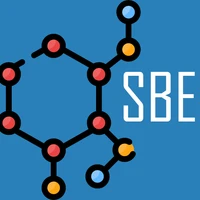
premise
stringlengths 7
402
| hypothesis
stringlengths 1
295
| label
class label 3
classes |
---|---|---|
A person on a horse jumps over a broken down airplane. | A person is training his horse for a competition. | 1neutral
|
A person on a horse jumps over a broken down airplane. | A person is at a diner, ordering an omelette. | 2contradiction
|
A person on a horse jumps over a broken down airplane. | A person is outdoors, on a horse. | 0entailment
|
Children smiling and waving at camera | They are smiling at their parents | 1neutral
|
Children smiling and waving at camera | There are children present | 0entailment
|
Children smiling and waving at camera | The kids are frowning | 2contradiction
|
A boy is jumping on skateboard in the middle of a red bridge. | The boy skates down the sidewalk. | 2contradiction
|
A boy is jumping on skateboard in the middle of a red bridge. | The boy does a skateboarding trick. | 0entailment
|
A boy is jumping on skateboard in the middle of a red bridge. | The boy is wearing safety equipment. | 1neutral
|
An older man sits with his orange juice at a small table in a coffee shop while employees in bright colored shirts smile in the background. | An older man drinks his juice as he waits for his daughter to get off work. | 1neutral
|
An older man sits with his orange juice at a small table in a coffee shop while employees in bright colored shirts smile in the background. | A boy flips a burger. | 2contradiction
|
An older man sits with his orange juice at a small table in a coffee shop while employees in bright colored shirts smile in the background. | An elderly man sits in a small shop. | 1neutral
|
Two blond women are hugging one another. | Some women are hugging on vacation. | 1neutral
|
Two blond women are hugging one another. | The women are sleeping. | 2contradiction
|
Two blond women are hugging one another. | There are women showing affection. | 0entailment
|
A few people in a restaurant setting, one of them is drinking orange juice. | The people are eating omelettes. | 1neutral
|
A few people in a restaurant setting, one of them is drinking orange juice. | The people are sitting at desks in school. | 2contradiction
|
A few people in a restaurant setting, one of them is drinking orange juice. | The diners are at a restaurant. | 0entailment
|
An older man is drinking orange juice at a restaurant. | A man is drinking juice. | 0entailment
|
An older man is drinking orange juice at a restaurant. | Two women are at a restaurant drinking wine. | 2contradiction
|
An older man is drinking orange juice at a restaurant. | A man in a restaurant is waiting for his meal to arrive. | 1neutral
|
A man with blond-hair, and a brown shirt drinking out of a public water fountain. | A blond man getting a drink of water from a fountain in the park. | 1neutral
|
A man with blond-hair, and a brown shirt drinking out of a public water fountain. | A blond man wearing a brown shirt is reading a book on a bench in the park | 2contradiction
|
A man with blond-hair, and a brown shirt drinking out of a public water fountain. | A blond man drinking water from a fountain. | 0entailment
|
Two women who just had lunch hugging and saying goodbye. | The friends scowl at each other over a full dinner table. | 2contradiction
|
Two women who just had lunch hugging and saying goodbye. | There are two woman in this picture. | 0entailment
|
Two women who just had lunch hugging and saying goodbye. | The friends have just met for the first time in 20 years, and have had a great time catching up. | 1neutral
|
Two women, holding food carryout containers, hug. | The two sisters saw each other across the crowded diner and shared a hug, both clutching their doggie bags. | 1neutral
|
Two women, holding food carryout containers, hug. | Two groups of rival gang members flipped each other off. | 2contradiction
|
Two women, holding food carryout containers, hug. | Two women hug each other. | 0entailment
|
A Little League team tries to catch a runner sliding into a base in an afternoon game. | A team is trying to score the games winning out. | 1neutral
|
A Little League team tries to catch a runner sliding into a base in an afternoon game. | A team is trying to tag a runner out. | 0entailment
|
A Little League team tries to catch a runner sliding into a base in an afternoon game. | A team is playing baseball on Saturn. | 2contradiction
|
The school is having a special event in order to show the american culture on how other cultures are dealt with in parties. | A school hosts a basketball game. | 2contradiction
|
The school is having a special event in order to show the american culture on how other cultures are dealt with in parties. | A high school is hosting an event. | 1neutral
