File size: 11,619 Bytes
5a3a07c a534d17 5a3a07c a534d17 5a3a07c a534d17 5a3a07c a534d17 5a3a07c a534d17 5a3a07c a534d17 5a3a07c a534d17 5a3a07c a534d17 5a3a07c a534d17 5a3a07c a534d17 5a3a07c a534d17 5a3a07c a534d17 5a3a07c a534d17 |
1 2 3 4 5 6 7 8 9 10 11 12 13 14 15 16 17 18 19 20 21 22 23 24 25 26 27 28 29 30 31 32 33 34 35 36 37 38 39 40 41 42 43 44 45 46 47 48 49 50 51 52 53 54 55 56 57 58 59 60 61 62 63 64 65 66 67 68 69 70 71 72 73 74 75 76 77 78 79 80 81 82 83 84 85 86 87 88 89 90 91 92 93 94 95 96 97 98 99 100 101 102 103 104 105 106 107 108 109 110 111 112 113 114 115 116 117 118 119 120 121 122 123 124 125 126 127 128 129 130 131 132 133 134 135 136 137 138 139 140 141 142 143 144 145 146 147 148 149 150 151 152 153 154 155 156 157 158 159 160 161 162 163 164 165 166 167 168 169 170 171 172 173 174 175 176 177 178 179 180 181 182 183 184 185 186 187 188 189 190 191 192 193 194 195 196 197 198 199 200 201 202 203 204 205 206 207 208 209 210 211 212 213 214 215 216 217 218 219 220 221 222 223 224 225 226 227 228 229 230 231 232 233 234 235 236 237 238 239 240 241 242 243 244 245 246 247 248 249 250 251 252 253 254 255 256 257 258 259 260 261 262 263 264 265 266 267 268 269 270 271 272 273 274 275 276 277 278 279 280 281 282 283 284 285 286 287 288 289 290 291 292 293 294 295 296 297 298 299 300 301 302 303 304 305 306 307 308 309 310 311 312 313 314 315 316 317 318 319 320 321 322 323 324 325 326 327 328 329 330 331 332 333 334 335 336 337 338 339 340 341 342 343 344 345 346 347 348 349 350 351 |
---
license: apache-2.0
task_categories:
- graph-ml
tags:
- horology
size_categories:
- 100K<n<1M
---
# Watch Market Analysis Graph Neural Network Dataset
## Executive Summary
This dataset transforms traditional watch market data into a Graph Neural Network (GNN) structure, specifically designed to capture the complex dynamics of the pre-owned luxury watch market.
