|
--- |
|
datasets: |
|
- danjacobellis/LSDIR_540 |
|
- danjacobellis/musdb_segments |
|
--- |
|
# Wavelet Learned Lossy Compression |
|
|
|
- [Project page and documentation](https://danjacobellis.net/walloc) |
|
- [Paper: "Learned Compression for Compressed Learning"](https://danjacobellis.net/_static/walloc.pdf) |
|
- [Additional code accompanying the paper](https://github.com/danjacobellis/lccl) |
|
|
|
WaLLoC (Wavelet-Domain Learned Lossy Compression) is an architecture for learned compression that simultaneously satisfies three key |
|
requirements of compressed-domain learning: |
|
|
|
1. **Computationally efficient encoding** to reduce overhead in compressed-domain learning and support resource constrained mobile and remote sensors. WaLLoC uses a wavelet packet transform to expose signal redundancies prior to autoencoding. This allows us to replace the encoding DNN with a single linear layer (<100k parameters) without significant loss in quality. WaLLoC incurs <5% of the encoding cost compared to other neural codecs. |
|
|
|
2. **High compression ratio** for storage and transmission efficiency. Lossy codecs typically achieve high compression with a combination of quantization and entropy coding. However, naive quantization of autoencoder latents leads to unpredictable and unbounded distortion. Instead, we apply additive noise during training as an |
|
entropy bottleneck, leading to quantization-resiliant latents. When combined with entropy coding, this provides nearly 12× higher compression ratio compared to the VAE used in Stable Diffusion 3, despite offering a higher degree of dimensionality reduction and similar quality. |
|
|
|
3. **Dimensionality reduction** to accelerate compressed-domain modeling. WaLLoC’s encoder projects high-dimensional signal patches to low-dimensional latent representations, providing a reduction of up to 20×. This allows WaLLoC to be used as a drop-in replacement for resolution reduction while providing superior detail preservation and downstream accuracy. |
|
|
|
WaLLoC does not require perceptual or adversarial losses to represent high-frequency detail, making it compatible with a wide variety of signal types. It currently supports 1D and 2D signals, including mono, stereo, and multi-channel audio and grayscale, RGB, and hyperspectral images. |
|
|
|
 |
|
WaLLoC’s encode-decode pipeline. The entropy bottleneck and entropy coding steps are only required to achieve high compression ratios for storage and transmission. For compressed-domain learning where dimensionality reduction is the primary goal, these steps can be skipped to reduce overhead and completely eliminate CPU-GPU transfers. |
|
|
|
|
|
|
|
 |
|
Comparison of WaLLoC with other autoencoder designs for RGB Images and stereo audio. |
