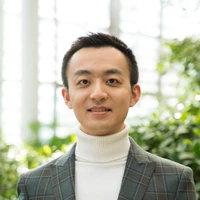
yuntian-deng/ak-paper-selection-deberta
Text Classification
•
Updated
•
18
•
5
arxiv_id
stringlengths 10
10
| published
stringlengths 20
20
| titles
stringlengths 9
243
| authors
sequencelengths 1
389
| abstract
stringlengths 96
3.09k
| categories
sequencelengths 1
10
| selected
bool 2
classes |
---|---|---|---|---|---|---|
2305.03264 | 2023-05-05T03:37:25Z | Robust Face Morphing Attack Detection Using Fusion of Multiple Features
and Classification Techniques | [
"Jag Mohan Singh Sushma Venkatesh Raghavendra Ramachandra"
] | Face Recognition System (FRS) are shown to be vulnerable to morphed images of
newborns. Detecting morphing attacks stemming from face images of newborn is
important to avoid unwanted consequences, both for security and society. In
this paper, we present a new reference-based/Differential Morphing Attack
Detection (MAD) method to detect newborn morphing images using Wavelet
Scattering Network (WSN). We propose a two-layer WSN with 250 $\times$ 250
pixels and six rotations of wavelets per layer, resulting in 577 paths. The
proposed approach is validated on a dataset of 852 bona fide images and 2460
morphing images constructed using face images of 42 unique newborns. The
obtained results indicate a gain of over 10\% in detection accuracy over other
existing D-MAD techniques. | [
"cs.CV"
] | false |
2305.03277 | 2023-05-05T04:28:48Z | FM-ViT: Flexible Modal Vision Transformers for Face Anti-Spoofing | [
"Ajian Liu",
"Zichang Tan",
"Zitong Yu",
"Chenxu Zhao",
"Jun Wan",
"Yanyan Liang",
"Zhen Lei",
"Du Zhang",
"Stan Z. Li",
"Guodong Guo"
] | The availability of handy multi-modal (i.e., RGB-D) sensors has brought about
a surge of face anti-spoofing research. However, the current multi-modal face
presentation attack detection (PAD) has two defects: (1) The framework based on
multi-modal fusion requires providing modalities consistent with the training
input, which seriously limits the deployment scenario. (2) The performance of
ConvNet-based model on high fidelity datasets is increasingly limited. In this
work, we present a pure transformer-based framework, dubbed the Flexible Modal
Vision Transformer (FM-ViT), for face anti-spoofing to flexibly target any
single-modal (i.e., RGB) attack scenarios with the help of available
multi-modal data. Specifically, FM-ViT retains a specific branch for each
modality to capture different modal information and introduces the Cross-Modal
Transformer Block (CMTB), which consists of two cascaded attentions named
Multi-headed Mutual-Attention (MMA) and Fusion-Attention (MFA) to guide each
modal branch to mine potential features from informative patch tokens, and to
learn modality-agnostic liveness features by enriching the modal information of
own CLS token, respectively. Experiments demonstrate that the single model
trained based on FM-ViT can not only flexibly evaluate different modal samples,
but also outperforms existing single-modal frameworks by a large margin, and
approaches the multi-modal frameworks introduced with smaller FLOPs and model
parameters. | [
"cs.CV"
] | false |
2305.03302 | 2023-05-05T06:10:15Z | High-Fidelity 3D Face Generation from Natural Language Descriptions | [
"Menghua Wu",
"Hao Zhu",
"Linjia Huang",
"Yiyu Zhuang",
"Yuanxun Lu",
"Xun Cao"
] | Synthesizing high-quality 3D face models from natural language descriptions
is very valuable for many applications, including avatar creation, virtual
reality, and telepresence. However, little research ever tapped into this task.
We argue the major obstacle lies in 1) the lack of high-quality 3D face data
with descriptive text annotation, and 2) the complex mapping relationship
between descriptive language space and shape/appearance space. To solve these
problems, we build Describe3D dataset, the first large-scale dataset with
fine-grained text descriptions for text-to-3D face generation task. Then we
propose a two-stage framework to first generate a 3D face that matches the
concrete descriptions, then optimize the parameters in the 3D shape and texture
space with abstract description to refine the 3D face model. Extensive
experimental results show that our method can produce a faithful 3D face that
conforms to the input descriptions with higher accuracy and quality than
previous methods. The code and Describe3D dataset are released at
https://github.com/zhuhao-nju/describe3d . | [
"cs.CV"
] | false |
2305.03327 | 2023-05-05T07:15:49Z | FlowText: Synthesizing Realistic Scene Text Video with Optical Flow
Estimation | [
"Yuzhong Zhao",
"Weijia Wu",
"Zhuang Li",
"Jiahong Li",
"Weiqiang Wang"
] | Current video text spotting methods can achieve preferable performance,
powered with sufficient labeled training data. However, labeling data manually
is time-consuming and labor-intensive. To overcome this, using low-cost
synthetic data is a promising alternative. This paper introduces a novel video
text synthesis technique called FlowText, which utilizes optical flow
estimation to synthesize a large amount of text video data at a low cost for
training robust video text spotters. Unlike existing methods that focus on
image-level synthesis, FlowText concentrates on synthesizing temporal
information of text instances across consecutive frames using optical flow.
This temporal information is crucial for accurately tracking and spotting text
in video sequences, including text movement, distortion, appearance,
disappearance, shelter, and blur. Experiments show that combining general
detectors like TransDETR with the proposed FlowText produces remarkable results
on various datasets, such as ICDAR2015video and ICDAR2013video. Code is
available at https://github.com/callsys/FlowText. | [
"cs.CV"
] | false |
2305.03347 | 2023-05-05T08:00:14Z | A Large Cross-Modal Video Retrieval Dataset with Reading Comprehension | [
"Weijia Wu",
"Yuzhong Zhao",
"Zhuang Li",
"Jiahong Li",
"Hong Zhou",
"Mike Zheng Shou",
"Xiang Bai"
] | Most existing cross-modal language-to-video retrieval (VR) research focuses
on single-modal input from video, i.e., visual representation, while the text
is omnipresent in human environments and frequently critical to understand
video. To study how to retrieve video with both modal inputs, i.e., visual and
text semantic representations, we first introduce a large-scale and cross-modal
Video Retrieval dataset with text reading comprehension, TextVR, which contains
42.2k sentence queries for 10.5k videos of 8 scenario domains, i.e., Street
View (indoor), Street View (outdoor), Games, Sports, Driving, Activity, TV
Show, and Cooking. The proposed TextVR requires one unified cross-modal model
to recognize and comprehend texts, relate them to the visual context, and
decide what text semantic information is vital for the video retrieval task.
Besides, we present a detailed analysis of TextVR compared to the existing
datasets and design a novel multimodal video retrieval baseline for the
text-based video retrieval task. The dataset analysis and extensive experiments
show that our TextVR benchmark provides many new technical challenges and
insights from previous datasets for the video-and-language community. The
project website and GitHub repo can be found at
https://sites.google.com/view/loveucvpr23/guest-track and
https://github.com/callsys/TextVR, respectively. | [
"cs.CV"
] | false |
2305.03351 | 2023-05-05T08:11:31Z | Leaf Cultivar Identification via Prototype-enhanced Learning | [
"Yiyi Zhang",
"Zhiwen Ying",
"Ying Zheng",
"Cuiling Wu",
"Nannan Li",
"Jun Wang",
"Xianzhong Feng",
"Xiaogang Xu"
] | Plant leaf identification is crucial for biodiversity protection and
conservation and has gradually attracted the attention of academia in recent
years. Due to the high similarity among different varieties, leaf cultivar
recognition is also considered to be an ultra-fine-grained visual
classification (UFGVC) task, which is facing a huge challenge. In practice, an
instance may be related to multiple varieties to varying degrees, especially in
the UFGVC datasets. However, deep learning methods trained on one-hot labels
fail to reflect patterns shared across categories and thus perform poorly on
this task. To address this issue, we generate soft targets integrated with
inter-class similarity information. Specifically, we continuously update the
prototypical features for each category and then capture the similarity scores
between instances and prototypes accordingly. Original one-hot labels and the
similarity scores are incorporated to yield enhanced labels. Prototype-enhanced
soft labels not only contain original one-hot label information, but also
introduce rich inter-category semantic association information, thus providing
more effective supervision for deep model training. Extensive experimental
results on public datasets show that our method can significantly improve the
performance on the UFGVC task of leaf cultivar identification. | [
"cs.CV"
] | false |
2305.03393 | 2023-05-05T09:38:47Z | Optimized Table Tokenization for Table Structure Recognition | [
"Maksym Lysak",
"Ahmed Nassar",
"Nikolaos Livathinos",
"Christoph Auer",
"Peter Staar"
] | Extracting tables from documents is a crucial task in any document conversion
pipeline. Recently, transformer-based models have demonstrated that
table-structure can be recognized with impressive accuracy using
Image-to-Markup-Sequence (Im2Seq) approaches. Taking only the image of a table,
such models predict a sequence of tokens (e.g. in HTML, LaTeX) which represent
the structure of the table. Since the token representation of the table
structure has a significant impact on the accuracy and run-time performance of
any Im2Seq model, we investigate in this paper how table-structure
representation can be optimised. We propose a new, optimised table-structure
language (OTSL) with a minimized vocabulary and specific rules. The benefits of
OTSL are that it reduces the number of tokens to 5 (HTML needs 28+) and
shortens the sequence length to half of HTML on average. Consequently, model
accuracy improves significantly, inference time is halved compared to
HTML-based models, and the predicted table structures are always syntactically
correct. This in turn eliminates most post-processing needs. | [
"cs.CV"
] | false |
2305.03425 | 2023-05-05T10:46:05Z | GAANet: Ghost Auto Anchor Network for Detecting Varying Size Drones in
Dark | [
"Misha Urooj Khan",
"Maham Misbah",
"Zeeshan Kaleem",
"Yansha Deng",
"Abbas Jamalipour"
] | The usage of drones has tremendously increased in different sectors spanning
from military to industrial applications. Despite all the benefits they offer,
their misuse can lead to mishaps, and tackling them becomes more challenging
particularly at night due to their small size and low visibility conditions. To
overcome those limitations and improve the detection accuracy at night, we
propose an object detector called Ghost Auto Anchor Network (GAANet) for
infrared (IR) images. The detector uses a YOLOv5 core to address challenges in
object detection for IR images, such as poor accuracy and a high false alarm
rate caused by extended altitudes, poor lighting, and low image resolution. To
improve performance, we implemented auto anchor calculation, modified the
conventional convolution block to ghost-convolution, adjusted the input channel
size, and used the AdamW optimizer. To enhance the precision of multiscale tiny
object recognition, we also introduced an additional extra-small object feature
extractor and detector. Experimental results in a custom IR dataset with
multiple classes (birds, drones, planes, and helicopters) demonstrate that
GAANet shows improvement compared to state-of-the-art detectors. In comparison
to GhostNet-YOLOv5, GAANet has higher overall mean average precision (mAP@50),
recall, and precision around 2.5\%, 2.3\%, and 1.4\%, respectively. The dataset
and code for this paper are available as open source at
https://github.com/ZeeshanKaleem/GhostAutoAnchorNet. | [
"cs.CV"
] | false |
2305.03487 | 2023-05-05T12:57:04Z | HD2Reg: Hierarchical Descriptors and Detectors for Point Cloud
Registration | [
"Canhui Tang",
"Yiheng Li",
"Shaoyi Du",
"Guofa Wang",
"Zhiqiang Tian"
] | Feature Descriptors and Detectors are two main components of feature-based
point cloud registration. However, little attention has been drawn to the
explicit representation of local and global semantics in the learning of
descriptors and detectors. In this paper, we present a framework that
explicitly extracts dual-level descriptors and detectors and performs
coarse-to-fine matching with them. First, to explicitly learn local and global
semantics, we propose a hierarchical contrastive learning strategy, training
the robust matching ability of high-level descriptors, and refining the local
feature space using low-level descriptors. Furthermore, we propose to learn
dual-level saliency maps that extract two groups of keypoints in two different
senses. To overcome the weak supervision of binary matchability labels, we
propose a ranking strategy to label the significance ranking of keypoints, and
thus provide more fine-grained supervision signals. Finally, we propose a
global-to-local matching scheme to obtain robust and accurate correspondences
by leveraging the complementary dual-level features.Quantitative experiments on
3DMatch and KITTI odometry datasets show that our method achieves robust and
accurate point cloud registration and outperforms recent keypoint-based
methods. | [
"cs.CV"
] | false |
2305.03595 | 2023-05-05T15:00:14Z | HSCNet++: Hierarchical Scene Coordinate Classification and Regression
for Visual Localization with Transformer | [
"Shuzhe Wang",
"Zakaria Laskar",
"Iaroslav Melekhov",
"Xiaotian Li",
"Yi Zhao",
"Giorgos Tolias",
"Juho Kannala"
] | Visual localization is critical to many applications in computer vision and
robotics. To address single-image RGB localization, state-of-the-art
feature-based methods match local descriptors between a query image and a
pre-built 3D model. Recently, deep neural networks have been exploited to
regress the mapping between raw pixels and 3D coordinates in the scene, and
thus the matching is implicitly performed by the forward pass through the
network. However, in a large and ambiguous environment, learning such a
regression task directly can be difficult for a single network. In this work,
we present a new hierarchical scene coordinate network to predict pixel scene
coordinates in a coarse-to-fine manner from a single RGB image. The proposed
method, which is an extension of HSCNet, allows us to train compact models
which scale robustly to large environments. It sets a new state-of-the-art for
single-image localization on the 7-Scenes, 12 Scenes, Cambridge Landmarks
datasets, and the combined indoor scenes. | [
"cs.CV"
] | false |
2305.03640 | 2023-05-05T15:56:46Z | Asynchronous Events-based Panoptic Segmentation using Graph Mixer Neural
Network | [
"Sanket Kachole",
"Yusra Alkendi",
"Fariborz Baghaei Naeini",
"Dimitrios Makris",
"Yahya Zweiri"
] | In the context of robotic grasping, object segmentation encounters several
difficulties when faced with dynamic conditions such as real-time operation,
occlusion, low lighting, motion blur, and object size variability. In response
to these challenges, we propose the Graph Mixer Neural Network that includes a
novel collaborative contextual mixing layer, applied to 3D event graphs formed
on asynchronous events. The proposed layer is designed to spread spatiotemporal
correlation within an event graph at four nearest neighbor levels parallelly.