It addresses three key market characteristics that traditional recommendation systems often miss:
- **Condition-Based Value Dynamics**: Captures how a watch's condition influences its market position and value relative to other timepieces
- **Temporal Price Behaviors**: Models non-linear price patterns where certain watches appreciate while others depreciate
- **Inter-Model Relationships**: Maps complex value relationships between different models that transcend traditional brand hierarchies
### Key Statistics
- Total Watches: 284,491
- Total Brands: 28
- Price Range: $50 - $3.2M
- Year Range: 1559-2024
### Primary Use Cases
- Advanced watch recommendation systems
- Market positioning analysis
- Value relationship modeling
- Temporal trend analysis
## Dataset Description
### Data Structure
The dataset is structured as a PyTorch Geometric Data object with three main components:
- Node features tensor (watch attributes)
- Edge index matrix (watch connections)
- Edge attributes (similarity weights)
### Features
Key features include:
- **Brand Embeddings**: 128-dimensional vectors capturing brand identity and market position
- **Material Embeddings**: 64-dimensional vectors for material types and values
- **Movement Embeddings**: 64-dimensional vectors representing technical hierarchies
- **Temporal Features**: 32-dimensional cyclical embeddings for year and seasonal patterns
- **Condition Scores**: Standardized scale (0.5-1.0) based on watch condition
- **Price Features**: Log-transformed and normalized across market segments
- **Physical Attributes**: Standardized measurements in millimeters
### Network Properties
- **Node Connections**: 3-5 edges per watch