|
|
|
|
|
|
|
 |
|
|
|
 |
|
|
|
 |
|
|
|
 |
|
|
|
``` |
|
@inproceedings{jacobellis2024learned, |
|
title={Learned Compression for Compressed Learning}, |
|
author={Jacobellis, Dan and Yadwadkar, Neeraja J.}, |
|
booktitle={IEEE Data Compression Conference (DCC)}, |
|
note={Preprint} |
|
year={2024}, |
|
url={http://danjacobellis.net/walloc} |
|
} |
|
|
|
``` |
|
|
|
## Installation |
|
|
|
1. Follow the installation instructions for [torch](https://pytorch.org/get-started/locally/) |
|
2. Install WaLLoC and other dependencies via pip |
|
|
|
```pip install walloc PyWavelets pytorch-wavelets``` |
|
|
|
## Image compression |
|
|
|
|
|
```python |
|
import os |
|
import torch |
|
import json |
|
import matplotlib.pyplot as plt |
|
import numpy as np |
|
from types import SimpleNamespace |
|
from PIL import Image, ImageEnhance |
|
from IPython.display import display |
|
from torchvision.transforms import ToPILImage, PILToTensor |
|
from walloc import walloc |
|
from walloc.walloc import latent_to_pil, pil_to_latent |
|
``` |
|
|
|
### Load the model from a pre-trained checkpoint |
|
|
|
```wget https://hf.co/danjacobellis/walloc/resolve/main/RGB_16x.pth``` |
|
|
|
```wget https://hf.co/danjacobellis/walloc/resolve/main/RGB_16x.json``` |
|
|
|
|
|
```python |
|
device = "cpu" |
|
codec_config = SimpleNamespace(**json.load(open("RGB_16x.json"))) |
|
checkpoint = torch.load("RGB_16x.pth",map_location="cpu",weights_only=False) |
|
codec = walloc.Codec2D( |
|
channels = codec_config.channels, |
|
J = codec_config.J, |
|
Ne = codec_config.Ne, |
|
Nd = codec_config.Nd, |
|
latent_dim = codec_config.latent_dim, |
|
latent_bits = codec_config.latent_bits, |
|
lightweight_encode = codec_config.lightweight_encode |
|
) |
|
codec.load_state_dict(checkpoint['model_state_dict']) |
|
codec = codec.to(device) |
|
codec.eval(); |
|
``` |
|
|
|
### Load an example image |
|
|
|
```wget "https://r0k.us/graphics/kodak/kodak/kodim05.png"``` |
|
|
|
|
|
```python |
|
img = Image.open("kodim05.png") |
|
img |
|
``` |
|
|
|
|
|
|
|
|
|
|
|
 |
|
|
|
|
|
|
|
|
|
### Full encoding and decoding pipeline with .forward() |
|
|
|
* If `codec.eval()` is called, the latent is rounded to nearest integer. |
|
|
|
* If `codec.train()` is called, uniform noise is added instead of rounding. |
|
|
|
|
|
```python |
|
with torch.no_grad(): |
|
codec.eval() |
|
x = PILToTensor()(img).to(torch.float) |
|
x = (x/255 - 0.5).unsqueeze(0).to(device) |
|
x_hat, _, _ = codec(x) |
|
ToPILImage()(x_hat[0]+0.5) |
|
``` |
|
|
|
|
|
|
|
|
|
|
|
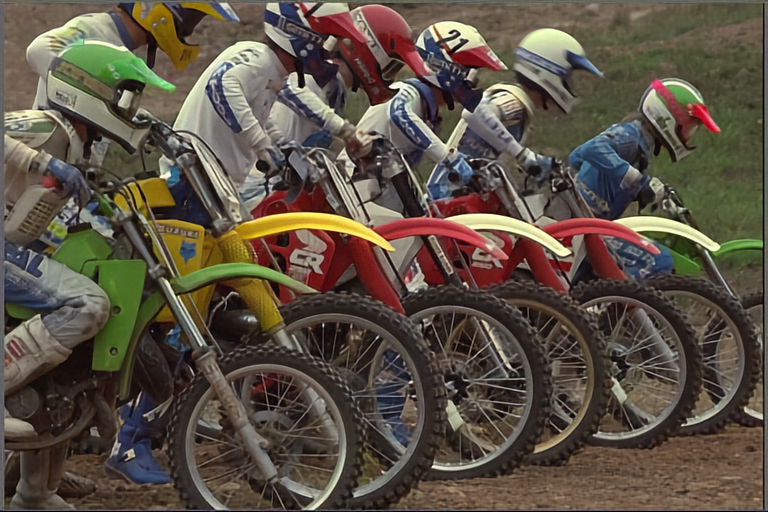 |
|
|
|
|
|
|
|
|
|
### Accessing latents |
|
|
|
|
|
```python |
|
with torch.no_grad(): |
|
X = codec.wavelet_analysis(x,J=codec.J) |
|
z = codec.encoder[0:2](X) |
|