We evaluate the effectiveness of our proposed method on the Event-based
Segmentation (ESD) Dataset, which includes five unique image degradation
challenges, including occlusion, blur, brightness, trajectory, scale variance,
and segmentation of known and unknown objects. The results show that our
proposed approach outperforms state-of-the-art methods in terms of mean
intersection over the union and pixel accuracy. Code available at:
https://github.com/sanket0707/GNN-Mixer.git | [
"cs.CV"
] | false |
2305.03226 | 2023-05-05T01:03:37Z | Sign-Coded Exposure Sensing for Noise-Robust High-Speed Imaging | [
"R. Wes Baldwin",
"Vijayan Asari",
"Keigo Hirakawa"
] | We present a novel Fourier camera, an in-hardware optical compression of
high-speed frames employing pixel-level sign-coded exposure where pixel
intensities temporally modulated as positive and negative exposure are combined
to yield Hadamard coefficients. The orthogonality of Walsh functions ensures
that the noise is not amplified during high-speed frame reconstruction, making
it a much more attractive option for coded exposure systems aimed at very high
frame rate operation. Frame reconstruction is carried out by a single-pass
demosaicking of the spatially multiplexed Walsh functions in a lattice
arrangement, significantly reducing the computational complexity. The
simulation prototype confirms the improved robustness to noise compared to the
binary-coded exposure patterns, such as one-hot encoding and pseudo-random
encoding. Our hardware prototype demonstrated the reconstruction of 4kHz frames
of a moving scene lit by ambient light only. | [
"eess.IV",
"cs.CV"
] | false |
2305.03252 | 2023-05-05T02:43:16Z | HeteroEdge: Addressing Asymmetry in Heterogeneous Collaborative
Autonomous Systems | [
"Mohammad Saeid Anwar",
"Emon Dey",
"Maloy Kumar Devnath",
"Indrajeet Ghosh",
"Naima Khan",
"Jade Freeman",
"Timothy Gregory",
"Niranjan Suri",
"Kasthuri Jayaraja",
"Sreenivasan Ramasamy Ramamurthy",
"Nirmalya Roy"
] | Gathering knowledge about surroundings and generating situational awareness
for IoT devices is of utmost importance for systems developed for smart urban
and uncontested environments. For example, a large-area surveillance system is
typically equipped with multi-modal sensors such as cameras and LIDARs and is
required to execute deep learning algorithms for action, face, behavior, and
object recognition. However, these systems face power and memory constraints
due to their ubiquitous nature, making it crucial to optimize data processing,
deep learning algorithm input, and model inference communication. In this
paper, we propose a self-adaptive optimization framework for a testbed
comprising two Unmanned Ground Vehicles (UGVs) and two NVIDIA Jetson devices.
This framework efficiently manages multiple tasks (storage, processing,
computation, transmission, inference) on heterogeneous nodes concurrently. It
involves compressing and masking input image frames, identifying similar
frames, and profiling devices to obtain boundary conditions for optimization..
Finally, we propose and optimize a novel parameter split-ratio, which indicates
the proportion of the data required to be offloaded to another device while
considering the networking bandwidth, busy factor, memory (CPU, GPU, RAM), and
power constraints of the devices in the testbed. Our evaluations captured while
executing multiple tasks (e.g., PoseNet, SegNet, ImageNet, DetectNet, DepthNet)
simultaneously, reveal that executing 70% (split-ratio=70%) of the data on the
auxiliary node minimizes the offloading latency by approx. 33% (18.7 ms/image
to 12.5 ms/image) and the total operation time by approx. 47% (69.32s to
36.43s) compared to the baseline configuration (executing on the primary node). | [
"cs.DC",
"cs.CV"
] | false |
2305.03289 | 2023-05-05T05:39:12Z | BadSAM: Exploring Security Vulnerabilities of SAM via Backdoor Attacks | [
"Zihan Guan",
"Mengxuan Hu",
"Zhongliang Zhou",
"Jielu Zhang",
"Sheng Li",
"Ninghao Liu"
] | Recently, the Segment Anything Model (SAM) has gained significant attention
as an image segmentation foundation model due to its strong performance on
various downstream tasks. However, it has been found that SAM does not always
perform satisfactorily when faced with challenging downstream tasks. This has
led downstream users to demand a customized SAM model that can be adapted to
these downstream tasks. In this paper, we present BadSAM, the first backdoor
attack on the image segmentation foundation model. Our preliminary experiments
on the CAMO dataset demonstrate the effectiveness of BadSAM. | [
"cs.CV",
"cs.AI"
] | false |
2305.03343 | 2023-05-05T07:53:13Z | LOGO-Former: Local-Global Spatio-Temporal Transformer for Dynamic Facial
Expression Recognition | [
"Fuyan Ma",
"Bin Sun",
"Shutao Li"
] | Previous methods for dynamic facial expression recognition (DFER) in the wild
are mainly based on Convolutional Neural Networks (CNNs), whose local
operations ignore the long-range dependencies in videos. Transformer-based
methods for DFER can achieve better performances but result in higher FLOPs and
computational costs. To solve these problems, the local-global spatio-temporal
Transformer (LOGO-Former) is proposed to capture discriminative features within
each frame and model contextual relationships among frames while balancing the
complexity. Based on the priors that facial muscles move locally and facial
expressions gradually change, we first restrict both the space attention and
the time attention to a local window to capture local interactions among
feature tokens. Furthermore, we perform the global attention by querying a
token with features from each local window iteratively to obtain long-range
information of the whole video sequence. In addition, we propose the compact
loss regularization term to further encourage the learned features have the
minimum intra-class distance and the maximum inter-class distance. Experiments
on two in-the-wild dynamic facial expression datasets (i.e., DFEW and FERV39K)
indicate that our method provides an effective way to make use of the spatial
and temporal dependencies for DFER. | [
"cs.CV",
"cs.MM"
] | false |
2305.03352 | 2023-05-05T08:13:53Z | Contrastive Learning for Low-light Raw Denoising | [
"Taoyong Cui",
"Yuhan Dong"
] | Image/video denoising in low-light scenes is an extremely challenging problem
due to limited photon count and high noise. In this paper, we propose a novel
approach with contrastive learning to address this issue. Inspired by the
success of contrastive learning used in some high-level computer vision tasks,
we bring in this idea to the low-level denoising task. In order to achieve this
goal, we introduce a new denoising contrastive regularization (DCR) to exploit
the information of noisy images and clean images. In the feature space, DCR
makes the denoised image closer to the clean image and far away from the noisy
image. In addition, we build a new feature embedding network called Wnet, which
is more effective to extract high-frequency information. We conduct the
experiments on a real low-light dataset that captures still images taken on a
moonless clear night in 0.6 millilux and videos under starlight (no moon
present, <0.001 lux). The results show that our method can achieve a higher
PSNR and better visual quality compared with existing methods | [
"cs.CV",
"cs.AI"
] | false |
2305.03378 | 2023-05-05T09:16:06Z | Towards Effective Collaborative Learning in Long-Tailed Recognition | [
"Zhengzhuo Xu",
"Zenghao Chai",
"Chengyin Xu",
"Chun Yuan",
"Haiqin Yang"
] | Real-world data usually suffers from severe class imbalance and long-tailed
distributions, where minority classes are significantly underrepresented
compared to the majority ones. Recent research prefers to utilize multi-expert
architectures to mitigate the model uncertainty on the minority, where
collaborative learning is employed to aggregate the knowledge of experts, i.e.,
online distillation. In this paper, we observe that the knowledge transfer
between experts is imbalanced in terms of class distribution, which results in
limited performance improvement of the minority classes. To address it, we
propose a re-weighted distillation loss by comparing two classifiers'
predictions, which are supervised by online distillation and label annotations,
respectively. We also emphasize that feature-level distillation will
significantly improve model performance and increase feature robustness.
Finally, we propose an Effective Collaborative Learning (ECL) framework that
integrates a contrastive proxy task branch to further improve feature quality.
Quantitative and qualitative experiments on four standard datasets demonstrate
that ECL achieves state-of-the-art performance and the detailed ablation
studies manifest the effectiveness of each component in ECL. | [
"cs.CV",
"cs.LG"
] | false |
2305.03383 | 2023-05-05T09:28:22Z | WWFedCBMIR: World-Wide Federated Content-Based Medical Image Retrieval | [
"Zahra Tabatabaei",
"Yuandou Wang",
"Adrián Colomer",
"Javier Oliver Moll",
"Zhiming Zhao",
"Valery Naranjo"
] | The paper proposes a Federated Content-Based Medical Image Retrieval
(FedCBMIR) platform that utilizes Federated Learning (FL) to address the
challenges of acquiring a diverse medical data set for training CBMIR models.
CBMIR assists pathologists in diagnosing breast cancer more rapidly by
identifying similar medical images and relevant patches in prior cases compared
to traditional cancer detection methods. However, CBMIR in histopathology
necessitates a pool of Whole Slide Images (WSIs) to train to extract an optimal
embedding vector that leverages search engine performance, which may not be
available in all centers. The strict regulations surrounding data sharing in
medical data sets also hinder research and model development, making it
difficult to collect a rich data set. The proposed FedCBMIR distributes the
model to collaborative centers for training without sharing the data set,
resulting in shorter training times than local training. FedCBMIR was evaluated
in two experiments with three scenarios on BreaKHis and Camelyon17 (CAM17). The
study shows that the FedCBMIR method increases the F1-Score (F1S) of each
client to 98%, 96%, 94%, and 97% in the BreaKHis experiment with a generalized
model of four magnifications and does so in 6.30 hours less time than total
local training. FedCBMIR also achieves 98% accuracy with CAM17 in 2.49 hours
less training time than local training, demonstrating that our FedCBMIR is both
fast and accurate for both pathologists and engineers. In addition, our
FedCBMIR provides similar images with higher magnification for non-developed
countries where participate in the worldwide FedCBMIR with developed countries
to facilitate mitosis measuring in breast cancer diagnosis. We evaluate this
scenario by scattering BreaKHis into four centers with different
magnifications. | [
"eess.IV",
"cs.CV"
] | false |
2305.03387 | 2023-05-05T09:33:34Z | AsConvSR: Fast and Lightweight Super-Resolution Network with Assembled
Convolutions | [
"Jiaming Guo",
"Xueyi Zou",
"Yuyi Chen",
"Yi Liu",
"Jia Hao",
"Jianzhuang Liu",
"Youliang Yan"
] | In recent years, videos and images in 720p (HD), 1080p (FHD) and 4K (UHD)
resolution have become more popular for display devices such as TVs, mobile
phones and VR. However, these high resolution images cannot achieve the
expected visual effect due to the limitation of the internet bandwidth, and
bring a great challenge for super-resolution networks to achieve real-time
performance. Following this challenge, we explore multiple efficient network
designs, such as pixel-unshuffle, repeat upscaling, and local skip connection
removal, and propose a fast and lightweight super-resolution network.
Furthermore, by analyzing the applications of the idea of divide-and-conquer in
super-resolution, we propose assembled convolutions which can adapt convolution
kernels according to the input features. Experiments suggest that our method
outperforms all the state-of-the-art efficient super-resolution models, and
achieves optimal results in terms of runtime and quality. In addition, our
method also wins the first place in NTIRE 2023 Real-Time Super-Resolution -
Track 1 ($\times$2). The code will be available at
https://gitee.com/mindspore/models/tree/master/research/cv/AsConvSR | [
"eess.IV",
"cs.CV"
] | false |
2305.03416 | 2023-05-05T10:29:29Z | Evolution under Length Constraints for CNN Architecture design | [
"Ousmane Youme",
"Jean Marie Dembele",
"Eugene C. Ezin",
"Christophe Cambier"
] | In recent years, the CNN architectures designed by evolution algorithms have
proven to be competitive with handcrafted architectures designed by experts.
However, these algorithms need a lot of computational power, which is beyond
the capabilities of most researchers and engineers. To overcome this problem,
we propose an evolution architecture under length constraints. It consists of
two algorithms: a search length strategy to find an optimal space and a search
architecture strategy based on genetic algorithm to find the best individual in
the optimal space. Our algorithms reduce drastically resource cost and also
keep good performance. On the Cifar-10 dataset, our framework presents
outstanding performance with an error rate of 5.12% and only 4.6 GPU a day to
converge to the optimal individual -22 GPU a day less than the lowest cost
automatic evolutionary algorithm in the peer competition. | [
"cs.CV",
"cs.NE"
] | false |
2305.03500 | 2023-05-05T13:20:41Z | High-Level Context Representation for Emotion Recognition in Images | [
"Willams de Lima Costa",
"Estefania Talavera Martinez",
"Lucas Silva Figueiredo",
"Veronica Teichrieb"
] | Emotion recognition is the task of classifying perceived emotions in people.
Previous works have utilized various nonverbal cues to extract features from
images and correlate them to emotions. Of these cues, situational context is
particularly crucial in emotion perception since it can directly influence the
emotion of a person. In this paper, we propose an approach for high-level
context representation extraction from images. The model relies on a single cue
and a single encoding stream to correlate this representation with emotions.
Our model competes with the state-of-the-art, achieving an mAP of 0.3002 on the
EMOTIC dataset while also being capable of execution on consumer-grade hardware
at approximately 90 frames per second. Overall, our approach is more efficient
than previous models and can be easily deployed to address real-world problems
related to emotion recognition. | [
"cs.CV",
"cs.HC"
] | false |
2305.03601 | 2023-05-05T15:05:07Z | Human Attention-Guided Explainable Artificial Intelligence for Computer
Vision Models | [
"Guoyang Liu",
"Jindi Zhang",
"Antoni B. Chan",
"Janet H. Hsiao"
] | We examined whether embedding human attention knowledge into saliency-based
explainable AI (XAI) methods for computer vision models could enhance their
plausibility and faithfulness. We first developed new gradient-based XAI
methods for object detection models to generate object-specific explanations by
extending the current methods for image classification models. Interestingly,
while these gradient-based methods worked well for explaining image
classification models, when being used for explaining object detection models,
the resulting saliency maps generally had lower faithfulness than human
attention maps when performing the same task. We then developed Human
Attention-Guided XAI (HAG-XAI) to learn from human attention how to best
combine explanatory information from the models to enhance explanation
plausibility by using trainable activation functions and smoothing kernels to
maximize XAI saliency map's similarity to human attention maps. While for image
classification models, HAG-XAI enhanced explanation plausibility at the expense
of faithfulness, for object detection models it enhanced plausibility and
faithfulness simultaneously and outperformed existing methods. The learned
functions were model-specific, well generalizable to other databases. | [
"cs.CV",
"cs.AI",
"68T45",
"I.2.0; I.4.0"
] | false |
2305.03706 | 2023-05-05T17:38:00Z | Fine-Grained Product Classification on Leaflet Advertisements | [
"Daniel Ladwig",
"Bianca Lamm",
"Janis Keuper"
] | In this paper, we describe a first publicly available fine-grained product
recognition dataset based on leaflet images. Using advertisement leaflets,
collected over several years from different European retailers, we provide a
total of 41.6k manually annotated product images in 832 classes. Further, we
investigate three different approaches for this fine-grained product
classification task, Classification by Image, by Text, as well as by Image and
Text. The approach "Classification by Text" uses the text extracted directly
from the leaflet product images. We show, that the combination of image and
text as input improves the classification of visual difficult to distinguish
products. The final model leads to an accuracy of 96.4% with a Top-3 score of
99.2%. We release our code at
https://github.com/ladwigd/Leaflet-Product-Classification. | [
"cs.CV",
"cs.LG"
] | false |
2305.03726 | 2023-05-05T17:59:46Z | Otter: A Multi-Modal Model with In-Context Instruction Tuning | [
"Bo Li",
"Yuanhan Zhang",
"Liangyu Chen",
"Jinghao Wang",
"Jingkang Yang",
"Ziwei Liu"
] | Large language models (LLMs) have demonstrated significant universal
capabilities as few/zero-shot learners in various tasks due to their
pre-training on vast amounts of text data, as exemplified by GPT-3, which
boosted to InstrctGPT and ChatGPT, effectively following natural language
instructions to accomplish real-world tasks. In this paper, we propose to
introduce instruction tuning into multi-modal models, motivated by the Flamingo
model's upstream interleaved format pretraining dataset. We adopt a similar
approach to construct our MultI-Modal In-Context Instruction Tuning (MIMIC-IT)
dataset. We then introduce Otter, a multi-modal model based on OpenFlamingo
(open-sourced version of DeepMind's Flamingo), trained on MIMIC-IT and
showcasing improved instruction-following ability and in-context learning. We
also optimize OpenFlamingo's implementation for researchers, democratizing the
required training resources from 1$\times$ A100 GPU to 4$\times$ RTX-3090 GPUs,
and integrate both OpenFlamingo and Otter into Huggingface Transformers for
more researchers to incorporate the models into their customized training and
inference pipelines. | [
"cs.CV",
"cs.CL"
] | true |
2305.03810 | 2023-05-05T19:26:06Z | Distilled Mid-Fusion Transformer Networks for Multi-Modal Human Activity
Recognition | [
"Jingcheng Li",
"Lina Yao",
"Binghao Li",
"Claude Sammut"
] | Human Activity Recognition is an important task in many human-computer
collaborative scenarios, whilst having various practical applications. Although
uni-modal approaches have been extensively studied, they suffer from data
quality and require modality-specific feature engineering, thus not being
robust and effective enough for real-world deployment. By utilizing various
sensors, Multi-modal Human Activity Recognition could utilize the complementary
information to build models that can generalize well. While deep learning
methods have shown promising results, their potential in extracting salient
multi-modal spatial-temporal features and better fusing complementary
information has not been fully explored. Also, reducing the complexity of the
multi-modal approach for edge deployment is another problem yet to resolve. To
resolve the issues, a knowledge distillation-based Multi-modal Mid-Fusion
approach, DMFT, is proposed to conduct informative feature extraction and
fusion to resolve the Multi-modal Human Activity Recognition task efficiently.