- **Similarity Threshold**: 70% minimum similarity for edge creation
- **Edge Weights**: Based on multiple similarity factors:
- Price (50% influence)
- Brand similarity
- Material type
- Temporal proximity
- Condition score
### Processing Parameters
- Batch Size: 50 watches per chunk
- Processing Window: 1000 watches
- Edge Generation Batch: 32 watches
- Network Architecture: Combined GCN and GAT layers with 4 attention heads
## Exploratory Data Analysis
### Brand Distribution
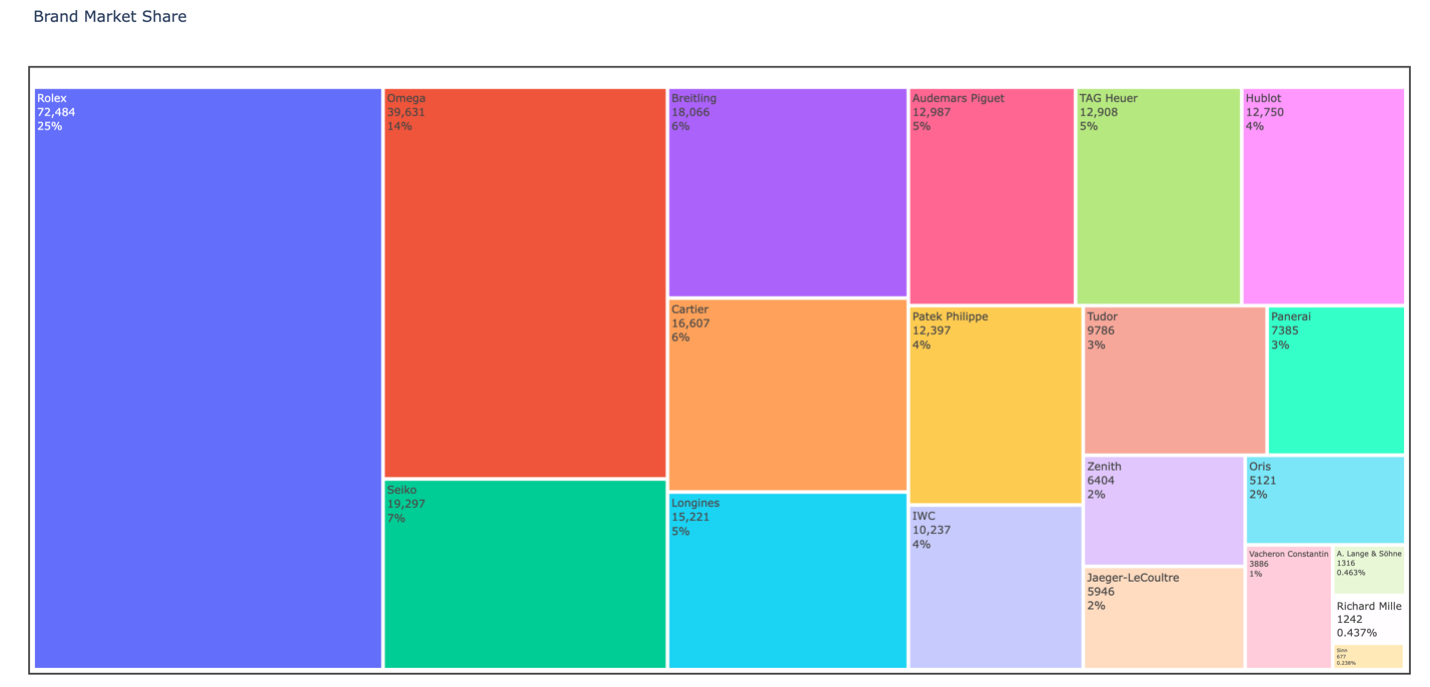
The treemap visualization provides a hierarchical view of market presence:
- Rolex dominates with the highest representation, reflecting its market leadership
- Omega and Seiko follow as major players, indicating a strong market presence
- Distribution reveals clear tiers in the luxury watch market
- Brand representation correlates with market positioning and availability
### Feature Correlations
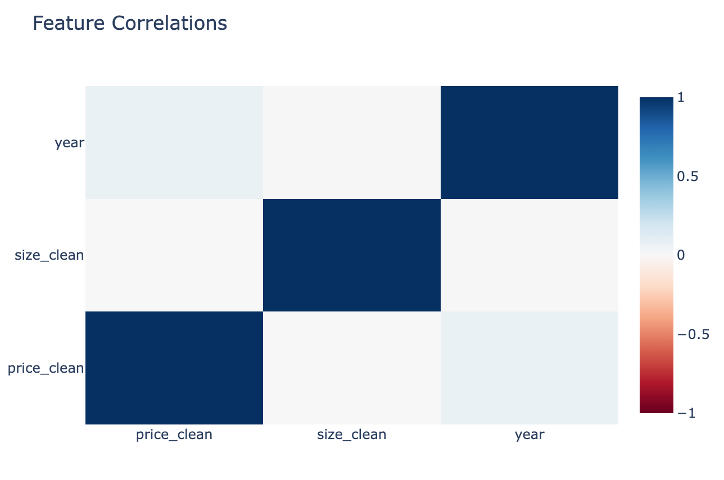
The correlation matrix reveals important market dynamics:
- **Size vs. Year**: Positive correlation indicating a trend toward larger case sizes in modern watches
- **Price vs. Size**: Moderate correlation showing larger watches generally command higher prices
- **Price vs. Year**: Notably low correlation, demonstrating that vintage watches maintain value
- Each feature contributes unique information, validated by the lack of strong correlations across all variables
### Market Structure Visualizations
#### UMAP Analysis
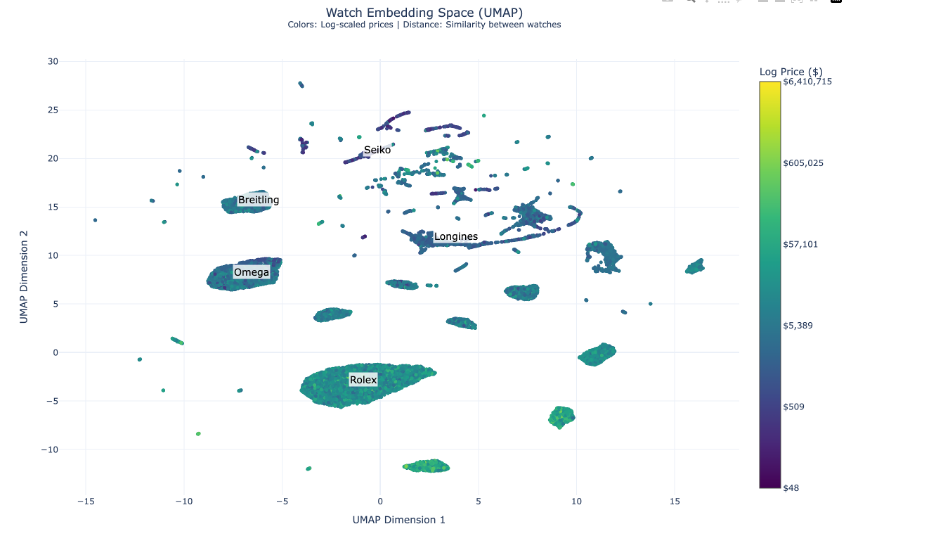
The UMAP visualization unveils complex market positioning dynamics:
- Rolex maintains a dominant central position around coordinates (0, -5), showing unparalleled brand cohesion
- Omega and Breitling cluster in the left segment, indicating strategic market alignment