|
The school is having a special event in order to show the american culture on how other cultures are dealt with in parties. | A school is hosting an event. | 0entailment
|
High fashion ladies wait outside a tram beside a crowd of people in the city. | The women do not care what clothes they wear. | 2contradiction
|
High fashion ladies wait outside a tram beside a crowd of people in the city. | Women are waiting by a tram. | 0entailment
|
High fashion ladies wait outside a tram beside a crowd of people in the city. | The women enjoy having a good fashion sense. | 1neutral
|
A man, woman, and child enjoying themselves on a beach. | A child with mom and dad, on summer vacation at the beach. | 1neutral
|
A man, woman, and child enjoying themselves on a beach. | A family of three is at the beach. | 0entailment
|
A man, woman, and child enjoying themselves on a beach. | A family of three is at the mall shopping. | 2contradiction
|
People waiting to get on a train or just getting off. | The people waiting on the train are sitting. | 1neutral
|
People waiting to get on a train or just getting off. | There are people just getting on a train | 0entailment
|
People waiting to get on a train or just getting off. | There are people waiting on a train. | 0entailment
|
A couple playing with a little boy on the beach. | A couple are playing with a young child outside. | 0entailment
|
A couple playing with a little boy on the beach. | A couple are playing frisbee with a young child at the beach. | 1neutral
|
A couple playing with a little boy on the beach. | A couple watch a little girl play by herself on the beach. | 2contradiction
|
A couple play in the tide with their young son. | The family is sitting down for dinner. | 2contradiction
|
A couple play in the tide with their young son. | The family is outside. | 0entailment
|
A couple play in the tide with their young son. | The family is on vacation. | 1neutral
|
A man and a woman cross the street in front of a pizza and gyro restaurant. | The people are standing still on the curb. | 2contradiction
|
A man and a woman cross the street in front of a pizza and gyro restaurant. | Near a couple of restaurants, two people walk across the street. | 0entailment
|
A man and a woman cross the street in front of a pizza and gyro restaurant. | The couple are walking across the street together. | 1neutral
|
A woman in a green jacket and hood over her head looking towards a valley. | The woman is nake. | 2contradiction
|
A woman in a green jacket and hood over her head looking towards a valley. | The woman is cold. | 1neutral
|
A woman in a green jacket and hood over her head looking towards a valley. | The woman is wearing green. | 0entailment
|
Woman in white in foreground and a man slightly behind walking with a sign for John's Pizza and Gyro in the background. | The man with the sign is caucasian. | 1neutral
|
Woman in white in foreground and a man slightly behind walking with a sign for John's Pizza and Gyro in the background. | They are protesting outside the capital. | 2contradiction
|
Woman in white in foreground and a man slightly behind walking with a sign for John's Pizza and Gyro in the background. | A woman in white. | 0entailment
|
Woman in white in foreground and a man slightly behind walking with a sign for John's Pizza and Gyro in the background. | A man is advertising for a restaurant. | 0entailment
|
Woman in white in foreground and a man slightly behind walking with a sign for John's Pizza and Gyro in the background. | The woman is wearing black. | 2contradiction
|
Woman in white in foreground and a man slightly behind walking with a sign for John's Pizza and Gyro in the background. | A man and a woman walk down a crowded city street. | 1neutral
|
Woman in white in foreground and a man slightly behind walking with a sign for John's Pizza and Gyro in the background. | The woman is wearing white. | 0entailment
|
Woman in white in foreground and a man slightly behind walking with a sign for John's Pizza and Gyro in the background. | They are working for John's Pizza. | 1neutral
|
Woman in white in foreground and a man slightly behind walking with a sign for John's Pizza and Gyro in the background. | Olympic swimming. | 2contradiction
|
Woman in white in foreground and a man slightly behind walking with a sign for John's Pizza and Gyro in the background. | A man and a soman are eating together at John's Pizza and Gyro. | 2contradiction
|
Woman in white in foreground and a man slightly behind walking with a sign for John's Pizza and Gyro in the background. | They are walking with a sign. | 0entailment
|
