
180 |
+
attn = (q @ k.transpose(-2, -1)) * self.scale
|
181 |
+
attn = attn.softmax(dim=-1)
|
182 |
+
attn = self.attn_drop(attn)
|
183 |
+
|
184 |
+
x = attn @ v
|
185 |
+
x = x.transpose(1,2).reshape(B, N, C*self.num_heads)
|
186 |
+
return x
|
187 |
+
|
188 |
+
def forward_temporal(self, q, k, v, seqlen=8):
|
189 |
+
B, _, N, C = q.shape
|
190 |
+
qt = q.reshape(-1, seqlen, self.num_heads, N, C).permute(0, 2, 3, 1, 4) #(B, H, N, T, C)
|
191 |
+
kt = k.reshape(-1, seqlen, self.num_heads, N, C).permute(0, 2, 3, 1, 4) #(B, H, N, T, C)
|
192 |
+
vt = v.reshape(-1, seqlen, self.num_heads, N, C).permute(0, 2, 3, 1, 4) #(B, H, N, T, C)
|
193 |
+
|
194 |
+
attn = (qt @ kt.transpose(-2, -1)) * self.scale
|
195 |
+
attn = attn.softmax(dim=-1)
|
196 |
+
attn = self.attn_drop(attn)
|
197 |
+
|
198 |
+
x = attn @ vt #(B, H, N, T, C)
|
199 |
+
x = x.permute(0, 3, 2, 1, 4).reshape(B, N, C*self.num_heads)
|
200 |
+
return x
|
201 |
+
|
202 |
+
def count_attn(self, attn):
|
203 |
+
attn = attn.detach().cpu().numpy()
|
204 |
+
attn = attn.mean(axis=1)
|
205 |
+
attn_t = attn[:, :, 1].mean(axis=1)
|
206 |
+
attn_s = attn[:, :, 0].mean(axis=1)
|
207 |
+
if self.attn_count_s is None:
|
208 |
+
self.attn_count_s = attn_s
|
209 |
+
self.attn_count_t = attn_t
|
210 |
+
else:
|
211 |
+
self.attn_count_s = np.concatenate([self.attn_count_s, attn_s], axis=0)
|
212 |
+
self.attn_count_t = np.concatenate([self.attn_count_t, attn_t], axis=0)
|
213 |
+
|
214 |
+
class Block(nn.Module):
|
215 |
+
|
216 |
+
def __init__(self, dim, num_heads, mlp_ratio=4., mlp_out_ratio=1., qkv_bias=True, qk_scale=None, drop=0., attn_drop=0.,
|
217 |
+
drop_path=0., act_layer=nn.GELU, norm_layer=nn.LayerNorm, st_mode='stage_st', att_fuse=False):
|
218 |
+
super().__init__()
|
219 |
+
# assert 'stage' in st_mode
|
220 |
+
self.st_mode = st_mode
|
221 |
+
self.norm1_s = norm_layer(dim)
|
222 |
+
self.norm1_t = norm_layer(dim)
|
223 |
+
self.attn_s = Attention(
|
224 |
+
dim, num_heads=num_heads, qkv_bias=qkv_bias, qk_scale=qk_scale, attn_drop=attn_drop, proj_drop=drop, st_mode="spatial")
|
225 |
+
self.attn_t = Attention(
|
226 |
+
dim, num_heads=num_heads, qkv_bias=qkv_bias, qk_scale=qk_scale, attn_drop=attn_drop, proj_drop=drop, st_mode="temporal")
|
227 |
+
|
228 |
+
# NOTE: drop path for stochastic depth, we shall see if this is better than dropout here
|
229 |
+
self.drop_path = DropPath(drop_path) if drop_path > 0. else nn.Identity()
|
230 |
+
self.norm2_s = norm_layer(dim)
|
231 |
+
self.norm2_t = norm_layer(dim)
|
232 |
+
mlp_hidden_dim = int(dim * mlp_ratio)
|
233 |
+
mlp_out_dim = int(dim * mlp_out_ratio)
|
234 |
+
self.mlp_s = MLP(in_features=dim, hidden_features=mlp_hidden_dim, out_features=mlp_out_dim, act_layer=act_layer, drop=drop)
|
235 |
+
self.mlp_t = MLP(in_features=dim, hidden_features=mlp_hidden_dim, out_features=mlp_out_dim, act_layer=act_layer, drop=drop)
|
236 |
+
self.att_fuse = att_fuse
|
237 |
+
if self.att_fuse:
|
238 |
+
self.ts_attn = nn.Linear(dim*2, dim*2)
|
239 |
+
def forward(self, x, seqlen=1):
|
240 |
+
if self.st_mode=='stage_st':
|
241 |
+
x = x + self.drop_path(self.attn_s(self.norm1_s(x), seqlen))
|
242 |
+
x = x + self.drop_path(self.mlp_s(self.norm2_s(x)))
|
243 |
+
x = x + self.drop_path(self.attn_t(self.norm1_t(x), seqlen))
|
244 |
+
x = x + self.drop_path(self.mlp_t(self.norm2_t(x)))
|
245 |
+
elif self.st_mode=='stage_ts':
|
246 |
+
x = x + self.drop_path(self.attn_t(self.norm1_t(x), seqlen))
|
247 |
+
x = x + self.drop_path(self.mlp_t(self.norm2_t(x)))
|
248 |
+
x = x + self.drop_path(self.attn_s(self.norm1_s(x), seqlen))
|
249 |
+
x = x + self.drop_path(self.mlp_s(self.norm2_s(x)))
|
250 |
+
elif self.st_mode=='stage_para':
|
251 |
+
x_t = x + self.drop_path(self.attn_t(self.norm1_t(x), seqlen))
|
252 |
+
x_t = x_t + self.drop_path(self.mlp_t(self.norm2_t(x_t)))
|
253 |
+
x_s = x + self.drop_path(self.attn_s(self.norm1_s(x), seqlen))
|
254 |
+
x_s = x_s + self.drop_path(self.mlp_s(self.norm2_s(x_s)))
|
255 |
+
if self.att_fuse:
|
256 |
+
# x_s, x_t: [BF, J, dim]
|
257 |
+
alpha = torch.cat([x_s, x_t], dim=-1)
|
258 |
+
BF, J = alpha.shape[:2]
|
259 |
+
# alpha = alpha.mean(dim=1, keepdim=True)
|
260 |
+
alpha = self.ts_attn(alpha).reshape(BF, J, -1, 2)
|
261 |
+
alpha = alpha.softmax(dim=-1)
|
262 |
+
x = x_t * alpha[:,:,:,1] + x_s * alpha[:,:,:,0]
|
263 |
+
else:
|
264 |
+
x = (x_s + x_t)*0.5
|
265 |
+
else:
|
266 |
+
raise NotImplementedError(self.st_mode)
|
267 |
+
return x
|
268 |
+
|
269 |
+
class DSTformer(nn.Module):
|
270 |
+
def __init__(self, dim_in=3, dim_out=3, dim_feat=256, dim_rep=512,
|
271 |
+
depth=5, num_heads=8, mlp_ratio=4,
|
272 |
+
num_joints=17, maxlen=243,
|
273 |
+
qkv_bias=True, qk_scale=None, drop_rate=0., attn_drop_rate=0., drop_path_rate=0., norm_layer=nn.LayerNorm, att_fuse=True):
|
274 |
+
super().__init__()
|
275 |
+
self.dim_out = dim_out
|
276 |
+
self.dim_feat = dim_feat
|
277 |
+
self.joints_embed = nn.Linear(dim_in, dim_feat)
|
278 |
+
self.pos_drop = nn.Dropout(p=drop_rate)
|
279 |
+
dpr = [x.item() for x in torch.linspace(0, drop_path_rate, depth)] # stochastic depth decay rule
|
280 |
+
self.blocks_st = nn.ModuleList([
|
281 |
+
Block(
|
282 |
+
dim=dim_feat, num_heads=num_heads, mlp_ratio=mlp_ratio, qkv_bias=qkv_bias, qk_scale=qk_scale,
|
283 |
+
drop=drop_rate, attn_drop=attn_drop_rate, drop_path=dpr[i], norm_layer=norm_layer,
|
284 |
+
st_mode="stage_st")
|
285 |
+
for i in range(depth)])
|
286 |
+
self.blocks_ts = nn.ModuleList([
|
287 |
+
Block(
|
288 |
+
dim=dim_feat, num_heads=num_heads, mlp_ratio=mlp_ratio, qkv_bias=qkv_bias, qk_scale=qk_scale,
|
289 |
+
drop=drop_rate, attn_drop=attn_drop_rate, drop_path=dpr[i], norm_layer=norm_layer,
|
290 |
+
st_mode="stage_ts")
|
291 |
+
for i in range(depth)])
|
292 |
+
self.norm = norm_layer(dim_feat)
|
293 |
+
if dim_rep:
|
294 |
+
self.pre_logits = nn.Sequential(OrderedDict([
|
295 |
+
('fc', nn.Linear(dim_feat, dim_rep)),
|
296 |
+
('act', nn.Tanh())
|
297 |
+
]))
|
298 |
+
else:
|
299 |
+
self.pre_logits = nn.Identity()
|
300 |
+
self.head = nn.Linear(dim_rep, dim_out) if dim_out > 0 else nn.Identity()
|
301 |
+
self.temp_embed = nn.Parameter(torch.zeros(1, maxlen, 1, dim_feat))
|
302 |
+
self.pos_embed = nn.Parameter(torch.zeros(1, num_joints, dim_feat))
|
303 |
+
trunc_normal_(self.temp_embed, std=.02)
|
304 |
+
trunc_normal_(self.pos_embed, std=.02)
|
305 |
+
self.apply(self._init_weights)
|
306 |
+
self.att_fuse = att_fuse
|
307 |
+
if self.att_fuse:
|
308 |
+
self.ts_attn = nn.ModuleList([nn.Linear(dim_feat*2, 2) for i in range(depth)])
|
309 |
+
for i in range(depth):
|
310 |
+
self.ts_attn[i].weight.data.fill_(0)
|
311 |
+
self.ts_attn[i].bias.data.fill_(0.5)
|
312 |
+
|
313 |
+
def _init_weights(self, m):
|
314 |
+
if isinstance(m, nn.Linear):
|
315 |
+
trunc_normal_(m.weight, std=.02)
|
316 |
+
if isinstance(m, nn.Linear) and m.bias is not None:
|
317 |
+
nn.init.constant_(m.bias, 0)
|
318 |
+
elif isinstance(m, nn.LayerNorm):
|
319 |
+
nn.init.constant_(m.bias, 0)
|
320 |
+
nn.init.constant_(m.weight, 1.0)
|
321 |
+
|
322 |
+
def get_classifier(self):
|
323 |
+
return self.head
|
324 |
+
|
325 |
+
def reset_classifier(self, dim_out, global_pool=''):
|
326 |
+
self.dim_out = dim_out
|
327 |
+
self.head = nn.Linear(self.dim_feat, dim_out) if dim_out > 0 else nn.Identity()
|
328 |
+
|
329 |
+
def forward(self, x, return_rep=False):
|
330 |
+
B, F, J, C = x.shape
|
331 |
+
x = x.reshape(-1, J, C)
|
332 |
+
BF = x.shape[0]
|
333 |
+
x = self.joints_embed(x)
|
334 |
+
x = x + self.pos_embed
|
335 |
+
_, J, C = x.shape
|
336 |
+
x = x.reshape(-1, F, J, C) + self.temp_embed[:,:F,:,:]
|
337 |
+
x = x.reshape(BF, J, C)
|
338 |
+
x = self.pos_drop(x)
|
339 |
+
alphas = []
|
340 |
+
for idx, (blk_st, blk_ts) in enumerate(zip(self.blocks_st, self.blocks_ts)):
|
341 |
+
x_st = blk_st(x, F)
|
342 |
+
x_ts = blk_ts(x, F)
|
343 |
+
if self.att_fuse:
|
344 |
+
att = self.ts_attn[idx]
|
345 |
+
alpha = torch.cat([x_st, x_ts], dim=-1)
|
346 |
+
BF, J = alpha.shape[:2]
|
347 |
+
alpha = att(alpha)
|
348 |
+
alpha = alpha.softmax(dim=-1)
|
349 |
+
x = x_st * alpha[:,:,0:1] + x_ts * alpha[:,:,1:2]
|
350 |
+
else:
|
351 |
+
x = (x_st + x_ts)*0.5
|
352 |
+
x = self.norm(x)
|
353 |
+
x = x.reshape(B, F, J, -1)
|
354 |
+
x = self.pre_logits(x) # [B, F, J, dim_feat]
|
355 |
+
if return_rep:
|
356 |
+
return x
|
357 |
+
x = self.head(x)
|
358 |
+
return x
|
359 |
+
|
360 |
+
def get_representation(self, x):
|
361 |
+
return self.forward(x, return_rep=True)
|
362 |
+
|
lib/model/drop.py
ADDED
@@ -0,0 +1,43 @@
|
|
|
|
|
|
|
|
|
|
|
|
|
|
|
|
|
|
|
|
|
|
|
|
|
|
|
|
|
|
|
|
|
|
|
|
|
|
|
|
|
|
|
|
|
|
|
|
|
|
|
|
|
|
|
|
|
|
|
|
|
|
|
|
|
|
|
|
|
|
|
|
|
|
|
|
|
|
|
|
|
|
|
|
|
|
|
|
|
1 |
+
""" DropBlock, DropPath
|
2 |
+
PyTorch implementations of DropBlock and DropPath (Stochastic Depth) regularization layers.
|
3 |
+
Papers:
|
4 |
+
DropBlock: A regularization method for convolutional networks (https://arxiv.org/abs/1810.12890)
|
5 |
+
Deep Networks with Stochastic Depth (https://arxiv.org/abs/1603.09382)
|
6 |
+
Code:
|
7 |
+
DropBlock impl inspired by two Tensorflow impl that I liked:
|
8 |
+
- https://github.com/tensorflow/tpu/blob/master/models/official/resnet/resnet_model.py#L74
|
9 |
+
- https://github.com/clovaai/assembled-cnn/blob/master/nets/blocks.py
|
10 |
+
Hacked together by / Copyright 2020 Ross Wightman
|
11 |
+
"""
|
12 |
+
|
13 |
+
import torch
|
14 |
+
import torch.nn as nn
|
15 |
+
import torch.nn.functional as F
|
16 |
+
|
17 |
+
def drop_path(x, drop_prob: float = 0., training: bool = False):
|
18 |
+
"""Drop paths (Stochastic Depth) per sample (when applied in main path of residual blocks).
|
19 |
+
This is the same as the DropConnect impl I created for EfficientNet, etc networks, however,
|
20 |
+
the original name is misleading as 'Drop Connect' is a different form of dropout in a separate paper...
|
21 |
+
See discussion: https://github.com/tensorflow/tpu/issues/494#issuecomment-532968956 ... I've opted for
|
22 |
+
changing the layer and argument names to 'drop path' rather than mix DropConnect as a layer name and use
|
23 |
+
'survival rate' as the argument.
|
24 |
+
"""
|
25 |
+
if drop_prob == 0. or not training:
|
26 |
+
return x
|
27 |
+
keep_prob = 1 - drop_prob
|
28 |
+
shape = (x.shape[0],) + (1,) * (x.ndim - 1) # work with diff dim tensors, not just 2D ConvNets
|
29 |
+
random_tensor = keep_prob + torch.rand(shape, dtype=x.dtype, device=x.device)
|
30 |
+
random_tensor.floor_() # binarize
|
31 |
+
output = x.div(keep_prob) * random_tensor
|
32 |
+
return output
|
33 |
+
|
34 |
+
|
35 |
+
class DropPath(nn.Module):
|
36 |
+
"""Drop paths (Stochastic Depth) per sample (when applied in main path of residual blocks).
|
37 |
+
"""
|
38 |
+
def __init__(self, drop_prob=None):
|
39 |
+
super(DropPath, self).__init__()
|
40 |
+
self.drop_prob = drop_prob
|
41 |
+
|
42 |
+
def forward(self, x):
|
43 |
+
return drop_path(x, self.drop_prob, self.training)
|
lib/model/loss.py
ADDED
@@ -0,0 +1,204 @@
|
|
|
|
|
|
|
|
|
|
|
|
|
|
|
|
|
|
|
|
|
|
|
|
|
|
|
|
|
|
|
|
|
|
|
|
|
|
|
|
|
|
|
|
|
|
|
|
|
|
|
|
|
|
|
|
|
|
|
|
|
|
|
|
|
|
|
|
|
|
|
|
|
|
|
|
|
|
|
|
|
|
|
|
|
|
|
|
|
|
|
|
|
|
|
|
|
|
|
|
|
|
|
|
|
|
|
|
|
|
|
|
|
|
|
|
|
|
|
|
|
|
|
|
|
|
|
|
|
|
|
|
|
|
|
|
|
|
|
|
|
|
|
|
|
|
|
|
|
|
|
|
|
|
|
|
|
|
|
|
|
|
|
|
|
|
|
|
|
|
|
|
|
|
|
|
|
|
|
|
|
|
|
|
|
|
|
|
|
|
|
|
|
|
|
|
|
|
|
|
|
|
|
|
|
|
|
|
|
|
|
|
|
|
|
|
|
|
|
|
|
|
|
|
|
|
|
|
|
|
|
|
|
|
|
|
|
|
|
|
|
|
|
|
|
|
|
|
|
|
|
|
|
|
|
|
|
|
|
|
|
|
|
|
|
|
|
|
|
|
|
|
|
|
|
|
|
|
|
|
|
|
|
|
|
|
|
|
|
|
|
|
|
|
|
|
|
|
|
|
|
|
|
|
|
|
|
|
|
|
|
|
|
|
|
|
|
|
|
|
|
|
|
|
|
|
|
|
|
|
|
|
|
|
|
|
|
|
|
|
|
|
|
|
|
|
|
|
|
|
|
|
|
|
|
|
|
|
|
|
|
|
|
|
|
|
|
|
|
|
|
|
|
|
|
|
|
|
|
|
|
|
|
|
|
|
|
|
|
|
|
|
|
|
|
|
|
|
|
|
|
|
|
|
|
|
|
|
|
|
|
1 |
+
import torch
|
2 |
+
import torch.nn as nn
|
3 |
+
import numpy as np
|
4 |
+
import torch.nn.functional as F
|
5 |
+
|
6 |
+
# Numpy-based errors
|
7 |
+
|
8 |
+
def mpjpe(predicted, target):
|
9 |
+
"""
|
10 |
+
Mean per-joint position error (i.e. mean Euclidean distance),
|
11 |
+
often referred to as "Protocol #1" in many papers.
|
12 |
+
"""
|
13 |
+
assert predicted.shape == target.shape
|
14 |
+
return np.mean(np.linalg.norm(predicted - target, axis=len(target.shape)-1), axis=1)
|
15 |
+
|
16 |
+
def p_mpjpe(predicted, target):
|
17 |
+
"""
|
18 |
+
Pose error: MPJPE after rigid alignment (scale, rotation, and translation),
|
19 |
+
often referred to as "Protocol #2" in many papers.
