metadata
{}
Kosmos-2: Grounding Multimodal Large Language Models to the World
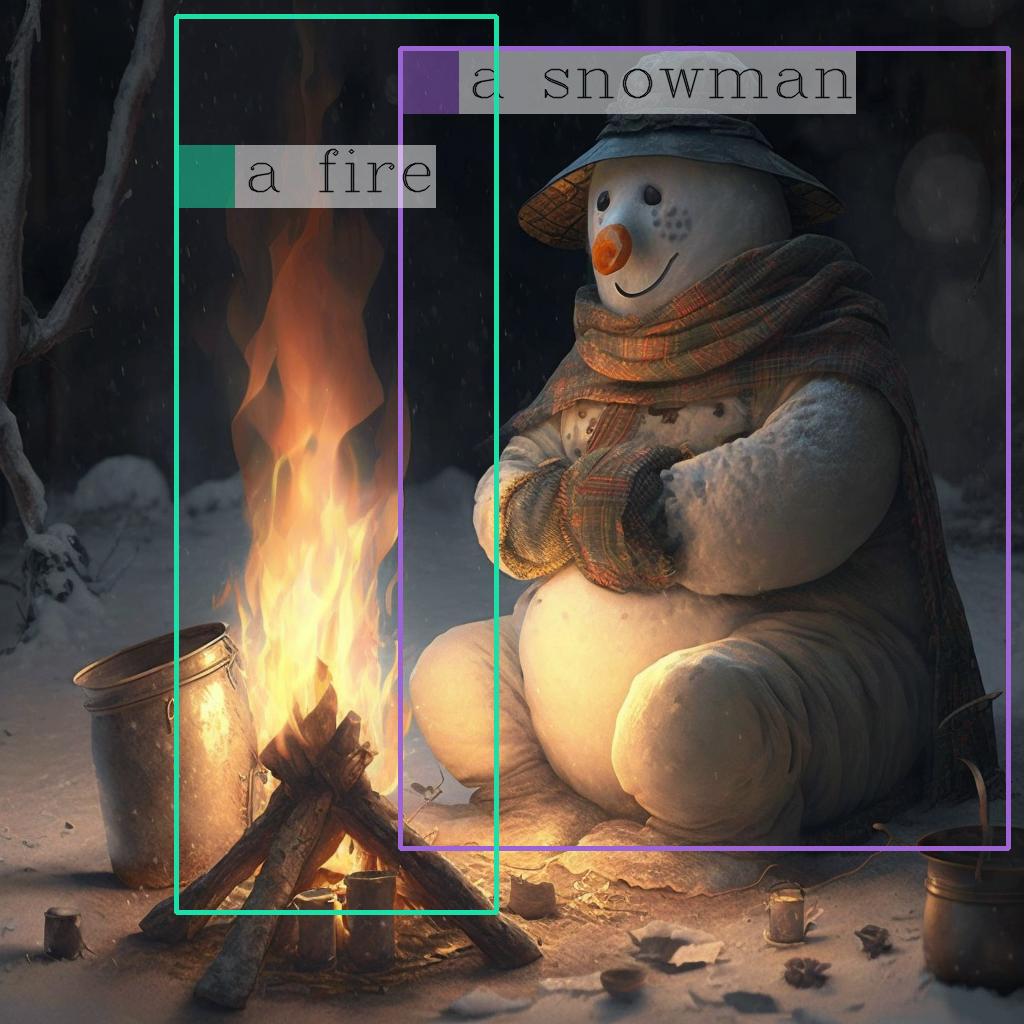
This Hub repository contains a HuggingFace's transformers
implementation of the original Kosmos-2 model from Microsoft.