Woman in white in foreground and a man slightly behind walking with a sign for John's Pizza and Gyro in the background. | The woman is waiting for a friend. | 1neutral
|
Woman in white in foreground and a man slightly behind walking with a sign for John's Pizza and Gyro in the background. | The man is sitting down while he has a sign for John's Pizza and Gyro in his arms. | 2contradiction
|
Woman in white in foreground and a man slightly behind walking with a sign for John's Pizza and Gyro in the background. | The woman and man are outdoors. | 0entailment
|
Woman in white in foreground and a man slightly behind walking with a sign for John's Pizza and Gyro in the background. | A woman ordering pizza. | 1neutral
|
Two adults, one female in white, with shades and one male, gray clothes, walking across a street, away from a eatery with a blurred image of a dark colored red shirted person in the foreground. | The people are related. | 1neutral
|
Two adults, one female in white, with shades and one male, gray clothes, walking across a street, away from a eatery with a blurred image of a dark colored red shirted person in the foreground. | Two adults run across the street to get away from a red shirted person chasing them. | 2contradiction
|
Two adults, one female in white, with shades and one male, gray clothes, walking across a street, away from a eatery with a blurred image of a dark colored red shirted person in the foreground. | The adults are both male and female. | 0entailment
|
Two adults, one female in white, with shades and one male, gray clothes, walking across a street, away from a eatery with a blurred image of a dark colored red shirted person in the foreground. | Two people walk home after a tasty steak dinner. | 1neutral
|
Two adults, one female in white, with shades and one male, gray clothes, walking across a street, away from a eatery with a blurred image of a dark colored red shirted person in the foreground. | Two adults swimming in water | 2contradiction
|
Two adults, one female in white, with shades and one male, gray clothes, walking across a street, away from a eatery with a blurred image of a dark colored red shirted person in the foreground. | Two adults walk across a street. | 0entailment
|
Two adults, one female in white, with shades and one male, gray clothes, walking across a street, away from a eatery with a blurred image of a dark colored red shirted person in the foreground. | Two people ride bicycles into a tunnel. | 2contradiction
|
Two adults, one female in white, with shades and one male, gray clothes, walking across a street, away from a eatery with a blurred image of a dark colored red shirted person in the foreground. | Two people walk away from a restaurant across a street. | 0entailment
|
Two adults, one female in white, with shades and one male, gray clothes, walking across a street, away from a eatery with a blurred image of a dark colored red shirted person in the foreground. | Two adults walking across a road near the convicted prisoner dressed in red | 1neutral
|
Two adults, one female in white, with shades and one male, gray clothes, walking across a street, away from a eatery with a blurred image of a dark colored red shirted person in the foreground. | Two friends cross a street. | 1neutral
|
Two adults, one female in white, with shades and one male, gray clothes, walking across a street, away from a eatery with a blurred image of a dark colored red shirted person in the foreground. | Some people board a train. | 2contradiction
|
Two adults, one female in white, with shades and one male, gray clothes, walking across a street, away from a eatery with a blurred image of a dark colored red shirted person in the foreground. | Two adults walk across the street. | 0entailment
|
Two adults, one female in white, with shades and one male, gray clothes, walking across a street, away from a eatery with a blurred image of a dark colored red shirted person in the foreground. | Two adults walking across a road | 0entailment
|
Two adults, one female in white, with shades and one male, gray clothes, walking across a street, away from a eatery with a blurred image of a dark colored red shirted person in the foreground. | There are no women in the picture. | 2contradiction
|
Two adults, one female in white, with shades and one male, gray clothes, walking across a street, away from a eatery with a blurred image of a dark colored red shirted person in the foreground. | Two adults walk across the street to get away from a red shirted person who is chasing them. | 1neutral
|
A woman wearing all white and eating, walks next to a man holding a briefcase. | A married couple is sleeping. | 2contradiction
|
A woman wearing all white and eating, walks next to a man holding a briefcase. | A female is next to a man. | 0entailment