|
20 |
+
"""
|
21 |
+
assert predicted.shape == target.shape
|
22 |
+
|
23 |
+
muX = np.mean(target, axis=1, keepdims=True)
|
24 |
+
muY = np.mean(predicted, axis=1, keepdims=True)
|
25 |
+
|
26 |
+
X0 = target - muX
|
27 |
+
Y0 = predicted - muY
|
28 |
+
|
29 |
+
normX = np.sqrt(np.sum(X0**2, axis=(1, 2), keepdims=True))
|
30 |
+
normY = np.sqrt(np.sum(Y0**2, axis=(1, 2), keepdims=True))
|
31 |
+
|
32 |
+
X0 /= normX
|
33 |
+
Y0 /= normY
|
34 |
+
|
35 |
+
H = np.matmul(X0.transpose(0, 2, 1), Y0)
|
36 |
+
U, s, Vt = np.linalg.svd(H)
|
37 |
+
V = Vt.transpose(0, 2, 1)
|
38 |
+
R = np.matmul(V, U.transpose(0, 2, 1))
|
39 |
+
|
40 |
+
# Avoid improper rotations (reflections), i.e. rotations with det(R) = -1
|
41 |
+
sign_detR = np.sign(np.expand_dims(np.linalg.det(R), axis=1))
|
42 |
+
V[:, :, -1] *= sign_detR
|
43 |
+
s[:, -1] *= sign_detR.flatten()
|
44 |
+
R = np.matmul(V, U.transpose(0, 2, 1)) # Rotation
|
45 |
+
tr = np.expand_dims(np.sum(s, axis=1, keepdims=True), axis=2)
|
46 |
+
a = tr * normX / normY # Scale
|
47 |
+
t = muX - a*np.matmul(muY, R) # Translation
|
48 |
+
# Perform rigid transformation on the input
|
49 |
+
predicted_aligned = a*np.matmul(predicted, R) + t
|
50 |
+
# Return MPJPE
|
51 |
+
return np.mean(np.linalg.norm(predicted_aligned - target, axis=len(target.shape)-1), axis=1)
|
52 |
+
|
53 |
+
|
54 |
+
# PyTorch-based errors (for losses)
|
55 |
+
|
56 |
+
def loss_mpjpe(predicted, target):
|
57 |
+
"""
|
58 |
+
Mean per-joint position error (i.e. mean Euclidean distance),
|
59 |
+
often referred to as "Protocol #1" in many papers.
|
60 |
+
"""
|
61 |
+
assert predicted.shape == target.shape
|
62 |
+
return torch.mean(torch.norm(predicted - target, dim=len(target.shape)-1))
|
63 |
+
|
64 |
+
def weighted_mpjpe(predicted, target, w):
|
65 |
+
"""
|
66 |
+
Weighted mean per-joint position error (i.e. mean Euclidean distance)
|
67 |
+
"""
|
68 |
+
assert predicted.shape == target.shape
|
69 |
+
assert w.shape[0] == predicted.shape[0]
|
70 |
+
return torch.mean(w * torch.norm(predicted - target, dim=len(target.shape)-1))
|
71 |
+
|
72 |
+
def loss_2d_weighted(predicted, target, conf):
|
73 |
+
assert predicted.shape == target.shape
|
74 |
+
predicted_2d = predicted[:,:,:,:2]
|
75 |
+
target_2d = target[:,:,:,:2]
|
76 |
+
diff = (predicted_2d - target_2d) * conf
|
77 |
+
return torch.mean(torch.norm(diff, dim=-1))
|
78 |
+
|
79 |
+
def n_mpjpe(predicted, target):
|
80 |
+
"""
|
81 |
+
Normalized MPJPE (scale only), adapted from:
|
82 |
+
https://github.com/hrhodin/UnsupervisedGeometryAwareRepresentationLearning/blob/master/losses/poses.py
|
83 |
+
"""
|
84 |
+
assert predicted.shape == target.shape
|
85 |
+
norm_predicted = torch.mean(torch.sum(predicted**2, dim=3, keepdim=True), dim=2, keepdim=True)
|
86 |
+
norm_target = torch.mean(torch.sum(target*predicted, dim=3, keepdim=True), dim=2, keepdim=True)
|
87 |
+
scale = norm_target / norm_predicted
|
88 |
+
return loss_mpjpe(scale * predicted, target)
|
89 |
+
|
90 |
+
def weighted_bonelen_loss(predict_3d_length, gt_3d_length):
|
91 |
+
loss_length = 0.001 * torch.pow(predict_3d_length - gt_3d_length, 2).mean()
|
92 |
+
return loss_length
|
93 |
+
|
94 |
+
def weighted_boneratio_loss(predict_3d_length, gt_3d_length):
|
95 |
+
loss_length = 0.1 * torch.pow((predict_3d_length - gt_3d_length)/gt_3d_length, 2).mean()
|
96 |
+
return loss_length
|
97 |
+
|
98 |
+
def get_limb_lens(x):
|
99 |
+
'''
|
100 |
+
Input: (N, T, 17, 3)
|
101 |
+
Output: (N, T, 16)
|
102 |
+
'''
|
103 |
+
limbs_id = [[0,1], [1,2], [2,3],
|
104 |
+
[0,4], [4,5], [5,6],
|
105 |
+
[0,7], [7,8], [8,9], [9,10],
|
106 |
+
[8,11], [11,12], [12,13],
|
107 |
+
[8,14], [14,15], [15,16]
|
108 |
+
]
|
109 |
+
limbs = x[:,:,limbs_id,:]
|
110 |
+
limbs = limbs[:,:,:,0,:]-limbs[:,:,:,1,:]
|
111 |
+
limb_lens = torch.norm(limbs, dim=-1)
|
112 |
+
return limb_lens
|
113 |
+
|
114 |
+
def loss_limb_var(x):
|
115 |
+
'''
|
116 |
+
Input: (N, T, 17, 3)
|
117 |
+
'''
|
118 |
+
if x.shape[1]<=1:
|
119 |
+
return torch.FloatTensor(1).fill_(0.)[0].to(x.device)
|
120 |
+
limb_lens = get_limb_lens(x)
|
121 |
+
limb_lens_var = torch.var(limb_lens, dim=1)
|
122 |
+
limb_loss_var = torch.mean(limb_lens_var)
|
123 |
+
return limb_loss_var
|
124 |
+
|
125 |
+
def loss_limb_gt(x, gt):
|
126 |
+
'''
|
127 |
+
Input: (N, T, 17, 3), (N, T, 17, 3)
|
128 |
+
'''
|
129 |
+
limb_lens_x = get_limb_lens(x)
|
130 |
+
limb_lens_gt = get_limb_lens(gt) # (N, T, 16)
|
131 |
+
return nn.L1Loss()(limb_lens_x, limb_lens_gt)
|
132 |
+
|
133 |
+
def loss_velocity(predicted, target):
|
134 |
+
"""
|
135 |
+
Mean per-joint velocity error (i.e. mean Euclidean distance of the 1st derivative)
|
136 |
+
"""
|
137 |
+
assert predicted.shape == target.shape
|
138 |
+
if predicted.shape[1]<=1:
|
139 |
+
return torch.FloatTensor(1).fill_(0.)[0].to(predicted.device)
|
140 |
+
velocity_predicted = predicted[:,1:] - predicted[:,:-1]
|
141 |
+
velocity_target = target[:,1:] - target[:,:-1]
|
142 |
+
return torch.mean(torch.norm(velocity_predicted - velocity_target, dim=-1))
|
143 |
+
|
144 |
+
def loss_joint(predicted, target):
|
145 |
+
assert predicted.shape == target.shape
|
146 |
+
return nn.L1Loss()(predicted, target)
|
147 |
+
|
148 |
+
def get_angles(x):
|
149 |
+
'''
|
150 |
+
Input: (N, T, 17, 3)
|
151 |
+
Output: (N, T, 16)
|
152 |
+
'''
|
153 |
+
limbs_id = [[0,1], [1,2], [2,3],
|
154 |
+
[0,4], [4,5], [5,6],
|
155 |
+
[0,7], [7,8], [8,9], [9,10],
|
156 |
+
[8,11], [11,12], [12,13],
|
157 |
+
[8,14], [14,15], [15,16]
|
158 |
+
]
|
159 |
+
angle_id = [[ 0, 3],
|
160 |
+
[ 0, 6],
|
161 |
+
[ 3, 6],
|
162 |
+
[ 0, 1],
|
163 |
+
[ 1, 2],
|
164 |
+
[ 3, 4],
|
165 |
+
[ 4, 5],
|
166 |
+
[ 6, 7],
|
167 |
+
[ 7, 10],
|
168 |
+
[ 7, 13],
|
169 |
+
[ 8, 13],
|
170 |
+
[10, 13],
|
171 |
+
[ 7, 8],
|
172 |
+
[ 8, 9],
|
173 |
+
[10, 11],
|
174 |
+
[11, 12],
|
175 |
+
[13, 14],
|
176 |
+
[14, 15] ]
|
177 |
+
eps = 1e-7
|
178 |
+
limbs = x[:,:,limbs_id,:]
|
179 |
+
limbs = limbs[:,:,:,0,:]-limbs[:,:,:,1,:]
|
180 |
+
angles = limbs[:,:,angle_id,:]
|
181 |
+
angle_cos = F.cosine_similarity(angles[:,:,:,0,:], angles[:,:,:,1,:], dim=-1)
|
182 |
+
return torch.acos(angle_cos.clamp(-1+eps, 1-eps))
|
183 |
+
|
184 |
+
def loss_angle(x, gt):
|
185 |
+
'''
|
186 |
+
Input: (N, T, 17, 3), (N, T, 17, 3)
|
187 |
+
'''
|
188 |
+
limb_angles_x = get_angles(x)
|
189 |
+
limb_angles_gt = get_angles(gt)
|
190 |
+
return nn.L1Loss()(limb_angles_x, limb_angles_gt)
|
191 |
+
|
192 |
+
def loss_angle_velocity(x, gt):
|
193 |
+
"""
|
194 |
+
Mean per-angle velocity error (i.e. mean Euclidean distance of the 1st derivative)
|
195 |
+
"""
|
196 |
+
assert x.shape == gt.shape
|
197 |
+
if x.shape[1]<=1:
|
198 |
+
return torch.FloatTensor(1).fill_(0.)[0].to(x.device)
|
199 |
+
x_a = get_angles(x)
|
200 |
+
gt_a = get_angles(gt)
|
201 |
+
x_av = x_a[:,1:] - x_a[:,:-1]
|
202 |
+
gt_av = gt_a[:,1:] - gt_a[:,:-1]
|
203 |
+
return nn.L1Loss()(x_av, gt_av)
|
204 |
+
|
lib/model/loss_mesh.py
ADDED
@@ -0,0 +1,68 @@
|
|
|
|
|
|
|
|
|
|
|
|
|
|
|
|
|
|
|
|
|
|
|
|
|
|
|
|
|
|
|
|
|
|
|
|
|
|
|
|
|
|
|
|
|
|
|
|
|
|
|
|
|
|
|
|
|
|
|
|
|
|
|
|
|
|
|
|
|
|
|
|
|
|
|
|
|
|
|
|
|
|
|
|
|
|
|
|
|
|
|
|
|
|
|
|
|
|
|
|
|
|
|
|
|
|
|
|
|
|
|
|
|
|
|
|
|
|
|
|
|
|
|
|
|
|
|
|
|
|
|
|
|
|
|
|
|
|
|
1 |
+
import torch
|
2 |
+
import torch.nn as nn
|
3 |
+
import ipdb
|
4 |
+
from lib.utils.utils_mesh import batch_rodrigues
|
5 |
+
from lib.model.loss import *
|
6 |
+
|
7 |
+
class MeshLoss(nn.Module):
|
8 |
+
def __init__(
|
9 |
+
self,
|
10 |
+
loss_type='MSE',
|
11 |
+
device='cuda',
|
12 |
+
):
|
13 |
+
super(MeshLoss, self).__init__()
|
14 |
+
self.device = device
|
15 |
+
self.loss_type = loss_type
|
16 |
+
if loss_type == 'MSE':
|
17 |
+
self.criterion_keypoints = nn.MSELoss(reduction='none').to(self.device)
|
18 |
+
self.criterion_regr = nn.MSELoss().to(self.device)
|
19 |
+
elif loss_type == 'L1':
|
20 |
+
self.criterion_keypoints = nn.L1Loss(reduction='none').to(self.device)
|
21 |
+
self.criterion_regr = nn.L1Loss().to(self.device)
|
22 |
+
|
23 |
+
def forward(
|
24 |
+
self,
|
25 |
+
smpl_output,
|
26 |
+
data_gt,
|
27 |
+
):
|
28 |
+
# to reduce time dimension
|
29 |
+
reduce = lambda x: x.reshape((x.shape[0] * x.shape[1],) + x.shape[2:])
|
30 |
+
data_3d_theta = reduce(data_gt['theta'])
|
31 |
+
|
32 |
+
preds = smpl_output[-1]
|
33 |
+
pred_theta = preds['theta']
|
34 |
+
theta_size = pred_theta.shape[:2]
|
35 |
+
pred_theta = reduce(pred_theta)
|
36 |
+
preds_local = preds['kp_3d'] - preds['kp_3d'][:, :, 0:1,:] # (N, T, 17, 3)
|
37 |
+
gt_local = data_gt['kp_3d'] - data_gt['kp_3d'][:, :, 0:1,:]
|
38 |
+
real_shape, pred_shape = data_3d_theta[:, 72:], pred_theta[:, 72:]
|
39 |
+
real_pose, pred_pose = data_3d_theta[:, :72], pred_theta[:, :72]
|
40 |
+
loss_dict = {}
|
41 |
+
loss_dict['loss_3d_pos'] = loss_mpjpe(preds_local, gt_local)
|
42 |
+
loss_dict['loss_3d_scale'] = n_mpjpe(preds_local, gt_local)
|
43 |
+
loss_dict['loss_3d_velocity'] = loss_velocity(preds_local, gt_local)
|
44 |
+
loss_dict['loss_lv'] = loss_limb_var(preds_local)
|
45 |
+
loss_dict['loss_lg'] = loss_limb_gt(preds_local, gt_local)
|
46 |
+
loss_dict['loss_a'] = loss_angle(preds_local, gt_local)
|
47 |
+
loss_dict['loss_av'] = loss_angle_velocity(preds_local, gt_local)
|
48 |
+
|
49 |
+
if pred_theta.shape[0] > 0:
|
50 |
+
loss_pose, loss_shape = self.smpl_losses(pred_pose, pred_shape, real_pose, real_shape)
|
51 |
+
loss_norm = torch.norm(pred_theta, dim=-1).mean()
|
52 |
+
loss_dict['loss_shape'] = loss_shape
|
53 |
+
loss_dict['loss_pose'] = loss_pose
|
54 |
+
loss_dict['loss_norm'] = loss_norm
|
55 |
+
return loss_dict
|
56 |
+
|
57 |
+
def smpl_losses(self, pred_rotmat, pred_betas, gt_pose, gt_betas):
|
58 |
+
pred_rotmat_valid = batch_rodrigues(pred_rotmat.reshape(-1,3)).reshape(-1, 24, 3, 3)
|
59 |
+
gt_rotmat_valid = batch_rodrigues(gt_pose.reshape(-1,3)).reshape(-1, 24, 3, 3)
|
60 |
+
pred_betas_valid = pred_betas
|
61 |
+
gt_betas_valid = gt_betas
|
62 |
+
if len(pred_rotmat_valid) > 0:
|
63 |
+
loss_regr_pose = self.criterion_regr(pred_rotmat_valid, gt_rotmat_valid)
|
64 |
+
loss_regr_betas = self.criterion_regr(pred_betas_valid, gt_betas_valid)
|
65 |
+
else:
|
66 |
+
loss_regr_pose = torch.FloatTensor(1).fill_(0.).to(self.device)
|
67 |
+
loss_regr_betas = torch.FloatTensor(1).fill_(0.).to(self.device)
|
68 |
+
return loss_regr_pose, loss_regr_betas
|
lib/model/loss_supcon.py
ADDED
@@ -0,0 +1,98 @@
|
|
|
|
|
|
|
|
|
|
|
|
|
|
|
|
|
|
|
|
|
|
|
|
|
|
|
|
|
|
|
|
|
|
|
|
|
|
|
|
|
|
|
|
|
|
|
|
|
|
|
|
|
|
|
|
|
|
|
|
|
|
|
|
|
|
|
|
|
|
|
|
|
|
|
|
|
|
|
|
|
|
|
|
|
|
|
|
|
|
|
|
|
|
|
|
|
|
|
|
|
|
|
|
|
|
|
|
|
|
|
|
|
|
|
|
|
|
|
|
|
|
|
|
|
|
|
|
|
|
|
|
|
|
|
|
|
|
|
|
|
|
|
|
|
|
|
|
|
|
|
|
|
|
|
|
|
|
|
|
|
|
|
|
|
|
|
|
|
|
|
|
|
|
|
|
|
|
|
|
|
|
|
|
|
|
|
|
|
|
|
|
|
|
|
|
|
|
|
1 |
+
"""
|
2 |
+
Author: Yonglong Tian ([email protected])
|
3 |
+
Date: May 07, 2020
|
4 |
+
"""
|
5 |
+
from __future__ import print_function
|
6 |
+
|
7 |
+
import torch
|
8 |
+
import torch.nn as nn
|
9 |
+
|
10 |
+
|
11 |
+
class SupConLoss(nn.Module):
|
12 |
+
"""Supervised Contrastive Learning: https://arxiv.org/pdf/2004.11362.pdf.
|
13 |
+
It also supports the unsupervised contrastive loss in SimCLR"""
|
14 |
+
def __init__(self, temperature=0.07, contrast_mode='all',
|
15 |
+
base_temperature=0.07):
|
16 |
+
super(SupConLoss, self).__init__()
|
17 |
+
self.temperature = temperature
|
18 |
+
self.contrast_mode = contrast_mode
|
19 |
+
self.base_temperature = base_temperature
|
20 |
+
|
21 |
+
def forward(self, features, labels=None, mask=None):
|
22 |
+
"""Compute loss for model. If both `labels` and `mask` are None,
|
23 |
+
it degenerates to SimCLR unsupervised loss:
|
24 |
+
https://arxiv.org/pdf/2002.05709.pdf
|
25 |
+
|
26 |
+
Args:
|
27 |
+
features: hidden vector of shape [bsz, n_views, ...].
|
28 |
+
labels: ground truth of shape [bsz].
|
29 |
+
mask: contrastive mask of shape [bsz, bsz], mask_{i,j}=1 if sample j
|
30 |
+
has the same class as sample i. Can be asymmetric.
|
31 |
+
Returns:
|
32 |
+
A loss scalar.