DMFT first encodes the multi-modal input data into a unified representation.
Then the DMFT teacher model applies an attentive multi-modal spatial-temporal
transformer module that extracts the salient spatial-temporal features. A
temporal mid-fusion module is also proposed to further fuse the temporal
features. Then the knowledge distillation method is applied to transfer the
learned representation from the teacher model to a simpler DMFT student model,
which consists of a lite version of the multi-modal spatial-temporal
transformer module, to produce the results. Evaluation of DMFT was conducted on
two public multi-modal human activity recognition datasets with various
state-of-the-art approaches. The experimental results demonstrate that the
model achieves competitive performance in terms of effectiveness, scalability,
and robustness. | [
"cs.HC",
"cs.CV"
] | false |
2305.03844 | 2023-05-05T20:47:42Z | Physics-based network fine-tuning for robust quantitative susceptibility
mapping from high-pass filtered phase | [
"Jinwei Zhang",
"Alexey Dimov",
"Chao Li",
"Hang Zhang",
"Thanh D. Nguyen",
"Pascal Spincemaille",
"Yi Wang"
] | Purpose: To improve the generalization ability of convolutional neural
network (CNN) based prediction of quantitative susceptibility mapping (QSM)
from high-pass filtered phase (HPFP) image. Methods: The proposed network
addresses two common generalization issues that arise when using a pre-trained
network to predict QSM from HPFP: a) data with unseen voxel sizes, and b) data
with unknown high-pass filter parameters. A network fine-tuning step based on a
high-pass filtering dipole convolution forward model is proposed to reduce the
generalization error of the pre-trained network. A progressive Unet
architecture is proposed to improve prediction accuracy without increasing
fine-tuning computational cost. Results: In retrospective studies using RMSE,
PSNR, SSIM and HFEN as quality metrics, the performance of both Unet and
progressive Unet was improved after physics-based fine-tuning at all voxel
sizes and most high-pass filtering cutoff frequencies tested in the experiment.
Progressive Unet slightly outperformed Unet both before and after fine-tuning.
In a prospective study, image sharpness was improved after physics-based
fine-tuning for both Unet and progressive Unet. Compared to Unet, progressive
Unet had better agreement of regional susceptibility values with reference QSM.
Conclusion: The proposed method shows improved robustness compared to the
pre-trained network without fine-tuning when the test dataset deviates from
training. Our code is available at https://github.com/Jinwei1209/SWI_to_QSM/ | [
"eess.IV",
"cs.CV"
] | false |
2305.13261 | 2023-05-05T07:44:23Z | A Review of Benchmarks for Visual Defect Detection in the Manufacturing
Industry | [
"Philippe Carvalho",
"Alexandre Durupt",
"Yves Grandvalet"
] | The field of industrial defect detection using machine learning and deep
learning is a subject of active research. Datasets, also called benchmarks, are
used to compare and assess research results. There is a number of datasets in
industrial visual inspection, of varying quality. Thus, it is a difficult task
to determine which dataset to use. Generally speaking, datasets which include a
testing set, with precise labeling and made in real-world conditions should be
preferred. We propose a study of existing benchmarks to compare and expose
their characteristics and their use-cases. A study of industrial metrics
requirements, as well as testing procedures, will be presented and applied to
the studied benchmarks. We discuss our findings by examining the current state
of benchmarks for industrial visual inspection, and by exposing guidelines on
the usage of benchmarks. | [
"cs.CV",
"cs.LG"
] | false |
2305.03273 | 2023-05-05T04:11:00Z | Semantic Segmentation using Vision Transformers: A survey | [
"Hans Thisanke",
"Chamli Deshan",
"Kavindu Chamith",
"Sachith Seneviratne",
"Rajith Vidanaarachchi",
"Damayanthi Herath"
] | Semantic segmentation has a broad range of applications in a variety of
domains including land coverage analysis, autonomous driving, and medical image
analysis. Convolutional neural networks (CNN) and Vision Transformers (ViTs)
provide the architecture models for semantic segmentation. Even though ViTs
have proven success in image classification, they cannot be directly applied to
dense prediction tasks such as image segmentation and object detection since
ViT is not a general purpose backbone due to its patch partitioning scheme. In
this survey, we discuss some of the different ViT architectures that can be
used for semantic segmentation and how their evolution managed the above-stated
challenge. The rise of ViT and its performance with a high success rate
motivated the community to slowly replace the traditional convolutional neural
networks in various computer vision tasks. This survey aims to review and
compare the performances of ViT architectures designed for semantic
segmentation using benchmarking datasets. This will be worthwhile for the
community to yield knowledge regarding the implementations carried out in
semantic segmentation and to discover more efficient methodologies using ViTs. | [
"cs.CV",
"cs.AI",
"cs.LG"
] | false |
2305.03330 | 2023-05-05T07:22:20Z | Solution existence, uniqueness, and stability of discrete basis
sinograms in multispectral CT | [
"Yu Gao",
"Xiaochuan Pan",
"Chong Chen"
] | This work investigates conditions for quantitative image reconstruction in
multispectral computed tomography (MSCT), which remains a topic of active
research. In MSCT, one seeks to obtain from data the spatial distribution of
linear attenuation coefficient, referred to as a virtual monochromatic image
(VMI), at a given X-ray energy, within the subject imaged. As a VMI is
decomposed often into a linear combination of basis images with known
decomposition coefficients, the reconstruction of a VMI is thus tantamount to
that of the basis images. An empirical, but highly effective, two-step
data-domain-decomposition (DDD) method has been developed and used widely for
quantitative image reconstruction in MSCT. In the two-step DDD method, step (1)
estimates the so-called basis sinogram from data through solving a nonlinear
transform, whereas step (2) reconstructs basis images from their basis
sinograms estimated. Subsequently, a VMI can readily be obtained from the
linear combination of basis images reconstructed. As step (2) involves the
inversion of a straightforward linear system, step (1) is the key component of
the DDD method in which a nonlinear system needs to be inverted for estimating
the basis sinograms from data. In this work, we consider a {\it discrete} form
of the nonlinear system in step (1), and then carry out theoretical and
numerical analyses of conditions on the existence, uniqueness, and stability of
a solution to the discrete nonlinear system for accurately estimating the
discrete basis sinograms, leading to quantitative reconstruction of VMIs in
MSCT. | [
"math.NA",
"cs.CV",
"cs.NA",
"physics.med-ph",
"65R32, 94A08, 65F22, 65J22, 65D18"
] | false |
2305.03350 | 2023-05-05T08:11:00Z | Reconstructing Training Data from Multiclass Neural Networks | [
"Gon Buzaglo",
"Niv Haim",
"Gilad Yehudai",
"Gal Vardi",
"Michal Irani"
] | Reconstructing samples from the training set of trained neural networks is a
major privacy concern. Haim et al. (2022) recently showed that it is possible
to reconstruct training samples from neural network binary classifiers, based
on theoretical results about the implicit bias of gradient methods. In this
work, we present several improvements and new insights over this previous work.
As our main improvement, we show that training-data reconstruction is possible
in the multi-class setting and that the reconstruction quality is even higher
than in the case of binary classification. Moreover, we show that using
weight-decay during training increases the vulnerability to sample
reconstruction. Finally, while in the previous work the training set was of
size at most $1000$ from $10$ classes, we show preliminary evidence of the
ability to reconstruct from a model trained on $5000$ samples from $100$
classes. | [
"cs.LG",
"cs.CR",
"cs.CV"
] | false |
2305.03413 | 2023-05-05T10:26:50Z | Domain-agnostic segmentation of thalamic nuclei from joint structural
and diffusion MRI | [
"Henry F. J. Tregidgo",
"Sonja Soskic",
"Mark D. Olchanyi",
"Juri Althonayan",
"Benjamin Billot",
"Chiara Maffei",
"Polina Golland",
"Anastasia Yendiki",
"Daniel C. Alexander",
"Martina Bocchetta",
"Jonathan D. Rohrer",
"Juan Eugenio Iglesias"
] | The human thalamus is a highly connected subcortical grey-matter structure
within the brain. It comprises dozens of nuclei with different function and
connectivity, which are affected differently by disease. For this reason, there
is growing interest in studying the thalamic nuclei in vivo with MRI. Tools are
available to segment the thalamus from 1 mm T1 scans, but the contrast of the
lateral and internal boundaries is too faint to produce reliable segmentations.
Some tools have attempted to incorporate information from diffusion MRI in the
segmentation to refine these boundaries, but do not generalise well across
diffusion MRI acquisitions. Here we present the first CNN that can segment
thalamic nuclei from T1 and diffusion data of any resolution without retraining
or fine tuning. Our method builds on a public histological atlas of the
thalamic nuclei and silver standard segmentations on high-quality diffusion
data obtained with a recent Bayesian adaptive segmentation tool. We combine
these with an approximate degradation model for fast domain randomisation
during training. Our CNN produces a segmentation at 0.7 mm isotropic
resolution, irrespective of the resolution of the input. Moreover, it uses a
parsimonious model of the diffusion signal at each voxel (fractional anisotropy
and principal eigenvector) that is compatible with virtually any set of
directions and b-values, including huge amounts of legacy data. We show results
of our proposed method on three heterogeneous datasets acquired on dozens of
different scanners. An implementation of the method is publicly available at
https://freesurfer.net/fswiki/ThalamicNucleiDTI. | [
"eess.IV",
"cs.CV",
"cs.LG",
"q-bio.QM"
] | false |
2305.03572 | 2023-05-05T14:29:24Z | Learn how to Prune Pixels for Multi-view Neural Image-based Synthesis | [
"Marta Milovanović",
"Enzo Tartaglione",
"Marco Cagnazzo",
"Félix Henry"
] | Image-based rendering techniques stand at the core of an immersive experience
for the user, as they generate novel views given a set of multiple input
images. Since they have shown good performance in terms of objective and
subjective quality, the research community devotes great effort to their
improvement. However, the large volume of data necessary to render at the
receiver's side hinders applications in limited bandwidth environments or
prevents their employment in real-time applications. We present LeHoPP, a
method for input pixel pruning, where we examine the importance of each input
pixel concerning the rendered view, and we avoid the use of irrelevant pixels.
Even without retraining the image-based rendering network, our approach shows a
good trade-off between synthesis quality and pixel rate. When tested in the
general neural rendering framework, compared to other pruning baselines, LeHoPP
gains between $0.9$ dB and $3.6$ dB on average. | [
"cs.MM",
"cs.AI",
"cs.CV"
] | false |
2305.03691 | 2023-05-05T17:09:01Z | Mining bias-target Alignment from Voronoi Cells | [
"Rémi Nahon",
"Van-Tam Nguyen",
"Enzo Tartaglione"
] | Despite significant research efforts, deep neural networks are still
vulnerable to biases: this raises concerns about their fairness and limits
their generalization. In this paper, we propose a bias-agnostic approach to
mitigate the impact of bias in deep neural networks. Unlike traditional
debiasing approaches, we rely on a metric to quantify ``bias
alignment/misalignment'' on target classes, and use this information to
discourage the propagation of bias-target alignment information through the
network. We conduct experiments on several commonly used datasets for debiasing
and compare our method to supervised and bias-specific approaches. Our results
indicate that the proposed method achieves comparable performance to
state-of-the-art supervised approaches, although it is bias-agnostic, even in
presence of multiple biases in the same sample. | [
"cs.LG",
"cs.AI",
"cs.CV",
"cs.CY"
] | false |
2305.03724 | 2023-05-05T17:58:45Z | DualCross: Cross-Modality Cross-Domain Adaptation for Monocular BEV
Perception | [
"Yunze Man",
"Liang-Yan Gui",
"Yu-Xiong Wang"
] | Closing the domain gap between training and deployment and incorporating
multiple sensor modalities are two challenging yet critical topics for
self-driving. Existing work only focuses on single one of the above topics,
overlooking the simultaneous domain and modality shift which pervasively exists
in real-world scenarios. A model trained with multi-sensor data collected in
Europe may need to run in Asia with a subset of input sensors available. In
this work, we propose DualCross, a cross-modality cross-domain adaptation
framework to facilitate the learning of a more robust monocular bird's-eye-view
(BEV) perception model, which transfers the point cloud knowledge from a LiDAR
sensor in one domain during the training phase to the camera-only testing
scenario in a different domain. This work results in the first open analysis of
cross-domain cross-sensor perception and adaptation for monocular 3D tasks in
the wild. We benchmark our approach on large-scale datasets under a wide range
of domain shifts and show state-of-the-art results against various baselines. | [
"cs.CV",
"cs.AI",
"cs.RO"
] | false |
2305.03253 | 2023-05-05T02:46:22Z | VicunaNER: Zero/Few-shot Named Entity Recognition using Vicuna | [
"Bin Ji"
] | Large Language Models (LLMs, e.g., ChatGPT) have shown impressive zero- and
few-shot capabilities in Named Entity Recognition (NER). However, these models
can only be accessed via online APIs, which may cause data leak and
non-reproducible problems. In this paper, we propose VicunaNER, a zero/few-shot
NER framework based on the newly released open-source LLM -- Vicuna. VicunaNER
is a two-phase framework, where each phase leverages multi-turn dialogues with
Vicuna to recognize entities from texts. We name the second phase as
Re-Recognition, which recognizes those entities not recognized in the first
phase (a.k.a. Recognition). Moreover, we set entity correctness check dialogues
in each phase to filter out wrong entities. We evaluate VicunaNER's zero-shot
capacity on 10 datasets crossing 5 domains and few-shot capacity on Few-NERD.