- Seiko and Longines occupy the upper-right quadrant, reflecting distinct value propositions
- Premium timepieces (yellower/greener hues) show tighter clustering, suggesting standardized luxury attributes
- Smaller, specialized clusters indicate distinct horological collections and style categories
#### t-SNE Visualization
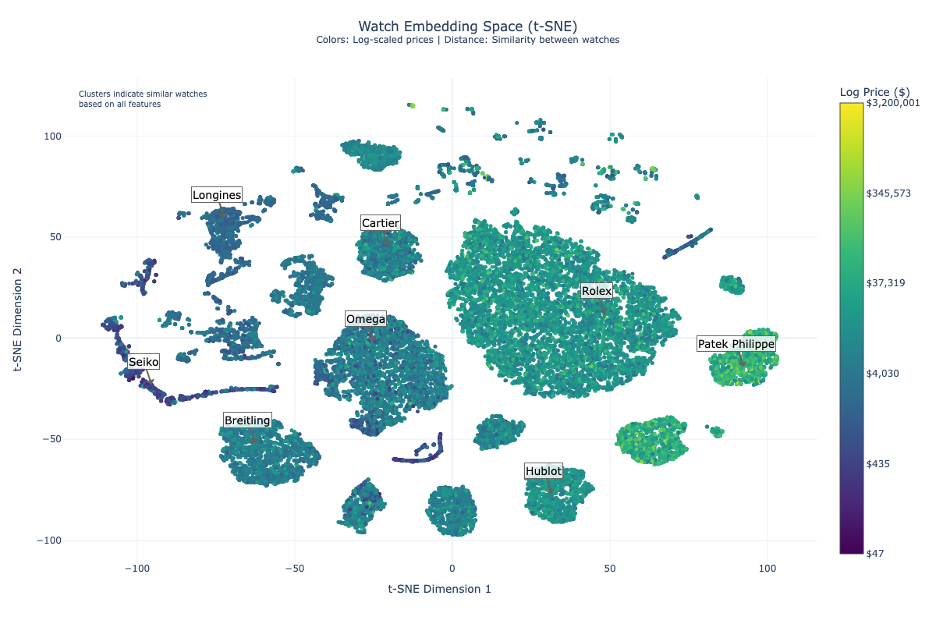
T-SNE analysis reveals clear market stratification with logarithmic pricing from $50 to $3.2M:
- **Entry-Level Segment ($50-$4,000)**
- Anchored by Seiko in the left segment
- High volume, accessible luxury positioning
- **Mid-Range Segment ($4,000-$35,000)**
- Occupies central space
- Shows competitive positioning between brands
- Cartier demonstrates strategic positioning between luxury and mid-range
- **Ultra-Luxury Segment ($35,000-$3.2M)**
- Dominated by Patek Philippe and Audemars Piguet
- Clear separation in the right segment
- Strong brand clustering indicating market alignment
#### PCA Analysis
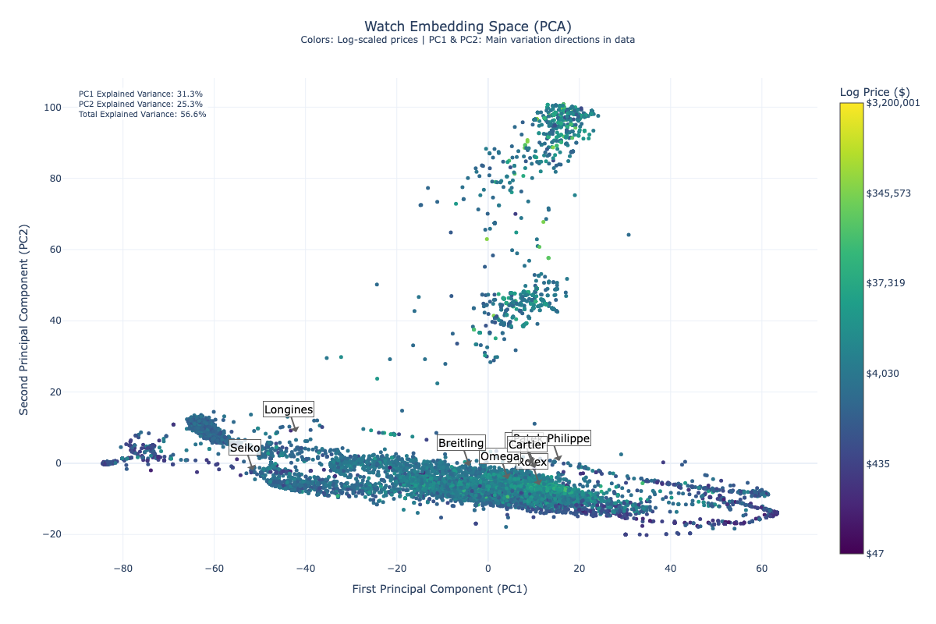
Principal Component Analysis provides robust market insights with 56.6% total explained variance:
- **First Principal Component (31.3%)**
- Predominantly captures price dynamics
- Shows clear separation between market segments
- **Second Principal Component (25.3%)**
- Reflects brand positioning and design philosophies
- Reveals vertical dispersion indicating intra-brand diversity
- **Brand Trajectory**
- Natural progression from Seiko through Longines, Breitling, and Omega
- Culminates in Rolex and Patek Philippe
- Diagonal trend line serves as a market positioning indicator
- **Market Implications**
- Successful brands occupy optimal positions along both dimensions
- Clear differentiation between adjacent competitors