|
33 |
+
"""
|
34 |
+
device = (torch.device('cuda')
|
35 |
+
if features.is_cuda
|
36 |
+
else torch.device('cpu'))
|
37 |
+
|
38 |
+
if len(features.shape) < 3:
|
39 |
+
raise ValueError('`features` needs to be [bsz, n_views, ...],'
|
40 |
+
'at least 3 dimensions are required')
|
41 |
+
if len(features.shape) > 3:
|
42 |
+
features = features.view(features.shape[0], features.shape[1], -1)
|
43 |
+
|
44 |
+
batch_size = features.shape[0]
|
45 |
+
if labels is not None and mask is not None:
|
46 |
+
raise ValueError('Cannot define both `labels` and `mask`')
|
47 |
+
elif labels is None and mask is None:
|
48 |
+
mask = torch.eye(batch_size, dtype=torch.float32).to(device)
|
49 |
+
elif labels is not None:
|
50 |
+
labels = labels.contiguous().view(-1, 1)
|
51 |
+
if labels.shape[0] != batch_size:
|
52 |
+
raise ValueError('Num of labels does not match num of features')
|
53 |
+
mask = torch.eq(labels, labels.T).float().to(device)
|
54 |
+
else:
|
55 |
+
mask = mask.float().to(device)
|
56 |
+
|
57 |
+
contrast_count = features.shape[1]
|
58 |
+
contrast_feature = torch.cat(torch.unbind(features, dim=1), dim=0)
|
59 |
+
if self.contrast_mode == 'one':
|
60 |
+
anchor_feature = features[:, 0]
|
61 |
+
anchor_count = 1
|
62 |
+
elif self.contrast_mode == 'all':
|
63 |
+
anchor_feature = contrast_feature
|
64 |
+
anchor_count = contrast_count
|
65 |
+
else:
|
66 |
+
raise ValueError('Unknown mode: {}'.format(self.contrast_mode))
|
67 |
+
|
68 |
+
# compute logits
|
69 |
+
anchor_dot_contrast = torch.div(
|
70 |
+
torch.matmul(anchor_feature, contrast_feature.T),
|
71 |
+
self.temperature)
|
72 |
+
# for numerical stability
|
73 |
+
logits_max, _ = torch.max(anchor_dot_contrast, dim=1, keepdim=True)
|
74 |
+
logits = anchor_dot_contrast - logits_max.detach()
|
75 |
+
|
76 |
+
# tile mask
|
77 |
+
mask = mask.repeat(anchor_count, contrast_count)
|
78 |
+
# mask-out self-contrast cases
|
79 |
+
logits_mask = torch.scatter(
|
80 |
+
torch.ones_like(mask),
|
81 |
+
1,
|
82 |
+
torch.arange(batch_size * anchor_count).view(-1, 1).to(device),
|
83 |
+
0
|
84 |
+
)
|
85 |
+
mask = mask * logits_mask
|
86 |
+
|
87 |
+
# compute log_prob
|
88 |
+
exp_logits = torch.exp(logits) * logits_mask
|
89 |
+
log_prob = logits - torch.log(exp_logits.sum(1, keepdim=True))
|
90 |
+
|
91 |
+
# compute mean of log-likelihood over positive
|
92 |
+
mean_log_prob_pos = (mask * log_prob).sum(1) / mask.sum(1)
|
93 |
+
|
94 |
+
# loss
|
95 |
+
loss = - (self.temperature / self.base_temperature) * mean_log_prob_pos
|
96 |
+
loss = loss.view(anchor_count, batch_size).mean()
|
97 |
+
|
98 |
+
return loss
|
lib/model/model_action.py
ADDED
@@ -0,0 +1,71 @@
|
|
|
|
|
|
|
|
|
|
|
|
|
|
|
|
|
|
|
|
|
|
|
|
|
|
|
|
|
|
|
|
|
|
|
|
|
|
|
|
|
|
|
|
|
|
|
|
|
|
|
|
|
|
|
|
|
|
|
|
|
|
|
|
|
|
|
|
|
|
|
|
|
|
|
|
|
|
|
|
|
|
|
|
|
|
|
|
|
|
|
|
|
|
|
|
|
|
|
|
|
|
|
|
|
|
|
|
|
|
|
|
|
|
|
|
|
|
|
|
|
|
|
|
|
|
|
|
|
|
|
|
|
|
|
|
|
|
|
|
|
|
|
|
|
1 |
+
import sys
|
2 |
+
import torch
|
3 |
+
import torch.nn as nn
|
4 |
+
import torch.nn.functional as F
|
5 |
+
|
6 |
+
class ActionHeadClassification(nn.Module):
|
7 |
+
def __init__(self, dropout_ratio=0., dim_rep=512, num_classes=60, num_joints=17, hidden_dim=2048):
|
8 |
+
super(ActionHeadClassification, self).__init__()
|
9 |
+
self.dropout = nn.Dropout(p=dropout_ratio)
|
10 |
+
self.bn = nn.BatchNorm1d(hidden_dim, momentum=0.1)
|
11 |
+
self.relu = nn.ReLU(inplace=True)
|
12 |
+
self.fc1 = nn.Linear(dim_rep*num_joints, hidden_dim)
|
13 |
+
self.fc2 = nn.Linear(hidden_dim, num_classes)
|
14 |
+
|
15 |
+
def forward(self, feat):
|
16 |
+
'''
|
17 |
+
Input: (N, M, T, J, C)
|
18 |
+
'''
|
19 |
+
N, M, T, J, C = feat.shape
|
20 |
+
feat = self.dropout(feat)
|
21 |
+
feat = feat.permute(0, 1, 3, 4, 2) # (N, M, T, J, C) -> (N, M, J, C, T)
|
22 |
+
feat = feat.mean(dim=-1)
|
23 |
+
feat = feat.reshape(N, M, -1) # (N, M, J*C)
|
24 |
+
feat = feat.mean(dim=1)
|
25 |
+
feat = self.fc1(feat)
|
26 |
+
feat = self.bn(feat)
|
27 |
+
feat = self.relu(feat)
|
28 |
+
feat = self.fc2(feat)
|
29 |
+
return feat
|
30 |
+
|
31 |
+
class ActionHeadEmbed(nn.Module):
|
32 |
+
def __init__(self, dropout_ratio=0., dim_rep=512, num_joints=17, hidden_dim=2048):
|
33 |
+
super(ActionHeadEmbed, self).__init__()
|
34 |
+
self.dropout = nn.Dropout(p=dropout_ratio)
|
35 |
+
self.fc1 = nn.Linear(dim_rep*num_joints, hidden_dim)
|
36 |
+
def forward(self, feat):
|
37 |
+
'''
|
38 |
+
Input: (N, M, T, J, C)
|
39 |
+
'''
|
40 |
+
N, M, T, J, C = feat.shape
|
41 |
+
feat = self.dropout(feat)
|
42 |
+
feat = feat.permute(0, 1, 3, 4, 2) # (N, M, T, J, C) -> (N, M, J, C, T)
|
43 |
+
feat = feat.mean(dim=-1)
|
44 |
+
feat = feat.reshape(N, M, -1) # (N, M, J*C)
|
45 |
+
feat = feat.mean(dim=1)
|
46 |
+
feat = self.fc1(feat)
|
47 |
+
feat = F.normalize(feat, dim=-1)
|
48 |
+
return feat
|
49 |
+
|
50 |
+
class ActionNet(nn.Module):
|
51 |
+
def __init__(self, backbone, dim_rep=512, num_classes=60, dropout_ratio=0., version='class', hidden_dim=2048, num_joints=17):
|
52 |
+
super(ActionNet, self).__init__()
|
53 |
+
self.backbone = backbone
|
54 |
+
self.feat_J = num_joints
|
55 |
+
if version=='class':
|
56 |
+
self.head = ActionHeadClassification(dropout_ratio=dropout_ratio, dim_rep=dim_rep, num_classes=num_classes, num_joints=num_joints)
|
57 |
+
elif version=='embed':
|
58 |
+
self.head = ActionHeadEmbed(dropout_ratio=dropout_ratio, dim_rep=dim_rep, hidden_dim=hidden_dim, num_joints=num_joints)
|
59 |
+
else:
|
60 |
+
raise Exception('Version Error.')
|
61 |
+
|
62 |
+
def forward(self, x):
|
63 |
+
'''
|
64 |
+
Input: (N, M x T x 17 x 3)
|
65 |
+
'''
|
66 |
+
N, M, T, J, C = x.shape
|
67 |
+
x = x.reshape(N*M, T, J, C)
|
68 |
+
feat = self.backbone.get_representation(x)
|
69 |
+
feat = feat.reshape([N, M, T, self.feat_J, -1]) # (N, M, T, J, C)
|
70 |
+
out = self.head(feat)
|
71 |
+
return out
|
lib/model/model_mesh.py
ADDED
@@ -0,0 +1,101 @@
|
|
|
|
|
|
|
|
|
|
|
|
|
|
|
|
|
|
|
|
|
|
|
|
|
|
|
|
|
|
|
|
|
|
|
|
|
|
|
|
|
|
|
|
|
|
|
|
|
|
|
|
|
|
|
|
|
|
|
|
|
|
|
|
|
|
|
|
|
|
|
|
|
|
|
|
|
|
|
|
|
|
|
|
|
|
|
|
|
|
|
|
|
|
|
|
|
|
|
|
|
|
|
|
|
|
|
|
|
|
|
|
|
|
|
|
|
|
|
|
|
|
|
|
|
|
|
|
|
|
|
|
|
|
|
|
|
|
|
|
|
|
|
|
|
|
|
|
|
|
|
|
|
|
|
|
|
|
|
|
|
|
|
|
|
|
|
|
|
|
|
|
|
|
|
|
|
|
|
|
|
|
|
|
|
|
|
|
|
|
|
|
|
|
|
|
|
|
|
|
|
|
|
|
|
1 |
+
import sys
|
2 |
+
import torch
|
3 |
+
import torch.nn as nn
|
4 |
+
import torch.nn.functional as F
|
5 |
+
import numpy as np
|
6 |
+
from lib.utils.utils_smpl import SMPL
|
7 |
+
from lib.utils.utils_mesh import rotation_matrix_to_angle_axis, rot6d_to_rotmat
|
8 |
+
|
9 |
+
class SMPLRegressor(nn.Module):
|
10 |
+
def __init__(self, args, dim_rep=512, num_joints=17, hidden_dim=2048, dropout_ratio=0.):
|
11 |
+
super(SMPLRegressor, self).__init__()
|
12 |
+
param_pose_dim = 24 * 6
|
13 |
+
self.dropout = nn.Dropout(p=dropout_ratio)
|
14 |
+
self.fc1 = nn.Linear(num_joints*dim_rep, hidden_dim)
|
15 |
+
self.pool2 = nn.AdaptiveAvgPool2d((None, 1))
|
16 |
+
self.fc2 = nn.Linear(num_joints*dim_rep, hidden_dim)
|
17 |
+
self.bn1 = nn.BatchNorm1d(hidden_dim, momentum=0.1)
|
18 |
+
self.bn2 = nn.BatchNorm1d(hidden_dim, momentum=0.1)
|
19 |
+
self.relu1 = nn.ReLU(inplace=True)
|
20 |
+
self.relu2 = nn.ReLU(inplace=True)
|
21 |
+
self.head_pose = nn.Linear(hidden_dim, param_pose_dim)
|
22 |
+
self.head_shape = nn.Linear(hidden_dim, 10)
|
23 |
+
nn.init.xavier_uniform_(self.head_pose.weight, gain=0.01)
|
24 |
+
nn.init.xavier_uniform_(self.head_shape.weight, gain=0.01)
|
25 |
+
self.smpl = SMPL(
|
26 |
+
args.data_root,
|
27 |
+
batch_size=64,
|
28 |
+
create_transl=False,
|
29 |
+
)
|
30 |
+
mean_params = np.load(self.smpl.smpl_mean_params)
|
31 |
+
init_pose = torch.from_numpy(mean_params['pose'][:]).unsqueeze(0)
|
32 |
+
init_shape = torch.from_numpy(mean_params['shape'][:].astype('float32')).unsqueeze(0)
|
33 |
+
self.register_buffer('init_pose', init_pose)
|
34 |
+
self.register_buffer('init_shape', init_shape)
|
35 |
+
self.J_regressor = self.smpl.J_regressor_h36m
|
36 |
+
|
37 |
+
def forward(self, feat, init_pose=None, init_shape=None):
|
38 |
+
N, T, J, C = feat.shape
|
39 |
+
NT = N * T
|
40 |
+
feat = feat.reshape(N, T, -1)
|
41 |
+
|
42 |
+
feat_pose = feat.reshape(NT, -1) # (N*T, J*C)
|
43 |
+
|
44 |
+
feat_pose = self.dropout(feat_pose)
|
45 |
+
feat_pose = self.fc1(feat_pose)
|
46 |
+
feat_pose = self.bn1(feat_pose)
|
47 |
+
feat_pose = self.relu1(feat_pose) # (NT, C)
|
48 |
+
|
49 |
+
feat_shape = feat.permute(0,2,1) # (N, T, J*C) -> (N, J*C, T)
|
50 |
+
feat_shape = self.pool2(feat_shape).reshape(N, -1) # (N, J*C)
|
51 |
+
|
52 |
+
feat_shape = self.dropout(feat_shape)
|
53 |
+
feat_shape = self.fc2(feat_shape)
|
54 |
+
feat_shape = self.bn2(feat_shape)
|
55 |
+
feat_shape = self.relu2(feat_shape) # (N, C)
|
56 |
+
|
57 |
+
pred_pose = self.init_pose.expand(NT, -1) # (NT, C)
|
58 |
+
pred_shape = self.init_shape.expand(N, -1) # (N, C)
|
59 |
+
|
60 |
+
pred_pose = self.head_pose(feat_pose) + pred_pose
|
61 |
+
pred_shape = self.head_shape(feat_shape) + pred_shape
|
62 |
+
pred_shape = pred_shape.expand(T, N, -1).permute(1, 0, 2).reshape(NT, -1)
|
63 |
+
pred_rotmat = rot6d_to_rotmat(pred_pose).view(-1, 24, 3, 3)
|
64 |
+
pred_output = self.smpl(
|
65 |
+
betas=pred_shape,
|
66 |
+
body_pose=pred_rotmat[:, 1:],
|
67 |
+
global_orient=pred_rotmat[:, 0].unsqueeze(1),
|
68 |
+
pose2rot=False
|
69 |
+
)
|
70 |
+
pred_vertices = pred_output.vertices*1000.0
|
71 |
+
assert self.J_regressor is not None
|
72 |
+
J_regressor_batch = self.J_regressor[None, :].expand(pred_vertices.shape[0], -1, -1).to(pred_vertices.device)
|
73 |
+
pred_joints = torch.matmul(J_regressor_batch, pred_vertices)
|
74 |
+
pose = rotation_matrix_to_angle_axis(pred_rotmat.reshape(-1, 3, 3)).reshape(-1, 72)
|
75 |
+
output = [{
|
76 |
+
'theta' : torch.cat([pose, pred_shape], dim=1), # (N*T, 72+10)
|
77 |
+
'verts' : pred_vertices, # (N*T, 6890, 3)
|
78 |
+
'kp_3d' : pred_joints, # (N*T, 17, 3)
|
79 |
+
}]
|
80 |
+
return output
|
81 |
+
|
82 |
+
class MeshRegressor(nn.Module):
|
83 |
+
def __init__(self, args, backbone, dim_rep=512, num_joints=17, hidden_dim=2048, dropout_ratio=0.5):
|
84 |
+
super(MeshRegressor, self).__init__()
|
85 |
+
self.backbone = backbone
|
86 |
+
self.feat_J = num_joints
|
87 |
+
self.head = SMPLRegressor(args, dim_rep, num_joints, hidden_dim, dropout_ratio)
|
88 |
+
|
89 |
+
def forward(self, x, init_pose=None, init_shape=None, n_iter=3):
|
90 |
+
'''
|
91 |
+
Input: (N x T x 17 x 3)
|
92 |
+
'''
|
93 |
+
N, T, J, C = x.shape
|
94 |
+
feat = self.backbone.get_representation(x)
|
95 |
+
feat = feat.reshape([N, T, self.feat_J, -1]) # (N, T, J, C)
|
96 |
+
smpl_output = self.head(feat)
|
97 |
+
for s in smpl_output:
|
98 |
+
s['theta'] = s['theta'].reshape(N, T, -1)
|
99 |
+
s['verts'] = s['verts'].reshape(N, T, -1, 3)
|
100 |
+
s['kp_3d'] = s['kp_3d'].reshape(N, T, -1, 3)
|
101 |
+
return smpl_output
|
lib/utils/learning.py
ADDED
@@ -0,0 +1,102 @@
|
|
|
|
|
|
|
|
|
|
|
|
|
|
|
|
|
|
|
|
|
|
|
|
|
|
|
|
|
|
|
|
|
|
|
|
|
|
|
|
|
|
|
|
|
|
|
|
|
|
|
|
|
|
|
|
|
|
|
|
|
|
|
|
|
|
|
|
|
|
|
|
|
|
|
|
|
|
|
|
|
|
|
|
|
|
|
|
|
|
|
|
|
|
|
|
|
|
|
|
|
|
|
|
|
|
|
|
|
|
|
|
|
|
|
|
|
|
|
|
|
|
|
|
|
|
|
|
|
|
|
|
|
|
|
|
|
|
|
|
|
|
|
|
|
|
|
|
|
|
|
|
|
|
|
|
|
|
|
|
|
|
|
|
|
|
|
|
|
|
|
|
|
|
|
|
|
|
|
|
|
|
|
|
|
|
|
|
|
|
|
|
|
|
|
|
|
|
|
|
|
|
|
|
|
|
|
1 |
+
import os
|
2 |
+
import numpy as np
|
3 |
+
import torch
|
4 |
+
import torch.nn as nn
|
5 |
+
from functools import partial
|
6 |
+
from lib.model.DSTformer import DSTformer
|
7 |
+
|
8 |
+
class AverageMeter(object):
|
9 |
+
"""Computes and stores the average and current value"""
|
10 |
+
def __init__(self):
|
11 |
+
self.reset()
|
12 |
+
|
13 |
+
def reset(self):
|
14 |
+
self.val = 0
|
15 |
+
self.avg = 0
|
16 |
+
self.sum = 0
|
17 |
+
self.count = 0
|
18 |
+
|
19 |
+
def update(self, val, n=1):
|
20 |
+
self.val = val
|
21 |
+
self.sum += val * n
|
22 |
+
self.count += n
|
23 |
+
self.avg = self.sum / self.count
|
24 |
+
|
25 |
+
def accuracy(output, target, topk=(1,)):
|
26 |
+
"""Computes the accuracy over the k top predictions for the specified values of k"""
|
27 |
+
with torch.no_grad():
|
28 |
+
maxk = max(topk)
|
29 |
+
batch_size = target.size(0)
|
30 |
+
_, pred = output.topk(maxk, 1, True, True)
|
31 |
+
pred = pred.t()
|
32 |
+
correct = pred.eq(target.view(1, -1).expand_as(pred))
|
33 |
+
res = []
|
34 |
+
for k in topk:
|
35 |
+
correct_k = correct[:k].reshape(-1).float().sum(0, keepdim=True)
|
36 |
+
res.append(correct_k.mul_(100.0 / batch_size))
|
37 |
+
return res
|
38 |
+
|
39 |
+
def load_pretrained_weights(model, checkpoint):
|
40 |
+
"""Load pretrianed weights to model
|
41 |
+
Incompatible layers (unmatched in name or size) will be ignored
|
42 |
+
Args:
|
43 |
+
- model (nn.Module): network model, which must not be nn.DataParallel
|
44 |
+
- weight_path (str): path to pretrained weights
|
45 |
+
"""
|
46 |
+
import collections
|
47 |
+
if 'state_dict' in checkpoint:
|
48 |
+
state_dict = checkpoint['state_dict']
|
49 |
+
else:
|
50 |
+
state_dict = checkpoint
|
51 |
+
model_dict = model.state_dict()
|
52 |
+
new_state_dict = collections.OrderedDict()
|
53 |
+
matched_layers, discarded_layers = [], []
|
54 |
+
for k, v in state_dict.items():
|
55 |
+
# If the pretrained state_dict was saved as nn.DataParallel,
|
56 |
+
# keys would contain "module.", which should be ignored.
|
57 |
+
if k.startswith('module.'):
|
58 |
+
k = k[7:]
|
59 |
+
if k in model_dict and model_dict[k].size() == v.size():
|
60 |
+
new_state_dict[k] = v
|
61 |
+
matched_layers.append(k)
|
62 |
+
else:
|
63 |
+
discarded_layers.append(k)
|
64 |
+
model_dict.update(new_state_dict)
|
65 |
+
model.load_state_dict(model_dict, strict=True)
|
66 |
+
print('load_weight', len(matched_layers))
|
67 |
+
return model
|
68 |
+
|
69 |
+
def partial_train_layers(model, partial_list):
|
70 |
+
"""Train partial layers of a given model."""
