File size: 13,528 Bytes
60adc6f 34e59f1 60adc6f fc74cbd 60adc6f fc74cbd 60adc6f fc74cbd 60adc6f fc74cbd 60adc6f fc74cbd 60adc6f fc74cbd c927559 fc74cbd c927559 fc74cbd c927559 fc74cbd 60adc6f fc74cbd 60adc6f fc74cbd 60adc6f fc74cbd 60adc6f fc74cbd 60adc6f fc74cbd 60adc6f fc74cbd 60adc6f 4bd8b0b 60adc6f 4bd8b0b 60adc6f fc74cbd 60adc6f fc74cbd 60adc6f fc74cbd 60adc6f cbd9c2f 60adc6f fc74cbd |
1 2 3 4 5 6 7 8 9 10 11 12 13 14 15 16 17 18 19 20 21 22 23 24 25 26 27 28 29 30 31 32 33 34 35 36 37 38 39 40 41 42 43 44 45 46 47 48 49 50 51 52 53 54 55 56 57 58 59 60 61 62 63 64 65 66 67 68 69 70 71 72 73 74 75 76 77 78 79 80 81 82 83 84 85 86 87 88 89 90 91 92 93 94 95 96 97 98 99 100 101 102 103 104 105 106 107 108 109 110 111 112 113 114 115 116 117 118 119 120 121 122 123 124 125 126 127 128 129 130 131 132 133 134 135 136 137 138 139 140 141 142 143 144 145 146 147 148 149 150 151 152 153 154 155 156 157 158 159 160 161 162 163 164 165 166 167 168 169 170 171 172 173 174 175 176 177 178 179 180 181 182 183 184 185 186 187 188 189 190 191 192 193 194 195 196 197 198 199 200 201 202 203 204 205 206 207 208 209 210 211 212 213 214 215 216 217 218 219 220 221 222 223 224 225 226 227 228 229 230 231 232 233 234 235 236 237 238 239 240 241 242 243 244 245 246 247 248 249 250 251 252 253 254 255 256 257 258 259 260 261 262 263 264 265 266 267 268 269 270 271 272 273 274 275 276 277 278 279 280 281 282 283 284 285 286 287 288 289 290 291 292 293 294 295 296 297 298 299 300 301 302 303 304 305 306 307 308 309 310 311 312 313 314 315 316 317 318 319 320 321 322 323 324 325 326 327 328 329 330 331 332 333 334 335 336 337 338 339 340 341 342 343 344 345 346 347 348 349 350 351 352 353 354 355 356 357 358 359 360 361 362 363 364 365 366 367 368 369 370 371 372 373 374 375 376 377 378 379 380 381 382 383 384 385 386 387 388 389 390 391 392 393 394 395 396 397 398 399 400 401 402 403 404 405 406 407 408 409 410 411 412 413 414 415 416 417 418 419 420 421 422 423 424 425 426 427 428 429 430 431 432 433 434 435 436 437 438 439 440 441 442 443 444 445 446 447 448 449 450 451 452 453 454 455 456 457 458 459 460 461 462 463 464 465 466 467 468 469 470 471 472 473 474 475 476 477 478 479 480 481 482 483 484 485 486 487 488 |
---
datasets:
- danjacobellis/LSDIR_540
- danjacobellis/musdb_segments
---
# Wavelet Learned Lossy Compression
- [Project page and documentation](https://danjacobellis.net/walloc)
- [Paper: "Learned Compression for Compressed Learning"](https://arxiv.org/abs/2412.09405)
- [Additional code accompanying the paper](https://github.com/danjacobellis/lccl)
WaLLoC (Wavelet-Domain Learned Lossy Compression) is an architecture for learned compression that simultaneously satisfies three key
requirements of compressed-domain learning:
1. **Computationally efficient encoding** to reduce overhead in compressed-domain learning and support resource constrained mobile and remote sensors. WaLLoC uses a wavelet packet transform to expose signal redundancies prior to autoencoding. This allows us to replace the encoding DNN with a single linear layer (<100k parameters) without significant loss in quality. WaLLoC incurs <5% of the encoding cost compared to other neural codecs.