- Evidence of strategic market positioning
#### Network Visualizations
**Force-Directed Graph**
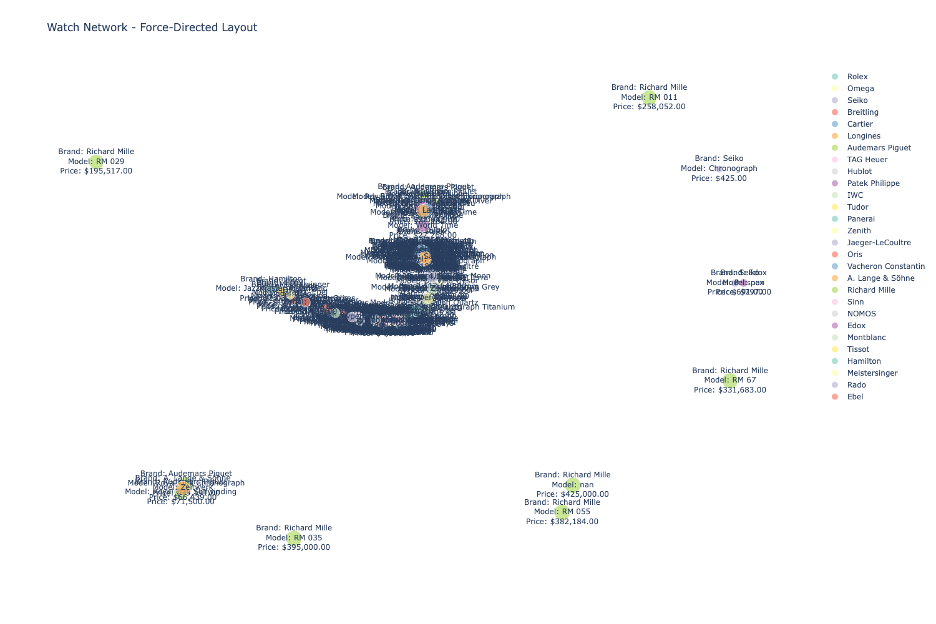
The force-directed layout reveals natural market clustering:
- Richard Mille's peripheral positioning highlights ultra-luxury strategy
- Dense central clustering shows mainstream luxury brand interconnectivity
- Edge patterns reveal shared market characteristics
- Node proximity indicates competitive positioning
**Starburst Visualization**
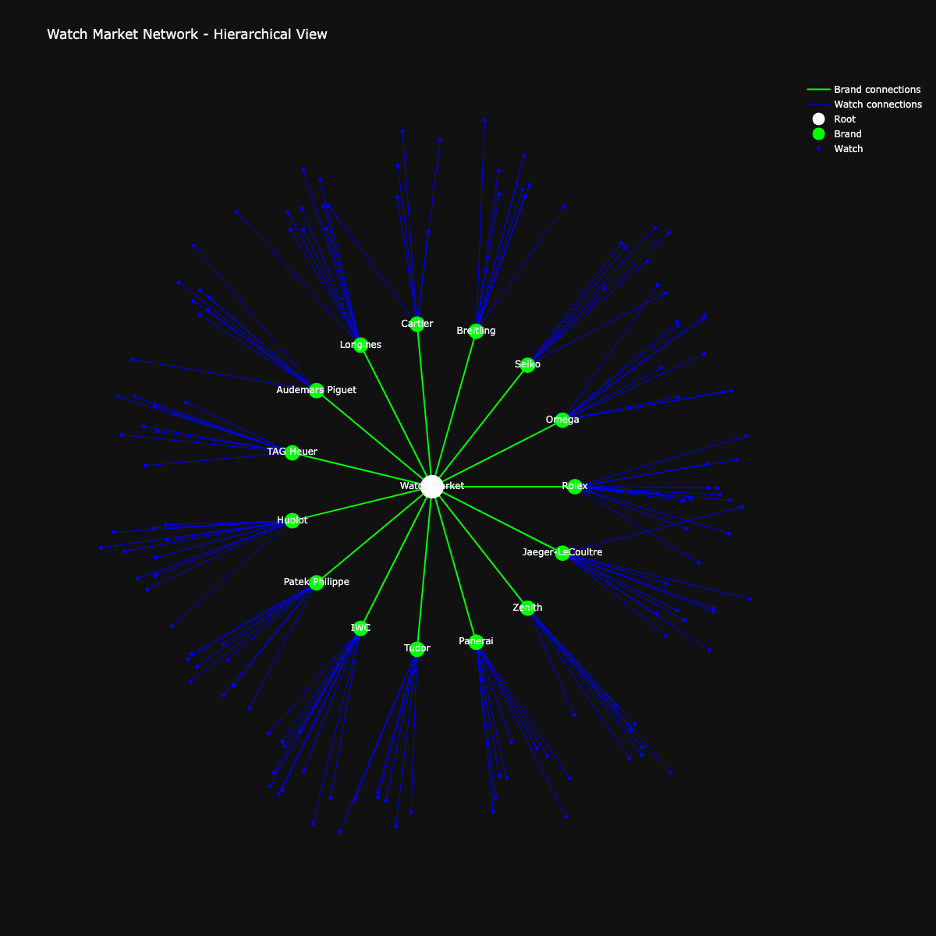
Radial architecture provides a hierarchical market perspective:
- Central node represents the overall market
- Green nodes show brand territories with strategic spacing
- Blue peripheral nodes indicate individual timepieces
- Node density reveals:
- Brand portfolio breadth
- Market penetration depth
- Segment diversification
- Balanced spacing between brand nodes indicates market segmentation
## Ethics and Limitations
### Data Collection and Privacy
- Dataset consists of publicly available watch listings
- No personal information, seller details, or private transaction data
- Serial numbers and identifying marks removed
- Strict privacy standards maintained throughout collection
### Known Biases
#### Connection Strength Bias
- Edge weights and connections based on author's domain expertise
- Similarity thresholds (70%) chosen based on personal market understanding
- Brand value weightings reflect author's market analysis
- Connection strengths may not universally reflect all market perspectives
#### Market Representation Bias
- Predominantly represents online listings
- May not fully capture private sales and in-person transactions
- Popular brands overrepresented (Rolex 25%, Omega 14%)
- Limited editions and rare pieces underrepresented