|
71 |
+
for name, p in model.named_parameters():
|
72 |
+
p.requires_grad = False
|
73 |
+
for trainable in partial_list:
|
74 |
+
if trainable in name:
|
75 |
+
p.requires_grad = True
|
76 |
+
break
|
77 |
+
return model
|
78 |
+
|
79 |
+
def load_backbone(args):
|
80 |
+
if not(hasattr(args, "backbone")):
|
81 |
+
args.backbone = 'DSTformer' # Default
|
82 |
+
if args.backbone=='DSTformer':
|
83 |
+
model_backbone = DSTformer(dim_in=3, dim_out=3, dim_feat=args.dim_feat, dim_rep=args.dim_rep,
|
84 |
+
depth=args.depth, num_heads=args.num_heads, mlp_ratio=args.mlp_ratio, norm_layer=partial(nn.LayerNorm, eps=1e-6),
|
85 |
+
maxlen=args.maxlen, num_joints=args.num_joints)
|
86 |
+
elif args.backbone=='TCN':
|
87 |
+
from lib.model.model_tcn import PoseTCN
|
88 |
+
model_backbone = PoseTCN()
|
89 |
+
elif args.backbone=='poseformer':
|
90 |
+
from lib.model.model_poseformer import PoseTransformer
|
91 |
+
model_backbone = PoseTransformer(num_frame=args.maxlen, num_joints=args.num_joints, in_chans=3, embed_dim_ratio=32, depth=4,
|
92 |
+
num_heads=8, mlp_ratio=2., qkv_bias=True, qk_scale=None,drop_path_rate=0, attn_mask=None)
|
93 |
+
elif args.backbone=='mixste':
|
94 |
+
from lib.model.model_mixste import MixSTE2
|
95 |
+
model_backbone = MixSTE2(num_frame=args.maxlen, num_joints=args.num_joints, in_chans=3, embed_dim_ratio=512, depth=8,
|
96 |
+
num_heads=8, mlp_ratio=2., qkv_bias=True, qk_scale=None,drop_path_rate=0)
|
97 |
+
elif args.backbone=='stgcn':
|
98 |
+
from lib.model.model_stgcn import Model as STGCN
|
99 |
+
model_backbone = STGCN()
|
100 |
+
else:
|
101 |
+
raise Exception("Undefined backbone type.")
|
102 |
+
return model_backbone
|
lib/utils/tools.py
ADDED
@@ -0,0 +1,69 @@
|
|
|
|
|
|
|
|
|
|
|
|
|
|
|
|
|
|
|
|
|
|
|
|
|
|
|
|
|
|
|
|
|
|
|
|
|
|
|
|
|
|
|
|
|
|
|
|
|
|
|
|
|
|
|
|
|
|
|
|
|
|
|
|
|
|
|
|
|
|
|
|
|
|
|
|
|
|
|
|
|
|
|
|
|
|
|
|
|
|
|
|
|
|
|
|
|
|
|
|
|
|
|
|
|
|
|
|
|
|
|
|
|
|
|
|
|
|
|
|
|
|
|
|
|
|
|
|
|
|
|
|
|
|
|
|
|
|
|
|
|
1 |
+
import numpy as np
|
2 |
+
import os, sys
|
3 |
+
import pickle
|
4 |
+
import yaml
|
5 |
+
from easydict import EasyDict as edict
|
6 |
+
from typing import Any, IO
|
7 |
+
|
8 |
+
ROOT_PATH = os.path.join(os.path.dirname(os.path.realpath(__file__)), '..')
|
9 |
+
|
10 |
+
class TextLogger:
|
11 |
+
def __init__(self, log_path):
|
12 |
+
self.log_path = log_path
|
13 |
+
with open(self.log_path, "w") as f:
|
14 |
+
f.write("")
|
15 |
+
def log(self, log):
|
16 |
+
with open(self.log_path, "a+") as f:
|
17 |
+
f.write(log + "\n")
|
18 |
+
|
19 |
+
class Loader(yaml.SafeLoader):
|
20 |
+
"""YAML Loader with `!include` constructor."""
|
21 |
+
|
22 |
+
def __init__(self, stream: IO) -> None:
|
23 |
+
"""Initialise Loader."""
|
24 |
+
|
25 |
+
try:
|
26 |
+
self._root = os.path.split(stream.name)[0]
|
27 |
+
except AttributeError:
|
28 |
+
self._root = os.path.curdir
|
29 |
+
|
30 |
+
super().__init__(stream)
|
31 |
+
|
32 |
+
def construct_include(loader: Loader, node: yaml.Node) -> Any:
|
33 |
+
"""Include file referenced at node."""
|
34 |
+
|
35 |
+
filename = os.path.abspath(os.path.join(loader._root, loader.construct_scalar(node)))
|
36 |
+
extension = os.path.splitext(filename)[1].lstrip('.')
|
37 |
+
|
38 |
+
with open(filename, 'r') as f:
|
39 |
+
if extension in ('yaml', 'yml'):
|
40 |
+
return yaml.load(f, Loader)
|
41 |
+
elif extension in ('json', ):
|
42 |
+
return json.load(f)
|
43 |
+
else:
|
44 |
+
return ''.join(f.readlines())
|
45 |
+
|
46 |
+
def get_config(config_path):
|
47 |
+
yaml.add_constructor('!include', construct_include, Loader)
|
48 |
+
with open(config_path, 'r') as stream:
|
49 |
+
config = yaml.load(stream, Loader=Loader)
|
50 |
+
config = edict(config)
|
51 |
+
_, config_filename = os.path.split(config_path)
|
52 |
+
config_name, _ = os.path.splitext(config_filename)
|
53 |
+
config.name = config_name
|
54 |
+
return config
|
55 |
+
|
56 |
+
def ensure_dir(path):
|
57 |
+
"""
|
58 |
+
create path by first checking its existence,
|
59 |
+
:param paths: path
|
60 |
+
:return:
|
61 |
+
"""
|
62 |
+
if not os.path.exists(path):
|
63 |
+
os.makedirs(path)
|
64 |
+
|
65 |
+
def read_pkl(data_url):
|
66 |
+
file = open(data_url,'rb')
|
67 |
+
content = pickle.load(file)
|
68 |
+
file.close()
|
69 |
+
return content
|
lib/utils/utils_data.py
ADDED
@@ -0,0 +1,112 @@
|
|
|
|
|
|
|
|
|
|
|
|
|
|
|
|
|
|
|
|
|
|
|
|
|
|
|
|
|
|
|
|
|
|
|
|
|
|
|
|
|
|
|
|
|
|
|
|
|
|
|
|
|
|
|
|
|
|
|
|
|
|
|
|
|
|
|
|
|
|
|
|
|
|
|
|
|
|
|
|
|
|
|
|
|
|
|
|
|
|
|
|
|
|
|
|
|
|
|
|
|
|
|
|
|
|
|
|
|
|
|
|
|
|
|
|
|
|
|
|
|
|
|
|
|
|
|
|
|
|
|
|
|
|
|
|
|
|
|
|
|
|
|
|
|
|
|
|
|
|
|
|
|
|
|
|
|
|
|
|
|
|
|
|
|
|
|
|
|
|
|
|
|
|
|
|
|
|
|
|
|
|
|
|
|
|
|
|
|
|
|
|
|
|
|
|
|
|
|
|
|
|
|
|
|
|
|
|
|
|
|
|
|
|
|
|
|
|
|
|
|
|
|
|
|
|
|
1 |
+
import os
|
2 |
+
import torch
|
3 |
+
import torch.nn.functional as F
|
4 |
+
import numpy as np
|
5 |
+
import copy
|
6 |
+
|
7 |
+
def crop_scale(motion, scale_range=[1, 1]):
|
8 |
+
'''
|
9 |
+
Motion: [(M), T, 17, 3].
|
10 |
+
Normalize to [-1, 1]
|
11 |
+
'''
|
12 |
+
result = copy.deepcopy(motion)
|
13 |
+
valid_coords = motion[motion[..., 2]!=0][:,:2]
|
14 |
+
if len(valid_coords) < 4:
|
15 |
+
return np.zeros(motion.shape)
|
16 |
+
xmin = min(valid_coords[:,0])
|
17 |
+
xmax = max(valid_coords[:,0])
|
18 |
+
ymin = min(valid_coords[:,1])
|
19 |
+
ymax = max(valid_coords[:,1])
|
20 |
+
ratio = np.random.uniform(low=scale_range[0], high=scale_range[1], size=1)[0]
|
21 |
+
scale = max(xmax-xmin, ymax-ymin) * ratio
|
22 |
+
if scale==0:
|
23 |
+
return np.zeros(motion.shape)
|
24 |
+
xs = (xmin+xmax-scale) / 2
|
25 |
+
ys = (ymin+ymax-scale) / 2
|
26 |
+
result[...,:2] = (motion[..., :2]- [xs,ys]) / scale
|
27 |
+
result[...,:2] = (result[..., :2] - 0.5) * 2
|
28 |
+
result = np.clip(result, -1, 1)
|
29 |
+
return result
|
30 |
+
|
31 |
+
def crop_scale_3d(motion, scale_range=[1, 1]):
|
32 |
+
'''
|
33 |
+
Motion: [T, 17, 3]. (x, y, z)
|
34 |
+
Normalize to [-1, 1]
|
35 |
+
Z is relative to the first frame's root.
|
36 |
+
'''
|
37 |
+
result = copy.deepcopy(motion)
|
38 |
+
result[:,:,2] = result[:,:,2] - result[0,0,2]
|
39 |
+
xmin = np.min(motion[...,0])
|
40 |
+
xmax = np.max(motion[...,0])
|
41 |
+
ymin = np.min(motion[...,1])
|
42 |
+
ymax = np.max(motion[...,1])
|
43 |
+
ratio = np.random.uniform(low=scale_range[0], high=scale_range[1], size=1)[0]
|
44 |
+
scale = max(xmax-xmin, ymax-ymin) / ratio
|
45 |
+
if scale==0:
|
46 |
+
return np.zeros(motion.shape)
|
47 |
+
xs = (xmin+xmax-scale) / 2
|
48 |
+
ys = (ymin+ymax-scale) / 2
|
49 |
+
result[...,:2] = (motion[..., :2]- [xs,ys]) / scale
|
50 |
+
result[...,2] = result[...,2] / scale
|
51 |
+
result = (result - 0.5) * 2
|
52 |
+
return result
|
53 |
+
|
54 |
+
def flip_data(data):
|
55 |
+
"""
|
56 |
+
horizontal flip
|
57 |
+
data: [N, F, 17, D] or [F, 17, D]. X (horizontal coordinate) is the first channel in D.
|
58 |
+
Return
|
59 |
+
result: same
|
60 |
+
"""
|
61 |
+
left_joints = [4, 5, 6, 11, 12, 13]
|
62 |
+
right_joints = [1, 2, 3, 14, 15, 16]
|
63 |
+
flipped_data = copy.deepcopy(data)
|
64 |
+
flipped_data[..., 0] *= -1 # flip x of all joints
|
65 |
+
flipped_data[..., left_joints+right_joints, :] = flipped_data[..., right_joints+left_joints, :]
|
66 |
+
return flipped_data
|
67 |
+
|
68 |
+
def resample(ori_len, target_len, replay=False, randomness=True):
|
69 |
+
if replay:
|
70 |
+
if ori_len > target_len:
|
71 |
+
st = np.random.randint(ori_len-target_len)
|
72 |
+
return range(st, st+target_len) # Random clipping from sequence
|
73 |
+
else:
|
74 |
+
return np.array(range(target_len)) % ori_len # Replay padding
|
75 |
+
else:
|
76 |
+
if randomness:
|
77 |
+
even = np.linspace(0, ori_len, num=target_len, endpoint=False)
|
78 |
+
if ori_len < target_len:
|
79 |
+
low = np.floor(even)
|
80 |
+
high = np.ceil(even)
|
81 |
+
sel = np.random.randint(2, size=even.shape)
|
82 |
+
result = np.sort(sel*low+(1-sel)*high)
|
83 |
+
else:
|
84 |
+
interval = even[1] - even[0]
|
85 |
+
result = np.random.random(even.shape)*interval + even
|
86 |
+
result = np.clip(result, a_min=0, a_max=ori_len-1).astype(np.uint32)
|
87 |
+
else:
|
88 |
+
result = np.linspace(0, ori_len, num=target_len, endpoint=False, dtype=int)
|
89 |
+
return result
|
90 |
+
|
91 |
+
def split_clips(vid_list, n_frames, data_stride):
|
92 |
+
result = []
|
93 |
+
n_clips = 0
|
94 |
+
st = 0
|
95 |
+
i = 0
|
96 |
+
saved = set()
|
97 |
+
while i<len(vid_list):
|
98 |
+
i += 1
|
99 |
+
if i-st == n_frames:
|
100 |
+
result.append(range(st,i))
|
101 |
+
saved.add(vid_list[i-1])
|
102 |
+
st = st + data_stride
|
103 |
+
n_clips += 1
|
104 |
+
if i==len(vid_list):
|
105 |
+
break
|
106 |
+
if vid_list[i]!=vid_list[i-1]:
|
107 |
+
if not (vid_list[i-1] in saved):
|
108 |
+
resampled = resample(i-st, n_frames) + st
|
109 |
+
result.append(resampled)
|
110 |
+
saved.add(vid_list[i-1])
|
111 |
+
st = i
|
112 |
+
return result
|
lib/utils/utils_mesh.py
ADDED
@@ -0,0 +1,521 @@
|
|
|
|
|
|
|
|
|
|
|
|
|
|
|
|
|
|
|
|
|
|
|
|
|
|
|
|
|
|
|
|
|
|
|
|
|
|
|
|
|
|
|
|
|
|
|
|
|
|
|
|
|
|
|
|
|
|
|
|
|
|
|
|
|
|
|
|
|
|
|
|
|
|
|
|
|
|
|
|
|
|
|
|
|
|
|
|
|
|
|
|
|
|
|
|
|
|
|
|
|
|
|
|
|
|
|
|
|
|
|
|
|
|
|
|
|
|
|
|
|
|
|
|
|
|
|
|
|
|
|
|
|
|
|
|
|
|
|
|
|
|
|
|
|
|
|
|
|
|
|
|
|
|
|
|
|
|
|
|
|
|
|
|
|
|
|
|
|
|
|
|
|
|
|
|
|
|
|
|
|
|
|
|
|
|
|
|
|
|
|
|
|
|
|
|
|
|
|
|
|
|
|
|
|
|
|
|
|
|
|
|
|
|
|
|
|
|
|
|
|
|
|
|
|
|
|
|
|
|
|
|
|
|
|
|
|
|
|
|
|
|
|
|
|
|
|
|
|
|
|
|
|
|
|
|
|
|
|
|
|
|
|
|
|
|
|
|
|
|
|
|
|
|
|
|
|
|
|
|
|
|
|
|
|
|
|
|
|
|
|
|
|
|
|
|
|
|
|
|
|
|
|
|
|
|
|
|
|
|
|
|
|
|
|
|
|
|
|
|
|
|
|
|
|
|
|
|
|
|
|
|
|
|
|
|
|
|
|
|
|
|
|
|
|
|
|
|
|
|
|
|
|
|
|
|
|
|
|
|
|
|
|
|
|
|
|
|
|
|
|
|
|
|
|
|
|
|
|
|
|
|
|
|
|
|
|
|
|
|
|
|
|
|
|
|
|
|
|
|
|
|
|
|
|
|
|
|
|
|
|
|
|
|
|
|
|
|
|
|
|
|
|
|
|
|
|
|
|
|
|
|
|
|
|
|
|
|
|
|
|
|
|
|
|
|
|
|
|
|
|
|
|
|
|
|
|
|
|
|
|
|
|
|
|
|
|
|
|
|
|
|
|
|
|
|
|
|
|
|
|
|
|
|
|
|
|
|
|
|
|
|
|
|
|
|
|
|
|
|
|
|
|
|
|
|
|
|
|
|
|
|
|
|
|
|
|
|
|
|
|
|
|
|
|
|
|
|
|
|
|
|
|
|
|
|
|
|
|
|
|
|
|
|
|
|
|
|
|
|
|
|
|
|
|
|
|
|
|
|
|
|
|
|
|
|
|
|
|
|
|
|
|
|
|
|
|
|
|
|
|
|
|
|
|
|
|
|
|
|
|
|
|
|
|
|
|
|
|
|
|
|
|
|
|
|
|
|
|
|
|
|
|
|
|
|
|
|
|
|
|
|
|
|
|
|
|
|
|
|
|
|
|
|
|
|
|
|
|
|
|
|
|
|
|
|
|
|
|
|
|
|
|
|
|
|
|
|
|
|
|
|
|
|
|
|
|
|
|
|
|
|
|
|
|
|
|
|
|
|
|
|
|
|
|
|
|
|
|
|
|
|
|
|
|
|
|
|
|
|
|
|
|
|
|
|
|
|
|
|
|
|
|
|
|
|
|
|
|
|
|
|
|
|
|
|
|
|
|
|
|
|
|
|
|
|
|
|
|
|
|
|
|
|
|
|
|
|
|
|
|
|
|
|
|
|
|
|
|
|
|
|
|
|
|
|
|
|
|
|
|
|
|
|
|
|
|
|
|
|
|
|
|
|
|
|
|
|
|
|
|
|
|
|
|
|
|
|
|
|
|
|
|
|
|
|
|
|
|
|
|
|
|
|
|
|
|
|
|
|
|
|
|
|
|
|
|
|
|
|
|
|
|
|
|
|
|
|
|
|
|
|
|
|
|
|
|
|
|
|
|
|
|
|
|
|
|
|
|
|
|
|
|
|
|
|
|
|
|
|
|
|
|
|
|
|
|
|
|
|
|
|
|
|
|
|
|
|
|
|
|
|
|
|
|
|
|
|
|
|
|
|
|
|
|
|
|
|
|
|
|
|
|
|
|
|
|
|
|
|
|
|
|
|
|
|
|
|
|
|
|
|
|
|
|
|
|
|
|
|
|
|
|
|
|
|
|
|
|
|
|
|
|
|
|
|
|
|
|
|
|
|
|
|
|
|
|
|
|
|
|
|
|
|
|
|
|
|
|
|
|
|
|
|
|
|
|
|
|
|
|
|
|
|
|
|
|
|
|
|
|
|
|
|
|
|
|
|
|
|
|
|
|
|
|
|
|
|
|
|
|
|
|
|
|
|
|
|
|
|
|
|
|
|
|
|
|
|
|
|
|
|
|
|
|
1 |
+
import torch
|
2 |
+
import numpy as np
|
3 |
+
from torch.nn import functional as F
|
4 |
+
import copy
|
5 |
+
# from lib.utils.rotation_conversions import axis_angle_to_matrix, matrix_to_rotation_6d
|
6 |
+
|
7 |
+
|
8 |
+
def batch_rodrigues(axisang):
|
9 |
+
# This function is borrowed from https://github.com/MandyMo/pytorch_HMR/blob/master/src/util.py#L37
|
10 |
+
# axisang N x 3
|
11 |
+
axisang_norm = torch.norm(axisang + 1e-8, p=2, dim=1)
|
12 |
+
angle = torch.unsqueeze(axisang_norm, -1)
|
13 |
+
axisang_normalized = torch.div(axisang, angle)
|
14 |
+
angle = angle * 0.5
|
15 |
+
v_cos = torch.cos(angle)
|
16 |
+
v_sin = torch.sin(angle)
|
17 |
+
quat = torch.cat([v_cos, v_sin * axisang_normalized], dim=1)
|
18 |
+
rot_mat = quat2mat(quat)
|
19 |
+
rot_mat = rot_mat.view(rot_mat.shape[0], 9)
|
20 |
+
return rot_mat
|
21 |
+
|
22 |
+
|
23 |
+
def quat2mat(quat):
|
24 |
+
"""
|
25 |
+
This function is borrowed from https://github.com/MandyMo/pytorch_HMR/blob/master/src/util.py#L50
|
26 |
+
|
27 |
+
Convert quaternion coefficients to rotation matrix.