2. **High compression ratio** for storage and transmission efficiency. Lossy codecs typically achieve high compression with a combination of quantization and entropy coding. However, naive quantization of autoencoder latents leads to unpredictable and unbounded distortion. Instead, we apply additive noise during training as an
entropy bottleneck, leading to quantization-resiliant latents. When combined with entropy coding, this provides nearly 12× higher compression ratio compared to the VAE used in Stable Diffusion 3, despite offering a higher degree of dimensionality reduction and similar quality.
3. **Dimensionality reduction** to accelerate compressed-domain modeling. WaLLoC’s encoder projects high-dimensional signal patches to low-dimensional latent representations, providing a reduction of up to 20×. This allows WaLLoC to be used as a drop-in replacement for resolution reduction while providing superior detail preservation and downstream accuracy.
WaLLoC does not require perceptual or adversarial losses to represent high-frequency detail, making it compatible with a wide variety of signal types. It currently supports 1D and 2D signals, including mono, stereo, and multi-channel audio and grayscale, RGB, and hyperspectral images.

WaLLoC’s encode-decode pipeline. The entropy bottleneck and entropy coding steps are only required to achieve high compression ratios for storage and transmission. For compressed-domain learning where dimensionality reduction is the primary goal, these steps can be skipped to reduce overhead and completely eliminate CPU-GPU transfers.

Comparison of WaLLoC with other autoencoder designs for RGB Images and stereo audio.




```
@inproceedings{jacobellis2024learned,
title={Learned Compression for Compressed Learning},
author={Jacobellis, Dan and Yadwadkar, Neeraja J.},
booktitle={IEEE Data Compression Conference (DCC)},
note={Preprint}
year={2024},
url={http://danjacobellis.net/walloc}
}
```
## Installation
1. Follow the installation instructions for [torch](https://pytorch.org/get-started/locally/)
2. Install WaLLoC and other dependencies via pip
```pip install walloc PyWavelets pytorch-wavelets```
## Image compression
```python
import os
import torch
import json
import matplotlib.pyplot as plt
import numpy as np
from types import SimpleNamespace
from PIL import Image, ImageEnhance
from IPython.display import display
from torchvision.transforms import ToPILImage, PILToTensor
from walloc import walloc
from walloc.walloc import latent_to_pil, pil_to_latent
```
### Load the model from a pre-trained checkpoint
```wget https://hf.co/danjacobellis/walloc/resolve/main/RGB_16x.pth```
```wget https://hf.co/danjacobellis/walloc/resolve/main/RGB_16x.json```
```python
device = "cpu"
codec_config = SimpleNamespace(**json.load(open("RGB_16x.json")))
checkpoint = torch.load("RGB_16x.pth",map_location="cpu",weights_only=False)
codec = walloc.Codec2D(
channels = codec_config.channels,
J = codec_config.J,
Ne = codec_config.Ne,
Nd = codec_config.Nd,
latent_dim = codec_config.latent_dim,
latent_bits = codec_config.latent_bits,
lightweight_encode = codec_config.lightweight_encode
)
codec.load_state_dict(checkpoint['model_state_dict'])
codec = codec.to(device)
codec.eval();
```
### Load an example image
```wget "https://r0k.us/graphics/kodak/kodak/kodim05.png"```
```python
img = Image.open("kodim05.png")
img
```

### Full encoding and decoding pipeline with .forward()
* If `codec.eval()` is called, the latent is rounded to nearest integer.
* If `codec.train()` is called, uniform noise is added instead of rounding.