z_hat = codec.encoder[2](z) |
|
X_hat = codec.decoder(z_hat) |
|
x_rec = codec.wavelet_synthesis(X_hat,J=codec.J) |
|
print(f"dimensionality reduction: {x.numel()/z.numel()}×") |
|
``` |
|
|
|
dimensionality reduction: 16.0× |
|
|
|
|
|
|
|
```python |
|
plt.figure(figsize=(5,3),dpi=150) |
|
plt.hist( |
|
z.flatten().numpy(), |
|
range=(-25,25), |
|
bins=151, |
|
density=True, |
|
); |
|
plt.title("Histogram of latents") |
|
plt.xlim([-25,25]); |
|
``` |
|
|
|
|
|
|
|
 |
|
|
|
|
|
|
|
# Lossless compression of latents |
|
|
|
|
|
```python |
|
def scale_for_display(img, n_bits): |
|
scale_factor = (2**8 - 1) / (2**n_bits - 1) |
|
lut = [int(i * scale_factor) for i in range(2**n_bits)] |
|
channels = img.split() |
|
scaled_channels = [ch.point(lut * 2**(8-n_bits)) for ch in channels] |
|
return Image.merge(img.mode, scaled_channels) |
|
``` |
|
|
|
### Single channel PNG (L) |
|
|
|
|
|
```python |
|
z_padded = torch.nn.functional.pad(z_hat, (0, 0, 0, 0, 0, 4)) |
|
z_pil = latent_to_pil(z_padded,codec.latent_bits,1) |
|
display(scale_for_display(z_pil[0], codec.latent_bits)) |
|
z_pil[0].save('latent.png') |
|
png = [Image.open("latent.png")] |
|
z_rec = pil_to_latent(png,16,codec.latent_bits,1) |
|
assert(z_rec.equal(z_padded)) |
|
print("compression_ratio: ", x.numel()/os.path.getsize("latent.png")) |
|
``` |
|
|
|
|
|
|
|
 |
|
|
|
|
|
|
|
compression_ratio: 26.729991842653856 |
|
|
|
|
|
### Three channel WebP (RGB) |
|
|
|
|
|
```python |
|
z_pil = latent_to_pil(z_hat,codec.latent_bits,3) |
|
display(scale_for_display(z_pil[0], codec.latent_bits)) |
|
z_pil[0].save('latent.webp',lossless=True) |
|
webp = [Image.open("latent.webp")] |
|
z_rec = pil_to_latent(webp,12,codec.latent_bits,3) |
|
assert(z_rec.equal(z_hat)) |
|
print("compression_ratio: ", x.numel()/os.path.getsize("latent.webp")) |
|
``` |
|
|
|
|
|
|
|
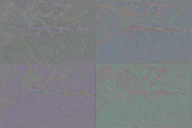 |
|
|
|
|
|
|
|
compression_ratio: 28.811254396248536 |
|
|
|
|
|
### Four channel TIF (CMYK) |
|
|
|
|
|
```python |
|
z_padded = torch.nn.functional.pad(z_hat, (0, 0, 0, 0, 0, 4)) |
|
z_pil = latent_to_pil(z_padded,codec.latent_bits,4) |
|
display(scale_for_display(z_pil[0], codec.latent_bits)) |
|
z_pil[0].save('latent.tif',compression="tiff_adobe_deflate") |
|
tif = [Image.open("latent.tif")] |
|
z_rec = pil_to_latent(tif,16,codec.latent_bits,4) |
|
assert(z_rec.equal(z_padded)) |
|
print("compression_ratio: ", x.numel()/os.path.getsize("latent.tif")) |
|
``` |
|
|
|
|
|
|
|
 |
|
|
|
|
|
|
|
compression_ratio: 21.04034530731638 |
|
|
|
|
|
# Audio Compression |
|
|
|
|
|
```python |
|
import io |
|
import os |
|
import torch |
|
import torchaudio |
|
import json |
|
import matplotlib.pyplot as plt |
|
from types import SimpleNamespace |
|
from PIL import Image |