Experimental results demonstrate that VicunaNER achieves superior performance
in both shot settings. Additionally, we conduct comprehensive investigations on
Vicuna from multiple perspectives. | [
"cs.CL"
] | false |
2305.03256 | 2023-05-05T03:02:41Z | Stylized Data-to-Text Generation: A Case Study in the E-Commerce Domain | [
"Liqiang Jing",
"Xuemeng Song",
"Xuming Lin",
"Zhongzhou Zhao",
"Wei Zhou",
"Liqiang Nie"
] | Existing data-to-text generation efforts mainly focus on generating a
coherent text from non-linguistic input data, such as tables and
attribute-value pairs, but overlook that different application scenarios may
require texts of different styles. Inspired by this, we define a new task,
namely stylized data-to-text generation, whose aim is to generate coherent text
for the given non-linguistic data according to a specific style. This task is
non-trivial, due to three challenges: the logic of the generated text,
unstructured style reference, and biased training samples. To address these
challenges, we propose a novel stylized data-to-text generation model, named
StyleD2T, comprising three components: logic planning-enhanced data embedding,
mask-based style embedding, and unbiased stylized text generation. In the first
component, we introduce a graph-guided logic planner for attribute organization
to ensure the logic of generated text. In the second component, we devise
feature-level mask-based style embedding to extract the essential style signal
from the given unstructured style reference. In the last one, pseudo triplet
augmentation is utilized to achieve unbiased text generation, and a
multi-condition based confidence assignment function is designed to ensure the
quality of pseudo samples. Extensive experiments on a newly collected dataset
from Taobao have been conducted, and the results show the superiority of our
model over existing methods. | [
"cs.CL"
] | false |
2305.03268 | 2023-05-05T03:49:14Z | Verify-and-Edit: A Knowledge-Enhanced Chain-of-Thought Framework | [
"Ruochen Zhao",
"Xingxuan Li",
"Shafiq Joty",
"Chengwei Qin",
"Lidong Bing"
] | As large language models (LLMs) have become the norm in NLP, demonstrating
good performance in generation and reasoning tasks, one of its most fatal
disadvantages is the lack of factual correctness. Generating unfactual texts
not only leads to lower performances but also degrades the trust and validity
of their applications. Chain-of-Thought (CoT) prompting improves trust and
model performance on complex reasoning tasks by generating interpretable
reasoning chains, but still suffers from factuality concerns in
knowledge-intensive tasks. In this paper, we propose the Verify-and-Edit
framework for CoT prompting, which seeks to increase prediction factuality by
post-editing reasoning chains according to external knowledge. Building on top
of GPT-3, our framework lead to accuracy improvements in multiple open-domain
question-answering tasks. | [
"cs.CL"
] | false |
2305.03296 | 2023-05-05T05:50:26Z | TransESC: Smoothing Emotional Support Conversation via Turn-Level State
Transition | [
"Weixiang Zhao",
"Yanyan Zhao",
"Shilong Wang",
"Bing Qin"
] | Emotion Support Conversation (ESC) is an emerging and challenging task with
the goal of reducing the emotional distress of people. Previous attempts fail
to maintain smooth transitions between utterances in ESC because they ignore to
grasp the fine-grained transition information at each dialogue turn. To solve
this problem, we propose to take into account turn-level state
\textbf{Trans}itions of \textbf{ESC} (\textbf{TransESC}) from three
perspectives, including semantics transition, strategy transition and emotion
transition, to drive the conversation in a smooth and natural way.
Specifically, we construct the state transition graph with a two-step way,
named transit-then-interact, to grasp such three types of turn-level transition
information. Finally, they are injected into the transition-aware decoder to
generate more engaging responses. Both automatic and human evaluations on the
benchmark dataset demonstrate the superiority of TransESC to generate more
smooth and effective supportive responses. Our source code is available at
\url{https://github.com/circle-hit/TransESC}. | [
"cs.CL"
] | false |
2305.03300 | 2023-05-05T06:05:45Z | LLM-RM at SemEval-2023 Task 2: Multilingual Complex NER using
XLM-RoBERTa | [
"Rahul Mehta",
"Vasudeva Varma"
] | Named Entity Recognition(NER) is a task of recognizing entities at a token
level in a sentence. This paper focuses on solving NER tasks in a multilingual
setting for complex named entities. Our team, LLM-RM participated in the
recently organized SemEval 2023 task, Task 2: MultiCoNER II,Multilingual
Complex Named Entity Recognition. We approach the problem by leveraging
cross-lingual representation provided by fine-tuning XLM-Roberta base model on
datasets of all of the 12 languages provided -- Bangla, Chinese, English,
Farsi, French, German, Hindi, Italian, Portuguese, Spanish, Swedish and
Ukrainian | [
"cs.CL"
] | false |
2305.03314 | 2023-05-05T06:43:56Z | Block the Label and Noise: An N-Gram Masked Speller for Chinese Spell
Checking | [
"Haiyun Yang"
] | Recently, Chinese Spell Checking(CSC), a task to detect erroneous characters
in a sentence and correct them, has attracted extensive interest because of its
wide applications in various NLP tasks. Most of the existing methods have
utilized BERT to extract semantic information for CSC task. However, these
methods directly take sentences with only a few errors as inputs, where the
correct characters may leak answers to the model and dampen its ability to
capture distant context; while the erroneous characters may disturb the
semantic encoding process and result in poor representations. Based on such
observations, this paper proposes an n-gram masking layer that masks current
and/or surrounding tokens to avoid label leakage and error disturbance.
Moreover, considering that the mask strategy may ignore multi-modal information
indicated by errors, a novel dot-product gating mechanism is proposed to
integrate the phonological and morphological information with semantic
representation. Extensive experiments on SIGHAN datasets have demonstrated that
the pluggable n-gram masking mechanism can improve the performance of prevalent
CSC models and the proposed methods in this paper outperform multiple powerful
state-of-the-art models. | [
"cs.CL"
] | false |
2305.03407 | 2023-05-05T10:17:22Z | Online Gesture Recognition using Transformer and Natural Language
Processing | [
"G. C. M. Silvestre",
"F. Balado",
"O. Akinremi",
"M. Ramo"
] | The Transformer architecture is shown to provide a powerful machine
transduction framework for online handwritten gestures corresponding to glyph
strokes of natural language sentences. The attention mechanism is successfully
used to create latent representations of an end-to-end encoder-decoder model,
solving multi-level segmentation while also learning some language features and
syntax rules. The additional use of a large decoding space with some learned
Byte-Pair-Encoding (BPE) is shown to provide robustness to ablated inputs and
syntax rules. The encoder stack was directly fed with spatio-temporal data
tokens potentially forming an infinitely large input vocabulary, an approach
that finds applications beyond that of this work. Encoder transfer learning
capabilities is also demonstrated on several languages resulting in faster
optimisation and shared parameters. A new supervised dataset of online
handwriting gestures suitable for generic handwriting recognition tasks was
used to successfully train a small transformer model to an average normalised
Levenshtein accuracy of 96% on English or German sentences and 94% in French. | [
"cs.CL"
] | false |
2305.03429 | 2023-05-05T11:03:03Z | Simulating H.P. Lovecraft horror literature with the ChatGPT large
language model | [
"Eduardo C. Garrido-Merchán",
"José Luis Arroyo-Barrigüete",
"Roberto Gozalo-Brizuela"
] | In this paper, we present a novel approach to simulating H.P. Lovecraft's
horror literature using the ChatGPT large language model, specifically the
GPT-4 architecture. Our study aims to generate text that emulates Lovecraft's
unique writing style and themes, while also examining the effectiveness of
prompt engineering techniques in guiding the model's output. To achieve this,
we curated a prompt containing several specialized literature references and
employed advanced prompt engineering methods. We conducted an empirical
evaluation of the generated text by administering a survey to a sample of
undergraduate students. Utilizing statistical hypothesis testing, we assessed
the students ability to distinguish between genuine Lovecraft works and those
generated by our model. Our findings demonstrate that the participants were
unable to reliably differentiate between the two, indicating the effectiveness
of the GPT-4 model and our prompt engineering techniques in emulating
Lovecraft's literary style. In addition to presenting the GPT model's
capabilities, this paper provides a comprehensive description of its underlying
architecture and offers a comparative analysis with related work that simulates
other notable authors and philosophers, such as Dennett. By exploring the
potential of large language models in the context of literary emulation, our
study contributes to the body of research on the applications and limitations
of these models in various creative domains. | [
"cs.CL"
] | false |
2305.03461 | 2023-05-05T12:06:01Z | Interactive Acquisition of Fine-grained Visual Concepts by Exploiting
Semantics of Generic Characterizations in Discourse | [
"Jonghyuk Park",
"Alex Lascarides",
"Subramanian Ramamoorthy"
] | Interactive Task Learning (ITL) concerns learning about unforeseen domain
concepts via natural interactions with human users. The learner faces a number
of significant constraints: learning should be online, incremental and
few-shot, as it is expected to perform tangible belief updates right after
novel words denoting unforeseen concepts are introduced. In this work, we
explore a challenging symbol grounding task--discriminating among object
classes that look very similar--within the constraints imposed by ITL. We
demonstrate empirically that more data-efficient grounding results from
exploiting the truth-conditions of the teacher's generic statements (e.g., "Xs
have attribute Z.") and their implicatures in context (e.g., as an answer to
"How are Xs and Ys different?", one infers Y lacks attribute Z). | [
"cs.CL"
] | false |
2305.03715 | 2023-05-05T17:55:49Z | Large Language Models in Ambulatory Devices for Home Health Diagnostics:
A case study of Sickle Cell Anemia Management | [
"Oluwatosin Ogundare",
"Subuola Sofolahan"
] | This study investigates the potential of an ambulatory device that
incorporates Large Language Models (LLMs) in cadence with other specialized ML
models to assess anemia severity in sickle cell patients in real time. The
device would rely on sensor data that measures angiogenic material levels to
assess anemia severity, providing real-time information to patients and
clinicians to reduce the frequency of vaso-occlusive crises because of the
early detection of anemia severity, allowing for timely interventions and
potentially reducing the likelihood of serious complications. The main
challenges in developing such a device are the creation of a reliable
non-invasive tool for angiogenic level assessment, a biophysics model and the
practical consideration of an LLM communicating with emergency personnel on
behalf of an incapacitated patient. A possible system is proposed, and the
limitations of this approach are discussed. | [
"cs.CL"
] | false |
2305.03788 | 2023-05-05T18:39:07Z | Harnessing the Power of BERT in the Turkish Clinical Domain: Pretraining
Approaches for Limited Data Scenarios | [
"Hazal Türkmen",
"Oğuz Dikenelli",
"Cenk Eraslan",
"Mehmet Cem Çallı",
"Süha Süreyya Özbek"
] | In recent years, major advancements in natural language processing (NLP) have
been driven by the emergence of large language models (LLMs), which have
significantly revolutionized research and development within the field.
Building upon this progress, our study delves into the effects of various
pre-training methodologies on Turkish clinical language models' performance in
a multi-label classification task involving radiology reports, with a focus on
addressing the challenges posed by limited language resources. Additionally, we
evaluated the simultaneous pretraining approach by utilizing limited clinical
task data for the first time. We developed four models, including
TurkRadBERT-task v1, TurkRadBERT-task v2, TurkRadBERT-sim v1, and
TurkRadBERT-sim v2. Our findings indicate that the general Turkish BERT model
(BERTurk) and TurkRadBERT-task v1, both of which utilize knowledge from a
substantial general-domain corpus, demonstrate the best overall performance.
Although the task-adaptive pre-training approach has the potential to capture
domain-specific patterns, it is constrained by the limited task-specific corpus
and may be susceptible to overfitting. Furthermore, our results underscore the
significance of domain-specific vocabulary during pre-training for enhancing
model performance. Ultimately, we observe that the combination of
general-domain knowledge and task-specific fine-tuning is essential for
achieving optimal performance across a range of categories. This study offers
valuable insights for developing effective Turkish clinical language models and
can guide future research on pre-training techniques for other low-resource
languages within the clinical domain. | [
"cs.CL"
] | false |
2305.03796 | 2023-05-05T18:54:40Z | Transformer Working Memory Enables Regular Language Reasoning and
Natural Language Length Extrapolation | [
"Ta-Chung Chi",
"Ting-Han Fan",
"Alexander I. Rudnicky",
"Peter J. Ramadge"
] | Unlike recurrent models, conventional wisdom has it that Transformers cannot
perfectly model regular languages. Inspired by the notion of working memory, we
propose a new Transformer variant named RegularGPT. With its novel combination
of Weight-Sharing, Adaptive-Depth, and Sliding-Dilated-Attention, RegularGPT
constructs working memory along the depth dimension, thereby enabling efficient
and successful modeling of regular languages such as PARITY. We further test
RegularGPT on the task of natural language length extrapolation and
surprisingly find that it rediscovers the local windowed attention effect
deemed necessary in prior work for length extrapolation. | [
"cs.CL"
] | false |
2305.03819 | 2023-05-05T19:47:41Z | Adapting Transformer Language Models for Predictive Typing in
Brain-Computer Interfaces | [
"Shijia Liu",
"David A. Smith"
] | Brain-computer interfaces (BCI) are an important mode of alternative and
augmentative communication for many people. Unlike keyboards, many BCI systems
do not display even the 26 letters of English at one time, let alone all the
symbols in more complex systems. Using language models to make character-level
predictions, therefore, can greatly speed up BCI typing (Ghosh and Kristensson,
2017). While most existing BCI systems employ character n-gram models or no LM
at all, this paper adapts several wordpiece-level Transformer LMs to make
character predictions and evaluates them on typing tasks. GPT-2 fares best on
clean text, but different LMs react differently to noisy histories. We further
analyze the effect of character positions in a word and context lengths. | [
"cs.CL"
] | false |
2305.03851 | 2023-05-05T21:20:02Z | Large Language Models in Sport Science & Medicine: Opportunities, Risks
and Considerations | [
"Mark Connor",
"Michael O'Neill"
] | This paper explores the potential opportunities, risks, and challenges
associated with the use of large language models (LLMs) in sports science and
medicine. LLMs are large neural networks with transformer style architectures
trained on vast amounts of textual data, and typically refined with human
feedback. LLMs can perform a large range of natural language processing tasks.