```python
with torch.no_grad():
codec.eval()
x = PILToTensor()(img).to(torch.float)
x = (x/255 - 0.5).unsqueeze(0).to(device)
x_hat, _, _ = codec(x)
ToPILImage()(x_hat[0]+0.5)
```
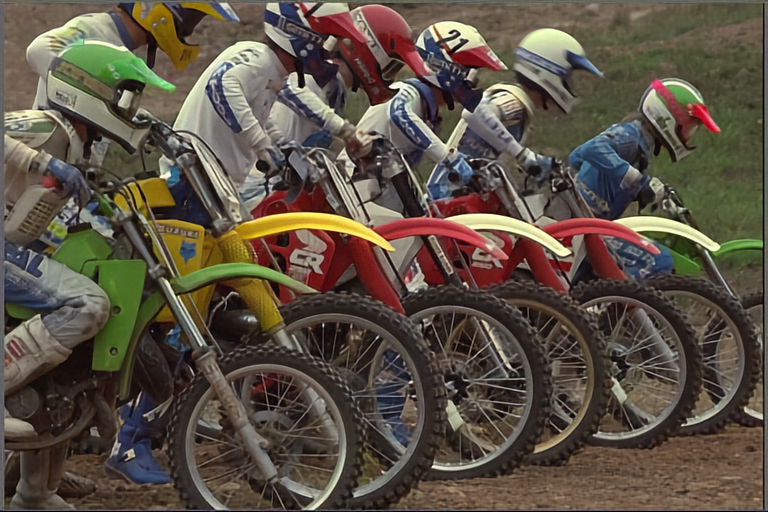
### Accessing latents
```python
with torch.no_grad():
X = codec.wavelet_analysis(x,J=codec.J)
z = codec.encoder[0:2](X)
z_hat = codec.encoder[2](z)
X_hat = codec.decoder(z_hat)
x_rec = codec.wavelet_synthesis(X_hat,J=codec.J)
print(f"dimensionality reduction: {x.numel()/z.numel()}×")
```
dimensionality reduction: 16.0×
```python
plt.figure(figsize=(5,3),dpi=150)
plt.hist(
z.flatten().numpy(),
range=(-25,25),
bins=151,
density=True,
);
plt.title("Histogram of latents")
plt.xlim([-25,25]);
```

# Lossless compression of latents
```python
def scale_for_display(img, n_bits):
scale_factor = (2**8 - 1) / (2**n_bits - 1)
lut = [int(i * scale_factor) for i in range(2**n_bits)]
channels = img.split()
scaled_channels = [ch.point(lut * 2**(8-n_bits)) for ch in channels]
return Image.merge(img.mode, scaled_channels)
```
### Single channel PNG (L)
```python
z_padded = torch.nn.functional.pad(z_hat, (0, 0, 0, 0, 0, 4))
z_pil = latent_to_pil(z_padded,codec.latent_bits,1)
display(scale_for_display(z_pil[0], codec.latent_bits))
z_pil[0].save('latent.png')
png = [Image.open("latent.png")]
z_rec = pil_to_latent(png,16,codec.latent_bits,1)
assert(z_rec.equal(z_padded))
print("compression_ratio: ", x.numel()/os.path.getsize("latent.png"))
```

compression_ratio: 26.729991842653856
### Three channel WebP (RGB)
```python
z_pil = latent_to_pil(z_hat,codec.latent_bits,3)
display(scale_for_display(z_pil[0], codec.latent_bits))
z_pil[0].save('latent.webp',lossless=True)
webp = [Image.open("latent.webp")]
z_rec = pil_to_latent(webp,12,codec.latent_bits,3)
assert(z_rec.equal(z_hat))
print("compression_ratio: ", x.numel()/os.path.getsize("latent.webp"))
```
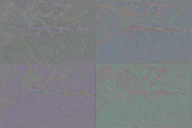
compression_ratio: 28.811254396248536
### Four channel TIF (CMYK)
```python
z_padded = torch.nn.functional.pad(z_hat, (0, 0, 0, 0, 0, 4))
z_pil = latent_to_pil(z_padded,codec.latent_bits,4)
display(scale_for_display(z_pil[0], codec.latent_bits))
z_pil[0].save('latent.tif',compression="tiff_adobe_deflate")
tif = [Image.open("latent.tif")]
z_rec = pil_to_latent(tif,16,codec.latent_bits,4)
assert(z_rec.equal(z_padded))
print("compression_ratio: ", x.numel()/os.path.getsize("latent.tif"))
```

compression_ratio: 21.04034530731638
# Audio Compression
```python
import io
import os
import torch
import torchaudio
import json
import matplotlib.pyplot as plt
from types import SimpleNamespace
from PIL import Image
from datasets import load_dataset
from einops import rearrange
from IPython.display import Audio
from walloc import walloc
```
### Load the model from a pre-trained checkpoint
```wget https://hf.co/danjacobellis/walloc/resolve/main/stereo_5x.pth```
```wget https://hf.co/danjacobellis/walloc/resolve/main/stereo_5x.json```
```python
codec_config = SimpleNamespace(**json.load(open("stereo_5x.json")))
checkpoint = torch.load("stereo_5x.pth",map_location="cpu",weights_only=False)
codec = walloc.Codec1D(
channels = codec_config.channels,
J = codec_config.J,
Ne = codec_config.Ne,
Nd = codec_config.Nd,
latent_dim = codec_config.latent_dim,
latent_bits = codec_config.latent_bits,
lightweight_encode = codec_config.lightweight_encode,
post_filter = codec_config.post_filter
)
codec.load_state_dict(checkpoint['model_state_dict'])
codec.eval();
```
/home/dan/g/lib/python3.12/site-packages/torch/nn/utils/weight_norm.py:143: FutureWarning: `torch.nn.utils.weight_norm` is deprecated in favor of `torch.nn.utils.parametrizations.weight_norm`.