|
28 |
+
Args:
|
29 |
+
quat: size = [batch_size, 4] 4 <===>(w, x, y, z)
|
30 |
+
Returns:
|
31 |
+
Rotation matrix corresponding to the quaternion -- size = [batch_size, 3, 3]
|
32 |
+
"""
|
33 |
+
norm_quat = quat
|
34 |
+
norm_quat = norm_quat / norm_quat.norm(p=2, dim=1, keepdim=True)
|
35 |
+
w, x, y, z = norm_quat[:, 0], norm_quat[:, 1], norm_quat[:,
|
36 |
+
2], norm_quat[:,
|
37 |
+
3]
|
38 |
+
|
39 |
+
batch_size = quat.size(0)
|
40 |
+
|
41 |
+
w2, x2, y2, z2 = w.pow(2), x.pow(2), y.pow(2), z.pow(2)
|
42 |
+
wx, wy, wz = w * x, w * y, w * z
|
43 |
+
xy, xz, yz = x * y, x * z, y * z
|
44 |
+
|
45 |
+
rotMat = torch.stack([
|
46 |
+
w2 + x2 - y2 - z2, 2 * xy - 2 * wz, 2 * wy + 2 * xz, 2 * wz + 2 * xy,
|
47 |
+
w2 - x2 + y2 - z2, 2 * yz - 2 * wx, 2 * xz - 2 * wy, 2 * wx + 2 * yz,
|
48 |
+
w2 - x2 - y2 + z2
|
49 |
+
],
|
50 |
+
dim=1).view(batch_size, 3, 3)
|
51 |
+
return rotMat
|
52 |
+
|
53 |
+
|
54 |
+
def rotation_matrix_to_angle_axis(rotation_matrix):
|
55 |
+
"""
|
56 |
+
This function is borrowed from https://github.com/kornia/kornia
|
57 |
+
|
58 |
+
Convert 3x4 rotation matrix to Rodrigues vector
|
59 |
+
|
60 |
+
Args:
|
61 |
+
rotation_matrix (Tensor): rotation matrix.
|
62 |
+
|
63 |
+
Returns:
|
64 |
+
Tensor: Rodrigues vector transformation.
|
65 |
+
|
66 |
+
Shape:
|
67 |
+
- Input: :math:`(N, 3, 4)`
|
68 |
+
- Output: :math:`(N, 3)`
|
69 |
+
|
70 |
+
Example:
|
71 |
+
>>> input = torch.rand(2, 3, 4) # Nx4x4
|
72 |
+
>>> output = tgm.rotation_matrix_to_angle_axis(input) # Nx3
|
73 |
+
"""
|
74 |
+
if rotation_matrix.shape[1:] == (3,3):
|
75 |
+
rot_mat = rotation_matrix.reshape(-1, 3, 3)
|
76 |
+
hom = torch.tensor([0, 0, 1], dtype=torch.float32,
|
77 |
+
device=rotation_matrix.device).reshape(1, 3, 1).expand(rot_mat.shape[0], -1, -1)
|
78 |
+
rotation_matrix = torch.cat([rot_mat, hom], dim=-1)
|
79 |
+
|
80 |
+
quaternion = rotation_matrix_to_quaternion(rotation_matrix)
|
81 |
+
aa = quaternion_to_angle_axis(quaternion)
|
82 |
+
aa[torch.isnan(aa)] = 0.0
|
83 |
+
return aa
|
84 |
+
|
85 |
+
|
86 |
+
def quaternion_to_angle_axis(quaternion: torch.Tensor) -> torch.Tensor:
|
87 |
+
"""
|
88 |
+
This function is borrowed from https://github.com/kornia/kornia
|
89 |
+
|
90 |
+
Convert quaternion vector to angle axis of rotation.
|
91 |
+
|
92 |
+
Adapted from ceres C++ library: ceres-solver/include/ceres/rotation.h
|
93 |
+
|
94 |
+
Args:
|
95 |
+
quaternion (torch.Tensor): tensor with quaternions.
|
96 |
+
|
97 |
+
Return:
|
98 |
+
torch.Tensor: tensor with angle axis of rotation.
|
99 |
+
|
100 |
+
Shape:
|
101 |
+
- Input: :math:`(*, 4)` where `*` means, any number of dimensions
|
102 |
+
- Output: :math:`(*, 3)`
|
103 |
+
|
104 |
+
Example:
|
105 |
+
>>> quaternion = torch.rand(2, 4) # Nx4
|
106 |
+
>>> angle_axis = tgm.quaternion_to_angle_axis(quaternion) # Nx3
|
107 |
+
"""
|
108 |
+
if not torch.is_tensor(quaternion):
|
109 |
+
raise TypeError("Input type is not a torch.Tensor. Got {}".format(
|
110 |
+
type(quaternion)))
|
111 |
+
|
112 |
+
if not quaternion.shape[-1] == 4:
|
113 |
+
raise ValueError("Input must be a tensor of shape Nx4 or 4. Got {}"
|
114 |
+
.format(quaternion.shape))
|
115 |
+
# unpack input and compute conversion
|
116 |
+
q1: torch.Tensor = quaternion[..., 1]
|
117 |
+
q2: torch.Tensor = quaternion[..., 2]
|
118 |
+
q3: torch.Tensor = quaternion[..., 3]
|
119 |
+
sin_squared_theta: torch.Tensor = q1 * q1 + q2 * q2 + q3 * q3
|
120 |
+
|
121 |
+
sin_theta: torch.Tensor = torch.sqrt(sin_squared_theta)
|
122 |
+
cos_theta: torch.Tensor = quaternion[..., 0]
|
123 |
+
two_theta: torch.Tensor = 2.0 * torch.where(
|
124 |
+
cos_theta < 0.0,
|
125 |
+
torch.atan2(-sin_theta, -cos_theta),
|
126 |
+
torch.atan2(sin_theta, cos_theta))
|
127 |
+
|
128 |
+
k_pos: torch.Tensor = two_theta / sin_theta
|
129 |
+
k_neg: torch.Tensor = 2.0 * torch.ones_like(sin_theta)
|
130 |
+
k: torch.Tensor = torch.where(sin_squared_theta > 0.0, k_pos, k_neg)
|
131 |
+
|
132 |
+
angle_axis: torch.Tensor = torch.zeros_like(quaternion)[..., :3]
|
133 |
+
angle_axis[..., 0] += q1 * k
|
134 |
+
angle_axis[..., 1] += q2 * k
|
135 |
+
angle_axis[..., 2] += q3 * k
|
136 |
+
return angle_axis
|
137 |
+
|
138 |
+
|
139 |
+
def rotation_matrix_to_quaternion(rotation_matrix, eps=1e-6):
|
140 |
+
"""
|
141 |
+
This function is borrowed from https://github.com/kornia/kornia
|
142 |
+
|
143 |
+
Convert 3x4 rotation matrix to 4d quaternion vector
|
144 |
+
|
145 |
+
This algorithm is based on algorithm described in
|
146 |
+
https://github.com/KieranWynn/pyquaternion/blob/master/pyquaternion/quaternion.py#L201
|
147 |
+
|
148 |
+
Args:
|
149 |
+
rotation_matrix (Tensor): the rotation matrix to convert.
|
150 |
+
|
151 |
+
Return:
|
152 |
+
Tensor: the rotation in quaternion
|
153 |
+
|
154 |
+
Shape:
|
155 |
+
- Input: :math:`(N, 3, 4)`
|
156 |
+
- Output: :math:`(N, 4)`
|
157 |
+
|
158 |
+
Example:
|
159 |
+
>>> input = torch.rand(4, 3, 4) # Nx3x4
|
160 |
+
>>> output = tgm.rotation_matrix_to_quaternion(input) # Nx4
|
161 |
+
"""
|
162 |
+
if not torch.is_tensor(rotation_matrix):
|
163 |
+
raise TypeError("Input type is not a torch.Tensor. Got {}".format(
|
164 |
+
type(rotation_matrix)))
|
165 |
+
|
166 |
+
if len(rotation_matrix.shape) > 3:
|
167 |
+
raise ValueError(
|
168 |
+
"Input size must be a three dimensional tensor. Got {}".format(
|
169 |
+
rotation_matrix.shape))
|
170 |
+
if not rotation_matrix.shape[-2:] == (3, 4):
|
171 |
+
raise ValueError(
|
172 |
+
"Input size must be a N x 3 x 4 tensor. Got {}".format(
|
173 |
+
rotation_matrix.shape))
|
174 |
+
|
175 |
+
rmat_t = torch.transpose(rotation_matrix, 1, 2)
|
176 |
+
|
177 |
+
mask_d2 = rmat_t[:, 2, 2] < eps
|
178 |
+
|
179 |
+
mask_d0_d1 = rmat_t[:, 0, 0] > rmat_t[:, 1, 1]
|
180 |
+
mask_d0_nd1 = rmat_t[:, 0, 0] < -rmat_t[:, 1, 1]
|
181 |
+
|
182 |
+
t0 = 1 + rmat_t[:, 0, 0] - rmat_t[:, 1, 1] - rmat_t[:, 2, 2]
|
183 |
+
q0 = torch.stack([rmat_t[:, 1, 2] - rmat_t[:, 2, 1],
|
184 |
+
t0, rmat_t[:, 0, 1] + rmat_t[:, 1, 0],
|
185 |
+
rmat_t[:, 2, 0] + rmat_t[:, 0, 2]], -1)
|
186 |
+
t0_rep = t0.repeat(4, 1).t()
|
187 |
+
|
188 |
+
t1 = 1 - rmat_t[:, 0, 0] + rmat_t[:, 1, 1] - rmat_t[:, 2, 2]
|
189 |
+
q1 = torch.stack([rmat_t[:, 2, 0] - rmat_t[:, 0, 2],
|
190 |
+
rmat_t[:, 0, 1] + rmat_t[:, 1, 0],
|
191 |
+
t1, rmat_t[:, 1, 2] + rmat_t[:, 2, 1]], -1)
|
192 |
+
t1_rep = t1.repeat(4, 1).t()
|
193 |
+
|
194 |
+
t2 = 1 - rmat_t[:, 0, 0] - rmat_t[:, 1, 1] + rmat_t[:, 2, 2]
|
195 |
+
q2 = torch.stack([rmat_t[:, 0, 1] - rmat_t[:, 1, 0],
|
196 |
+
rmat_t[:, 2, 0] + rmat_t[:, 0, 2],
|
197 |
+
rmat_t[:, 1, 2] + rmat_t[:, 2, 1], t2], -1)
|
198 |
+
t2_rep = t2.repeat(4, 1).t()
|
199 |
+
|
200 |
+
t3 = 1 + rmat_t[:, 0, 0] + rmat_t[:, 1, 1] + rmat_t[:, 2, 2]
|
201 |
+
q3 = torch.stack([t3, rmat_t[:, 1, 2] - rmat_t[:, 2, 1],
|
202 |
+
rmat_t[:, 2, 0] - rmat_t[:, 0, 2],
|
203 |
+
rmat_t[:, 0, 1] - rmat_t[:, 1, 0]], -1)
|
204 |
+
t3_rep = t3.repeat(4, 1).t()
|
205 |
+
|
206 |
+
mask_c0 = mask_d2 * mask_d0_d1
|
207 |
+
mask_c1 = mask_d2 * ~mask_d0_d1
|
208 |
+
mask_c2 = ~mask_d2 * mask_d0_nd1
|
209 |
+
mask_c3 = ~mask_d2 * ~mask_d0_nd1
|
210 |
+
mask_c0 = mask_c0.view(-1, 1).type_as(q0)
|
211 |
+
mask_c1 = mask_c1.view(-1, 1).type_as(q1)
|
212 |
+
mask_c2 = mask_c2.view(-1, 1).type_as(q2)
|
213 |
+
mask_c3 = mask_c3.view(-1, 1).type_as(q3)
|
214 |
+
|
215 |
+
q = q0 * mask_c0 + q1 * mask_c1 + q2 * mask_c2 + q3 * mask_c3
|
216 |
+
q /= torch.sqrt(t0_rep * mask_c0 + t1_rep * mask_c1 + # noqa
|
217 |
+
t2_rep * mask_c2 + t3_rep * mask_c3) # noqa
|
218 |
+
q *= 0.5
|
219 |
+
return q
|
220 |
+
|
221 |
+
|
222 |
+
def estimate_translation_np(S, joints_2d, joints_conf, focal_length=5000., img_size=224.):
|
223 |
+
"""
|
224 |
+
This function is borrowed from https://github.com/nkolot/SPIN/utils/geometry.py
|
225 |
+
|
226 |
+
Find camera translation that brings 3D joints S closest to 2D the corresponding joints_2d.
|
227 |
+
Input:
|
228 |
+
S: (25, 3) 3D joint locations
|
229 |
+
joints: (25, 3) 2D joint locations and confidence
|
230 |
+
Returns:
|
231 |
+
(3,) camera translation vector
|
232 |
+
"""
|
233 |
+
|
234 |
+
num_joints = S.shape[0]
|
235 |
+
# focal length
|
236 |
+
f = np.array([focal_length,focal_length])
|
237 |
+
# optical center
|
238 |
+
center = np.array([img_size/2., img_size/2.])
|
239 |
+
|
240 |
+
# transformations
|
241 |
+
Z = np.reshape(np.tile(S[:,2],(2,1)).T,-1)
|
242 |
+
XY = np.reshape(S[:,0:2],-1)
|
243 |
+
O = np.tile(center,num_joints)
|
244 |
+
F = np.tile(f,num_joints)
|
245 |
+
weight2 = np.reshape(np.tile(np.sqrt(joints_conf),(2,1)).T,-1)
|
246 |
+
|
247 |
+
# least squares
|
248 |
+
Q = np.array([F*np.tile(np.array([1,0]),num_joints), F*np.tile(np.array([0,1]),num_joints), O-np.reshape(joints_2d,-1)]).T
|
249 |
+
c = (np.reshape(joints_2d,-1)-O)*Z - F*XY
|
250 |
+
|
251 |
+
# weighted least squares
|
252 |
+
W = np.diagflat(weight2)
|
253 |
+
Q = np.dot(W,Q)
|
254 |
+
c = np.dot(W,c)
|
255 |
+
|
256 |
+
# square matrix
|
257 |
+
A = np.dot(Q.T,Q)
|
258 |
+
b = np.dot(Q.T,c)
|
259 |
+
|
260 |
+
# solution
|
261 |
+
trans = np.linalg.solve(A, b)
|
262 |
+
|
263 |
+
return trans
|
264 |
+
|
265 |
+
|
266 |
+
def estimate_translation(S, joints_2d, focal_length=5000., img_size=224.):
|
267 |
+
"""
|
268 |
+
This function is borrowed from https://github.com/nkolot/SPIN/utils/geometry.py
|
269 |
+
|
270 |
+
Find camera translation that brings 3D joints S closest to 2D the corresponding joints_2d.
|
271 |
+
Input:
|
272 |
+
S: (B, 49, 3) 3D joint locations
|
273 |
+
joints: (B, 49, 3) 2D joint locations and confidence
|
274 |
+
Returns:
|
275 |
+
(B, 3) camera translation vectors
|
276 |
+
"""
|
277 |
+
|
278 |
+
device = S.device
|
279 |
+
# Use only joints 25:49 (GT joints)
|
280 |
+
S = S[:, 25:, :].cpu().numpy()
|
281 |
+
joints_2d = joints_2d[:, 25:, :].cpu().numpy()
|
282 |
+
joints_conf = joints_2d[:, :, -1]
|
283 |
+
joints_2d = joints_2d[:, :, :-1]
|
284 |
+
trans = np.zeros((S.shape[0], 3), dtype=np.float32)
|
285 |
+
# Find the translation for each example in the batch
|
286 |
+
for i in range(S.shape[0]):
|
287 |
+
S_i = S[i]
|
288 |
+
joints_i = joints_2d[i]
|
289 |
+
conf_i = joints_conf[i]
|
290 |
+
trans[i] = estimate_translation_np(S_i, joints_i, conf_i, focal_length=focal_length, img_size=img_size)
|
291 |
+
return torch.from_numpy(trans).to(device)
|
292 |
+
|
293 |
+
|
294 |
+
def rot6d_to_rotmat_spin(x):
|
295 |
+
"""Convert 6D rotation representation to 3x3 rotation matrix.