|
A woman wearing all white and eating, walks next to a man holding a briefcase. | A married couple is walking next to each other. | 1neutral
|
A woman is walking across the street eating a banana, while a man is following with his briefcase. | Nobody has food. | 2contradiction
|
A woman is walking across the street eating a banana, while a man is following with his briefcase. | A woman eats a banana and walks across a street, and there is a man trailing behind her. | 0entailment
|
A woman is walking across the street eating a banana, while a man is following with his briefcase. | The woman and man are playing baseball together. | 2contradiction
|
A woman is walking across the street eating a banana, while a man is following with his briefcase. | two coworkers cross pathes on a street | 1neutral
|
A woman is walking across the street eating a banana, while a man is following with his briefcase. | A woman eats ice cream walking down the sidewalk, and there is another woman in front of her with a purse. | 2contradiction
|
A woman is walking across the street eating a banana, while a man is following with his briefcase. | The mans briefcase is for work. | 1neutral
|
A woman is walking across the street eating a banana, while a man is following with his briefcase. | A person eating. | 0entailment
|
A woman is walking across the street eating a banana, while a man is following with his briefcase. | A person that is hungry. | 1neutral
|
A woman is walking across the street eating a banana, while a man is following with his briefcase. | An actress and her favorite assistant talk a walk in the city. | 1neutral
|
A woman is walking across the street eating a banana, while a man is following with his briefcase. | a woman eating a banana crosses a street | 0entailment
|
Dataset Card for SNLI
Dataset Summary
The SNLI corpus (version 1.0) is a collection of 570k human-written English sentence pairs manually labeled for balanced classification with the labels entailment, contradiction, and neutral, supporting the task of natural language inference (NLI), also known as recognizing textual entailment (RTE).
Supported Tasks and Leaderboards
Natural Language Inference (NLI), also known as Recognizing Textual Entailment (RTE), is the task of determining the inference relation between two (short, ordered) texts: entailment, contradiction, or neutral (MacCartney and Manning 2008).
See the corpus webpage for a list of published results.
Languages
The language in the dataset is English as spoken by users of the website Flickr and as spoken by crowdworkers from Amazon Mechanical Turk. The BCP-47 code for English is en.
Dataset Structure
Data Instances
For each instance, there is a string for the premise, a string for the hypothesis, and an integer for the label. Note that each premise may appear three times with a different hypothesis and label. See the SNLI corpus viewer to explore more examples.
{'premise': 'Two women are embracing while holding to go packages.'
'hypothesis': 'The sisters are hugging goodbye while holding to go packages after just eating lunch.'
'label': 1}
The average token count for the premises and hypotheses are given below:
Feature | Mean Token Count |
---|---|
Premise | 14.1 |
Hypothesis | 8.3 |
Data Fields
premise
: a string used to determine the truthfulness of the hypothesishypothesis
: a string that may be true, false, or whose truth conditions may not be knowable when compared to the premiselabel
: an integer whose value may be either 0, indicating that the hypothesis entails the premise, 1, indicating that the premise and hypothesis neither entail nor contradict each other, or 2, indicating that the hypothesis contradicts the premise. Dataset instances which don't have any gold label are marked with -1 label. Make sure you filter them before starting the training usingdatasets.Dataset.filter
.
Data Splits
The SNLI dataset has 3 splits: train, validation, and test. All of the examples in the validation and test sets come from the set that was annotated in the validation task with no-consensus examples removed. The remaining multiply-annotated examples are in the training set with no-consensus examples removed. Each unique premise/caption shows up in only one split, even though they usually appear in at least three different examples.
Dataset Split | Number of Instances in Split |
---|---|
Train | 550,152 |
Validation | 10,000 |
Test | 10,000 |
Dataset Creation
Curation Rationale
The SNLI corpus (version 1.0) was developed as a benchmark for natural langauge inference (NLI), also known as recognizing textual entailment (RTE), with the goal of producing a dataset large enough to train models using neural methodologies.
