|
--- |
|
license: mit |
|
tags: |
|
- biology |
|
- fish |
|
- images |
|
- traits |
|
pipeline_tag: image-segmentation |
|
--- |
|
# Model Card for Model ID |
|
|
|
This model takes in an image of a fish and segments out traits, as described [below](#Trait-List) |
|
|
|
## Model Details |
|
|
|
`Trained_model_SM.pth` is the fish segmentation model. |
|
|
|
`se_resnext50_32x4d-a260b3a4.pth` is a pretrained ConvNets for pytorch ResNeXt used by BGNN-trait-segmentation. |
|
See [github.com/Cadene/pretrained-models.pytorch#resnext](https://github.com/Cadene/pretrained-models.pytorch#resnext) for documentation about the source. |
|
|
|
The segmentation model was first trained on ImageNet ([Deng et al., 2009](https://doi.org/10.1109/CVPR.2009.5206848)), and then the model was fine-tuned on a specific set of image data relevant to the domain: [Illinois Natural History Survey Fish Collection](https://fish.inhs.illinois.edu/) (INHS Fish). |
|
The Feature Pyramid Network (FPN) architecture was used for fine-tuning, since it is a CNN-based architecture designed to handle multi-scale feature maps (Lin et al., 2017: [IEEE](https://doi.org/10.1109/CVPR.2017.106), [arXiv](https://doi.org/10.48550/arXiv.1612.03144)). |
|
The FPN uses SE-ResNeXt as the base network (Hu et al., 2018: [IEEE](https://doi.org/10.1109/CVPR.2018.00745), [arXiv](https://doi.org/10.48550/arXiv.1709.01507)). |
|
|
|
|
|
### Model Description |
|
|
|
PyTorch implementation of Fish trait segmentation model. |
|
This segmentation model is based on pretrained model using the [segementation models torch](https://github.com/qubvel/segmentation_models.pytorch). |
|
Then the model is fine tuned on fish images in order to identify (segment) the different traits. |
|
|
|
#### Trait list: |
|
``` |
|
background |
|
dorsal_fin |
|
adipos_fin |
|
caudal_fin |
|
anal_fin |
|
pelvic_fin |
|
pectoral_fin |
|
head |
|
eye |
|
caudal_fin_ray |
|
alt_fin_ray |
|
trunk |
|
``` |
|
|
|
|
|
- **Developed by:** M. Maruf and Anuj Karpatne |
|
<!--- **Shared by [optional]:** [More Information Needed]--> |
|
<!--- **Model type:** [More Information Needed]--> |
|
<!--- **Language(s) (NLP):** [More Information Needed]--> |
|
- **License:** MIT |
|
<!--- **Finetuned from model [optional]:** [More Information Needed]--> |
|
|
|
### Model Sources |
|
|
|
<!-- Provide the basic links for the model. --> |
|
|
|
- **Repository:** [BGNN-trait-segmentation](https://github.com/hdr-bgnn/BGNN-trait-segmentation) |
|
- **Paper:** [In progress] |
|
<!--- **Demo [optional]:** [More Information Needed]--> |
|
|
|
<!-- ## Uses |
|
|
|
<!-- Address questions around how the model is intended to be used, including the foreseeable users of the model and those affected by the model. --> |
|
|
|
<!-- ### Direct Use |
|
|
|
<!-- This section is for the model use without fine-tuning or plugging into a larger ecosystem/app. --> |
|
|
|
<!-- [More Information Needed] |
|
|
|
<!-- ### Downstream Use [optional] |
|
|
|
<!-- This section is for the model use when fine-tuned for a task, or when plugged into a larger ecosystem/app --> |
|
|
|
<!-- [More Information Needed] |
|
|
|
<!-- ### Out-of-Scope Use |
|
|
|
<!-- This section addresses misuse, malicious use, and uses that the model will not work well for. --> |
|
|
|
<!-- [More Information Needed] |
|
|
|
<!-- ## Bias, Risks, and Limitations |
|
|
|
<!-- This section is meant to convey both technical and sociotechnical limitations. --> |
|
|
|
<!-- [More Information Needed] --> |
|
|
|
<!-- ### Recommendations --> |
|
|
|
<!-- This section is meant to convey recommendations with respect to the bias, risk, and technical limitations. --> |
|
|
|
<!-- Users (both direct and downstream) should be made aware of the risks, biases and limitations of the model. More information needed for further recommendations. --> |
|
|
|
## How to Get Started with the Model |
|
|
|
<!--Use the code below to get started with the model.--> |
|
|
|
See instructions for use [here](https://github.com/hdr-bgnn/BGNN-trait-segmentation/blob/main/Segment_mini/README.md). |