In sports science and medicine, LLMs have the potential to support and augment
the knowledge of sports medicine practitioners, make recommendations for
personalised training programs, and potentially distribute high-quality
information to practitioners in developing countries. However, there are also
potential risks associated with the use and development of LLMs, including
biases in the dataset used to create the model, the risk of exposing
confidential data, the risk of generating harmful output, and the need to align
these models with human preferences through feedback. Further research is
needed to fully understand the potential applications of LLMs in sports science
and medicine and to ensure that their use is ethical and beneficial to
athletes, clients, patients, practitioners, and the general public. | [
"cs.CL",
"I.2.7"
] | false |
2305.03873 | 2023-05-05T23:22:16Z | Train Global, Tailor Local: Minimalist Multilingual Translation into
Endangered Languages | [
"Zhong Zhou",
"Jan Niehues",
"Alex Waibel"
] | In many humanitarian scenarios, translation into severely low resource
languages often does not require a universal translation engine, but a
dedicated text-specific translation engine. For example, healthcare records,
hygienic procedures, government communication, emergency procedures and
religious texts are all limited texts. While generic translation engines for
all languages do not exist, translation of multilingually known limited texts
into new, endangered languages may be possible and reduce human translation
effort. We attempt to leverage translation resources from many rich resource
languages to efficiently produce best possible translation quality for a well
known text, which is available in multiple languages, in a new, severely low
resource language. We examine two approaches: 1. best selection of seed
sentences to jump start translations in a new language in view of best
generalization to the remainder of a larger targeted text(s), and 2. we adapt
large general multilingual translation engines from many other languages to
focus on a specific text in a new, unknown language. We find that adapting
large pretrained multilingual models to the domain/text first and then to the
severely low resource language works best. If we also select a best set of seed
sentences, we can improve average chrF performance on new test languages from a
baseline of 21.9 to 50.7, while reducing the number of seed sentences to only
around 1,000 in the new, unknown language. | [
"cs.CL"
] | false |
2305.03262 | 2023-05-05T03:28:49Z | Rescue Conversations from Dead-ends: Efficient Exploration for
Task-oriented Dialogue Policy Optimization | [
"Yangyang Zhao",
"Zhenyu Wang",
"Mehdi Dastani",
"Shihan Wang"
] | Training a dialogue policy using deep reinforcement learning requires a lot
of exploration of the environment. The amount of wasted invalid exploration
makes their learning inefficient. In this paper, we find and define an
important reason for the invalid exploration: dead-ends. When a conversation
enters a dead-end state, regardless of the actions taken afterward, it will
continue in a dead-end trajectory until the agent reaches a termination state
or maximum turn. We propose a dead-end resurrection (DDR) algorithm that
detects the initial dead-end state in a timely and efficient manner and
provides a rescue action to guide and correct the exploration direction. To
prevent dialogue policies from repeatedly making the same mistake, DDR also
performs dialogue data augmentation by adding relevant experiences containing
dead-end states. We first validate the dead-end detection reliability and then
demonstrate the effectiveness and generality of the method by reporting
experimental results on several dialogue datasets from different domains. | [
"cs.HC",
"cs.CL"
] | false |
2305.03287 | 2023-05-05T05:32:50Z | Low-Resource Multi-Granularity Academic Function Recognition Based on
Multiple Prompt Knowledge | [
"Jiawei Liu",
"Zi Xiong",
"Yi Jiang",
"Yongqiang Ma",
"Wei Lu",
"Yong Huang",
"Qikai Cheng"
] | Fine-tuning pre-trained language models (PLMs), e.g., SciBERT, generally
requires large numbers of annotated data to achieve state-of-the-art
performance on a range of NLP tasks in the scientific domain. However,
obtaining the fine-tune data for scientific NLP task is still challenging and
expensive. Inspired by recent advancement in prompt learning, in this paper, we
propose the Mix Prompt Tuning (MPT), which is a semi-supervised method to
alleviate the dependence on annotated data and improve the performance of
multi-granularity academic function recognition tasks with a small number of
labeled examples. Specifically, the proposed method provides multi-perspective
representations by combining manual prompt templates with automatically learned
continuous prompt templates to help the given academic function recognition
task take full advantage of knowledge in PLMs. Based on these prompt templates
and the fine-tuned PLM, a large number of pseudo labels are assigned to the
unlabeled examples. Finally, we fine-tune the PLM using the pseudo training
set. We evaluate our method on three academic function recognition tasks of
different granularity including the citation function, the abstract sentence
function, and the keyword function, with datasets from computer science domain
and biomedical domain. Extensive experiments demonstrate the effectiveness of
our method and statistically significant improvements against strong baselines.
In particular, it achieves an average increase of 5% in Macro-F1 score compared
with fine-tuning, and 6% in Macro-F1 score compared with other semi-supervised
method under low-resource settings. In addition, MPT is a general method that
can be easily applied to other low-resource scientific classification tasks. | [
"cs.CL",
"cs.AI"
] | false |
2305.03299 | 2023-05-05T06:03:54Z | Open Information Extraction via Chunks | [
"Kuicai Dong",
"Aixin Sun",
"Jung-Jae Kim",
"Xiaoli Li"
] | Open Information Extraction (OIE) aims to extract relational tuples from
open-domain sentences. Existing OIE systems split a sentence into tokens and
recognize token spans as tuple relations and arguments. We instead propose
Sentence as Chunk sequence (SaC) and recognize chunk spans as tuple relations
and arguments. We argue that SaC has better quantitative and qualitative
properties for OIE than sentence as token sequence, and evaluate four choices
of chunks (i.e., CoNLL chunks, simple phrases, NP chunks, and spans from
SpanOIE) against gold OIE tuples. Accordingly, we propose a simple BERT-based
model for sentence chunking, and propose Chunk-OIE for tuple extraction on top
of SaC. Chunk-OIE achieves state-of-the-art results on multiple OIE datasets,
showing that SaC benefits OIE task. | [
"cs.CL",
"cs.AI"
] | false |
2305.03458 | 2023-05-05T12:00:58Z | Multi-View Graph Representation Learning for Answering Hybrid Numerical
Reasoning Question | [
"Yifan Wei",
"Fangyu Lei",
"Yuanzhe Zhang",
"Jun Zhao",
"Kang Liu"
] | Hybrid question answering (HybridQA) over the financial report contains both
textual and tabular data, and requires the model to select the appropriate
evidence for the numerical reasoning task. Existing methods based on
encoder-decoder framework employ a expression tree-based decoder to solve
numerical reasoning problems. However, encoders rely more on Machine Reading
Comprehension (MRC) methods, which take table serialization and text splicing
as input, damaging the granularity relationship between table and text as well
as the spatial structure information of table itself. In order to solve these
problems, the paper proposes a Multi-View Graph (MVG) Encoder to take the
relations among the granularity into account and capture the relations from
multiple view. By utilizing MVGE as a module, we constuct Tabular View,
Relation View and Numerical View which aim to retain the original
characteristics of the hybrid data. We validate our model on the publicly
available table-text hybrid QA benchmark (TAT-QA) and outperform the
state-of-the-art model. | [
"cs.CL",
"cs.AI"
] | false |
2305.03573 | 2023-05-05T14:30:20Z | In-context Learning as Maintaining Coherency: A Study of On-the-fly
Machine Translation Using Large Language Models | [
"Suzanna Sia",
"Kevin Duh"
] | The phenomena of in-context learning has typically been thought of as
"learning from examples". In this work which focuses on Machine Translation, we
present a perspective of in-context learning as the desired generation task
maintaining coherency with its context, i.e., the prompt examples. We first
investigate randomly sampled prompts across 4 domains, and find that
translation performance improves when shown in-domain prompts. Next, we
investigate coherency for the in-domain setting, which uses prompt examples
from a moving window. We study this with respect to other factors that have
previously been identified in the literature such as length, surface similarity
and sentence embedding similarity. Our results across 3 models (GPTNeo2.7B,
Bloom3B, XGLM2.9B), and three translation directions
(\texttt{en}$\rightarrow$\{\texttt{pt, de, fr}\}) suggest that the long-term
coherency of the prompts and the test sentence is a good indicator of
downstream translation performance. In doing so, we demonstrate the efficacy of
In-context Machine Translation for on-the-fly adaptation. | [
"cs.CL",
"cs.AI"
] | false |
2305.03661 | 2023-05-05T16:28:44Z | Predicting COVID-19 and pneumonia complications from admission texts | [
"Dmitriy Umerenkov",
"Oleg Cherkashin",
"Alexander Nesterov",
"Victor Gombolevskiy",
"Irina Demko",
"Alexander Yalunin",
"Vladimir Kokh"
] | In this paper we present a novel approach to risk assessment for patients
hospitalized with pneumonia or COVID-19 based on their admission reports. We
applied a Longformer neural network to admission reports and other textual data
available shortly after admission to compute risk scores for the patients. We
used patient data of multiple European hospitals to demonstrate that our
approach outperforms the Transformer baselines. Our experiments show that the
proposed model generalises across institutions and diagnoses. Also, our method
has several other advantages described in the paper. | [
"cs.CL",
"cs.AI"
] | false |
2305.03793 | 2023-05-05T18:47:18Z | Towards Zero-Shot Frame Semantic Parsing with Task Agnostic Ontologies
and Simple Labels | [
"Danilo Ribeiro",
"Omid Abdar",
"Jack Goetz",
"Mike Ross",
"Annie Dong",
"Kenneth Forbus",
"Ahmed Mohamed"
] | Frame semantic parsing is an important component of task-oriented dialogue
systems. Current models rely on a significant amount training data to
successfully identify the intent and slots in the user's input utterance. This
creates a significant barrier for adding new domains to virtual assistant
capabilities, as creation of this data requires highly specialized NLP
expertise. In this work we propose OpenFSP, a framework that allows for easy
creation of new domains from a handful of simple labels that can be generated
without specific NLP knowledge. Our approach relies on creating a small, but
expressive, set of domain agnostic slot types that enables easy annotation of
new domains. Given such annotation, a matching algorithm relying on sentence
encoders predicts the intent and slots for domains defined by end-users.
Extensive experiments on the TopV2 dataset shows that our model outperforms
strong baselines in this simple labels setting. | [
"cs.CL",
"cs.LG",
"I.2.7; I.2.6"
] | false |
2305.03845 | 2023-05-05T20:49:40Z | CLaC at SemEval-2023 Task 2: Comparing Span-Prediction and
Sequence-Labeling approaches for NER | [
"Harsh Verma",
"Sabine Bergler"
] | This paper summarizes the CLaC submission for the MultiCoNER 2 task which
concerns the recognition of complex, fine-grained named entities. We compare
two popular approaches for NER, namely Sequence Labeling and Span Prediction.
We find that our best Span Prediction system performs slightly better than our
best Sequence Labeling system on test data. Moreover, we find that using the
larger version of XLM RoBERTa significantly improves performance.
Post-competition experiments show that Span Prediction and Sequence Labeling
approaches improve when they use special input tokens (<s> and </s>) of
XLM-RoBERTa. The code for training all models, preprocessing, and
post-processing is available at
https://github.com/harshshredding/semeval2023-multiconer-paper. | [
"cs.CL",
"cs.AI"
] | false |
2305.06157 | 2023-05-05T08:59:03Z | Implications of Multi-Word Expressions on English to Bharti Braille
Machine Translation | [
"Nisheeth Joshi",
"Pragya Katyayan"
] | In this paper, we have shown the improvement of English to Bharti Braille
machine translation system. We have shown how we can improve a baseline NMT
model by adding some linguistic knowledge to it. This was done for five
language pairs where English sentences were translated into five Indian
languages and then subsequently to corresponding Bharti Braille. This has been
demonstrated by adding a sub-module for translating multi-word expressions. The
approach shows promising results as across language pairs, we could see
improvement in the quality of NMT outputs. The least improvement was observed
in English-Nepali language pair with 22.08% and the most improvement was
observed in the English-Hindi language pair with 23.30%. | [
"cs.CL",
"cs.AI"
] | false |
2305.06475 | 2023-05-05T09:21:13Z | A Model for Translation of Text from Indian Languages to Bharti Braille
Characters | [
"Nisheeth Joshi",
"Pragya Katyayan"
] | People who are visually impaired face a lot of difficulties while studying.
One of the major causes to this is lack of available text in Bharti Braille
script. In this paper, we have suggested a scheme to convert text in major
Indian languages into Bharti Braille. The system uses a hybrid approach where
at first the text in Indian language is given to a rule based system and in
case if there is any ambiguity then it is resolved by applying a LSTM based
model. The developed model has also been tested and found to have produced near
accurate results. | [
"cs.CL",
"cs.AI"
] | false |
2305.03336 | 2023-05-05T07:40:41Z | QCRI at SemEval-2023 Task 3: News Genre, Framing and Persuasion
Techniques Detection using Multilingual Models | [
"Maram Hasanain",
"Ahmed Oumar El-Shangiti",
"Rabindra Nath Nandi",
"Preslav Nakov",
"Firoj Alam"
] | Misinformation spreading in mainstream and social media has been misleading
users in different ways. Manual detection and verification efforts by
journalists and fact-checkers can no longer cope with the great scale and quick
spread of misleading information. This motivated research and industry efforts
to develop systems for analyzing and verifying news spreading online. The
SemEval-2023 Task 3 is an attempt to address several subtasks under this
overarching problem, targeting writing techniques used in news articles to
affect readers' opinions. The task addressed three subtasks with six languages,
in addition to three ``surprise'' test languages, resulting in 27 different
test setups. This paper describes our participating system to this task. Our
team is one of the 6 teams that successfully submitted runs for all setups. The
official results show that our system is ranked among the top 3 systems for 10
out of the 27 setups. | [
"cs.CL",
"cs.AI",
"cs.CY",
"68T50",
"F.2.2; I.2.7"
] | false |
2305.03356 | 2023-05-05T08:20:09Z | From Parse-Execute to Parse-Execute-Refine: Improving Semantic Parser
for Complex Question Answering over Knowledge Base | [
"Wangzhen Guo",
"Linyin Luo",
"Hanjiang Lai",
"Jian Yin"
] | Parsing questions into executable logical forms has showed impressive results
for knowledge-base question answering (KBQA). However, complex KBQA is a more
challenging task that requires to perform complex multi-step reasoning.
Recently, a new semantic parser called KoPL has been proposed to explicitly
model the reasoning processes, which achieved the state-of-the-art on complex
KBQA. In this paper, we further explore how to unlock the reasoning ability of
semantic parsers by a simple proposed parse-execute-refine paradigm. We refine
and improve the KoPL parser by demonstrating the executed intermediate
reasoning steps to the KBQA model. We show that such simple strategy can
significantly improve the ability of complex reasoning. Specifically, we
propose three components: a parsing stage, an execution stage and a refinement
stage, to enhance the ability of complex reasoning. The parser uses the KoPL to
generate the transparent logical forms. Then, the execution stage aligns and
executes the logical forms over knowledge base to obtain intermediate reasoning
processes. Finally, the intermediate step-by-step reasoning processes are
demonstrated to the KBQA model in the refinement stage. With the explicit
reasoning processes, it is much easier to answer the complex questions.
Experiments on benchmark dataset shows that the proposed PER-KBQA performs
significantly better than the stage-of-the-art baselines on the complex KBQA. | [
"cs.CL",
"cs.AI",
"cs.LG"
] | false |
2305.03369 | 2023-05-05T08:53:57Z | The MuSe 2023 Multimodal Sentiment Analysis Challenge: Mimicked
Emotions, Cross-Cultural Humour, and Personalisation | [
"Lukas Christ",
"Shahin Amiriparian",
"Alice Baird",
"Alexander Kathan",
"Niklas Müller",
"Steffen Klug",
"Chris Gagne",
"Panagiotis Tzirakis",
"Eva-Maria Meßner",
"Andreas König",
"Alan Cowen",
"Erik Cambria",
"Björn W. Schuller"
] | The MuSe 2023 is a set of shared tasks addressing three different
contemporary multimodal affect and sentiment analysis problems: In the Mimicked
Emotions Sub-Challenge (MuSe-Mimic), participants predict three continuous
emotion targets. This sub-challenge utilises the Hume-Vidmimic dataset
comprising of user-generated videos. For the Cross-Cultural Humour Detection
Sub-Challenge (MuSe-Humour), an extension of the Passau Spontaneous Football
Coach Humour (Passau-SFCH) dataset is provided. Participants predict the
presence of spontaneous humour in a cross-cultural setting. The Personalisation
Sub-Challenge (MuSe-Personalisation) is based on the Ulm-Trier Social Stress
Test (Ulm-TSST) dataset, featuring recordings of subjects in a stressed
situation. Here, arousal and valence signals are to be predicted, whereas parts
of the test labels are made available in order to facilitate personalisation.
