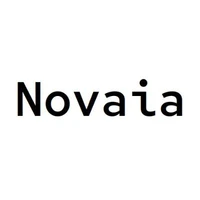
novaia/terra-4m
Unconditional Image Generation
•
Updated
•
24
•
2
heightmap
imagewidth (px) 256
256
| latitude
stringclasses 115
values | longitude
stringclasses 329
values |
---|---|---|
n47 | e006 |
|
n26 | w113 |
|
n52 | w002 |
|
s28 | e117 |
|
n38 | e073 |
|
n35 | e086 |
|
n40 | w102 |
|
s37 | w066 |
|
s01 | e020 |
|
n27 | e068 |
|
n02 | w056 |
|
n21 | e098 |
|
n32 | w091 |
|
n58 | e122 |
|
n24 | w001 |
|
n00 | e021 |
|
n38 | e083 |
|
n03 | e012 |
|
n48 | e108 |
|
s33 | e150 |
|
n45 | e038 |
|
n30 | e010 |
|
n48 | e077 |
|
s23 | w058 |
|
n28 | e017 |
|
s37 | w072 |
|
n31 | e074 |
|
s04 | e039 |
|
n29 | e075 |
|
n53 | e078 |
|
n09 | w006 |
|
n35 | e072 |
|
n48 | e048 |
|
s24 | w062 |
|
n39 | e034 |
|
n23 | e090 |
|
n44 | w089 |
|
s09 | w039 |
|
s01 | e022 |
|
n25 | e117 |
|
n29 | e015 |
|
n46 | w071 |
|
s31 | e125 |
|
n04 | w069 |
|
n36 | e113 |
|
s32 | w063 |
|
n58 | e026 |
|
n30 | w005 |
|
n21 | e018 |
|
n48 | w081 |
|
s35 | e117 |
|
s22 | e029 |
|
n38 | w107 |
|
n38 | e082 |
|
n48 | e003 |
|
n50 | e055 |
|
n54 | e030 |
|
n33 | w102 |
|
n34 | w119 |
|
s23 | e137 |
|
n14 | w087 |
|
n46 | e015 |
|
n26 | e066 |
|
s24 | e130 |
|
n42 | e121 |
|
n24 | e082 |
|
n34 | w087 |
|
n44 | e130 |
|
s08 | w073 |
|
n39 | w099 |
|
n27 | e036 |
|
s18 | w041 |
|
n39 | e113 |
|
n38 | e113 |
|
s19 | e015 |
|
n35 | e134 |
|
s04 | w043 |
|
n36 | e002 |
|
n36 | e003 |
|
n14 | e015 |
|
n41 | e126 |
|
n39 | w104 |
|
n42 | w103 |
|
n34 | e133 |
|
s20 | w051 |
|
n25 | e007 |
|
n36 | w105 |
|
s10 | w049 |
|
n39 | e093 |
|
n46 | e112 |
|
n46 | e124 |
|
n47 | e126 |
|
n37 | e041 |
|
n46 | e056 |
|
n40 | e075 |
|
n32 | e004 |
|
n48 | w057 |
|
n30 | w109 |
|
n34 | e088 |
|
n02 | e016 |
This is a dataset of 256x256 Earth heightmaps generated from SRTM 1 Arc-Second Global. Each heightmap is labelled according to its latitude and longitude. There are 573,995 samples. It is the same as World Heightmaps 360px but downsampled to 256x256.
import rasterio
import matplotlib.pyplot as plt
import os
input_directory = '...'
output_directory = '...'
file_list = os.listdir(input_directory)
for i in range(len(file_list)):
image = rasterio.open(input_directory + file_list[i])
plt.imsave(output_directory + file_list[i][0:-4] + '.png', image.read(1), cmap='gray')
from split_image import split_image
import os
input_directory = '...'
output_directory = '...'
file_list = os.listdir(input_directory)
for i in range(len(file_list)):
split_image(input_directory + file_list[i], 10, 10, should_square=True, should_cleanup=False, output_dir=output_directory)
Hand pick a dataset of corrupted and uncorrupted heightmaps then train a discriminator to automatically filter the whole dataset.
Downsample from 360x360 to 256x256 with Pillow and the Lanczos resampling method.
import glob
from PIL import Image
paths = glob.glob('world-heightmaps-360px-png/data/*/*')
for file_name in paths:
image = Image.open(file_name)
if image.width == 256:
continue
print(file_name)
image = image.resize((256, 256), resample=Image.LANCZOS)
image.save(file_name)
import pyarrow as pa
import pyarrow.parquet as pq
import pandas as pd
from PIL import Image
import os
import io
import json
samples_per_file = 10_000
root_dir = 'data/datasets/world-heightmaps-256px-png'
df = pd.read_csv(os.path.join(root_dir, 'metadata.csv'))
df = df.sample(frac=1).reset_index(drop=True)
def save_table(image_data, table_number):
print(f'Entries in table {table_number}: {len(image_data)}')
schema = pa.schema(
fields=[
('heightmap', pa.struct([('bytes', pa.binary()), ('path', pa.string())])),
('latitude', pa.string()),
('longitude', pa.string())
],
metadata={
b'huggingface': json.dumps({
'info': {
'features': {
'heightmap': {'_type': 'Image'},
'latitude': {'_type': 'Value', 'dtype': 'string'},
'longitude': {'_type': 'Value', 'dtype': 'string'}
}
}
}).encode('utf-8')
}
)
table = pa.Table.from_pylist(image_data, schema=schema)
pq.write_table(table, f'data/world-heightmaps-256px-parquet/{str(table_number).zfill(4)}.parquet')
image_data = []
samples_in_current_file = 0
current_file_number = 0
for i, row in df.iterrows():
if samples_in_current_file >= samples_per_file:
save_table(image_data, current_file_number)
image_data = []
samples_in_current_file = 0
current_file_number += 1
samples_in_current_file += 1
image_path = row['file_name']
with Image.open(os.path.join(root_dir, image_path)) as image:
image_bytes = io.BytesIO()
image.save(image_bytes, format='PNG')
image_dict = {
'heightmap': {
'bytes': image_bytes.getvalue(),
'path': image_path
},
'latitude': str(row['latitude']),
'longitude': str(row['longitude'])
}
image_data.append(image_dict)
save_table(image_data, current_file_number)