WeightNorm.apply(module, name, dim)
### Load example audio track
```python
MUSDB = load_dataset("danjacobellis/musdb_segments_val",split='validation')
audio_buff = io.BytesIO(MUSDB[40]['audio_mix']['bytes'])
x, fs = torchaudio.load(audio_buff,normalize=False)
x = x.to(torch.float)
x = x - x.mean()
max_abs = x.abs().max()
x = x / (max_abs + 1e-8)
x = x/2
```
```python
Audio(x[:,:2**20],rate=44100)
```
<audio controls>
<source src="https://huggingface.co/danjacobellis/walloc/resolve/main/README_files/README_0.wav" type="audio/wav">
</audio>
### Full encoding and decoding pipeline with .forward()
* If `codec.eval()` is called, the latent is rounded to nearest integer.
* If `codec.train()` is called, uniform noise is added instead of rounding.
```python
with torch.no_grad():
codec.eval()
x_hat, _, _ = codec(x.unsqueeze(0))
```
```python
Audio(x_hat[0,:,:2**20],rate=44100)
```
<audio controls>
<source src="https://huggingface.co/danjacobellis/walloc/resolve/main/README_files/README_1.wav" type="audio/wav">
</audio>
### Accessing latents
```python
with torch.no_grad():
X = codec.wavelet_analysis(x.unsqueeze(0),J=codec.J)
z = codec.encoder[0:2](X)
z_hat = codec.encoder[2](z)
X_hat = codec.decoder(z_hat)
x_rec = codec.wavelet_synthesis(X_hat,J=codec.J)
print(f"dimensionality reduction: {x.numel()/z.numel():.4g}×")
```
dimensionality reduction: 4.74×
```python
plt.figure(figsize=(5,3),dpi=150)
plt.hist(
z.flatten().numpy(),
range=(-25,25),
bins=151,
density=True,
);
plt.title("Histogram of latents")
plt.xlim([-25,25]);
```

# Lossless compression of latents
```python
def pad(audio, p=2**16):
B,C,L = audio.shape
padding_size = (p - (L % p)) % p
if padding_size > 0:
audio = torch.nn.functional.pad(audio, (0, padding_size), mode='constant', value=0)
return audio
with torch.no_grad():
L = x.shape[-1]