MuSe 2023 seeks to bring together a broad audience from different research
communities such as audio-visual emotion recognition, natural language
processing, signal processing, and health informatics. In this baseline paper,
we introduce the datasets, sub-challenges, and provided feature sets. As a
competitive baseline system, a Gated Recurrent Unit (GRU)-Recurrent Neural
Network (RNN) is employed. On the respective sub-challenges' test datasets, it
achieves a mean (across three continuous intensity targets) Pearson's
Correlation Coefficient of .4727 for MuSe-Mimic, an Area Under the Curve (AUC)
value of .8310 for MuSe-Humor and Concordance Correlation Coefficient (CCC)
values of .7482 for arousal and .7827 for valence in the MuSe-Personalisation
sub-challenge. | [
"cs.LG",
"cs.AI",
"cs.CL",
"cs.MM"
] | false |
2305.03660 | 2023-05-05T16:28:03Z | Retrieval Augmented Chest X-Ray Report Generation using OpenAI GPT
models | [
"Mercy Ranjit",
"Gopinath Ganapathy",
"Ranjit Manuel",
"Tanuja Ganu"
] | We propose Retrieval Augmented Generation (RAG) as an approach for automated
radiology report writing that leverages multimodally aligned embeddings from a
contrastively pretrained vision language model for retrieval of relevant
candidate radiology text for an input radiology image and a general domain
generative model like OpenAI text-davinci-003, gpt-3.5-turbo and gpt-4 for
report generation using the relevant radiology text retrieved. This approach
keeps hallucinated generations under check and provides capabilities to
generate report content in the format we desire leveraging the instruction
following capabilities of these generative models. Our approach achieves better
clinical metrics with a BERTScore of 0.2865 ({\Delta}+ 25.88%) and Semb score
of 0.4026 ({\Delta}+ 6.31%). Our approach can be broadly relevant for different
clinical settings as it allows to augment the automated radiology report
generation process with content relevant for that setting while also having the
ability to inject user intents and requirements in the prompts as part of the
report generation process to modulate the content and format of the generated
reports as applicable for that clinical setting. | [
"cs.CL",
"cs.AI",
"cs.IR",
"cs.LG",
"I.2; J.3; H.3"
] | false |
2305.03742 | 2023-05-05T07:24:46Z | Improved Logical Reasoning of Language Models via Differentiable
Symbolic Programming | [
"Hanlin Zhang",
"Jiani Huang",
"Ziyang Li",
"Mayur Naik",
"Eric Xing"
] | Pre-trained large language models (LMs) struggle to perform logical reasoning
reliably despite advances in scale and compositionality. In this work, we
tackle this challenge through the lens of symbolic programming. We propose
DSR-LM, a Differentiable Symbolic Reasoning framework where pre-trained LMs
govern the perception of factual knowledge, and a symbolic module performs
deductive reasoning. In contrast to works that rely on hand-crafted logic
rules, our differentiable symbolic reasoning framework efficiently learns
weighted rules and applies semantic loss to further improve LMs. DSR-LM is
scalable, interpretable, and allows easy integration of prior knowledge,
thereby supporting extensive symbolic programming to robustly derive a logical
conclusion. The results of our experiments suggest that DSR-LM improves the
logical reasoning abilities of pre-trained language models, resulting in a
significant increase in accuracy of over 20% on deductive reasoning benchmarks.
Furthermore, DSR-LM outperforms a variety of competitive baselines when faced
with systematic changes in sequence length. | [
"cs.AI",
"cs.CL",
"cs.LG"
] | false |
2305.04927 | 2023-05-05T08:25:07Z | Detecting and Reasoning of Deleted Tweets before they are Posted | [
"Hamdy Mubarak",
"Samir Abdaljalil",
"Azza Nassar",
"Firoj Alam"
] | Social media platforms empower us in several ways, from information
dissemination to consumption. While these platforms are useful in promoting
citizen journalism, public awareness etc., they have misuse potentials.
Malicious users use them to disseminate hate-speech, offensive content, rumor
etc. to gain social and political agendas or to harm individuals, entities and
organizations. Often times, general users unconsciously share information
without verifying it, or unintentionally post harmful messages. Some of such
content often get deleted either by the platform due to the violation of terms
and policies, or users themselves for different reasons, e.g., regrets. There
is a wide range of studies in characterizing, understanding and predicting
deleted content. However, studies which aims to identify the fine-grained
reasons (e.g., posts are offensive, hate speech or no identifiable reason)
behind deleted content, are limited. In this study we address this gap, by
identifying deleted tweets, particularly within the Arabic context, and
labeling them with a corresponding fine-grained disinformation category. We
then develop models that can predict the potentiality of tweets getting
deleted, as well as the potential reasons behind deletion. Such models can help
in moderating social media posts before even posting. | [
"cs.CL",
"cs.AI",
"cs.CY",
"68T50",
"F.2.2; I.2.7"
] | false |
2305.03648 | 2023-05-05T16:10:31Z | On the Effectiveness of Equivariant Regularization for Robust Online
Continual Learning | [
"Lorenzo Bonicelli",
"Matteo Boschini",
"Emanuele Frascaroli",
"Angelo Porrello",
"Matteo Pennisi",
"Giovanni Bellitto",
"Simone Palazzo",
"Concetto Spampinato",
"Simone Calderara"
] | Humans can learn incrementally, whereas neural networks forget previously
acquired information catastrophically. Continual Learning (CL) approaches seek
to bridge this gap by facilitating the transfer of knowledge to both previous
tasks (backward transfer) and future ones (forward transfer) during training.
Recent research has shown that self-supervision can produce versatile models
that can generalize well to diverse downstream tasks. However, contrastive
self-supervised learning (CSSL), a popular self-supervision technique, has
limited effectiveness in online CL (OCL). OCL only permits one iteration of the
input dataset, and CSSL's low sample efficiency hinders its use on the input
data-stream.
In this work, we propose Continual Learning via Equivariant Regularization
(CLER), an OCL approach that leverages equivariant tasks for self-supervision,
avoiding CSSL's limitations. Our method represents the first attempt at
combining equivariant knowledge with CL and can be easily integrated with
existing OCL methods. Extensive ablations shed light on how equivariant pretext
tasks affect the network's information flow and its impact on CL dynamics. | [
"cs.LG"
] | false |
2305.03740 | 2023-05-05T02:21:08Z | Judge Me in Context: A Telematics-Based Driving Risk Prediction
Framework in Presence of Weak Risk Labels | [
"Sobhan Moosavi",
"Rajiv Ramnath"
] | Driving risk prediction has been a topic of much research over the past few
decades to minimize driving risk and increase safety. The use of demographic
information in risk prediction is a traditional solution with applications in
insurance planning, however, it is difficult to capture true driving behavior
via such coarse-grained factors. Therefor, the use of telematics data has
gained a widespread popularity over the past decade. While most of the existing
studies leverage demographic information in addition to telematics data, our
objective is to maximize the use of telematics as well as contextual
information (e.g., road-type) to build a risk prediction framework with
real-world applications. We contextualize telematics data in a variety of
forms, and then use it to develop a risk classifier, assuming that there are
some weak risk labels available (e.g., past traffic citation records). Before
building a risk classifier though, we employ a novel data-driven process to
augment weak risk labels. Extensive analysis and results based on real-world
data from multiple major cities in the United States demonstrate usefulness of
the proposed framework. | [
"cs.LG"
] | false |
2305.03784 | 2023-05-05T18:34:49Z | Neural Exploitation and Exploration of Contextual Bandits | [
"Yikun Ban",
"Yuchen Yan",
"Arindam Banerjee",
"Jingrui He"
] | In this paper, we study utilizing neural networks for the exploitation and
exploration of contextual multi-armed bandits. Contextual multi-armed bandits
have been studied for decades with various applications. To solve the
exploitation-exploration trade-off in bandits, there are three main techniques:
epsilon-greedy, Thompson Sampling (TS), and Upper Confidence Bound (UCB). In
recent literature, a series of neural bandit algorithms have been proposed to
adapt to the non-linear reward function, combined with TS or UCB strategies for
exploration. In this paper, instead of calculating a large-deviation based
statistical bound for exploration like previous methods, we propose,
``EE-Net,'' a novel neural-based exploitation and exploration strategy. In
addition to using a neural network (Exploitation network) to learn the reward
function, EE-Net uses another neural network (Exploration network) to
adaptively learn the potential gains compared to the currently estimated reward
for exploration. We provide an instance-based
$\widetilde{\mathcal{O}}(\sqrt{T})$ regret upper bound for EE-Net and show that
EE-Net outperforms related linear and neural contextual bandit baselines on
real-world datasets. | [
"cs.LG"
] | false |
2305.03863 | 2023-05-05T22:25:42Z | Software-based Automatic Differentiation is Flawed | [
"Daniel Johnson",
"Trevor Maxfield",
"Yongxu Jin",
"Ronald Fedkiw"
] | Various software efforts embrace the idea that object oriented programming
enables a convenient implementation of the chain rule, facilitating so-called
automatic differentiation via backpropagation. Such frameworks have no
mechanism for simplifying the expressions (obtained via the chain rule) before
evaluating them. As we illustrate below, the resulting errors tend to be
unbounded. | [
"cs.LG"
] | false |
2305.03178 | 2023-05-05T12:16:01Z | Contrastive Learning for Sleep Staging based on Inter Subject
Correlation | [
"Tongxu Zhang",
"Bei Wang"
] | In recent years, multitudes of researches have applied deep learning to
automatic sleep stage classification. Whereas actually, these works have paid
less attention to the issue of cross-subject in sleep staging. At the same
time, emerging neuroscience theories on inter-subject correlations can provide
new insights for cross-subject analysis. This paper presents the MViTime model
that have been used in sleep staging study. And we implement the inter-subject
correlation theory through contrastive learning, providing a feasible solution
to address the cross-subject problem in sleep stage classification. Finally,
experimental results and conclusions are presented, demonstrating that the
developed method has achieved state-of-the-art performance on sleep staging.
The results of the ablation experiment also demonstrate the effectiveness of
the cross-subject approach based on contrastive learning. | [
"eess.SP",
"cs.LG"
] | false |
2305.03224 | 2023-05-05T01:02:08Z | Carbon Price Forecasting with Quantile Regression and Feature Selection | [
"Tianqi Pang",
"Kehui Tan",
"Chenyou Fan"
] | Carbon futures has recently emerged as a novel financial asset in the trading
markets such as the European Union and China. Monitoring the trend of the
carbon price has become critical for both national policy-making as well as
industrial manufacturing planning. However, various geopolitical, social, and
economic factors can impose substantial influence on the carbon price. Due to
its volatility and non-linearity, predicting accurate carbon prices is
generally a difficult task. In this study, we propose to improve carbon price
forecasting with several novel practices. First, we collect various influencing
factors, including commodity prices, export volumes such as oil and natural
gas, and prosperity indices. Then we select the most significant factors and
disclose their optimal grouping for explainability. Finally, we use the Sparse
Quantile Group Lasso and Adaptive Sparse Quantile Group Lasso for robust price
predictions. We demonstrate through extensive experimental studies that our
proposed methods outperform existing ones. Also, our quantile predictions
provide a complete profile of future prices at different levels, which better
describes the distributions of the carbon market. | [
"cs.LG",
"q-fin.ST"
] | false |
2305.03249 | 2023-05-05T02:27:27Z | PMP: Learning to Physically Interact with Environments using Part-wise
Motion Priors | [
"Jinseok Bae",
"Jungdam Won",
"Donggeun Lim",
"Cheol-Hui Min",
"Young Min Kim"
] | We present a method to animate a character incorporating multiple part-wise
motion priors (PMP). While previous works allow creating realistic articulated
motions from reference data, the range of motion is largely limited by the
available samples. Especially for the interaction-rich scenarios, it is
impractical to attempt acquiring every possible interacting motion, as the
combination of physical parameters increases exponentially. The proposed PMP
allows us to assemble multiple part skills to animate a character, creating a
diverse set of motions with different combinations of existing data. In our
pipeline, we can train an agent with a wide range of part-wise priors.
Therefore, each body part can obtain a kinematic insight of the style from the
motion captures, or at the same time extract dynamics-related information from
the additional part-specific simulation. For example, we can first train a
general interaction skill, e.g. grasping, only for the dexterous part, and then
combine the expert trajectories from the pre-trained agent with the kinematic
priors of other limbs. Eventually, our whole-body agent learns a novel physical
interaction skill even with the absence of the object trajectories in the
reference motion sequence. | [
"cs.GR",
"cs.LG"
] | false |
2305.03263 | 2023-05-05T03:29:34Z | Bayesian Reinforcement Learning with Limited Cognitive Load | [
"Dilip Arumugam",
"Mark K. Ho",
"Noah D. Goodman",
"Benjamin Van Roy"
] | All biological and artificial agents must learn and make decisions given
limits on their ability to process information. As such, a general theory of
adaptive behavior should be able to account for the complex interactions
between an agent's learning history, decisions, and capacity constraints.
Recent work in computer science has begun to clarify the principles that shape
these dynamics by bridging ideas from reinforcement learning, Bayesian
decision-making, and rate-distortion theory. This body of work provides an
account of capacity-limited Bayesian reinforcement learning, a unifying
normative framework for modeling the effect of processing constraints on
learning and action selection. Here, we provide an accessible review of recent
algorithms and theoretical results in this setting, paying special attention to
how these ideas can be applied to studying questions in the cognitive and
behavioral sciences. | [
"cs.LG",
"cs.AI"
] | false |
2305.03414 | 2023-05-05T10:27:23Z | Adaptive Graph Convolutional Subspace Clustering | [
"Lai Wei",
"Zhengwei Chen",
"Jun Yin",
"Changming Zhu",
"Rigui Zhou",
"Jin Liu"
] | Spectral-type subspace clustering algorithms have shown excellent performance
in many subspace clustering applications. The existing spectral-type subspace
clustering algorithms either focus on designing constraints for the
reconstruction coefficient matrix or feature extraction methods for finding
latent features of original data samples. In this paper, inspired by graph
convolutional networks, we use the graph convolution technique to develop a
feature extraction method and a coefficient matrix constraint simultaneously.
And the graph-convolutional operator is updated iteratively and adaptively in
our proposed algorithm. Hence, we call the proposed method adaptive graph
convolutional subspace clustering (AGCSC). We claim that by using AGCSC, the
aggregated feature representation of original data samples is suitable for
subspace clustering, and the coefficient matrix could reveal the subspace
structure of the original data set more faithfully. Finally, plenty of subspace
clustering experiments prove our conclusions and show that AGCSC outperforms
some related methods as well as some deep models. | [
"cs.LG",
"cs.AI"
] | false |
2305.03452 | 2023-05-05T11:56:26Z | A technical note on bilinear layers for interpretability | [
"Lee Sharkey"
] | The ability of neural networks to represent more features than neurons makes
interpreting them challenging. This phenomenon, known as superposition, has
spurred efforts to find architectures that are more interpretable than standard
multilayer perceptrons (MLPs) with elementwise activation functions. In this
note, I examine bilinear layers, which are a type of MLP layer that are
mathematically much easier to analyze while simultaneously performing better
than standard MLPs. Although they are nonlinear functions of their input, I
demonstrate that bilinear layers can be expressed using only linear operations
and third order tensors. We can integrate this expression for bilinear layers
into a mathematical framework for transformer circuits, which was previously
limited to attention-only transformers. These results suggest that bilinear
layers are easier to analyze mathematically than current architectures and thus
may lend themselves to deeper safety insights by allowing us to talk more
formally about circuits in neural networks. Additionally, bilinear layers may
offer an alternative path for mechanistic interpretability through
understanding the mechanisms of feature construction instead of enumerating a
(potentially exponentially) large number of features in large models. | [
"cs.LG",
"cs.NE"
] | false |
2305.03555 | 2023-05-05T14:04:52Z | Contrastive Graph Clustering in Curvature Spaces | [
"Li Sun",
"Feiyang Wang",
"Junda Ye",
"Hao Peng",
"Philip S. Yu"
] | Graph clustering is a longstanding research topic, and has achieved
remarkable success with the deep learning methods in recent years.