#### Temporal Bias
- Stronger representation of recent listings
- Historical data may be underrepresented
- Current market conditions more heavily weighted
- Seasonal variations may affect price patterns
#### Brand and Model Bias
- Skewed toward mainstream luxury brands
- Limited representation of boutique manufacturers
- Popular models have more data points
- Vintage and discontinued models may lack comprehensive data
#### Price Bias
- Asking prices may differ from actual transaction values
- Regional price variations not fully captured
- Currency conversion effects on price relationships
- Market fluctuations may not be fully represented
### Usage Guidelines
#### Appropriate Uses
- Market research and analysis
- Academic research
- Watch relationship modeling
- Price trend studies
- Educational purposes
#### Prohibited Uses
- Price manipulation or market distortion
- Unfair trading practices
- Personal data extraction
- Misleading market analysis
- Anti-competitive practices
### License
This dataset is released under the Apache 2.0 License, which allows:
- Commercial use
- Modification
- Distribution
- Private use
While requiring:
- License and copyright notice
- State changes
- Preserve attributions
## Technical Details
### Power Analysis
Minimum sample requirements based on statistical analysis:
- Basic Network: 10,671 nodes (95% confidence, 3% margin)
- GNN Requirements: 14,400 samples (feature space dimensionality)
- Brand Coverage: 768 watches per brand
- Price Segments: 4,320 watches per segment
Current dataset (284,491 watches) exceeds requirements with:
- 5,000+ samples per major brand
- 50,000+ samples per price segment
- Sufficient network density
### Implementation Details
#### Network Architecture
- 3 GNN layers with residual connections
- 64 hidden channels