|
296 |
+
Based on Zhou et al., "On the Continuity of Rotation Representations in Neural Networks", CVPR 2019
|
297 |
+
Input:
|
298 |
+
(B,6) Batch of 6-D rotation representations
|
299 |
+
Output:
|
300 |
+
(B,3,3) Batch of corresponding rotation matrices
|
301 |
+
"""
|
302 |
+
x = x.view(-1,3,2)
|
303 |
+
a1 = x[:, :, 0]
|
304 |
+
a2 = x[:, :, 1]
|
305 |
+
b1 = F.normalize(a1)
|
306 |
+
b2 = F.normalize(a2 - torch.einsum('bi,bi->b', b1, a2).unsqueeze(-1) * b1)
|
307 |
+
|
308 |
+
# inp = a2 - torch.einsum('bi,bi->b', b1, a2).unsqueeze(-1) * b1
|
309 |
+
# denom = inp.pow(2).sum(dim=1).sqrt().unsqueeze(-1) + 1e-8
|
310 |
+
# b2 = inp / denom
|
311 |
+
|
312 |
+
b3 = torch.cross(b1, b2)
|
313 |
+
return torch.stack((b1, b2, b3), dim=-1)
|
314 |
+
|
315 |
+
|
316 |
+
def rot6d_to_rotmat(x):
|
317 |
+
x = x.view(-1,3,2)
|
318 |
+
|
319 |
+
# Normalize the first vector
|
320 |
+
b1 = F.normalize(x[:, :, 0], dim=1, eps=1e-6)
|
321 |
+
|
322 |
+
dot_prod = torch.sum(b1 * x[:, :, 1], dim=1, keepdim=True)
|
323 |
+
# Compute the second vector by finding the orthogonal complement to it
|
324 |
+
b2 = F.normalize(x[:, :, 1] - dot_prod * b1, dim=-1, eps=1e-6)
|
325 |
+
|
326 |
+
# Finish building the basis by taking the cross product
|
327 |
+
b3 = torch.cross(b1, b2, dim=1)
|
328 |
+
rot_mats = torch.stack([b1, b2, b3], dim=-1)
|
329 |
+
|
330 |
+
return rot_mats
|
331 |
+
|
332 |
+
|
333 |
+
def rigid_transform_3D(A, B):
|
334 |
+
n, dim = A.shape
|
335 |
+
centroid_A = np.mean(A, axis = 0)
|
336 |
+
centroid_B = np.mean(B, axis = 0)
|
337 |
+
H = np.dot(np.transpose(A - centroid_A), B - centroid_B) / n
|
338 |
+
U, s, V = np.linalg.svd(H)
|
339 |
+
R = np.dot(np.transpose(V), np.transpose(U))
|
340 |
+
if np.linalg.det(R) < 0:
|
341 |
+
s[-1] = -s[-1]
|
342 |
+
V[2] = -V[2]
|
343 |
+
R = np.dot(np.transpose(V), np.transpose(U))
|
344 |
+
|
345 |
+
varP = np.var(A, axis=0).sum()
|
346 |
+
c = 1/varP * np.sum(s)
|
347 |
+
|
348 |
+
t = -np.dot(c*R, np.transpose(centroid_A)) + np.transpose(centroid_B)
|
349 |
+
return c, R, t
|
350 |
+
|
351 |
+
|
352 |
+
def rigid_align(A, B):
|
353 |
+
c, R, t = rigid_transform_3D(A, B)
|
354 |
+
A2 = np.transpose(np.dot(c*R, np.transpose(A))) + t
|
355 |
+
return A2
|
356 |
+
|
357 |
+
def compute_error(output, target):
|
358 |
+
with torch.no_grad():
|
359 |
+
pred_verts = output[0]['verts'].reshape(-1, 6890, 3)
|
360 |
+
target_verts = target['verts'].reshape(-1, 6890, 3)
|
361 |
+
|
362 |
+
pred_j3ds = output[0]['kp_3d'].reshape(-1, 17, 3)
|
363 |
+
target_j3ds = target['kp_3d'].reshape(-1, 17, 3)
|
364 |
+
|
365 |
+
# mpve
|
366 |
+
pred_verts = pred_verts - pred_j3ds[:, :1, :]
|
367 |
+
target_verts = target_verts - target_j3ds[:, :1, :]
|
368 |
+
mpves = torch.sqrt(((pred_verts - target_verts) ** 2).sum(dim=-1)).mean(dim=-1).cpu()
|
369 |
+
|
370 |
+
# mpjpe
|
371 |
+
pred_j3ds = pred_j3ds - pred_j3ds[:, :1, :]
|
372 |
+
target_j3ds = target_j3ds - target_j3ds[:, :1, :]
|
373 |
+
mpjpes = torch.sqrt(((pred_j3ds - target_j3ds) ** 2).sum(dim=-1)).mean(dim=-1).cpu()
|
374 |
+
return mpjpes.mean(), mpves.mean()
|
375 |
+
|
376 |
+
def compute_error_frames(output, target):
|
377 |
+
with torch.no_grad():
|
378 |
+
pred_verts = output[0]['verts'].reshape(-1, 6890, 3)
|
379 |
+
target_verts = target['verts'].reshape(-1, 6890, 3)
|
380 |
+
|
381 |
+
pred_j3ds = output[0]['kp_3d'].reshape(-1, 17, 3)
|
382 |
+
target_j3ds = target['kp_3d'].reshape(-1, 17, 3)
|
383 |
+
|
384 |
+
# mpve
|
385 |
+
pred_verts = pred_verts - pred_j3ds[:, :1, :]
|
386 |
+
target_verts = target_verts - target_j3ds[:, :1, :]
|
387 |
+
mpves = torch.sqrt(((pred_verts - target_verts) ** 2).sum(dim=-1)).mean(dim=-1).cpu()
|
388 |
+
|
389 |
+
# mpjpe
|
390 |
+
pred_j3ds = pred_j3ds - pred_j3ds[:, :1, :]
|
391 |
+
target_j3ds = target_j3ds - target_j3ds[:, :1, :]
|
392 |
+
mpjpes = torch.sqrt(((pred_j3ds - target_j3ds) ** 2).sum(dim=-1)).mean(dim=-1).cpu()
|
393 |
+
return mpjpes, mpves
|
394 |
+
|
395 |
+
def evaluate_mesh(results):
|
396 |
+
pred_verts = results['verts'].reshape(-1, 6890, 3)
|
397 |
+
target_verts = results['verts_gt'].reshape(-1, 6890, 3)
|
398 |
+
|
399 |
+
pred_j3ds = results['kp_3d'].reshape(-1, 17, 3)
|
400 |
+
target_j3ds = results['kp_3d_gt'].reshape(-1, 17, 3)
|
401 |
+
num_samples = pred_j3ds.shape[0]
|
402 |
+
|
403 |
+
# mpve
|
404 |
+
pred_verts = pred_verts - pred_j3ds[:, :1, :]
|
405 |
+
target_verts = target_verts - target_j3ds[:, :1, :]
|
406 |
+
mpve = np.mean(np.mean(np.sqrt(np.square(pred_verts - target_verts).sum(axis=2)), axis=1))
|
407 |
+
|
408 |
+
|
409 |
+
# mpjpe-17 & mpjpe-14
|
410 |
+
h36m_17_to_14 = (1, 2, 3, 4, 5, 6, 8, 10, 11, 12, 13, 14, 15, 16)
|
411 |
+
pred_j3ds_17j = (pred_j3ds - pred_j3ds[:, :1, :])
|
412 |
+
target_j3ds_17j = (target_j3ds - target_j3ds[:, :1, :])
|
413 |
+
|
414 |
+
pred_j3ds = pred_j3ds_17j[:, h36m_17_to_14, :].copy()
|
415 |
+
target_j3ds = target_j3ds_17j[:, h36m_17_to_14, :].copy()
|
416 |
+
|
417 |
+
mpjpe = np.mean(np.sqrt(np.square(pred_j3ds - target_j3ds).sum(axis=2)), axis=1) # (N, )
|
418 |
+
mpjpe_17j = np.mean(np.sqrt(np.square(pred_j3ds_17j - target_j3ds_17j).sum(axis=2)), axis=1) # (N, )
|
419 |
+
|
420 |
+
pred_j3ds_pa, pred_j3ds_pa_17j = [], []
|
421 |
+
for n in range(num_samples):
|
422 |
+
pred_j3ds_pa.append(rigid_align(pred_j3ds[n], target_j3ds[n]))
|
423 |
+
pred_j3ds_pa_17j.append(rigid_align(pred_j3ds_17j[n], target_j3ds_17j[n]))
|
424 |
+
pred_j3ds_pa = np.array(pred_j3ds_pa)
|
425 |
+
pred_j3ds_pa_17j = np.array(pred_j3ds_pa_17j)
|
426 |
+
|
427 |
+
pa_mpjpe = np.mean(np.sqrt(np.square(pred_j3ds_pa - target_j3ds).sum(axis=2)), axis=1) # (N, )
|
428 |
+
pa_mpjpe_17j = np.mean(np.sqrt(np.square(pred_j3ds_pa_17j - target_j3ds_17j).sum(axis=2)), axis=1) # (N, )
|
429 |
+
|
430 |
+
|
431 |
+
error_dict = {
|
432 |
+
'mpve': mpve.mean(),
|
433 |
+
'mpjpe': mpjpe.mean(),
|
434 |
+
'pa_mpjpe': pa_mpjpe.mean(),
|
435 |
+
'mpjpe_17j': mpjpe_17j.mean(),
|
436 |
+
'pa_mpjpe_17j': pa_mpjpe_17j.mean(),
|
437 |
+
}
|
438 |
+
return error_dict
|
439 |
+
|
440 |
+
|
441 |
+
def rectify_pose(pose):
|
442 |
+
"""
|
443 |
+
Rectify "upside down" people in global coord
|
444 |
+
|
445 |
+
Args:
|
446 |
+
pose (72,): Pose.
|
447 |
+
|
448 |
+
Returns:
|
449 |
+
Rotated pose.
|
450 |
+
"""
|
451 |
+
pose = pose.copy()
|
452 |
+
R_mod = cv2.Rodrigues(np.array([np.pi, 0, 0]))[0]
|
453 |
+
R_root = cv2.Rodrigues(pose[:3])[0]
|
454 |
+
new_root = R_root.dot(R_mod)
|
455 |
+
pose[:3] = cv2.Rodrigues(new_root)[0].reshape(3)
|
456 |
+
return pose
|
457 |
+
|
458 |
+
def flip_thetas(thetas):
|
459 |
+
"""Flip thetas.
|
460 |
+
|
461 |
+
Parameters
|
462 |
+
----------
|
463 |
+
thetas : numpy.ndarray
|
464 |
+
Joints in shape (F, num_thetas, 3)
|
465 |
+
theta_pairs : list
|
466 |
+
List of theta pairs.
|
467 |
+
|
468 |
+
Returns
|
469 |
+
-------
|
470 |
+
numpy.ndarray
|
471 |
+
Flipped thetas with shape (F, num_thetas, 3)
|
472 |
+
|
473 |
+
"""
|
474 |
+
#Joint pairs which defines the pairs of joint to be swapped when the image is flipped horizontally.
|
475 |
+
theta_pairs = ((1, 2), (4, 5), (7, 8), (10, 11), (13, 14), (16, 17), (18, 19), (20, 21), (22, 23))
|
476 |
+
thetas_flip = thetas.copy()
|
477 |
+
# reflect horizontally
|
478 |
+
thetas_flip[:, :, 1] = -1 * thetas_flip[:, :, 1]
|
479 |
+
thetas_flip[:, :, 2] = -1 * thetas_flip[:, :, 2]
|
480 |
+
# change left-right parts
|
481 |
+
for pair in theta_pairs:
|
482 |
+
thetas_flip[:, pair[0], :], thetas_flip[:, pair[1], :] = \
|
483 |
+
thetas_flip[:, pair[1], :], thetas_flip[:, pair[0], :].copy()
|
484 |
+
return thetas_flip
|
485 |
+
|
486 |
+
def flip_thetas_batch(thetas):
|
487 |
+
"""Flip thetas in batch.
|
488 |
+
|
489 |
+
Parameters
|
490 |
+
----------
|
491 |
+
thetas : numpy.array
|
492 |
+
Joints in shape (N, F, num_thetas*3)
|
493 |
+
theta_pairs : list
|
494 |
+
List of theta pairs.
|
495 |
+
|
496 |
+
Returns
|
497 |
+
-------
|
498 |
+
numpy.array
|
499 |
+
Flipped thetas with shape (N, F, num_thetas*3)
|
500 |
+
|
501 |
+
"""
|
502 |
+
#Joint pairs which defines the pairs of joint to be swapped when the image is flipped horizontally.
|
503 |
+
theta_pairs = ((1, 2), (4, 5), (7, 8), (10, 11), (13, 14), (16, 17), (18, 19), (20, 21), (22, 23))
|
504 |
+
thetas_flip = copy.deepcopy(thetas).reshape(*thetas.shape[:2], 24, 3)
|
505 |
+
# reflect horizontally
|
506 |
+
thetas_flip[:, :, :, 1] = -1 * thetas_flip[:, :, :, 1]
|
507 |
+
thetas_flip[:, :, :, 2] = -1 * thetas_flip[:, :, :, 2]
|
508 |
+
# change left-right parts
|
509 |
+
for pair in theta_pairs:
|
510 |
+
thetas_flip[:, :, pair[0], :], thetas_flip[:, :, pair[1], :] = \
|
511 |
+
thetas_flip[:, :, pair[1], :], thetas_flip[:, :, pair[0], :].clone()
|
512 |
+
|
513 |
+
return thetas_flip.reshape(*thetas.shape[:2], -1)
|
514 |
+
|
515 |
+
# def smpl_aa_to_ortho6d(smpl_aa):
|
516 |
+
# # [...,72] -> [...,144]
|
517 |
+
# rot_aa = smpl_aa.reshape([-1,24,3])
|
518 |
+
# rotmat = axis_angle_to_matrix(rot_aa)
|
519 |
+
# rot6d = matrix_to_rotation_6d(rotmat)
|
520 |
+
# rot6d = rot6d.reshape(-1,24*6)
|
521 |
+
# return rot6d
|
lib/utils/utils_smpl.py
ADDED
@@ -0,0 +1,88 @@
|
|
|
|
|
|
|
|
|
|
|
|
|
|
|
|
|
|
|
|
|
|
|
|
|
|
|
|
|
|
|
|
|
|
|
|
|
|
|
|
|
|
|
|
|
|
|
|
|
|
|
|
|
|
|
|
|
|
|
|
|
|
|
|
|
|
|
|
|
|
|
|
|
|
|
|
|
|
|
|
|
|
|
|
|
|
|
|
|
|
|
|
|
|
|
|
|
|
|
|
|
|
|
|
|
|
|
|
|
|
|
|
|
|
|
|
|
|
|
|
|
|
|
|
|
|
|
|
|
|
|
|
|
|
|
|
|
|
|
|
|
|
|
|
|
|
|
|
|
|
|
|
|
|
|
|
|
|
|
|
|
|
|
|
|
|
|
|
|
|
|
|
|
|
|
|
|
|
|
1 |
+
# This script is borrowed and extended from https://github.com/nkolot/SPIN/blob/master/models/hmr.py
|
2 |
+
# Adhere to their licence to use this script
|
3 |
+
|
4 |
+
import torch
|
5 |
+
import numpy as np
|
6 |
+
import os.path as osp
|
7 |
+
from smplx import SMPL as _SMPL
|
8 |
+
from smplx.utils import ModelOutput, SMPLOutput
|
9 |
+
from smplx.lbs import vertices2joints
|
10 |
+
|
11 |
+
|
12 |
+
# Map joints to SMPL joints
|
13 |
+
JOINT_MAP = {
|
14 |
+
'OP Nose': 24, 'OP Neck': 12, 'OP RShoulder': 17,
|
15 |
+
'OP RElbow': 19, 'OP RWrist': 21, 'OP LShoulder': 16,
|
16 |
+
'OP LElbow': 18, 'OP LWrist': 20, 'OP MidHip': 0,
|
17 |
+
'OP RHip': 2, 'OP RKnee': 5, 'OP RAnkle': 8,
|
18 |
+
'OP LHip': 1, 'OP LKnee': 4, 'OP LAnkle': 7,
|
19 |
+
'OP REye': 25, 'OP LEye': 26, 'OP REar': 27,
|
20 |
+
'OP LEar': 28, 'OP LBigToe': 29, 'OP LSmallToe': 30,
|
21 |
+
'OP LHeel': 31, 'OP RBigToe': 32, 'OP RSmallToe': 33, 'OP RHeel': 34,
|
22 |
+
'Right Ankle': 8, 'Right Knee': 5, 'Right Hip': 45,
|
23 |
+
'Left Hip': 46, 'Left Knee': 4, 'Left Ankle': 7,
|
24 |
+
'Right Wrist': 21, 'Right Elbow': 19, 'Right Shoulder': 17,
|
25 |
+
'Left Shoulder': 16, 'Left Elbow': 18, 'Left Wrist': 20,
|
26 |
+
'Neck (LSP)': 47, 'Top of Head (LSP)': 48,
|
27 |
+
'Pelvis (MPII)': 49, 'Thorax (MPII)': 50,
|
28 |
+
'Spine (H36M)': 51, 'Jaw (H36M)': 52,
|
29 |
+
'Head (H36M)': 53, 'Nose': 24, 'Left Eye': 26,
|
30 |
+
'Right Eye': 25, 'Left Ear': 28, 'Right Ear': 27
|
31 |
+
}
|
32 |
+
JOINT_NAMES = [
|
33 |
+
'OP Nose', 'OP Neck', 'OP RShoulder',
|
34 |
+
'OP RElbow', 'OP RWrist', 'OP LShoulder',
|
35 |
+
'OP LElbow', 'OP LWrist', 'OP MidHip',
|
36 |
+
'OP RHip', 'OP RKnee', 'OP RAnkle',
|
37 |
+
'OP LHip', 'OP LKnee', 'OP LAnkle',
|
38 |
+
'OP REye', 'OP LEye', 'OP REar',
|
39 |
+
'OP LEar', 'OP LBigToe', 'OP LSmallToe',
|
40 |
+
'OP LHeel', 'OP RBigToe', 'OP RSmallToe', 'OP RHeel',
|
41 |
+
'Right Ankle', 'Right Knee', 'Right Hip',
|
42 |
+
'Left Hip', 'Left Knee', 'Left Ankle',
|
43 |
+
'Right Wrist', 'Right Elbow', 'Right Shoulder',
|
44 |
+
'Left Shoulder', 'Left Elbow', 'Left Wrist',
|
45 |
+
'Neck (LSP)', 'Top of Head (LSP)',
|
46 |
+
'Pelvis (MPII)', 'Thorax (MPII)',
|
47 |
+
'Spine (H36M)', 'Jaw (H36M)',
|
48 |
+
'Head (H36M)', 'Nose', 'Left Eye',
|
49 |
+
'Right Eye', 'Left Ear', 'Right Ear'
|
50 |
+
]
|
51 |
+
|
52 |
+
JOINT_IDS = {JOINT_NAMES[i]: i for i in range(len(JOINT_NAMES))}
|
53 |
+
SMPL_MODEL_DIR = 'data/mesh'
|
54 |
+
H36M_TO_J17 = [6, 5, 4, 1, 2, 3, 16, 15, 14, 11, 12, 13, 8, 10, 0, 7, 9]
|
55 |
+
H36M_TO_J14 = H36M_TO_J17[:14]