Nevertheless, we observe that several important issues largely remain open. On
the one hand, graph clustering from the geometric perspective is appealing but
has rarely been touched before, as it lacks a promising space for geometric
clustering. On the other hand, contrastive learning boosts the deep graph
clustering but usually struggles in either graph augmentation or hard sample
mining. To bridge this gap, we rethink the problem of graph clustering from
geometric perspective and, to the best of our knowledge, make the first attempt
to introduce a heterogeneous curvature space to graph clustering problem.
Correspondingly, we present a novel end-to-end contrastive graph clustering
model named CONGREGATE, addressing geometric graph clustering with Ricci
curvatures. To support geometric clustering, we construct a theoretically
grounded Heterogeneous Curvature Space where deep representations are generated
via the product of the proposed fully Riemannian graph convolutional nets.
Thereafter, we train the graph clusters by an augmentation-free reweighted
contrastive approach where we pay more attention to both hard negatives and
hard positives in our curvature space. Empirical results on real-world graphs
show that our model outperforms the state-of-the-art competitors. | [
"cs.LG",
"stat.ML"
] | false |
2305.03574 | 2023-05-05T14:30:29Z | Scope Restriction for Scalable Real-Time Railway Rescheduling: An
Exploratory Study | [
"Erik Nygren",
"Christian Eichenberger",
"Emma Frejinger"
] | With the aim to stimulate future research, we describe an exploratory study
of a railway rescheduling problem. A widely used approach in practice and state
of the art is to decompose these complex problems by geographical scope.
Instead, we propose defining a core problem that restricts a rescheduling
problem in response to a disturbance to only trains that need to be
rescheduled, hence restricting the scope in both time and space. In this
context, the difficulty resides in defining a scoper that can predict a subset
of train services that will be affected by a given disturbance. We report
preliminary results using the Flatland simulation environment that highlights
the potential and challenges of this idea. We provide an extensible playground
open-source implementation based on the Flatland railway environment and
Answer-Set Programming. | [
"math.OC",
"cs.LG"
] | false |
2305.03623 | 2023-05-05T15:33:39Z | Optimizing Hyperparameters with Conformal Quantile Regression | [
"David Salinas",
"Jacek Golebiowski",
"Aaron Klein",
"Matthias Seeger",
"Cedric Archambeau"
] | Many state-of-the-art hyperparameter optimization (HPO) algorithms rely on
model-based optimizers that learn surrogate models of the target function to
guide the search. Gaussian processes are the de facto surrogate model due to
their ability to capture uncertainty but they make strong assumptions about the
observation noise, which might not be warranted in practice. In this work, we
propose to leverage conformalized quantile regression which makes minimal
assumptions about the observation noise and, as a result, models the target
function in a more realistic and robust fashion which translates to quicker HPO
convergence on empirical benchmarks. To apply our method in a multi-fidelity
setting, we propose a simple, yet effective, technique that aggregates observed
results across different resource levels and outperforms conventional methods
across many empirical tasks. | [
"cs.LG",
"stat.ML"
] | false |
2305.03710 | 2023-05-05T17:50:50Z | Data Encoding For Healthcare Data Democratisation and Information
Leakage Prevention | [
"Anshul Thakur",
"Tingting Zhu",
"Vinayak Abrol",
"Jacob Armstrong",
"Yujiang Wang",
"David A. Clifton"
] | The lack of data democratization and information leakage from trained models
hinder the development and acceptance of robust deep learning-based healthcare
solutions. This paper argues that irreversible data encoding can provide an
effective solution to achieve data democratization without violating the
privacy constraints imposed on healthcare data and clinical models. An ideal
encoding framework transforms the data into a new space where it is
imperceptible to a manual or computational inspection. However, encoded data
should preserve the semantics of the original data such that deep learning
models can be trained effectively. This paper hypothesizes the characteristics
of the desired encoding framework and then exploits random projections and
random quantum encoding to realize this framework for dense and longitudinal or
time-series data. Experimental evaluation highlights that models trained on
encoded time-series data effectively uphold the information bottleneck
principle and hence, exhibit lesser information leakage from trained models. | [
"cs.LG",
"cs.CR"
] | false |
2305.03741 | 2023-05-05T07:03:24Z | AmGCL: Feature Imputation of Attribute Missing Graph via Self-supervised
Contrastive Learning | [
"Xiaochuan Zhang",
"Mengran Li",
"Ye Wang",
"Haojun Fei"
] | Attribute graphs are ubiquitous in multimedia applications, and graph
representation learning (GRL) has been successful in analyzing attribute graph
data. However, incomplete graph data and missing node attributes can have a
negative impact on media knowledge discovery. Existing methods for handling
attribute missing graph have limited assumptions or fail to capture complex
attribute-graph dependencies. To address these challenges, we propose Attribute
missing Graph Contrastive Learning (AmGCL), a framework for handling missing
node attributes in attribute graph data. AmGCL leverages Dirichlet energy
minimization-based feature precoding to encode in missing attributes and a
self-supervised Graph Augmentation Contrastive Learning Structure (GACLS) to
learn latent variables from the encoded-in data. Specifically, AmGCL utilizies
feature reconstruction based on structure-attribute energy minimization while
maximizes the lower bound of evidence for latent representation mutual
information. Our experimental results on multiple real-world datasets
demonstrate that AmGCL outperforms state-of-the-art methods in both feature
imputation and node classification tasks, indicating the effectiveness of our
proposed method in real-world attribute graph analysis tasks. | [
"cs.LG",
"cs.AI"
] | false |
2305.03743 | 2023-05-05T10:04:03Z | Learning Sentinel-2 reflectance dynamics for data-driven assimilation
and forecasting | [
"Anthony Frion",
"Lucas Drumetz",
"Guillaume Tochon",
"Mauro Dalla Mura",
"Abdeldjalil Aïssa El Bey"
] | Over the last few years, massive amounts of satellite multispectral and
hyperspectral images covering the Earth's surface have been made publicly
available for scientific purpose, for example through the European Copernicus
project. Simultaneously, the development of self-supervised learning (SSL)
methods has sparked great interest in the remote sensing community, enabling to
learn latent representations from unlabeled data to help treating downstream
tasks for which there is few annotated examples, such as interpolation,
forecasting or unmixing. Following this line, we train a deep learning model
inspired from the Koopman operator theory to model long-term reflectance
dynamics in an unsupervised way. We show that this trained model, being
differentiable, can be used as a prior for data assimilation in a
straightforward way. Our datasets, which are composed of Sentinel-2
multispectral image time series, are publicly released with several levels of
treatment. | [
"eess.IV",
"cs.LG"
] | false |
2305.03814 | 2023-05-05T19:42:11Z | Deep Labeling of fMRI Brain Networks | [
"Ammar Ahmed Pallikonda Latheef",
"Sejal Ghate",
"Zhipeng Hui",
"Alberto Santamaria-Pang",
"Ivan Tarapov",
"Haris I Sair",
"Craig K Jones"
] | Resting State Networks (RSNs) of the brain extracted from Resting State
functional Magnetic Resonance Imaging (RS-fMRI) are used in the pre-surgical
planning to guide the neurosurgeon. This is difficult, though, as expert
knowledge is required to label each of the RSNs. There is a lack of efficient
and standardized methods to be used in clinical workflows. Additionally, these
methods need to be generalizable since the method needs to work well regardless
of the acquisition technique. We propose an accurate, fast, and lightweight
deep learning approach to label RSNs. Group Independent Component Analysis
(ICA) was used to extract large scale functional connectivity patterns in the
cohort and dual regression was used to back project them on individual subject
RSNs. We compare a Multi-Layer Perceptron (MLP) based method with 2D and 3D
Convolutional Neural Networks (CNNs) and find that the MLP is faster and more
accurate. The MLP method performs as good or better than other works despite
its compact size. We prove the generalizability of our method by showing that
the MLP performs at 100% accuracy in the holdout dataset and 98.3% accuracy in
three other sites' fMRI acquisitions. | [
"cs.LG",
"q-bio.NC"
] | false |
2305.03855 | 2023-05-05T21:43:00Z | Robust A-Optimal Experimental Design for Bayesian Inverse Problems | [
"Ahmed Attia",
"Sven Leyffer",
"Todd Munson"
] | Optimal design of experiments for Bayesian inverse problems has recently
gained wide popularity and attracted much attention, especially in the
computational science and Bayesian inversion communities. An optimal design
maximizes a predefined utility function that is formulated in terms of the
elements of an inverse problem, an example being optimal sensor placement for
parameter identification. The state-of-the-art algorithmic approaches following
this simple formulation generally overlook misspecification of the elements of
the inverse problem, such as the prior or the measurement uncertainties. This
work presents an efficient algorithmic approach for designing optimal
experimental design schemes for Bayesian inverse problems such that the optimal
design is robust to misspecification of elements of the inverse problem.
Specifically, we consider a worst-case scenario approach for the uncertain or
misspecified parameters, formulate robust objectives, and propose an
algorithmic approach for optimizing such objectives. Both relaxation and
stochastic solution approaches are discussed with detailed analysis and insight
into the interpretation of the problem and the proposed algorithmic approach.
Extensive numerical experiments to validate and analyze the proposed approach
are carried out for sensor placement in a parameter identification problem. | [
"math.OC",
"cs.LG",
"62K05, 35Q62, 62F15, 35R30, 35Q93, 65C60, 93E35"
] | false |
2305.04889 | 2023-05-05T14:34:20Z | Improving Real-Time Bidding in Online Advertising Using Markov Decision
Processes and Machine Learning Techniques | [
"Parikshit Sharma"
] | Real-time bidding has emerged as an effective online advertising technique.
With real-time bidding, advertisers can position ads per impression, enabling
them to optimise ad campaigns by targeting specific audiences in real-time.
This paper proposes a novel method for real-time bidding that combines deep
learning and reinforcement learning techniques to enhance the efficiency and
precision of the bidding process. In particular, the proposed method employs a
deep neural network to predict auction details and market prices and a
reinforcement learning algorithm to determine the optimal bid price. The model
is trained using historical data from the iPinYou dataset and compared to
cutting-edge real-time bidding algorithms. The outcomes demonstrate that the
proposed method is preferable regarding cost-effectiveness and precision. In
addition, the study investigates the influence of various model parameters on
the performance of the proposed algorithm. It offers insights into the efficacy
of the combined deep learning and reinforcement learning approach for real-time
bidding. This study contributes to advancing techniques and offers a promising
direction for future research. | [
"cs.IR",
"cs.LG"
] | false |
2305.05621 | 2023-05-05T16:22:17Z | Deep Learning-based Estimation for Multitarget Radar Detection | [
"Mamady Delamou",
"Ahmad Bazzi",
"Marwa Chafii",
"El Mehdi Amhoud"
] | Target detection and recognition is a very challenging task in a wireless
environment where a multitude of objects are located, whether to effectively
determine their positions or to identify them and predict their moves. In this
work, we propose a new method based on a convolutional neural network (CNN) to
estimate the range and velocity of moving targets directly from the
range-Doppler map of the detected signals. We compare the obtained results to
the two dimensional (2D) periodogram, and to the similar state of the art
methods, 2DResFreq and VGG-19 network and show that the estimation process
performed with our model provides better estimation accuracy of range and
velocity index in different signal to noise ratio (SNR) regimes along with a
reduced prediction time. Afterwards, we assess the performance of our proposed
algorithm using the peak signal to noise ratio (PSNR) which is a relevant
metric to analyse the quality of an output image obtained from compression or
noise reduction. Compared to the 2D-periodogram, 2DResFreq and VGG-19, we gain
33 dB, 21 dB and 10 dB, respectively, in terms of PSNR when SNR = 30 dB. | [
"eess.SP",
"cs.LG"
] | false |
2305.03257 | 2023-05-05T03:09:33Z | Data-driven and Physics Informed Modelling of Chinese Hamster Ovary Cell
Bioreactors | [
"Tianqi Cui",
"Tom S. Bertalan",
"Nelson Ndahiro",
"Pratik Khare",
"Michael Betenbaugh",
"Costas Maranas",
"Ioannis G. Kevrekidis"
] | Fed-batch culture is an established operation mode for the production of
biologics using mammalian cell cultures. Quantitative modeling integrates both
kinetics for some key reaction steps and optimization-driven metabolic flux
allocation, using flux balance analysis; this is known to lead to certain
mathematical inconsistencies. Here, we propose a physically-informed
data-driven hybrid model (a "gray box") to learn models of the dynamical
evolution of Chinese Hamster Ovary (CHO) cell bioreactors from process data.
The approach incorporates physical laws (e.g. mass balances) as well as kinetic
expressions for metabolic fluxes. Machine learning (ML) is then used to (a)
directly learn evolution equations (black-box modelling); (b) recover unknown
physical parameters ("white-box" parameter fitting) or -- importantly -- (c)
learn partially unknown kinetic expressions (gray-box modelling). We encode the
convex optimization step of the overdetermined metabolic biophysical system as
a differentiable, feed-forward layer into our architectures, connecting partial
physical knowledge with data-driven machine learning. | [
"q-bio.QM",
"cs.LG",
"math.DS"
] | false |
2305.03286 | 2023-05-05T05:02:41Z | Composite Motion Learning with Task Control | [
"Pei Xu",
"Xiumin Shang",
"Victor Zordan",
"Ioannis Karamouzas"
] | We present a deep learning method for composite and task-driven motion
control for physically simulated characters. In contrast to existing
data-driven approaches using reinforcement learning that imitate full-body
motions, we learn decoupled motions for specific body parts from multiple
reference motions simultaneously and directly by leveraging the use of multiple
discriminators in a GAN-like setup. In this process, there is no need of any
manual work to produce composite reference motions for learning. Instead, the
control policy explores by itself how the composite motions can be combined
automatically. We further account for multiple task-specific rewards and train
a single, multi-objective control policy. To this end, we propose a novel
framework for multi-objective learning that adaptively balances the learning of
disparate motions from multiple sources and multiple goal-directed control
objectives. In addition, as composite motions are typically augmentations of
simpler behaviors, we introduce a sample-efficient method for training
composite control policies in an incremental manner, where we reuse a
pre-trained policy as the meta policy and train a cooperative policy that
adapts the meta one for new composite tasks. We show the applicability of our
approach on a variety of challenging multi-objective tasks involving both
composite motion imitation and multiple goal-directed control. | [
"cs.GR",
"cs.AI",
"cs.LG"
] | true |
2305.03295 | 2023-05-05T05:50:07Z | Decentralized diffusion-based learning under non-parametric limited
prior knowledge | [
"Paweł Wachel",
"Krzysztof Kowalczyk",
"Cristian R. Rojas"
] | We study the problem of diffusion-based network learning of a nonlinear
phenomenon, $m$, from local agents' measurements collected in a noisy
environment. For a decentralized network and information spreading merely
between directly neighboring nodes, we propose a non-parametric learning
algorithm, that avoids raw data exchange and requires only mild \textit{a
priori} knowledge about $m$. Non-asymptotic estimation error bounds are derived
for the proposed method. Its potential applications are illustrated through
simulation experiments. | [
"stat.ML",
"cs.LG",
"cs.MA"
] | false |
2305.03331 | 2023-05-05T07:22:30Z | Generic and Robust Root Cause Localization for Multi-Dimensional Data in
Online Service Systems | [
"Zeyan Li",
"Junjie Chen",
"Yihao Chen",
"Chengyang Luo",
"Yiwei Zhao",
"Yongqian Sun",
"Kaixin Sui",
"Xiping Wang",
"Dapeng Liu",
"Xing Jin",
"Qi Wang",
"Dan Pei"
] | Localizing root causes for multi-dimensional data is critical to ensure
online service systems' reliability. When a fault occurs, only the measure
values within specific attribute combinations are abnormal. Such attribute
combinations are substantial clues to the underlying root causes and thus are
called root causes of multidimensional data. This paper proposes a generic and
robust root cause localization approach for multi-dimensional data, PSqueeze.