- 20% dropout rate
- 4 attention heads
- Learning rate: 0.001
#### Embedding Dimensions
- Brand: 128
- Material: 64
- Movement: 64
- Temporal: 32
#### Network Parameters
- Connections per watch: 3-5
- Similarity threshold: 70%
- Batch size: 50 watches
- Processing window: 1000 watches
#### Condition Scoring
- New: 1.0
- Unworn: 0.95
- Very Good: 0.8
- Good: 0.7
- Fair: 0.5
## Usage
### Required Files
The dataset consists of three main files:
- `watch_gnn_data.pt` (315 MB): Main PyTorch Geometric data object
- `edges.npz` (20.5 MB): Edge information
- `features.npy` (596 MB): Node features
### Loading the Dataset
```python
import torch
from torch_geometric.data import Data
# Load the main dataset
data = torch.load('watch_gnn_data.pt')
```
#### Access components
```
node_features = data.x # Shape: [284491, combined_embedding_dim]
edge_index = data.edge_index # Shape: [2, num_edges]
edge_attr = data.edge_attr # Shape: [num_edges, 1]
```
#### For direct feature access
```
features = np.load('features.npy')
```
#### Get number of nodes
```
num_nodes = data.num_nodes
```
#### Get number of edges
```
num_edges = data.num_edges
```
#### Find similar watches (k-nearest neighbors)
```
def find_similar_watches(watch_id, k=5):
# Get watch features
watch_features = data.x[watch_id]
# Calculate similarities
similarities = torch.cosine_similarity(
watch_features.unsqueeze(0),
data.x,
dim=1
)
# Get top k similar watches
_, indices = similarities.topk(k+1) # +1 to exclude self
return indices[1:] # Exclude self
# Get watch features
def get_watch_features(watch_id):
return data.x[watch_id]
```
## Note
- The dataset is optimized for PyTorch Geometric operations
- Recommended to use GPU for large-scale operations
- Consider batch processing for memory efficiency
|