x_padded = pad(x.unsqueeze(0), 2**16)
X = codec.wavelet_analysis(x_padded,codec.J)
z = codec.encoder(X)
ℓ = z.shape[-1]
z = pad(z,128)
z = rearrange(z, 'b c (w h) -> b c w h', h=128).to("cpu")
webp = walloc.latent_to_pil(z,codec.latent_bits,3)[0]
buff = io.BytesIO()
webp.save(buff, format='WEBP', lossless=True)
webp_bytes = buff.getbuffer()
```
```python
print("compression_ratio: ", x.numel()/len(webp_bytes))
webp
```
compression_ratio: 9.83650170496386
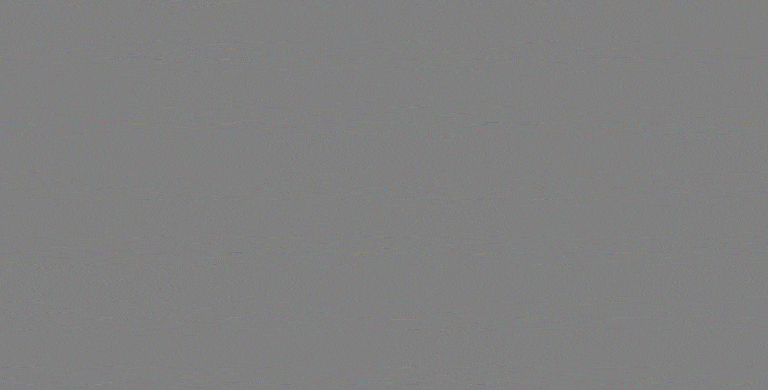
# Decoding
```python
with torch.no_grad():
z_hat = walloc.pil_to_latent(
[Image.open(buff)],
codec.latent_dim,
codec.latent_bits,
3)
X_hat = codec.decoder(rearrange(z_hat, 'b c h w -> b c (h w)')[:,:,:ℓ])
x_hat = codec.wavelet_synthesis(X_hat,codec.J)
x_hat = codec.post(x_hat)
x_hat = codec.clamp(x_hat[0,:,:L])
```
```python
start, end = 0, 1000
plt.figure(figsize=(8, 3), dpi=180)
plt.plot(x[0, start:end], alpha=0.5, c='b', label='Ch.1 (Uncompressed)')
plt.plot(x_hat[0, start:end], alpha=0.5, c='g', label='Ch.1 (WaLLoC)')
plt.plot(x[1, start:end], alpha=0.5, c='r', label='Ch.2 (Uncompressed)')
plt.plot(x_hat[1, start:end], alpha=0.5, c='purple', label='Ch.2 (WaLLoC)')
plt.xlim([400,1000])
plt.ylim([-0.6,0.3])
plt.legend(loc='lower center')
plt.box(False)
plt.xticks([])
plt.yticks([]);
```
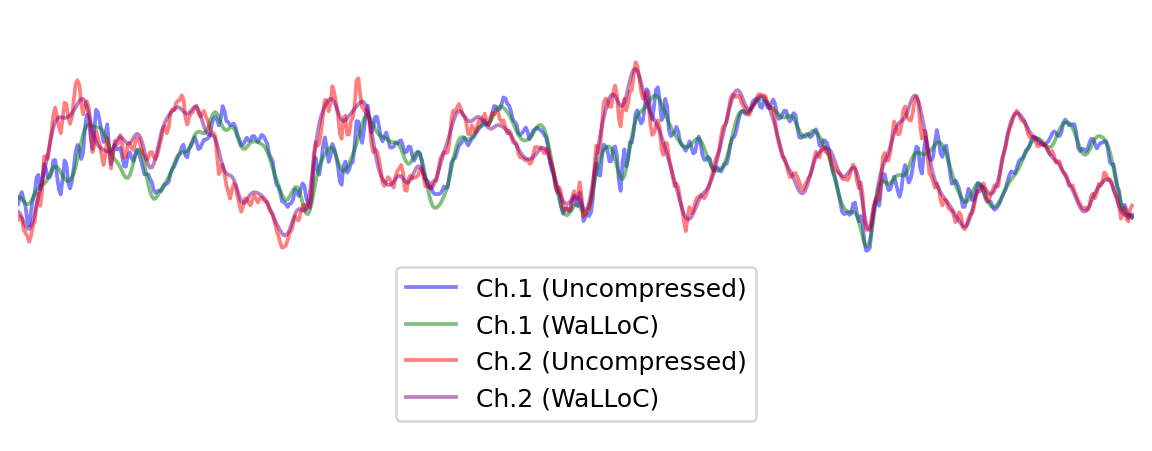
```python
!jupyter nbconvert --to markdown README.ipynb
```
[NbConvertApp] Converting notebook README.ipynb to markdown
[NbConvertApp] Support files will be in README_files/
[NbConvertApp] Writing 12900 bytes to README.md
```python
!sed -i 's|!\[png](README_files/\(README_[0-9]*_[0-9]*\.png\))||g' README.md
```
```python
!sed -i 's|src="README_files/\(README_[0-9]*\.wav\)"|src="https://huggingface.co/danjacobellis/walloc/resolve/main/README_files/\1"|g' README.md
```
|