We propose a generic property of root cause for multi-dimensional data,
generalized ripple effect (GRE). Based on it, we propose a novel probabilistic
cluster method and a robust heuristic search method. Moreover, we identify the
importance of determining external root causes and propose an effective method
for the first time in literature. Our experiments on two real-world datasets
with 5400 faults show that the F1-score of PSqueeze outperforms baselines by
32.89%, while the localization time is around 10 seconds across all cases. The
F1-score in determining external root causes of PSqueeze achieves 0.90.
Furthermore, case studies in several production systems demonstrate that
PSqueeze is helpful to fault diagnosis in the real world. | [
"cs.SE",
"cs.LG",
"cs.PF"
] | false |
2305.03360 | 2023-05-05T08:23:56Z | A Survey on Offline Model-Based Reinforcement Learning | [
"Haoyang He"
] | Model-based approaches are becoming increasingly popular in the field of
offline reinforcement learning, with high potential in real-world applications
due to the model's capability of thoroughly utilizing the large historical
datasets available with supervised learning techniques. This paper presents a
literature review of recent work in offline model-based reinforcement learning,
a field that utilizes model-based approaches in offline reinforcement learning.
The survey provides a brief overview of the concepts and recent developments in
both offline reinforcement learning and model-based reinforcement learning, and
discuss the intersection of the two fields. We then presents key relevant
papers in the field of offline model-based reinforcement learning and discuss
their methods, particularly their approaches in solving the issue of
distributional shift, the main problem faced by all current offline model-based
reinforcement learning methods. We further discuss key challenges faced by the
field, and suggest possible directions for future work. | [
"cs.LG",
"cs.AI",
"cs.SY",
"eess.SY",
"I.2.6; I.2.8"
] | false |
2305.03365 | 2023-05-05T08:33:28Z | Repairing Deep Neural Networks Based on Behavior Imitation | [
"Zhen Liang",
"Taoran Wu",
"Changyuan Zhao",
"Wanwei Liu",
"Bai Xue",
"Wenjing Yang",
"Ji Wang"
] | The increasing use of deep neural networks (DNNs) in safety-critical systems
has raised concerns about their potential for exhibiting ill-behaviors. While
DNN verification and testing provide post hoc conclusions regarding unexpected
behaviors, they do not prevent the erroneous behaviors from occurring. To
address this issue, DNN repair/patch aims to eliminate unexpected predictions
generated by defective DNNs. Two typical DNN repair paradigms are retraining
and fine-tuning. However, existing methods focus on the high-level abstract
interpretation or inference of state spaces, ignoring the underlying neurons'
outputs. This renders patch processes computationally prohibitive and limited
to piecewise linear (PWL) activation functions to great extent. To address
these shortcomings, we propose a behavior-imitation based repair framework,
BIRDNN, which integrates the two repair paradigms for the first time. BIRDNN
corrects incorrect predictions of negative samples by imitating the closest
expected behaviors of positive samples during the retraining repair procedure.
For the fine-tuning repair process, BIRDNN analyzes the behavior differences of
neurons on positive and negative samples to identify the most responsible
neurons for the erroneous behaviors. To tackle more challenging domain-wise
repair problems (DRPs), we synthesize BIRDNN with a domain behavior
characterization technique to repair buggy DNNs in a probably approximated
correct style. We also implement a prototype tool based on BIRDNN and evaluate
it on ACAS Xu DNNs. Our experimental results show that BIRDNN can successfully
repair buggy DNNs with significantly higher efficiency than state-of-the-art
repair tools. Additionally, BIRDNN is highly compatible with different
activation functions. | [
"cs.LG",
"cs.AI",
"cs.SE",
"68N99, , 68T99",
"D.2.5; I.2.5"
] | false |
2305.03395 | 2023-05-05T09:40:28Z | Sparsifying Bayesian neural networks with latent binary variables and
normalizing flows | [
"Lars Skaaret-Lund",
"Geir Storvik",
"Aliaksandr Hubin"
] | Artificial neural networks (ANNs) are powerful machine learning methods used
in many modern applications such as facial recognition, machine translation,
and cancer diagnostics. A common issue with ANNs is that they usually have
millions or billions of trainable parameters, and therefore tend to overfit to
the training data. This is especially problematic in applications where it is
important to have reliable uncertainty estimates. Bayesian neural networks
(BNN) can improve on this, since they incorporate parameter uncertainty. In
addition, latent binary Bayesian neural networks (LBBNN) also take into account
structural uncertainty by allowing the weights to be turned on or off, enabling
inference in the joint space of weights and structures. In this paper, we will
consider two extensions to the LBBNN method: Firstly, by using the local
reparametrization trick (LRT) to sample the hidden units directly, we get a
more computationally efficient algorithm. More importantly, by using
normalizing flows on the variational posterior distribution of the LBBNN
parameters, the network learns a more flexible variational posterior
distribution than the mean field Gaussian. Experimental results show that this
improves predictive power compared to the LBBNN method, while also obtaining
more sparse networks. We perform two simulation studies. In the first study, we
consider variable selection in a logistic regression setting, where the more
flexible variational distribution leads to improved results. In the second
study, we compare predictive uncertainty based on data generated from
two-dimensional Gaussian distributions. Here, we argue that our Bayesian
methods lead to more realistic estimates of predictive uncertainty. | [
"stat.ML",
"cs.LG",
"stat.CO",
"stat.ME",
"62-02, 62-09, 62F07, 62F15, 62J12, 62J05, 62J99, 62M05, 05A16,\n 60J22, 92D20, 90C27, 90C59",
"G.1.2; G.1.6; G.2.1; G.3; I.2.0; I.2.6; I.2.8; I.5.1; I.6; I.6.4"
] | false |
2305.03474 | 2023-05-05T12:36:18Z | Zoo Guide to Network Embedding | [
"Anthony Baptista",
"Rubén J. Sánchez-García",
"Anaïs Baudot",
"Ginestra Bianconi"
] | Networks have provided extremely successful models of data and complex
systems. Yet, as combinatorial objects, networks do not have in general
intrinsic coordinates and do not typically lie in an ambient space. The process
of assigning an embedding space to a network has attracted lots of interest in
the past few decades, and has been efficiently applied to fundamental problems
in network inference, such as link prediction, node classification, and
community detection. In this review, we provide a user-friendly guide to the
network embedding literature and current trends in this field which will allow
the reader to navigate through the complex landscape of methods and approaches
emerging from the vibrant research activity on these subjects. | [
"cs.SI",
"cs.LG",
"math-ph",
"math.MP"
] | false |
2305.03547 | 2023-05-05T13:56:40Z | Over-the-Air Federated Averaging with Limited Power and Privacy Budgets | [
"Na Yan",
"Kezhi Wang",
"Cunhua Pan",
"Kok Keong Chai",
"Feng Shu",
"Jiangzhou Wang"
] | To jointly overcome the communication bottleneck and privacy leakage of
wireless federated learning (FL), this paper studies a differentially private
over-the-air federated averaging (DP-OTA-FedAvg) system with a limited sum
power budget. With DP-OTA-FedAvg, the gradients are aligned by an alignment
coefficient and aggregated over the air, and channel noise is employed to
protect privacy. We aim to improve the learning performance by jointly
designing the device scheduling, alignment coefficient, and the number of
aggregation rounds of federated averaging (FedAvg) subject to sum power and
privacy constraints. We first present the privacy analysis based on
differential privacy (DP) to quantify the impact of the alignment coefficient
on privacy preservation in each communication round. Furthermore, to study how
the device scheduling, alignment coefficient, and the number of the global
aggregation affect the learning process, we conduct the convergence analysis of
DP-OTA-FedAvg in the cases of convex and non-convex loss functions. Based on
these analytical results, we formulate an optimization problem to minimize the
optimality gap of the DP-OTA-FedAvg subject to limited sum power and privacy
budgets. The problem is solved by decoupling it into two sub-problems. Given
the number of communication rounds, we conclude the relationship between the
number of scheduled devices and the alignment coefficient, which offers a set
of potential optimal solution pairs of device scheduling and the alignment
coefficient. Thanks to the reduced search space, the optimal solution can be
efficiently obtained. The effectiveness of the proposed policy is validated
through simulations. | [
"cs.LG",
"cs.CR",
"cs.IT",
"math.IT"
] | false |
2305.03568 | 2023-05-05T14:19:46Z | A vector quantized masked autoencoder for audiovisual speech emotion
recognition | [
"Samir Sadok",
"Simon Leglaive",
"Renaud Séguier"
] | While fully-supervised models have been shown to be effective for audiovisual
speech emotion recognition (SER), the limited availability of labeled data
remains a major challenge in the field. To address this issue, self-supervised
learning approaches, such as masked autoencoders (MAEs), have gained popularity
as potential solutions. In this paper, we propose the VQ-MAE-AV model, a vector
quantized MAE specifically designed for audiovisual speech self-supervised
representation learning. Unlike existing multimodal MAEs that rely on the
processing of the raw audiovisual speech data, the proposed method employs a
self-supervised paradigm based on discrete audio and visual speech
representations learned by two pre-trained vector quantized variational
autoencoders. Experimental results show that the proposed approach, which is
pre-trained on the VoxCeleb2 database and fine-tuned on standard emotional
audiovisual speech datasets, outperforms the state-of-the-art audiovisual SER
methods. | [
"cs.SD",
"cs.LG",
"cs.MM",
"eess.AS"
] | false |
2305.03739 | 2023-05-05T01:54:38Z | Neural Architecture Search for Intel Movidius VPU | [
"Qian Xu",
"Victor Li",
"Crews Darren S"
] | Hardware-aware Neural Architecture Search (NAS) technologies have been
proposed to automate and speed up model design to meet both quality and
inference efficiency requirements on a given hardware. Prior arts have shown
the capability of NAS on hardware specific network design. In this whitepaper,
we further extend the use of NAS to Intel Movidius VPU (Vision Processor
Units). To determine the hardware-cost to be incorporated into the NAS process,
we introduced two methods: pre-collected hardware-cost on device and
device-specific hardware-cost model VPUNN. With the help of NAS, for
classification task on VPU, we can achieve 1.3x fps acceleration over
Mobilenet-v2-1.4 and 2.2x acceleration over Resnet50 with the same accuracy
score. For super resolution task on VPU, we can achieve 1.08x PSNR and 6x
higher fps compared with EDSR3. | [
"cs.NE",
"cs.AR",
"cs.LG"
] | false |
2305.03761 | 2023-05-05T18:00:09Z | Weakly-Supervised Anomaly Detection in the Milky Way | [
"Mariel Pettee",
"Sowmya Thanvantri",
"Benjamin Nachman",
"David Shih",
"Matthew R. Buckley",
"Jack H. Collins"
] | Large-scale astrophysics datasets present an opportunity for new machine
learning techniques to identify regions of interest that might otherwise be
overlooked by traditional searches. To this end, we use Classification Without
Labels (CWoLa), a weakly-supervised anomaly detection method, to identify cold
stellar streams within the more than one billion Milky Way stars observed by
the Gaia satellite. CWoLa operates without the use of labeled streams or
knowledge of astrophysical principles. Instead, we train a classifier to
distinguish between mixed samples for which the proportions of signal and
background samples are unknown. This computationally lightweight strategy is
able to detect both simulated streams and the known stream GD-1 in data.
Originally designed for high-energy collider physics, this technique may have
broad applicability within astrophysics as well as other domains interested in
identifying localized anomalies. | [
"astro-ph.GA",
"cs.LG",
"hep-ph",
"physics.data-an"
] | false |
2305.03797 | 2023-05-05T18:55:32Z | Materials Informatics: An Algorithmic Design Rule | [
"Bhupesh Bishnoi"
] | Materials informatics, data-enabled investigation, is a "fourth paradigm" in
materials science research after the conventional empirical approach,
theoretical science, and computational research. Materials informatics has two
essential ingredients: fingerprinting materials proprieties and the theory of
statistical inference and learning. We have researched the organic
semiconductor's enigmas through the materials informatics approach. By applying
diverse neural network topologies, logical axiom, and inferencing information
science, we have developed data-driven procedures for novel organic
semiconductor discovery for the semiconductor industry and knowledge extraction
for the materials science community. We have reviewed and corresponded with
various algorithms for the neural network design topology for the materials
informatics dataset. | [
"cond-mat.mtrl-sci",
"cond-mat.stat-mech",
"cs.LG",
"37E25, 46N30, 05C85, 60G25, 62C10, 62M20, 68Q32, 93E10",
"I.2.3; J.2; G.2.2; G.3"
] | false |
2305.03804 | 2023-05-05T19:13:00Z | Equivariant Neural Networks for Spin Dynamics Simulations of Itinerant
Magnets | [
"Yu Miyazaki"
] | I present a novel equivariant neural network architecture for the large-scale
spin dynamics simulation of the Kondo lattice model. This neural network mainly
consists of tensor-product-based convolution layers and ensures two
equivariances: translations of the lattice and rotations of the spins. I
implement equivariant neural networks for two Kondo lattice models on
two-dimensional square and triangular lattices, and perform training and
validation. In the equivariant model for the square lattice, the validation
error (based on root mean squared error) is reduced to less than one-third
compared to a model using invariant descriptors as inputs. Furthermore, I
demonstrate the ability to reproduce phase transitions of skyrmion crystals in
the triangular lattice, by performing dynamics simulations using the trained
model. | [
"cond-mat.str-el",
"cond-mat.dis-nn",
"cond-mat.mtrl-sci",
"cs.LG"
] | false |
2305.03835 | 2023-05-05T20:30:30Z | Spatiotemporal Transformer for Stock Movement Prediction | [
"Daniel Boyle",
"Jugal Kalita"
] | Financial markets are an intriguing place that offer investors the potential
to gain large profits if timed correctly. Unfortunately, the dynamic,
non-linear nature of financial markets makes it extremely hard to predict
future price movements. Within the US stock exchange, there are a countless
number of factors that play a role in the price of a company's stock, including
but not limited to financial statements, social and news sentiment, overall
market sentiment, political happenings and trading psychology. Correlating
these factors is virtually impossible for a human. Therefore, we propose STST,
a novel approach using a Spatiotemporal Transformer-LSTM model for stock
movement prediction. Our model obtains accuracies of 63.707 and 56.879 percent
against the ACL18 and KDD17 datasets, respectively. In addition, our model was
used in simulation to determine its real-life applicability. It obtained a
minimum of 10.41% higher profit than the S&P500 stock index, with a minimum
annualized return of 31.24%. | [
"cs.LG",
"cs.AI",
"cs.CE"
] | false |