|
from datasets import load_dataset |
|
from einops import rearrange |
|
from IPython.display import Audio |
|
from walloc import walloc |
|
``` |
|
|
|
### Load the model from a pre-trained checkpoint |
|
|
|
```wget https://hf.co/danjacobellis/walloc/resolve/main/stereo_5x.pth``` |
|
|
|
```wget https://hf.co/danjacobellis/walloc/resolve/main/stereo_5x.json``` |
|
|
|
|
|
```python |
|
codec_config = SimpleNamespace(**json.load(open("stereo_5x.json"))) |
|
checkpoint = torch.load("stereo_5x.pth",map_location="cpu",weights_only=False) |
|
codec = walloc.Codec1D( |
|
channels = codec_config.channels, |
|
J = codec_config.J, |
|
Ne = codec_config.Ne, |
|
Nd = codec_config.Nd, |
|
latent_dim = codec_config.latent_dim, |
|
latent_bits = codec_config.latent_bits, |
|
lightweight_encode = codec_config.lightweight_encode, |
|
post_filter = codec_config.post_filter |
|
) |
|
codec.load_state_dict(checkpoint['model_state_dict']) |
|
codec.eval(); |
|
``` |
|
|
|
/home/dan/g/lib/python3.12/site-packages/torch/nn/utils/weight_norm.py:143: FutureWarning: `torch.nn.utils.weight_norm` is deprecated in favor of `torch.nn.utils.parametrizations.weight_norm`. |
|
WeightNorm.apply(module, name, dim) |
|
|
|
|
|
### Load example audio track |
|
|
|
|
|
```python |
|
MUSDB = load_dataset("danjacobellis/musdb_segments_val",split='validation') |
|
audio_buff = io.BytesIO(MUSDB[40]['audio_mix']['bytes']) |
|
x, fs = torchaudio.load(audio_buff,normalize=False) |
|
x = x.to(torch.float) |
|
x = x - x.mean() |
|
max_abs = x.abs().max() |
|
x = x / (max_abs + 1e-8) |
|
x = x/2 |
|
``` |
|
|
|
|
|
```python |
|
Audio(x[:,:2**20],rate=44100) |
|
``` |
|
|
|
<audio controls> |
|
<source src="https://huggingface.co/danjacobellis/walloc/resolve/main/README_files/README_0.wav" type="audio/wav"> |
|
</audio> |
|
|
|
### Full encoding and decoding pipeline with .forward() |
|
|
|
* If `codec.eval()` is called, the latent is rounded to nearest integer. |
|
|
|
* If `codec.train()` is called, uniform noise is added instead of rounding. |
|
|
|
|
|
```python |
|
with torch.no_grad(): |
|
codec.eval() |
|
x_hat, _, _ = codec(x.unsqueeze(0)) |
|
``` |
|
|
|
|
|
```python |
|
Audio(x_hat[0,:,:2**20],rate=44100) |
|
``` |
|
|
|
<audio controls> |
|
<source src="https://huggingface.co/danjacobellis/walloc/resolve/main/README_files/README_1.wav" type="audio/wav"> |
|
</audio> |
|
|
|
### Accessing latents |
|
|
|
|
|
```python |
|
with torch.no_grad(): |
|
X = codec.wavelet_analysis(x.unsqueeze(0),J=codec.J) |
|
z = codec.encoder[0:2](X) |
|
z_hat = codec.encoder[2](z) |
|
X_hat = codec.decoder(z_hat) |
|
x_rec = codec.wavelet_synthesis(X_hat,J=codec.J) |
|
print(f"dimensionality reduction: {x.numel()/z.numel():.4g}×") |
|
``` |
|
|
|
dimensionality reduction: 4.74× |