How to Get Started with the Model
Use the code below to get started with the model.
import requests
from PIL import Image
from transformers import AutoProcessor, AutoModelForVision2Seq
model = AutoModelForVision2Seq.from_pretrained("microsoft/kosmos-2-patch14-224")
processor = AutoProcessor.from_pretrained("microsoft/kosmos-2-patch14-224")
prompt = "<grounding>An image of"
url = "https://huggingface.co/microsoft/kosmos-2-patch14-224/resolve/main/snowman.png"
image = Image.open(requests.get(url, stream=True).raw)
# The original Kosmos-2 demo saves the image first then reload it. For some images, this will give slightly different image input and change the generation outputs.
image.save("new_image.jpg")
image = Image.open("new_image.jpg")
inputs = processor(text=prompt, images=image, return_tensors="pt", add_eos_token=False)
generated_ids = model.generate(
pixel_values=inputs["pixel_values"],
input_ids=inputs["input_ids"],
attention_mask=inputs["attention_mask"],
image_embeds=None,
image_embeds_position_mask=inputs["image_embeds_position_mask"],
use_cache=True,
max_new_tokens=128,
)
generated_text = processor.batch_decode(generated_ids, skip_special_tokens=True)[0]
# Specify `cleanup_and_extract=False` in order to see the raw model generation.
processed_text = processor.post_process_generation(generated_text, cleanup_and_extract=False)
print(processed_text)
# `<grounding> An image of<phrase> a snowman</phrase><object><patch_index_0044><patch_index_0863></object> warming himself by<phrase> a fire</phrase><object><patch_index_0005><patch_index_0911></object>.`
# By default, the generated text is cleanup and the entities are extracted.
processed_text, entities = processor.post_process_generation(generated_text)
print(processed_text)
# `An image of a snowman warming himself by a fire.`
print(entities)
# `[('a snowman', (12, 21), [(0.390625, 0.046875, 0.984375, 0.828125)]), ('a fire', (41, 47), [(0.171875, 0.015625, 0.484375, 0.890625)])]`
Tasks
This model is capable of performing different tasks through changing the prompts.
First, let's define a function to run a prompt.
import requests
from PIL import Image
from transformers import AutoProcessor, AutoModelForVision2Seq
model = AutoModelForVision2Seq.from_pretrained("microsoft/kosmos-2-patch14-224")
processor = AutoProcessor.from_pretrained("microsoft/kosmos-2-patch14-224")
url = "https://huggingface.co/microsoft/kosmos-2-patch14-224/resolve/main/snowman.png"
image = Image.open(requests.get(url, stream=True).raw)
def run_example(prompt):
inputs = processor(text=prompt, images=image, return_tensors="pt", add_eos_token=False)
generated_ids = model.generate(
pixel_values=inputs["pixel_values"],
input_ids=inputs["input_ids"],
attention_mask=inputs["attention_mask"],
image_embeds=None,
image_embeds_position_mask=inputs["image_embeds_position_mask"],
use_cache=True,
max_new_tokens=128,
)
generated_text = processor.batch_decode(generated_ids, skip_special_tokens=True)[0]
_processed_text = processor.post_process_generation(generated_text, cleanup_and_extract=False)
processed_text, entities = processor.post_process_generation(generated_text)
print(processed_text)
print(entities)
print(_processed_text)
Here are the tasks Kosmos-2
could perform:
Multimodal Grounding
• Phrase Grounding
prompt = "<grounding><phrase> a snowman</phrase>"
run_example(prompt)
# a snowman is warming himself by the fire
# [('a snowman', (0, 9), [(0.390625, 0.046875, 0.984375, 0.828125)]), ('the fire', (32, 40), [(0.203125, 0.015625, 0.453125, 0.859375)])]
# <grounding><phrase> a snowman</phrase><object><patch_index_0044><patch_index_0863></object> is warming himself by<phrase> the fire</phrase><object><patch_index_0006><patch_index_0878></object>
• Referring Expression Comprehension
prompt = "<grounding><phrase> a snowman next to a fire</phrase>"
run_example(prompt)
# a snowman next to a fire
# [('a snowman next to a fire', (0, 24), [(0.390625, 0.046875, 0.984375, 0.828125)])]
# <grounding><phrase> a snowman next to a fire</phrase><object><patch_index_0044><patch_index_0863></object>
Multimodal Referring
• Referring expression generation
prompt = "<grounding><phrase> It</phrase><object><patch_index_0044><patch_index_0863></object> is"
run_example(prompt)
# It is snowman in a hat and scarf
# [('It', (0, 2), [(0.390625, 0.046875, 0.984375, 0.828125)])]
# <grounding><phrase> It</phrase><object><patch_index_0044><patch_index_0863></object> is snowman in a hat and scarf
Perception-Language Tasks
• Grounded VQA
prompt = "<grounding> Question: What is special about this image? Answer:"
run_example(prompt)