|
|
|
## Training Details |
|
|
|
The image data were annotated using [SlicerMorph](https://slicermorph.github.io/) ([Rolfe et al., 2021](https://doi.org/10.1111/2041-210X.13669)) by collaborators W. Dahdul and K. Diamond. |
|
|
|
### Training Data |
|
|
|
<!-- This should link to a Data Card, perhaps with a short stub of information on what the training data is all about as well as documentation related to data pre-processing or additional filtering. --> |
|
|
|
<!--We only have 295 training images, 99 testing images, and 98 validation images. --> |
|
To increase the size and diversity of the training dataset (originally 295 images), we employed data augmentation techniques such as flipping, shifting, rotating, scaling, and adding noise to the original image data to increase the dataset 10-fold. |
|
We developed 12 target classes, or trait masks, for our segmentation problem, each representing different morphological traits of a fish specimen. |
|
The segmentation classes are: dorsal fin, adipose fin, caudal fin, anal fin, pelvic fin, pectoral fin, head minus the eye, eye, caudal fin-ray, alt fin-ray, alt fin-spine, and trunk. |
|
Although minnows do not have adipose fins, the segmentation model was trained on a variety of fish image data, some of which had adipose fins. |
|
We retained this class because the segmentation model may erroneously assign an adipose fin to a minnow (Fig. S1), and a domain scientist examining these outputs may want to analyze the accuracy of the model. |
|
|
|
|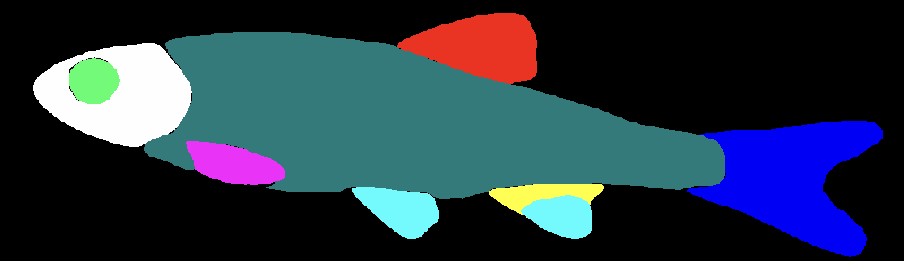| |
|
|:--| |
|
|**Figure S1.** [Image of a segmented minnow](https://huggingface.co/imageomics/BGNN-trait-segmentation/raw/main/Fig_S1.png) with an erroneously marked anal fin. |
|
The segmentation has 13 classes: background (black), dorsal fin (red), adipose fin (green), caudal fin (blue), anal fin (yellow), pelvic fin (light blue), pectoral fin (pink), head minus the eye (white), eye (bright green), trunk (teal), caudal fin-ray (light red), alt fin-ray (light pink), and alt fin-spine (peach).| |
|
|
|
The training dataset utilized the image files listed in [training_dataset_INHS.txt](https://huggingface.co/imageomics/BGNN-trait-segmentation/blob/main/training_dataset_INHS.txt). |
|
|
|
The validation dataset utilized the image files listed in [validation_dataset_INHS.txt](https://huggingface.co/imageomics/BGNN-trait-segmentation/blob/main/validation_dataset_INHS.txt). |
|
|
|
### Training Procedure |
|
|
|
<!-- This relates heavily to the Technical Specifications. Content here should link to that section when it is relevant to the training procedure. --> |
|
|
|
We prepared the model by using the [Segmentation Model PyTorch library](https://segmentation-modelspytorch.readthedocs.io/en/latest/) (Iakubovskii, 2019) to load an FPN segmentation model that was pretrained on the Imagenet dataset. |
|
We used SE-ResNeXt as the base network/encoder to extract features (embedding) from the input image data and replaced the last decoder layer with 12 target classes. |
|
During the fine-tuning procedure, the encoder of the pre-trained model was frozen as these layers already contain useful features that we can leverage. |
|
We only tuned the decoder weights of our segmentation model during this fine-tuning procedure. |
|
|
|
We then trained the prepared model for 120 epochs, updating the weights using dice loss as a measure of similarity between the predicted and ground-truth segmentation. |
|
The Adam optimizer ([Kingma & Ba, 2014](https://doi.org/10.48550/arXiv.1412.6980)) with a small learning rate (1e-4) was used to update the model weights. |
|
|
|
|
|
<!-- #### Preprocessing [optional] |
|
|
|
<!-- [More Information Needed] |
|
|
|
|
|
<!-- #### Training Hyperparameters |
|
|
|
<!-- - **Training regime:** [More Information Needed] <!--fp32, fp16 mixed precision, bf16 mixed precision, bf16 non-mixed precision, fp16 non-mixed precision, fp8 mixed precision --> |
|
|
|
<!-- #### Speeds, Sizes, Times [optional] |
|
|
|