Source Data
Initial Data Collection and Normalization
The hypotheses were elicited by presenting crowdworkers with captions from preexisting datasets without the associated photos, but the vocabulary of the hypotheses still reflects the content of the photos as well as the caption style of writing (e.g. mostly present tense). The dataset developers report 37,026 distinct words in the corpus, ignoring case. They allowed bare NPs as well as full sentences. Using the Stanford PCFG Parser 3.5.2 (Klein and Manning, 2003) trained on the standard training set as well as on the Brown Corpus (Francis and Kucera 1979), the authors report that 74% of the premises and 88.9% of the hypotheses result in a parse rooted with an 'S'. The corpus was developed between 2014 and 2015.
Crowdworkers were presented with a caption without the associated photo and asked to produce three alternate captions, one that is definitely true, one that might be true, and one that is definitely false. See Section 2.1 and Figure 1 for details (Bowman et al., 2015).
The corpus includes content from the Flickr 30k corpus and the VisualGenome corpus. The photo captions used to prompt the data creation were collected on Flickr by Young et al. (2014), who extended the Flickr 8K dataset developed by Hodosh et al. (2013). Hodosh et al. collected photos from the following Flickr groups: strangers!, Wild-Child (Kids in Action), Dogs in Action (Read the Rules), Outdoor Activities, Action Photography, Flickr-Social (two or more people in the photo). Young et al. do not list the specific groups they collected photos from. The VisualGenome corpus also contains images from Flickr, originally collected in MS-COCO and YFCC100M.
The premises from the Flickr 30k corpus corrected for spelling using the Linux spell checker and ungrammatical sentences were removed. Bowman et al. do not report any normalization, though they note that punctuation and capitalization are often omitted.
Who are the source language producers?
A large portion of the premises (160k) were produced in the Flickr 30k corpus by an unknown number of crowdworkers. About 2,500 crowdworkers from Amazon Mechanical Turk produced the associated hypotheses. The premises from the Flickr 30k project describe people and animals whose photos were collected and presented to the Flickr 30k crowdworkers, but the SNLI corpus did not present the photos to the hypotheses creators.
The Flickr 30k corpus did not report crowdworker or photo subject demographic information or crowdworker compensation. The SNLI crowdworkers were compensated per HIT at rates between $.1 and $.5 with no incentives. Workers who ignored the guidelines were disqualified, and automated bulk submissions were rejected. No demographic information was collected from the SNLI crowdworkers.
An additional 4,000 premises come from the pilot study of the VisualGenome corpus. Though the pilot study itself is not described, the location information of the 33,000 AMT crowdworkers that participated over the course of the 6 months of data collection are aggregated. Most of the workers were located in the United States (93%), with others from the Philippines, Kenya, India, Russia, and Canada. Workers were paid $6-$8 per hour.
Annotations
Annotation process
56,941 of the total sentence pairs were further annotated in a validation task. Four annotators each labeled a premise-hypothesis pair as entailment, contradiction, or neither, resulting in 5 total judgements including the original hypothesis author judgement. See Section 2.2 for more details (Bowman et al., 2015).
The authors report 3/5 annotator agreement on 98% of the validation set and unanimous annotator agreement on 58.3% of the validation set. If a label was chosen by three annotators, that label was made the gold label. Following from this, 2% of the data did not have a consensus label and was labeled '-' by the authors.
Label | Fleiss κ |
---|---|
contradiction | 0.77 |
entailment | 0.72 |
neutral | 0.60 |
overall | 0.70 |
Who are the annotators?
The annotators of the validation task were a closed set of about 30 trusted crowdworkers on Amazon Mechanical Turk. No demographic information was collected. Annotators were compensated per HIT between $.1 and $.5 with $1 bonuses in cases where annotator labels agreed with the curators' labels for 250 randomly distributed examples.
Personal and Sensitive Information
The dataset does not contain any personal information about the authors or the crowdworkers, but may contain descriptions of the people in the original Flickr photos.