|
|
|
|
|
|
|
```python |
|
plt.figure(figsize=(5,3),dpi=150) |
|
plt.hist( |
|
z.flatten().numpy(), |
|
range=(-25,25), |
|
bins=151, |
|
density=True, |
|
); |
|
plt.title("Histogram of latents") |
|
plt.xlim([-25,25]); |
|
``` |
|
|
|
|
|
|
|
 |
|
|
|
|
|
|
|
# Lossless compression of latents |
|
|
|
|
|
```python |
|
def pad(audio, p=2**16): |
|
B,C,L = audio.shape |
|
padding_size = (p - (L % p)) % p |
|
if padding_size > 0: |
|
audio = torch.nn.functional.pad(audio, (0, padding_size), mode='constant', value=0) |
|
return audio |
|
with torch.no_grad(): |
|
L = x.shape[-1] |
|
x_padded = pad(x.unsqueeze(0), 2**16) |
|
X = codec.wavelet_analysis(x_padded,codec.J) |
|
z = codec.encoder(X) |
|
ℓ = z.shape[-1] |
|
z = pad(z,128) |
|
z = rearrange(z, 'b c (w h) -> b c w h', h=128).to("cpu") |
|
webp = walloc.latent_to_pil(z,codec.latent_bits,3)[0] |
|
buff = io.BytesIO() |
|
webp.save(buff, format='WEBP', lossless=True) |
|
webp_bytes = buff.getbuffer() |
|
``` |
|
|
|
|
|
```python |
|
print("compression_ratio: ", x.numel()/len(webp_bytes)) |
|
webp |
|
``` |
|
|
|
compression_ratio: 9.83650170496386 |
|
|
|
|
|
|
|
|
|
|
|
|
|
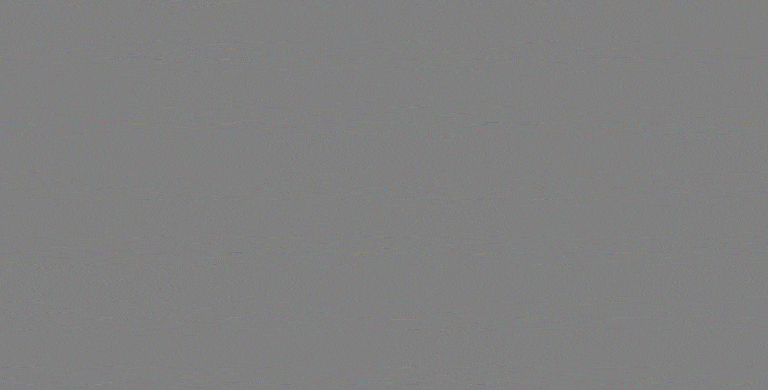 |
|
|
|
|
|
|
|
|
|
# Decoding |
|
|
|
|
|
```python |
|
with torch.no_grad(): |
|
z_hat = walloc.pil_to_latent( |
|
[Image.open(buff)], |
|
codec.latent_dim, |
|
codec.latent_bits, |
|
3) |
|
X_hat = codec.decoder(rearrange(z_hat, 'b c h w -> b c (h w)')[:,:,:ℓ]) |
|
x_hat = codec.wavelet_synthesis(X_hat,codec.J) |
|
x_hat = codec.post(x_hat) |
|
x_hat = codec.clamp(x_hat[0,:,:L]) |
|
``` |
|
|
|
|
|
```python |
|
start, end = 0, 1000 |
|
plt.figure(figsize=(8, 3), dpi=180) |
|
plt.plot(x[0, start:end], alpha=0.5, c='b', label='Ch.1 (Uncompressed)') |
|
plt.plot(x_hat[0, start:end], alpha=0.5, c='g', label='Ch.1 (WaLLoC)') |
|
plt.plot(x[1, start:end], alpha=0.5, c='r', label='Ch.2 (Uncompressed)') |
|
plt.plot(x_hat[1, start:end], alpha=0.5, c='purple', label='Ch.2 (WaLLoC)') |
|
|
|
plt.xlim([400,1000]) |
|
plt.ylim([-0.6,0.3]) |
|
plt.legend(loc='lower center') |
|
plt.box(False) |
|
plt.xticks([]) |
|
plt.yticks([]); |
|
``` |
|
|
|
|
|
|
|
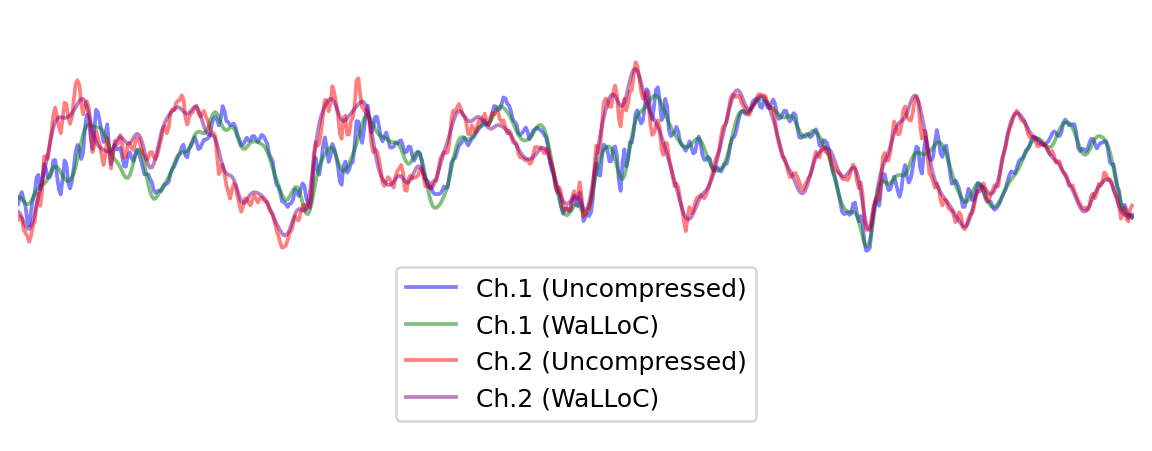 |
|
|
|
|
|
|
|
|
|
```python |
|
!jupyter nbconvert --to markdown README.ipynb |
|
``` |
|
|
|
[NbConvertApp] Converting notebook README.ipynb to markdown |
|
[NbConvertApp] Support files will be in README_files/ |
|
[NbConvertApp] Writing 12900 bytes to README.md |
|
|
|
|
|
|
|
```python |
|
!sed -i 's|!\[png](README_files/\(README_[0-9]*_[0-9]*\.png\))||g' README.md |
|
``` |
|
|
|
|
|
```python |
|
!sed -i 's|src="README_files/\(README_[0-9]*\.wav\)"|src="https://huggingface.co/danjacobellis/walloc/resolve/main/README_files/\1"|g' README.md |
|
``` |
|
|