# Question: What is special about this image? Answer: The image features a snowman sitting by a campfire in the snow.
# [('a snowman', (71, 80), [(0.390625, 0.046875, 0.984375, 0.828125)]), ('a campfire', (92, 102), [(0.109375, 0.640625, 0.546875, 0.984375)])]
# <grounding> Question: What is special about this image? Answer: The image features<phrase> a snowman</phrase><object><patch_index_0044><patch_index_0863></object> sitting by<phrase> a campfire</phrase><object><patch_index_0643><patch_index_1009></object> in the snow.
• Grounded VQA with multimodal referring via bounding boxes
prompt = "<grounding> Question: Where is<phrase> the fire</phrase><object><patch_index_0005><patch_index_0911></object> next to? Answer:"
run_example(prompt)
# Question: Where is the fire next to? Answer: Near the snowman.
# [('the fire', (19, 27), [(0.171875, 0.015625, 0.484375, 0.890625)]), ('the snowman', (50, 61), [(0.390625, 0.046875, 0.984375, 0.828125)])]
# <grounding> Question: Where is<phrase> the fire</phrase><object><patch_index_0005><patch_index_0911></object> next to? Answer: Near<phrase> the snowman</phrase><object><patch_index_0044><patch_index_0863></object>.
Grounded Image captioning
• Brief
prompt = "<grounding> An image of"
run_example(prompt)
# An image of a snowman warming himself by a campfire.
# [('a snowman', (12, 21), [(0.390625, 0.046875, 0.984375, 0.828125)]), ('a campfire', (41, 51), [(0.109375, 0.640625, 0.546875, 0.984375)])]
# <grounding> An image of<phrase> a snowman</phrase><object><patch_index_0044><patch_index_0863></object> warming himself by<phrase> a campfire</phrase><object><patch_index_0643><patch_index_1009></object>.
• Detailed
prompt = "<grounding> Describe this image in detail:"
run_example(prompt)
# Describe this image in detail: The image features a snowman sitting by a campfire in the snow. He is wearing a hat, scarf, and gloves, with a pot nearby and a cup nearby. The snowman appears to be enjoying the warmth of the fire, and it appears to have a warm and cozy atmosphere.
# [('a campfire', (71, 81), [(0.171875, 0.015625, 0.484375, 0.984375)]), ('a hat', (109, 114), [(0.515625, 0.046875, 0.828125, 0.234375)]), ('scarf', (116, 121), [(0.515625, 0.234375, 0.890625, 0.578125)]), ('gloves', (127, 133), [(0.515625, 0.390625, 0.640625, 0.515625)]), ('a pot', (140, 145), [(0.078125, 0.609375, 0.265625, 0.859375)]), ('a cup', (157, 162), [(0.890625, 0.765625, 0.984375, 0.984375)])]
# <grounding> Describe this image in detail: The image features a snowman sitting by<phrase> a campfire</phrase><object><patch_index_0005><patch_index_1007></object> in the snow. He is wearing<phrase> a hat</phrase><object><patch_index_0048><patch_index_0250></object>,<phrase> scarf</phrase><object><patch_index_0240><patch_index_0604></object>, and<phrase> gloves</phrase><object><patch_index_0400><patch_index_0532></object>, with<phrase> a pot</phrase><object><patch_index_0610><patch_index_0872></object> nearby and<phrase> a cup</phrase><object><patch_index_0796><patch_index_1023></object> nearby. The snowman appears to be enjoying the warmth of the fire, and it appears to have a warm and cozy atmosphere.