|
56 |
+
|
57 |
+
|
58 |
+
class SMPL(_SMPL):
|
59 |
+
""" Extension of the official SMPL implementation to support more joints """
|
60 |
+
|
61 |
+
def __init__(self, *args, **kwargs):
|
62 |
+
super(SMPL, self).__init__(*args, **kwargs)
|
63 |
+
joints = [JOINT_MAP[i] for i in JOINT_NAMES]
|
64 |
+
self.smpl_mean_params = osp.join(args[0], 'smpl_mean_params.npz')
|
65 |
+
J_regressor_extra = np.load(osp.join(args[0], 'J_regressor_extra.npy'))
|
66 |
+
self.register_buffer('J_regressor_extra', torch.tensor(J_regressor_extra, dtype=torch.float32))
|
67 |
+
J_regressor_h36m = np.load(osp.join(args[0], 'J_regressor_h36m_correct.npy'))
|
68 |
+
self.register_buffer('J_regressor_h36m', torch.tensor(J_regressor_h36m, dtype=torch.float32))
|
69 |
+
self.joint_map = torch.tensor(joints, dtype=torch.long)
|
70 |
+
|
71 |
+
def forward(self, *args, **kwargs):
|
72 |
+
kwargs['get_skin'] = True
|
73 |
+
smpl_output = super(SMPL, self).forward(*args, **kwargs)
|
74 |
+
extra_joints = vertices2joints(self.J_regressor_extra, smpl_output.vertices)
|
75 |
+
joints = torch.cat([smpl_output.joints, extra_joints], dim=1)
|
76 |
+
joints = joints[:, self.joint_map, :]
|
77 |
+
output = SMPLOutput(vertices=smpl_output.vertices,
|
78 |
+
global_orient=smpl_output.global_orient,
|
79 |
+
body_pose=smpl_output.body_pose,
|
80 |
+
joints=joints,
|
81 |
+
betas=smpl_output.betas,
|
82 |
+
full_pose=smpl_output.full_pose)
|
83 |
+
return output
|
84 |
+
|
85 |
+
|
86 |
+
def get_smpl_faces():
|
87 |
+
smpl = SMPL(SMPL_MODEL_DIR, batch_size=1, create_transl=False)
|
88 |
+
return smpl.faces
|
lib/utils/vismo.py
ADDED
@@ -0,0 +1,347 @@
|
|
|
|
|
|
|
|
|
|
|
|
|
|
|
|
|
|
|
|
|
|
|
|
|
|
|
|
|
|
|
|
|
|
|
|
|
|
|
|
|
|
|
|
|
|
|
|
|
|
|
|
|
|
|
|
|
|
|
|
|
|
|
|
|
|
|
|
|
|
|
|
|
|
|
|
|
|
|
|
|
|
|
|
|
|
|
|
|
|
|
|
|
|
|
|
|
|
|
|
|
|
|
|
|
|
|
|
|
|
|
|
|
|
|
|
|
|
|
|
|
|
|
|
|
|
|
|
|
|
|
|
|
|
|
|
|
|
|
|
|
|
|
|
|
|
|
|
|
|
|
|
|
|
|
|
|
|
|
|
|
|
|
|
|
|
|
|
|
|
|
|
|
|
|
|
|
|
|
|
|
|
|
|
|
|
|
|
|
|
|
|
|
|
|
|
|
|
|
|
|
|
|
|
|
|
|
|
|
|
|
|
|
|
|
|
|
|
|
|
|
|
|
|
|
|
|
|
|
|
|
|
|
|
|
|
|
|
|
|
|
|
|
|
|
|
|
|
|
|
|
|
|
|
|
|
|
|
|
|
|
|
|
|
|
|
|
|
|
|
|
|
|
|
|
|
|
|
|
|
|
|
|
|
|
|
|
|
|
|
|
|
|
|
|
|
|
|
|
|
|
|
|
|
|
|
|
|
|
|
|
|
|
|
|
|
|
|
|
|
|
|
|
|
|
|
|
|
|
|
|
|
|
|
|
|
|
|
|
|
|
|
|
|
|
|
|
|
|
|
|
|
|
|
|
|
|
|
|
|
|
|
|
|
|
|
|
|
|
|
|
|
|
|
|
|
|
|
|
|
|
|
|
|
|
|
|
|
|
|
|
|
|
|
|
|
|
|
|
|
|
|
|
|
|
|
|
|
|
|
|
|
|
|
|
|
|
|
|
|
|
|
|
|
|
|
|
|
|
|
|
|
|
|
|
|
|
|
|
|
|
|
|
|
|
|
|
|
|
|
|
|
|
|
|
|
|
|
|
|
|
|
|
|
|
|
|
|
|
|
|
|
|
|
|
|
|
|
|
|
|
|
|
|
|
|
|
|
|
|
|
|
|
|
|
|
|
|
|
|
|
|
|
|
|
|
|
|
|
|
|
|
|
|
|
|
|
|
|
|
|
|
|
|
|
|
|
|
|
|
|
|
|
|
|
|
|
|
|
|
|
|
|
|
|
|
|
|
|
|
|
|
|
|
|
|
|
|
|
|
|
|
|
|
|
|
|
|
|
|
|
|
|
|
|
|
|
|
|
|
|
|
|
|
|
|
|
|
|
|
|
|
|
|
|
|
|
|
|
|
|
|
|
|
|
|
|
|
|
|
|
|
|
|
|
|
|
|
|
|
|
|
|
|
|
|
|
|
|
|
|
|
|
|
|
|
|
|
|
|
|
|
|
|
|
|
|
|
|
|
|
|
|
|
|
|
|
|
|
|
|
|
|
|
|
|
|
|
|
|
|
|
|
|
|
|
|
|
|
|
|
|
|
|
|
|
|
|
|
|
|
|
|
|
|
|
|
1 |
+
import numpy as np
|
2 |
+
import os
|
3 |
+
import cv2
|
4 |
+
import math
|
5 |
+
import copy
|
6 |
+
import imageio
|
7 |
+
import io
|
8 |
+
from tqdm import tqdm
|
9 |
+
from PIL import Image
|
10 |
+
from lib.utils.tools import ensure_dir
|
11 |
+
import matplotlib
|
12 |
+
import matplotlib.pyplot as plt
|
13 |
+
from mpl_toolkits.mplot3d import Axes3D
|
14 |
+
from lib.utils.utils_smpl import *
|
15 |
+
import ipdb
|
16 |
+
|
17 |
+
def render_and_save(motion_input, save_path, keep_imgs=False, fps=25, color="#F96706#FB8D43#FDB381", with_conf=False, draw_face=False):
|
18 |
+
ensure_dir(os.path.dirname(save_path))
|
19 |
+
motion = copy.deepcopy(motion_input)
|
20 |
+
if motion.shape[-1]==2 or motion.shape[-1]==3:
|
21 |
+
motion = np.transpose(motion, (1,2,0)) #(T,17,D) -> (17,D,T)
|
22 |
+
if motion.shape[1]==2 or with_conf:
|
23 |
+
colors = hex2rgb(color)
|
24 |
+
if not with_conf:
|
25 |
+
J, D, T = motion.shape
|
26 |
+
motion_full = np.ones([J,3,T])
|
27 |
+
motion_full[:,:2,:] = motion
|
28 |
+
else:
|
29 |
+
motion_full = motion
|
30 |
+
motion_full[:,:2,:] = pixel2world_vis_motion(motion_full[:,:2,:])
|
31 |
+
motion2video(motion_full, save_path=save_path, colors=colors, fps=fps)
|
32 |
+
elif motion.shape[0]==6890:
|
33 |
+
# motion_world = pixel2world_vis_motion(motion, dim=3)
|
34 |
+
motion2video_mesh(motion, save_path=save_path, keep_imgs=keep_imgs, fps=fps, draw_face=draw_face)
|
35 |
+
else:
|
36 |
+
motion_world = pixel2world_vis_motion(motion, dim=3)
|
37 |
+
motion2video_3d(motion_world, save_path=save_path, keep_imgs=keep_imgs, fps=fps)
|
38 |
+
|
39 |
+
def pixel2world_vis(pose):
|
40 |
+
# pose: (17,2)
|
41 |
+
return (pose + [1, 1]) * 512 / 2
|
42 |
+
|
43 |
+
def pixel2world_vis_motion(motion, dim=2, is_tensor=False):
|
44 |
+
# pose: (17,2,N)
|
45 |
+
N = motion.shape[-1]
|
46 |
+
if dim==2:
|
47 |
+
offset = np.ones([2,N]).astype(np.float32)
|
48 |
+
else:
|
49 |
+
offset = np.ones([3,N]).astype(np.float32)
|
50 |
+
offset[2,:] = 0
|
51 |
+
if is_tensor:
|
52 |
+
offset = torch.tensor(offset)
|
53 |
+
return (motion + offset) * 512 / 2
|
54 |
+
|
55 |
+
def vis_data_batch(data_input, data_label, n_render=10, save_path='doodle/vis_train_data/'):
|
56 |
+
'''
|
57 |
+
data_input: [N,T,17,2/3]
|
58 |
+
data_label: [N,T,17,3]
|
59 |
+
'''
|
60 |
+
pathlib.Path(save_path).mkdir(parents=True, exist_ok=True)
|
61 |
+
for i in range(min(len(data_input), n_render)):
|
62 |
+
render_and_save(data_input[i][:,:,:2], '%s/input_%d.mp4' % (save_path, i))
|
63 |
+
render_and_save(data_label[i], '%s/gt_%d.mp4' % (save_path, i))
|
64 |
+
|
65 |
+
def get_img_from_fig(fig, dpi=120):
|
66 |
+
buf = io.BytesIO()
|
67 |
+
fig.savefig(buf, format="png", dpi=dpi, bbox_inches="tight", pad_inches=0)
|
68 |
+
buf.seek(0)
|
69 |
+
img_arr = np.frombuffer(buf.getvalue(), dtype=np.uint8)
|
70 |
+
buf.close()
|
71 |
+
img = cv2.imdecode(img_arr, 1)
|
72 |
+
img = cv2.cvtColor(img, cv2.COLOR_BGR2RGBA)
|
73 |
+
return img
|
74 |
+
|
75 |
+
def rgb2rgba(color):
|
76 |
+
return (color[0], color[1], color[2], 255)
|
77 |
+
|
78 |
+
def hex2rgb(hex, number_of_colors=3):
|
79 |
+
h = hex
|
80 |
+
rgb = []
|
81 |
+
for i in range(number_of_colors):
|
82 |
+
h = h.lstrip('#')
|
83 |
+
hex_color = h[0:6]
|
84 |
+
rgb_color = [int(hex_color[i:i+2], 16) for i in (0, 2 ,4)]
|
85 |
+
rgb.append(rgb_color)
|
86 |
+
h = h[6:]
|
87 |
+
return rgb
|
88 |
+
|
89 |
+
def joints2image(joints_position, colors, transparency=False, H=1000, W=1000, nr_joints=49, imtype=np.uint8, grayscale=False, bg_color=(255, 255, 255)):
|
90 |
+
# joints_position: [17*2]
|
91 |
+
nr_joints = joints_position.shape[0]
|
92 |
+
|
93 |
+
if nr_joints == 49: # full joints(49): basic(15) + eyes(2) + toes(2) + hands(30)
|
94 |
+
limbSeq = [[0, 1], [1, 2], [1, 5], [1, 8], [2, 3], [3, 4], [5, 6], [6, 7], \
|
95 |
+
[8, 9], [8, 13], [9, 10], [10, 11], [11, 12], [13, 14], [14, 15], [15, 16],
|
96 |
+
]#[0, 17], [0, 18]] #ignore eyes
|
97 |
+
|
98 |
+
L = rgb2rgba(colors[0]) if transparency else colors[0]
|
99 |
+
M = rgb2rgba(colors[1]) if transparency else colors[1]
|
100 |
+
R = rgb2rgba(colors[2]) if transparency else colors[2]
|
101 |
+
|
102 |
+
colors_joints = [M, M, L, L, L, R, R,
|
103 |
+
R, M, L, L, L, L, R, R, R,
|
104 |
+
R, R, L] + [L] * 15 + [R] * 15
|
105 |
+
|
106 |
+
colors_limbs = [M, L, R, M, L, L, R,
|
107 |
+
R, L, R, L, L, L, R, R, R,
|
108 |
+
R, R]
|
109 |
+
elif nr_joints == 15: # basic joints(15) + (eyes(2))
|
110 |
+
limbSeq = [[0, 1], [1, 2], [1, 5], [1, 8], [2, 3], [3, 4], [5, 6], [6, 7],
|
111 |
+
[8, 9], [8, 12], [9, 10], [10, 11], [12, 13], [13, 14]]
|
112 |
+
# [0, 15], [0, 16] two eyes are not drawn
|
113 |
+
|
114 |
+
L = rgb2rgba(colors[0]) if transparency else colors[0]
|
115 |
+
M = rgb2rgba(colors[1]) if transparency else colors[1]
|
116 |
+
R = rgb2rgba(colors[2]) if transparency else colors[2]
|
117 |
+
|
118 |
+
colors_joints = [M, M, L, L, L, R, R,
|
119 |
+
R, M, L, L, L, R, R, R]
|
120 |
+
|
121 |
+
colors_limbs = [M, L, R, M, L, L, R,
|
122 |
+
R, L, R, L, L, R, R]
|
123 |
+
elif nr_joints == 17: # H36M, 0: 'root',
|
124 |
+
# 1: 'rhip',
|
125 |
+
# 2: 'rkne',
|
126 |
+
# 3: 'rank',
|
127 |
+
# 4: 'lhip',
|
128 |
+
# 5: 'lkne',
|
129 |
+
# 6: 'lank',
|
130 |
+
# 7: 'belly',
|
131 |
+
# 8: 'neck',
|
132 |
+
# 9: 'nose',
|
133 |
+
# 10: 'head',
|
134 |
+
# 11: 'lsho',
|
135 |
+
# 12: 'lelb',
|
136 |
+
# 13: 'lwri',
|
137 |
+
# 14: 'rsho',
|
138 |
+
# 15: 'relb',
|
139 |
+
# 16: 'rwri'
|
140 |
+
limbSeq = [[0, 1], [1, 2], [2, 3], [0, 4], [4, 5], [5, 6], [0, 7], [7, 8], [8, 9], [8, 11], [8, 14], [9, 10], [11, 12], [12, 13], [14, 15], [15, 16]]
|
141 |
+
|
142 |
+
L = rgb2rgba(colors[0]) if transparency else colors[0]
|
143 |
+
M = rgb2rgba(colors[1]) if transparency else colors[1]
|
144 |
+
R = rgb2rgba(colors[2]) if transparency else colors[2]
|
145 |
+
|
146 |
+
colors_joints = [M, R, R, R, L, L, L, M, M, M, M, L, L, L, R, R, R]
|
147 |
+
colors_limbs = [R, R, R, L, L, L, M, M, M, L, R, M, L, L, R, R]
|
148 |
+
|
149 |
+
else:
|
150 |
+
raise ValueError("Only support number of joints be 49 or 17 or 15")
|
151 |
+
|
152 |
+
if transparency:
|
153 |
+
canvas = np.zeros(shape=(H, W, 4))
|
154 |
+
else:
|
155 |
+
canvas = np.ones(shape=(H, W, 3)) * np.array(bg_color).reshape([1, 1, 3])
|
156 |
+
hips = joints_position[0]
|
157 |
+
neck = joints_position[8]
|
158 |
+
torso_length = ((hips[1] - neck[1]) ** 2 + (hips[0] - neck[0]) ** 2) ** 0.5
|
159 |
+
head_radius = int(torso_length/4.5)
|
160 |
+
end_effectors_radius = int(torso_length/15)
|
161 |
+
end_effectors_radius = 7
|
162 |
+
joints_radius = 7
|
163 |
+
for i in range(0, len(colors_joints)):
|
164 |
+
if i in (17, 18):
|
165 |
+
continue
|
166 |
+
elif i > 18:
|
167 |
+
radius = 2
|
168 |
+
else:
|
169 |
+
radius = joints_radius
|
170 |
+
if len(joints_position[i])==3: # If there is confidence, weigh by confidence
|
171 |
+
weight = joints_position[i][2]
|
172 |
+
if weight==0:
|
173 |
+
continue
|
174 |
+
cv2.circle(canvas, (int(joints_position[i][0]),int(joints_position[i][1])), radius, colors_joints[i], thickness=-1)
|
175 |
+
|
176 |
+
stickwidth = 2
|
177 |
+
for i in range(len(limbSeq)):
|
178 |
+
limb = limbSeq[i]
|
179 |
+
cur_canvas = canvas.copy()
|
180 |
+
point1_index = limb[0]
|
181 |
+
point2_index = limb[1]
|
182 |
+
point1 = joints_position[point1_index]
|
183 |
+
point2 = joints_position[point2_index]
|
184 |
+
if len(point1)==3: # If there is confidence, weigh by confidence
|
185 |
+
limb_weight = min(point1[2], point2[2])
|
186 |
+
if limb_weight==0:
|
187 |
+
bb = bounding_box(canvas)
|
188 |
+
canvas_cropped = canvas[:,bb[2]:bb[3], :]
|
189 |
+
continue
|
190 |
+
X = [point1[1], point2[1]]
|
191 |
+
Y = [point1[0], point2[0]]
|
192 |
+
mX = np.mean(X)
|
193 |
+
mY = np.mean(Y)
|
194 |
+
length = ((X[0] - X[1]) ** 2 + (Y[0] - Y[1]) ** 2) ** 0.5
|
195 |
+
alpha = math.degrees(math.atan2(X[0] - X[1], Y[0] - Y[1]))
|
196 |
+
polygon = cv2.ellipse2Poly((int(mY), int(mX)), (int(length / 2), stickwidth), int(alpha), 0, 360, 1)
|
197 |
+
cv2.fillConvexPoly(cur_canvas, polygon, colors_limbs[i])
|
198 |
+
canvas = cv2.addWeighted(canvas, 0.4, cur_canvas, 0.6, 0)
|
199 |
+
bb = bounding_box(canvas)
|
200 |
+
canvas_cropped = canvas[:,bb[2]:bb[3], :]
|
201 |
+
canvas = canvas.astype(imtype)
|
202 |
+
canvas_cropped = canvas_cropped.astype(imtype)
|
203 |
+
if grayscale:
|
204 |
+
if transparency:
|
205 |
+
canvas = cv2.cvtColor(canvas, cv2.COLOR_RGBA2GRAY)
|
206 |
+
canvas_cropped = cv2.cvtColor(canvas_cropped, cv2.COLOR_RGBA2GRAY)
|
207 |
+
else:
|
208 |
+
canvas = cv2.cvtColor(canvas, cv2.COLOR_RGB2GRAY)
|
209 |
+
canvas_cropped = cv2.cvtColor(canvas_cropped, cv2.COLOR_RGB2GRAY)
|
210 |
+
return [canvas, canvas_cropped]
|
211 |
+
|
212 |
+
|
213 |
+
def motion2video(motion, save_path, colors, h=512, w=512, bg_color=(255, 255, 255), transparency=False, motion_tgt=None, fps=25, save_frame=False, grayscale=False, show_progress=True, as_array=False):
|
214 |
+
nr_joints = motion.shape[0]