<!-- This section provides information about throughput, start/end time, checkpoint size if relevant, etc. --> |
|
|
|
<!-- [More Information Needed] --> |
|
|
|
## Evaluation |
|
|
|
<!-- This section describes the evaluation protocols and provides the results. --> |
|
We evaluated the performance of the fine-tuned segmentation model on the test set using the Intersection over Union (IoU) score with a 0.5 threshold. |
|
(The IoU score ranges from 0 to 1, with 1 indicating a perfect overlap between the predicted segmentation and the ground-truth segmentation and 0 indicating no overlap.) |
|
Our segmentation model achieved a 0.90 mIoU score on the test dataset. |
|
|
|
### Testing Data, Factors & Metrics |
|
|
|
We had 99 testing images and 98 validation images. |
|
|
|
<!-- #### Testing Data |
|
|
|
<!-- This should link to a Data Card if possible. --> |
|
|
|
<!-- [More Information Needed] |
|
|
|
<!-- #### Factors |
|
|
|
<!-- These are the things the evaluation is disaggregating by, e.g., subpopulations or domains. --> |
|
|
|
<!-- [More Information Needed] |
|
|
|
<!-- #### Metrics |
|
|
|
<!-- These are the evaluation metrics being used, ideally with a description of why. --> |
|
|
|
<!-- [More Information Needed] |
|
|
|
<!-- ### Results |
|
|
|
<!-- [More Information Needed] |
|
|
|
|
|
|
|
<!-- #### Summary |
|
|
|
|
|
|
|
<!-- ## Model Examination [optional] |
|
|
|
<!-- Relevant interpretability work for the model goes here --> |
|
|
|
<!-- [More Information Needed] |
|
|
|
<!-- ## Environmental Impact |
|
|
|
<!-- Total emissions (in grams of CO2eq) and additional considerations, such as electricity usage, go here. Edit the suggested text below accordingly --> |
|
|
|
<!-- Carbon emissions can be estimated using the [Machine Learning Impact calculator](https://mlco2.github.io/impact#compute) presented in [Lacoste et al. (2019)](https://doi.org/10.48550/arXiv.1910.09700). |
|
|
|
- **Hardware Type:** [More Information Needed] |
|
- **Hours used:** [More Information Needed] |
|
- **Cloud Provider:** [More Information Needed] |
|
- **Compute Region:** [More Information Needed] |
|
- **Carbon Emitted:** [More Information Needed] --> |
|
|
|
<!-- ## Technical Specifications [optional] |
|
|
|
<!-- ### Model Architecture and Objective |
|
|
|
<!-- [More Information Needed] |
|
|
|
<!-- ### Compute Infrastructure |
|
|
|
<!-- [More Information Needed] |
|
|
|
<!-- #### Hardware |
|
|
|
<!-- [More Information Needed] |
|
|
|
<!-- #### Software |
|
|
|
<!-- [More Information Needed] --> |
|
|
|
<!-- ## Citation [optional] |
|
|
|
<!-- If there is a paper or blog post introducing the model, the APA and Bibtex information for that should go in this section. --> |
|
<!-- M. Maruf, 2023, "BGNN-trait-segmentation", DOI coming with model soon for paper citation --> |
|
<!-- **BibTeX:** |
|
|
|
[More Information Needed] |
|
|
|
**APA:** |
|
|
|
[More Information Needed] --> |
|
|
|
<!-- ## Glossary [optional] |
|
|
|
<!-- If relevant, include terms and calculations in this section that can help readers understand the model or model card. --> |
|
|
|
<!-- [More Information Needed] --> |
|
|
|
## More Information |
|
|
|
Research supported by NSF Office of Advanced Cyberinfrastructure (OAC) Awards [#2022042](https://www.nsf.gov/awardsearch/showAward?AWD_ID=2022042&HistoricalAwards=false), [#1940233](https://nsf.gov/awardsearch/showAward?AWD_ID=1940233&HistoricalAwards=false), [#1940322](https://www.nsf.gov/awardsearch/showAward?AWD_ID=1940322&HistoricalAwards=false), and [#1940247](https://www.nsf.gov/awardsearch/showAward?AWD_ID=1940247&HistoricalAwards=false), with additional support from NSF [Award #2118240](https://www.nsf.gov/awardsearch/showAward?AWD_ID=2118240). |
|
Any opinions, findings and conclusions or recommendations expressed in this material are those of the author(s) and do not necessarily reflect the views of the National Science Foundation. |
|
|
|
<!-- ## Model Card Authors [optional] |
|
|
|
[More Information Needed] |
|
|
|
## Model Card Contact |
|
|
|
[More Information Needed] --> |
|
|
|
|
|
|