Considerations for Using the Data
Social Impact of Dataset
This dataset was developed as a benchmark for evaluating representational systems for text, especially including those induced by representation learning methods, in the task of predicting truth conditions in a given context. (It should be noted that the truth conditions of a hypothesis given a premise does not necessarily match the truth conditions of the hypothesis in the real world.) Systems that are successful at such a task may be more successful in modeling semantic representations.
Discussion of Biases
The language reflects the content of the photos collected from Flickr, as described in the Data Collection section. Rudinger et al (2017) use pointwise mutual information to calculate a measure of association between a manually selected list of tokens corresponding to identity categories and the other words in the corpus, showing strong evidence of stereotypes across gender categories. They also provide examples in which crowdworkers reproduced harmful stereotypes or pejorative language in the hypotheses.
Other Known Limitations
Gururangan et al (2018), Poliak et al (2018), and Tsuchiya (2018) show that the SNLI corpus has a number of annotation artifacts. Using various classifiers, Poliak et al correctly predicted the label of the hypothesis 69% of the time without using the premise, Gururangan et al 67% of the time, and Tsuchiya 63% of the time.
Additional Information
Dataset Curators
The SNLI corpus was developed by Samuel R. Bowman, Gabor Angeli, Christopher Potts, and Christopher D. Manning as part of the Stanford NLP group.
It was supported by a Google Faculty Research Award, a gift from Bloomberg L.P., the Defense Advanced Research Projects Agency (DARPA) Deep Exploration and Filtering of Text (DEFT) Program under Air Force Research Laboratory (AFRL) contract no. FA8750-13-2-0040, the National Science Foundation under grant no. IIS 1159679, and the Department of the Navy, Office of Naval Research, under grant no. N00014-10-1-0109.
Licensing Information
The Stanford Natural Language Inference Corpus by The Stanford NLP Group is licensed under a Creative Commons Attribution-ShareAlike 4.0 International License.
The corpus includes content from the Flickr 30k corpus, also released under an Attribution-ShareAlike licence.
Citation Information
The following paper introduces the corpus in detail. If you use the corpus in published work, please cite it:
@inproceedings{bowman-etal-2015-large,
title = "A large annotated corpus for learning natural language inference",
author = "Bowman, Samuel R. and
Angeli, Gabor and
Potts, Christopher and
Manning, Christopher D.",
editor = "M{\`a}rquez, Llu{\'\i}s and
Callison-Burch, Chris and
Su, Jian",
booktitle = "Proceedings of the 2015 Conference on Empirical Methods in Natural Language Processing",
month = sep,
year = "2015",
address = "Lisbon, Portugal",
publisher = "Association for Computational Linguistics",
url = "https://aclanthology.org/D15-1075",
doi = "10.18653/v1/D15-1075",
pages = "632--642",
}
The corpus includes content from the Flickr 30k corpus, which can be cited by way of this paper:
@article{young-etal-2014-image,
title = "From image descriptions to visual denotations: New similarity metrics for semantic inference over event descriptions",
author = "Young, Peter and
Lai, Alice and
Hodosh, Micah and
Hockenmaier, Julia",
editor = "Lin, Dekang and
Collins, Michael and
Lee, Lillian",
journal = "Transactions of the Association for Computational Linguistics",
volume = "2",
year = "2014",
address = "Cambridge, MA",
publisher = "MIT Press",
url = "https://aclanthology.org/Q14-1006",
doi = "10.1162/tacl_a_00166",
pages = "67--78",
}
Contact Information
For any comments or questions, please email Samuel Bowman, Gabor Angeli and Chris Manning.
Contributions
Thanks to @mariamabarham, @thomwolf, @lewtun, @patrickvonplaten and @mcmillanmajora for adding this dataset.
- Downloads last month
- 13,580
Models trained or fine-tuned on stanfordnlp/snli
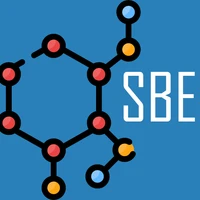