|
215 |
+
# as_array = save_path.endswith(".npy")
|
216 |
+
vlen = motion.shape[-1]
|
217 |
+
|
218 |
+
out_array = np.zeros([vlen, h, w, 3]) if as_array else None
|
219 |
+
videowriter = None if as_array else imageio.get_writer(save_path, fps=fps)
|
220 |
+
|
221 |
+
if save_frame:
|
222 |
+
frames_dir = save_path[:-4] + '-frames'
|
223 |
+
ensure_dir(frames_dir)
|
224 |
+
|
225 |
+
iterator = range(vlen)
|
226 |
+
if show_progress: iterator = tqdm(iterator)
|
227 |
+
for i in iterator:
|
228 |
+
[img, img_cropped] = joints2image(motion[:, :, i], colors, transparency=transparency, bg_color=bg_color, H=h, W=w, nr_joints=nr_joints, grayscale=grayscale)
|
229 |
+
if motion_tgt is not None:
|
230 |
+
[img_tgt, img_tgt_cropped] = joints2image(motion_tgt[:, :, i], colors, transparency=transparency, bg_color=bg_color, H=h, W=w, nr_joints=nr_joints, grayscale=grayscale)
|
231 |
+
img_ori = img.copy()
|
232 |
+
img = cv2.addWeighted(img_tgt, 0.3, img_ori, 0.7, 0)
|
233 |
+
img_cropped = cv2.addWeighted(img_tgt, 0.3, img_ori, 0.7, 0)
|
234 |
+
bb = bounding_box(img_cropped)
|
235 |
+
img_cropped = img_cropped[:, bb[2]:bb[3], :]
|
236 |
+
if save_frame:
|
237 |
+
save_image(img_cropped, os.path.join(frames_dir, "%04d.png" % i))
|
238 |
+
if as_array: out_array[i] = img
|
239 |
+
else: videowriter.append_data(img)
|
240 |
+
|
241 |
+
if not as_array:
|
242 |
+
videowriter.close()
|
243 |
+
|
244 |
+
return out_array
|
245 |
+
|
246 |
+
def motion2video_3d(motion, save_path, fps=25, keep_imgs = False):
|
247 |
+
# motion: (17,3,N)
|
248 |
+
videowriter = imageio.get_writer(save_path, fps=fps)
|
249 |
+
vlen = motion.shape[-1]
|
250 |
+
save_name = save_path.split('.')[0]
|
251 |
+
frames = []
|
252 |
+
joint_pairs = [[0, 1], [1, 2], [2, 3], [0, 4], [4, 5], [5, 6], [0, 7], [7, 8], [8, 9], [8, 11], [8, 14], [9, 10], [11, 12], [12, 13], [14, 15], [15, 16]]
|
253 |
+
joint_pairs_left = [[8, 11], [11, 12], [12, 13], [0, 4], [4, 5], [5, 6]]
|
254 |
+
joint_pairs_right = [[8, 14], [14, 15], [15, 16], [0, 1], [1, 2], [2, 3]]
|
255 |
+
|
256 |
+
color_mid = "#00457E"
|
257 |
+
color_left = "#02315E"
|
258 |
+
color_right = "#2F70AF"
|
259 |
+
for f in tqdm(range(vlen)):
|
260 |
+
j3d = motion[:,:,f]
|
261 |
+
fig = plt.figure(0, figsize=(10, 10))
|
262 |
+
ax = plt.axes(projection="3d")
|
263 |
+
ax.set_xlim(-512, 0)
|
264 |
+
ax.set_ylim(-256, 256)
|
265 |
+
ax.set_zlim(-512, 0)
|
266 |
+
# ax.set_xlabel('X')
|
267 |
+
# ax.set_ylabel('Y')
|
268 |
+
# ax.set_zlabel('Z')
|
269 |
+
ax.view_init(elev=12., azim=80)
|
270 |
+
plt.tick_params(left = False, right = False , labelleft = False ,
|
271 |
+
labelbottom = False, bottom = False)
|
272 |
+
for i in range(len(joint_pairs)):
|
273 |
+
limb = joint_pairs[i]
|
274 |
+
xs, ys, zs = [np.array([j3d[limb[0], j], j3d[limb[1], j]]) for j in range(3)]
|
275 |
+
if joint_pairs[i] in joint_pairs_left:
|
276 |
+
ax.plot(-xs, -zs, -ys, color=color_left, lw=3, marker='o', markerfacecolor='w', markersize=3, markeredgewidth=2) # axis transformation for visualization
|
277 |
+
elif joint_pairs[i] in joint_pairs_right:
|
278 |
+
ax.plot(-xs, -zs, -ys, color=color_right, lw=3, marker='o', markerfacecolor='w', markersize=3, markeredgewidth=2) # axis transformation for visualization
|
279 |
+
else:
|
280 |
+
ax.plot(-xs, -zs, -ys, color=color_mid, lw=3, marker='o', markerfacecolor='w', markersize=3, markeredgewidth=2) # axis transformation for visualization
|
281 |
+
|
282 |
+
frame_vis = get_img_from_fig(fig)
|
283 |
+
videowriter.append_data(frame_vis)
|
284 |
+
plt.close()
|
285 |
+
videowriter.close()
|
286 |
+
|
287 |
+
def motion2video_mesh(motion, save_path, fps=25, keep_imgs = False, draw_face=True):
|
288 |
+
videowriter = imageio.get_writer(save_path, fps=fps)
|
289 |
+
vlen = motion.shape[-1]
|
290 |
+
draw_skele = (motion.shape[0]==17)
|
291 |
+
save_name = save_path.split('.')[0]
|
292 |
+
smpl_faces = get_smpl_faces()
|
293 |
+
frames = []
|
294 |
+
joint_pairs = [[0, 1], [1, 2], [2, 3], [0, 4], [4, 5], [5, 6], [0, 7], [7, 8], [8, 9], [8, 11], [8, 14], [9, 10], [11, 12], [12, 13], [14, 15], [15, 16]]
|
295 |
+
|
296 |
+
|
297 |
+
X, Y, Z = motion[:, 0], motion[:, 1], motion[:, 2]
|
298 |
+
max_range = np.array([X.max()-X.min(), Y.max()-Y.min(), Z.max()-Z.min()]).max() / 2.0
|
299 |
+
mid_x = (X.max()+X.min()) * 0.5
|
300 |
+
mid_y = (Y.max()+Y.min()) * 0.5
|
301 |
+
mid_z = (Z.max()+Z.min()) * 0.5
|
302 |
+
|
303 |
+
for f in tqdm(range(vlen)):
|
304 |
+
j3d = motion[:,:,f]
|
305 |
+
plt.gca().set_axis_off()
|
306 |
+
plt.subplots_adjust(top=1, bottom=0, right=1, left=0, hspace=0, wspace=0)
|
307 |
+
plt.gca().xaxis.set_major_locator(plt.NullLocator())
|
308 |
+
plt.gca().yaxis.set_major_locator(plt.NullLocator())
|
309 |
+
fig = plt.figure(0, figsize=(8, 8))
|
310 |
+
ax = plt.axes(projection="3d", proj_type = 'ortho')
|
311 |
+
ax.set_xlim(mid_x - max_range, mid_x + max_range)
|
312 |
+
ax.set_ylim(mid_y - max_range, mid_y + max_range)
|
313 |
+
ax.set_zlim(mid_z - max_range, mid_z + max_range)
|
314 |
+
ax.view_init(elev=-90, azim=-90)
|
315 |
+
plt.subplots_adjust(top=1, bottom=0, right=1, left=0, hspace=0, wspace=0)
|
316 |
+
plt.margins(0, 0, 0)
|
317 |
+
plt.gca().xaxis.set_major_locator(plt.NullLocator())
|
318 |
+
plt.gca().yaxis.set_major_locator(plt.NullLocator())
|
319 |
+
plt.axis('off')
|
320 |
+
plt.xticks([])
|
321 |
+
plt.yticks([])
|
322 |
+
|
323 |
+
# plt.savefig("filename.png", transparent=True, bbox_inches="tight", pad_inches=0)
|
324 |
+
|
325 |
+
if draw_skele:
|
326 |
+
for i in range(len(joint_pairs)):
|
327 |
+
limb = joint_pairs[i]
|
328 |
+
xs, ys, zs = [np.array([j3d[limb[0], j], j3d[limb[1], j]]) for j in range(3)]
|
329 |
+
ax.plot(-xs, -zs, -ys, c=[0,0,0], lw=3, marker='o', markerfacecolor='w', markersize=3, markeredgewidth=2) # axis transformation for visualization
|
330 |
+
elif draw_face:
|
331 |
+
ax.plot_trisurf(j3d[:, 0], j3d[:, 1], triangles=smpl_faces, Z=j3d[:, 2], color=(166/255.0,188/255.0,218/255.0,0.9))
|
332 |
+
else:
|
333 |
+
ax.scatter(j3d[:, 0], j3d[:, 1], j3d[:, 2], s=3, c='w', edgecolors='grey')
|
334 |
+
frame_vis = get_img_from_fig(fig, dpi=128)
|
335 |
+
plt.cla()
|
336 |
+
videowriter.append_data(frame_vis)
|
337 |
+
plt.close()
|
338 |
+
videowriter.close()
|
339 |
+
|
340 |
+
def save_image(image_numpy, image_path):
|
341 |
+
image_pil = Image.fromarray(image_numpy)
|
342 |
+
image_pil.save(image_path)
|
343 |
+
|
344 |
+
def bounding_box(img):
|
345 |
+
a = np.where(img != 0)
|
346 |
+
bbox = np.min(a[0]), np.max(a[0]), np.min(a[1]), np.max(a[1])
|
347 |
+
return bbox
|
params/d2c_params.pkl
ADDED
@@ -0,0 +1,3 @@
|
|
|
|
|
|
|
|
|
1 |
+
version https://git-lfs.github.com/spec/v1
|
2 |
+
oid sha256:3b02023c3fc660f4808c735e2f8a9eae1206a411f1ad7e3429d33719da1cd0d1
|
3 |
+
size 184
|
run.sh
CHANGED
@@ -2,4 +2,5 @@
|
|
2 |
CONDA_ENV=$(head -1 /code/environment.yml | cut -d" " -f2)
|
3 |
eval "$(conda shell.bash hook)"
|
4 |
conda activate $CONDA_ENV
|
5 |
-
|
|
|
|
2 |
CONDA_ENV=$(head -1 /code/environment.yml | cut -d" " -f2)
|
3 |
eval "$(conda shell.bash hook)"
|
4 |
conda activate $CONDA_ENV
|
5 |
+
pip install -r requirements.txt
|
6 |
+
python app.py
|
tools/compress_amass.py
ADDED
@@ -0,0 +1,62 @@
|
|
|
|
|
|
|
|
|
|
|
|
|
|
|
|
|
|
|
|
|
|
|
|
|
|
|
|
|
|
|
|
|
|
|
|
|
|
|
|
|
|
|
|
|
|
|
|
|
|
|
|
|
|
|
|
|
|
|
|
|
|
|
|
|
|
|
|
|
|
|
|
|
|
|
|
|
|
|
|
|
|
|
|
|
|
|
|
|
|
|
|
|
|
|
|
|
|
|
|
|
|
|
|
|
|
|
|
|
|
|
|
|
|
|
|
|
|
|
|
|
|
|
|
|
|
|
1 |
+
import numpy as np
|
2 |
+
import os
|
3 |
+
import pickle
|
4 |
+
|
5 |
+
raw_dir = './data/AMASS/amass_202203/'
|
6 |
+
processed_dir = './data/AMASS/amass_fps60'
|
7 |
+
os.makedirs(processed_dir, exist_ok=True)
|
8 |
+
|
9 |
+
files = []
|
10 |
+
length = 0
|
11 |
+
target_fps = 60
|
12 |
+
|
13 |
+
def traverse(f):
|
14 |
+
fs = os.listdir(f)
|
15 |
+
for f1 in fs:
|
16 |
+
tmp_path = os.path.join(f,f1)
|
17 |
+
# file
|
18 |
+
if not os.path.isdir(tmp_path):
|
19 |
+
files.append(tmp_path)
|
20 |
+
# dir
|
21 |
+
else:
|
22 |
+
traverse(tmp_path)
|
23 |
+
|
24 |
+
traverse(raw_dir)
|
25 |
+
|
26 |
+
print('files:', len(files))
|
27 |
+
|
28 |
+
fnames = []
|
29 |
+
all_motions = []
|
30 |
+
|
31 |
+
with open('data/AMASS/fps.csv', 'w') as f:
|
32 |
+
print('fname_new, len_ori, fps, len_new', file=f)
|
33 |
+
for fname in sorted(files):
|
34 |
+
try:
|
35 |
+
raw_x = np.load(fname)
|
36 |
+
x = dict(raw_x)
|
37 |
+
fps = x['mocap_framerate']
|
38 |
+
len_ori = len(x['trans'])
|
39 |
+
sample_stride = round(fps / target_fps)
|
40 |
+
x['mocap_framerate'] = target_fps
|
41 |
+
x['trans'] = x['trans'][::sample_stride]
|
42 |
+
x['dmpls'] = x['dmpls'][::sample_stride]
|
43 |
+
x['poses'] = x['poses'][::sample_stride]
|
44 |
+
fname_new = '_'.join(fname.split('/')[2:])
|
45 |
+
len_new = len(x['trans'])
|
46 |
+
|
47 |
+
length += len_new
|
48 |
+
print(fname_new, ',', len_ori, ',', fps, ',', len_new, file=f)
|
49 |
+
fnames.append(fname_new)
|
50 |
+
all_motions.append(x)
|
51 |
+
np.savez('%s/%s' % (processed_dir, fname_new), x)
|
52 |
+
except:
|
53 |
+
pass
|
54 |
+
|
55 |
+
# break
|
56 |
+
|
57 |
+
print('poseFrame:', length)
|
58 |
+
print('motions:', len(fnames))
|
59 |
+
|
60 |
+
with open("data/AMASS/all_motions_fps%d.pkl" % target_fps, "wb") as myprofile:
|
61 |
+
pickle.dump(all_motions, myprofile)
|
62 |
+
|
tools/convert_amass.py
ADDED
@@ -0,0 +1,67 @@
|
|
|
|
|
|
|
|
|
|
|
|
|
|
|
|
|
|
|
|
|
|
|
|
|
|
|
|
|
|
|
|
|
|
|
|
|
|
|
|
|
|
|
|
|
|
|
|
|
|
|
|
|
|
|
|
|
|
|
|
|
|
|
|
|
|
|
|
|
|
|
|
|
|
|
|
|
|
|
|
|
|
|
|
|
|
|
|
|
|
|
|
|
|
|
|
|
|
|
|
|
|
|
|
|
|
|
|
|
|
|
|
|
|
|
|
|
|
|
|
|
|
|
|
|
|
|
|
|
|
|
|
|
|
|
|
|
1 |
+
import os
|
2 |
+
import sys
|
3 |
+
import copy
|
4 |
+
import pickle
|
5 |
+
import ipdb
|
6 |
+
import torch
|
7 |
+
import numpy as np
|
8 |
+
sys.path.insert(0, os.getcwd())
|
9 |
+
from lib.utils.utils_data import split_clips
|
10 |
+
from tqdm import tqdm
|
11 |
+
|
12 |
+
fileName = open('data/AMASS/amass_joints_h36m_60.pkl','rb')
|
13 |
+
joints_all = pickle.load(fileName)
|
14 |
+
|
15 |
+
joints_cam = []
|
16 |
+
vid_list = []
|
17 |
+
vid_len_list = []
|
18 |
+
scale_factor = 0.298
|
19 |
+
|
20 |
+
for i, item in enumerate(joints_all): # (17,N,3):
|
21 |
+
item = item.astype(np.float32)
|
22 |
+
vid_len = item.shape[1]
|
23 |
+
vid_len_list.append(vid_len)
|
24 |
+
for _ in range(vid_len):
|
25 |
+
vid_list.append(i)
|
26 |
+
real2cam = np.array([[1,0,0],
|
27 |
+
[0,0,1],
|
28 |
+
[0,-1,0]], dtype=np.float32)
|
29 |
+
item = np.transpose(item, (1,0,2)) # (17,N,3) -> (N,17,3)
|
30 |
+
motion_cam = item @ real2cam
|
31 |
+
motion_cam *= scale_factor
|
32 |
+
# motion_cam = motion_cam - motion_cam[0,0,:]
|
33 |
+
joints_cam.append(motion_cam)
|
34 |
+
|
35 |
+
joints_cam_all = np.vstack(joints_cam)
|
36 |
+
split_id = split_clips(vid_list, n_frames=243, data_stride=81)
|
37 |
+
print(joints_cam_all.shape) # (N,17,3)
|
38 |
+
|
39 |
+
max_x, minx_x = np.max(joints_cam_all[:,:,0]), np.min(joints_cam_all[:,:,0])
|
40 |
+
max_y, minx_y = np.max(joints_cam_all[:,:,1]), np.min(joints_cam_all[:,:,1])
|
41 |
+
max_z, minx_z = np.max(joints_cam_all[:,:,2]), np.min(joints_cam_all[:,:,2])
|
42 |
+
print(max_x, minx_x)
|
43 |
+
print(max_y, minx_y)
|
44 |
+
print(max_z, minx_z)
|
45 |
+
|
46 |
+
joints_cam_clip = joints_cam_all[split_id]
|
47 |
+
print(joints_cam_clip.shape) # (N,27,17,3)
|
48 |
+
|
49 |
+
# np.save('doodle/joints_cam_clip_amass_60.npy', joints_cam_clip)
|
50 |
+
|
51 |
+
root_path = "data/motion3d/MB3D_f243s81/AMASS"
|
52 |
+
subset_name = "train"
|
53 |
+
save_path = os.path.join(root_path, subset_name)
|
54 |
+
if not os.path.exists(save_path):
|
55 |
+
os.makedirs(save_path)
|
56 |
+
|
57 |
+
num_clips = len(joints_cam_clip)
|
58 |
+
for i in tqdm(range(num_clips)):
|
59 |
+
motion = joints_cam_clip[i]
|
60 |
+
data_dict = {
|
61 |
+
"data_input": None,
|
62 |
+
"data_label": motion
|
63 |
+
}
|
64 |
+
with open(os.path.join(save_path, "%08d.pkl" % i), "wb") as myprofile:
|
65 |
+
pickle.dump(data_dict, myprofile)
|
66 |
+
|
67 |
+
|